RIT Research Computing supplies advanced research technology resources and support to researchers in their quest to discover.
On this site you will find a list of scholarly publications that utilized RIT Research Computing services, including the High-Performance Computing (HPC) cluster, Spack software packages/environments, Ceph storage, file shares, virtual machines, REDCap (https://redcap.rc.rit.edu/), Mirrors (https://mirrors.rit.edu/), GitLab (https://git.rc.rit.edu/ – formerly https://kgcoe-git.rit.edu), and consultation (debugging, workflow optimization, grant proposals, data management plans).
Statistics
Since 2016, RIT researchers have published at least 526 scholarly works with support from Research Computing. The uptick in usage in 2019 correlates with the execution of the 2018 Research Computing Services Proposal, notably the installation of the current HPC cluster, SPORC, in late 2018.
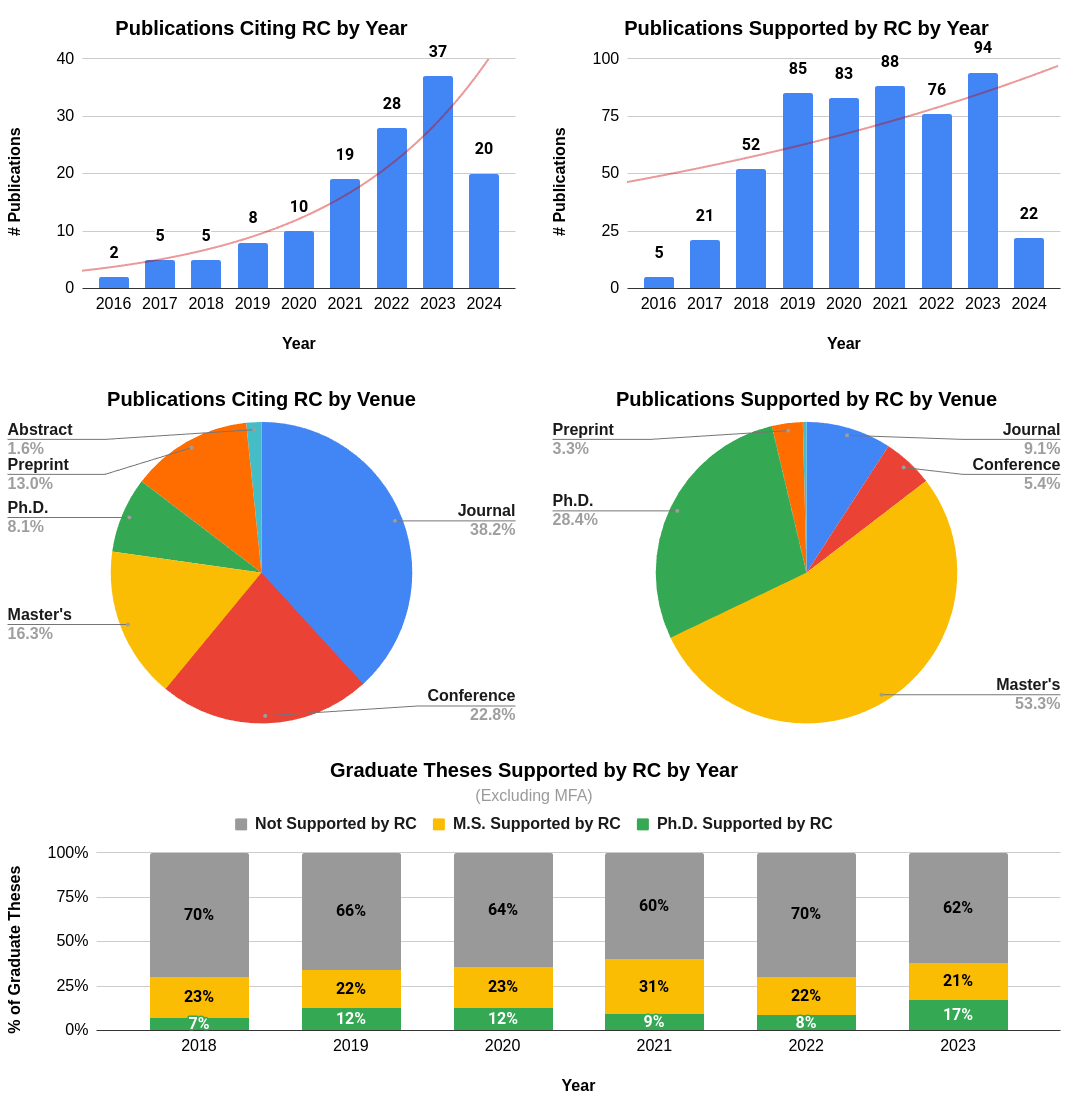
Notes:
- Publications are reported by calendar year, not fiscal or academic year
- Master’s Thesis data for 2016-2017 is still being processed
Data Sources
This data was collected from multiple sources:
- Graduate Theses published on repository.rit.edu and/or ProQuest cross-referenced with internal logs of Cluster, Spack, Storage, Virtual Machine, GitLab, REDCap, and Mirrors usage
- Publications that cite Research Computing’s DOI number
- Search queries run against Google Scholar
- Publications self-reported by RIT researchers using this form
Citation & Acknowledgment
Example Acknowledgment
The authors acknowledge Research Computing at the Rochester Institute of Technology for providing computational resources and support that have contributed to the research results reported in this publication.
Citation
Rochester Institute of Technology. Research Computing Services. Rochester Institute of Technology. https://doi.org/10.34788/0S3G-QD15
Bibtex:
@misc{https://doi.org/10.34788/0s3g-qd15,
doi = {10.34788/0S3G-QD15},
url = {https://www.rit.edu/researchcomputing/},
author = {Rochester Institute of Technology},
title = {Research Computing Services},
publisher = {Rochester Institute of Technology},
year = {<YEAR_OF_SUPPORT>}
}
RIS:
T1 - Research Computing Services
AU - Rochester Institute of Technology
DO - 10.34788/0S3G-QD15
UR - https://www.rit.edu/researchcomputing/
AB - RIT Research Computing supplies advanced research technology resources and support to researchers in their quest to discover.
PY - <YEAR_OF_SUPPORT>
PB - Rochester Institute of Technology
ER -
Contact Us
If you are an RIT researcher who has utlized Research Computing services in pursuit of a publication, please fill out this survey to tell us about your publication so we can add it to this site. Thank you and congratulations!
If you notice an error on this site, please submit a ticket or contact us on Slack.
Journal Papers
[J-2024-08] Mathews, Nate and Holland, James K and Hopper, Nicholas and Wright, Matthew. (2024). “LASERBEAK: Evolving Website Fingerprinting Attacks with Attention and Multi-Channel Feature Representation” Transactions on Information Forensics and Security.
Abstract: In this paper, we present Laserbeak, a new state-of-the-art website fingerprinting attack for Tor that achieves nearly 96% accuracy against FRONT-defended traffic by combining two innovations: 1) multi-channel traffic representations and 2) advanced techniques adapted from state-of-the-art computer vision models. Our work is the first to explore a range of different ways to represent traffic data for a classifier. We find a multi-channel input format that provides richer contextual information, enabling the model to learn robust representations even in the presence of heavy traffic obfuscation. We are also the first to examine how recent advances in transformer models can take advantage of these representations. Our novel model architecture utilizing multi-headed attention layers enhances the capture of both local and global patterns. By combining these innovations, Laserbeak demonstrates absolute performance improvements of up to 36.2% (e.g., from 27.6% to 63.8%) compared with prior attacks against defended traffic. Experiments highlight Laserbeak’s capabilities in multiple scenarios, including a large open-world dataset where it achieves over 80% recall at 99% precision on traffic obfuscated with padding defenses. These advances reduce the remaining anonymity in Tor against fingerprinting threats, underscoring the need for stronger defenses.
Publisher Site: IEEE Xplore
PDF (Requires Sign-In): PDF
Click for Bibtex
@article{mathews2024laserbeak, title = {LASERBEAK: Evolving Website Fingerprinting Attacks with Attention and Multi-Channel Feature Representation}, author = {Mathews, Nate and Holland, James K and Hopper, Nicholas and Wright, Matthew}, journal = {IEEE Transactions on Information Forensics and Security}, year = {2024}, publisher = {IEEE} }
[J-2024-07] Kasarabada, Viswateja and Nasir Ahamed, Nuzhet Nihaar and Vaghef-Koodehi, Alaleh and Martinez-Martinez, Gabriela and Lapizco-Encinas, Blanca H. (2024). “Separating the Living from the Dead: An Electrophoretic Approach.” Analytical Chemistry.
Abstract: Cell viability studies are essential in numerous applications, including drug development, clinical analysis, bioanalytical assessments, food safety, and environmental monitoring. Microfluidic electrokinetic (EK) devices have been proven to be effective platforms to discriminate microorganisms by their viability status. Two decades ago, live and dead Escherichia coli (E. coli) cells were trapped at distinct locations in an insulator-based EK (iEK) device with cylindrical insulating posts. At that time, the discrimination between live and dead cells was attributed to dielectrophoretic effects. This study presents the continuous separation between the live and dead E. coli cells, which was achieved primarily by combining linear and nonlinear electrophoretic effects in an iEK device. First, live and dead E. coli cells were characterized in terms of their electrophoretic migration, and then the properties of both live and dead E. coli cells were input into a mathematical model built using COMSOL Multiphysics software to identify appropriate voltages for performing an iEK separation in a T-cross iEK channel. Subsequently, live and dead cells were successfully separated experimentally in the form of an electropherogram, achieving a separation resolution of 1.87. This study demonstrated that linear and nonlinear electrophoresis phenomena are responsible for the discrimination between live and dead cells under DC electric fields in iEK devices. Continuous electrophoretic assessments, such as the one presented here, can be used to discriminate between distinct types of microorganisms including live and dead cell assessments.
Publisher Site: ACS
PDF (Requires Sign-In): PDF
Click for Bibtex
@article{kasarabada2024separating, title = {Separating the Living from the Dead: An Electrophoretic Approach}, author = {Kasarabada, Viswateja and Nasir Ahamed, Nuzhet Nihaar and Vaghef-Koodehi, Alaleh and Martinez-Martinez, Gabriela and Lapizco-Encinas, Blanca H}, journal = {Analytical Chemistry}, year = {2024}, publisher = {ACS Publications} }
[J-2024-06] Uche, Obioma U. (2024). “A DFT Analysis of the Cuboctahedral to Icosahedral Transformation of Gold-Silver Nanoparticles.” Computational Materials Science.
Abstract: In the current work, we investigate the transformation mechanics of gold-silver nanoparticles with cuboctahedral and icosahedral geometries by varying relevant attributes including size, composition, morphology, and chemical order. Our findings reveal that the transformation occurs via a martensitic, symmetric mechanism, irrespective of the specific attributes for all nanoparticles under consideration. The associated transformation barriers are observed to be strongly dependent on both size and composition as the activation energies increase with higher silver content. The chemical order is also a significant factor for determining how readily the transformation occurs since core–shell nanoparticles with gold exteriors display higher barriers in comparison to their silver counterparts. Likewise, for a given composition, core–shell morphologies indicate reduced ease of transformation relative to alloy nanoparticles.
Publisher Site: ScienceDirect
PDF (Requires Sign-In): PDF
Click for Bibtex
@article{uche2024dft, title = {A DFT analysis of the cuboctahedral to icosahedral transformation of gold-silver nanoparticles}, author = {Uche, Obioma U}, journal = {Computational Materials Science}, volume = {244}, pages = {113262}, year = {2024}, publisher = {Elsevier} }
[J-2024-05] Mathew, Roshan and Dannels, Wendy A. (2024). “Transforming Language Access for Deaf Patients in Healthcare.” The Journal on Technology and Persons with Disabilities.
Abstract: Deaf patients often depend on sign language interpreters and real-time captioners for communication during healthcare appointments. While they prefer in-person services, the scarcity of local qualified professionals and the faster turnaround times of remote options like Video Remote Interpreting (VRI) and remote captioning often lead hospitals and clinics to choose these alternatives. These remote methods often cause visibility and cognitive challenges for deaf patients, as they are forced to split their focus between the VRI or captioning screen and the healthcare provider, hindering their comprehension of medical information. This study proposes the use of augmented reality (AR) smart glasses as an alternative to traditional VRI and remote captioning methods. Certified healthcare interpreters and captioners are key stakeholders in improving communication for deaf patients in medical settings. Therefore, this study explores the perspectives of 25 interpreters and 22 captioners regarding adopting an AR smart glasses application to facilitate language accessibility in healthcare contexts. The findings indicate that interpreters prefer in-person services but recognize the advantages of using a smart glasses application when in-person services are not feasible. In contrast, captioners show a strong inclination toward the smart glasses application over traditional captioning techniques.
Publisher Site: Calstate
PDF (Requires Sign-In): PDF
Click for Bibtex
@article{mathew2024transforming, title = {Transforming Language Access for Deaf Patients in Healthcare}, author = {Mathew, Roshan and Dannels, Wendy A}, journal = {The Journal on Technology and Persons with Disabilities}, pages = {277}, year = {2024} }
[J-2024-04] Nasir Ahamed, Nuzhet Nihaar and Mendiola-Escobedo, Carlos A. and Perez-Gonzalez, Victor H. and Lapizco-Encinas, Blanca H. (2024). “Development of a DC-Biased AC-Stimulated Microfluidic Device for the Electrokinetic Separation of Bacterial and Yeast Cells.” Biosensors.
Abstract: Electrokinetic (EK) microsystems, which are capable of performing separations without the need for labeling analytes, are a rapidly growing area in microfluidics. The present work demonstrated three distinct binary microbial separations, computationally modeled and experimentally performed, in an insulator-based EK (iEK) system stimulated by DC-biased AC potentials. The separations had an increasing order of difficulty. First, a separation between cells of two distinct domains (Escherichia coli and Saccharomyces cerevisiae) was demonstrated. The second separation was for cells from the same domain but different species (Bacillus subtilis and Bacillus cereus). The last separation included cells from two closely related microbial strains of the same domain and the same species (two distinct S. cerevisiae strains). For each separation, a novel computational model, employing a continuous spatial and temporal function for predicting the particle velocity, was used to predict the retention time (tR,p) of each cell type, which aided the experimentation. All three cases resulted in separation resolution values Rs>1.5, indicating complete separation between the two cell species, with good reproducibility between the experimental repetitions (deviations < 6%) and good agreement (deviations < 18%) between the predicted tR,p and experimental (tR,e) retention time values. This study demonstrated the potential of DC-biased AC iEK systems for performing challenging microbial separations.
Publisher Site: MDPI
PDF (Requires Sign-In): PDF
Click for Bibtex
@article{nasir2024development, title = {Development of a DC-Biased AC-Stimulated Microfluidic Device for the Electrokinetic Separation of Bacterial and Yeast Cells}, author = {Nasir Ahamed, Nuzhet Nihaar and Mendiola-Escobedo, Carlos A and Perez-Gonzalez, Victor H and Lapizco-Encinas, Blanca H}, journal = {Biosensors}, volume = {14}, number = {5}, pages = {237}, year = {2024}, publisher = {MDPI} }
[J-2024-03] Chowdhury, Md Towhidul Absar and Contractor, Maheen Riaz and Rivero, Carlos R. (2024). “Flexible Control Flow Graph Alignment for Delivering Data-Driven Feedback to Novice Programming Learners.” Journal of Systems and Software.
Abstract: Supporting learners in introductory programming assignments at scale is a necessity. This support includes automated feedback on what learners did incorrectly. Existing approaches cast the problem as automatically repairing learners' incorrect programs extrapolating the data from an existing correct program from other learners. However, such approaches are limited because they only compare programs with similar control flow and order of statements. A potentially valuable set of repair feedback from flexible comparisons is thus missing. In this paper, we present several modifications to CLARA, a data-driven automated repair approach that is open source, to deal with real-world introductory programs. We extend CLARA's abstract syntax tree processor to handle common introductory programming constructs. Additionally, we propose a flexible alignment algorithm over control flow graphs where we enrich nodes with semantic annotations extracted from programs using operations and calls. Using this alignment, we modify an incorrect program's control flow graph to match correct programs to apply CLARA's original repair process. We evaluate our approach against a baseline on the twenty most popular programming problems in Codeforces. Our results indicate that flexible alignment has a significantly higher percentage of successful repairs at 46% compared to 5% for baseline CLARA. Our implementation is available at https://github.com/towhidabsar/clara
Publisher Site: ScienceDirect
PDF (Requires Sign-In): PDF
Click for Bibtex
@article{chowdhury2024flexible, title = {Flexible control flow graph alignment for delivering data-driven feedback to novice programming learners}, author = {Chowdhury, Md Towhidul Absar and Contractor, Maheen Riaz and Rivero, Carlos R}, journal = {Journal of Systems and Software}, volume = {210}, pages = {111960}, year = {2024}, publisher = {Elsevier} }
[J-2024-02] Ko, Eunmi. (2024). “An Affine Term Structure Model with Fed Chairs’ Speeches.” Finance Research Letters.
Abstract: I analyze the impact of the sentiment expressed in speeches by Fed chairs on Treasury bond yields, using an affine term structure model. The speeches by Fed chairs are numerically evaluated to generate three sentiment factors: negative, neutral, and positive. The variance decomposition analysis indicates that sentiment factors account for a considerable portion of the variance in yield forecasts.
Publisher Site: ScienceDirect
PDF (Requires Sign-In): PDF
Click for Bibtex
@article{ko2024affine, title = {An affine term structure model with fed chairs' speeches}, author = {Ko, Eunmi}, journal = {Finance Research Letters}, pages = {105336}, year = {2024}, publisher = {Elsevier} }
[J-2024-01] James, Winston and Perez-Raya, Isaac. (2024). “3D Simulations of Nucleate Boiling with Sharp Interface Vof and Localized Adaptive Mesh Refinement in Ansys-Fluent.” Heat and Mass Transfer.
Abstract: The present work demonstrates the use of customized Ansys-Fluent in performing 3D numerical simulations of nucleate boiling with a sharp interface and adaptive mesh refinement. The developed simulation approach is a reliable and effective tool to investigate 3D boiling phenomena by accurately capturing the thermal and fluid dynamic interfacial vapor-liquid interaction and reducing computational time. These methods account for 3D sharp interface and thermal conditions of saturation temperature refining the mesh around the bubble edge. User-Defined-Functions (UDFs) were developed to customize the software Ansys-Fluent to preserve the interface sharpness, maintain saturation temperature conditions, and perform effective adaptive mesh refinement in a localized region around the interface. Adaptive mesh refinement is accomplished by a UDF that identifies the cells near the contact line and the liquid-vapor interface and applies the adaptive mesh refinement algorithms only at the identified cells. Validating the approach considered spherical bubble growth with an observed acceptable difference between theoretical and simulation bubble growth rates of 10%. Bubble growth simulations with water reveal an influence region of 2.7 times the departure bubble diameter, and average heat transfer coefficient of 15000 W/m2-K. In addition, the results indicate a reduced computational time of 75 hours using adaptive mesh compared to uniform mesh.
Publisher Site: ASME Digital Collection
PDF (Requires Sign-In): PDF
Click for Bibtex
@article{james20243d, title = {3D Simulations of Nucleate Boiling with Sharp Interface Vof and Localized Adaptive Mesh Refinement in Ansys-Fluent}, author = {James, Winston and Perez-Raya, Isaac}, journal = {ASME Journal of Heat and Mass Transfer}, pages = {1--47}, year = {2024} }
[J-2023-12] Grant, Michael J. and Fingler, Brennan J. and Buchanan, Natalie and Padmanabhan, Poornima. (2023). “Coil—Helix Block Copolymers Can Exhibit Divergent Thermodynamics in the Disordered Phase.” Chemical Theory and Computation.
Abstract: Chiral building blocks have the ability to self-assemble and transfer chirality to larger hierarchical length scales, which can be leveraged for the development of novel nanomaterials. Chiral block copolymers, where one block is made completely chiral, are prime candidates for studying this phenomenon, but fundamental questions regarding the self-assembly are still unanswered. For one, experimental studies using different chemistries have shown unexplained diverging shifts in the order—disorder transition temperature. In this study, particle-based molecular simulations of chiral block copolymers in the disordered melt were performed to uncover the thermodynamic behavior of these systems. A wide range of helical models were selected, and several free energy calculations were performed. Specifically, we aimed to understand (1) the thermodynamic impact of changing the conformation of one block in chemically identical block copolymers and (2) the effect of the conformation on the Flory—Huggins interaction parameter, χ, when chemical disparity was introduced. We found that the effective block repulsion exhibits diverging behavior, depending on the specific conformational details of the helical block. Commonly used conformational metrics for flexible or stiff block copolymers do not capture the effective block repulsion because helical blocks are semiflexible and aspherical. Instead, pitch can quantitatively capture the effective block repulsion. Quite remarkably, the shift in χ for chemically dissimilar block copolymers can switch sign with small changes in the pitch of the helix.
Publisher Site: ACS Publications
PDF (Requires Sign-In): PDF
Click for Bibtex
@article{grant2023coil, title = {Coil--Helix Block Copolymers Can Exhibit Divergent Thermodynamics in the Disordered Phase}, author = {Grant, Michael J and Fingler, Brennan J and Buchanan, Natalie and Padmanabhan, Poornima}, journal = {Journal of Chemical Theory and Computation}, year = {2023}, publisher = {ACS Publications} }
[J-2023-11] Ebmeyer, William and Dholabhai, Pratik P. (2023). “High-Throughput Prediction of Oxygen Vacancy Defect Migration Near Misfit Dislocations in SrTiO3/BaZrO3 Heterostructures.” Materials Advances.
Abstract: Among their numerous technological applications, semi-coherent oxide heterostructures have emerged as promising candidates for applications in intermediate temperature solid oxide fuel cell electrolytes, wherein interfaces influence ionic transport. Since misfit dislocations impact ionic transport in these materials, oxygen vacancy formation and migration at misfit dislocations in oxide heterostructures is central to its performance as an ionic conductor. Herein, we report High-throughput atomistic simulations to predict thousands of activation energy barriers for oxygen vacancy migration at misfit dislocations in SrTiO3/BaZrO3 heterostructures. Dopants display a noticeable effect as higher activation energies are uncovered in their vicinity. Interface layer chemistry has a fundamental influence on the magnitude of activation energy barriers since they are dissimilar at misfit dislocations as compared to coherent terraces. Lower activation energies are uncovered when oxygen vacancies migrate toward misfit dislocations, but higher energies when they hop away, revealing that oxygen vacancies would get trapped at misfit dislocations and impact ionic transport. Results herein offer atomic scale insights into ionic transport at misfit dislocations and fundamental factors governing ionic conductivity of thin film oxide electrolytes.
Publisher Site: RSC.org
PDF (Requires Sign-In): PDF
Click for Bibtex
@article{ebmeyer2023high, title = {High-throughput prediction of oxygen vacancy defect migration near misfit dislocations in SrTiO3/BaZrO3 heterostructures}, author = {Ebmeyer, William and Dholabhai, Pratik P}, journal = {Materials Advances}, year = {2023}, publisher = {Royal Society of Chemistry} }
[J-2023-10] Apergi, Lida A. and Bjarnadóttir, Margrét Vilborg and Baras, John S. and Golden, Bruce L. (2023). “Cost Patterns of Multiple Chronic Conditions: A Novel Modeling Approach Using a Condition Hierarchy.” INFORMS Journal on Data Science.
Abstract: Healthcare cost predictions are widely used throughout the healthcare system. However, predicting these costs is complex because of both uncertainty and the complex interactions of multiple chronic diseases: chronic disease treatment decisions related to one condition are impacted by the presence of the other conditions. We propose a novel modeling approach inspired by backward elimination, designed to minimize information loss. Our approach is based on a cost hierarchy: the cost of each condition is modeled as a function of the number of other, more expensive chronic conditions the individual member has. Using this approach, we estimate the additive cost of chronic diseases and study their cost patterns. Using large-scale claims data collected from 2007 to 2012, we identify members that suffer from one or more chronic conditions and estimate their total 2012 healthcare expenditures. We apply regression analysis and clustering to characterize the cost patterns of 69 chronic conditions. We observe that the estimated cost of some conditions (for example, organic brain problem) decreases as the member's number of more expensive chronic conditions increases. Other conditions, such as obesity and paralysis, demonstrate the opposite pattern; their contribution to the overall cost increases as the member's number of other more serious chronic conditions increases. The modeling framework allows us to account for the complex interactions of multimorbidity and healthcare costs and, therefore, offers a deeper and more nuanced understanding of the cost burden of chronic conditions, which can be utilized by practitioners and policy makers to plan, design better intervention, and identify subpopulations that require additional resources. More broadly, our hierarchical model approach captures complex interactions and can be applied to improve decision making when the enumeration of all possible factor combinations is not possible, for example, in financial risk scoring and pay structure design.
Publisher Site: INFORMS
PDF (Requires Sign-In): PDF
Click for Bibtex
@article{apergi2023cost, title = {Cost Patterns of Multiple Chronic Conditions: A Novel Modeling Approach Using a Condition Hierarchy}, author = {Apergi, Lida Anna and Bjarnad{\'o}ttir, Margr{\'e}t Vilborg and Baras, John S and Golden, Bruce L}, journal = {INFORMS Journal on Data Science}, year = {2023}, publisher = {INFORMS} }
[J-2023-09] Rajendran, Madhusudan and Ferran, Maureen C. and Mouli, Leora and Babbitt, Gregory A. and Lynch, Miranda L. (2023). “Evolution of Drug Resistance Drives Destabilization of Flap Region Dynamics in HIV-1 Protease.” Biophysical Reports.
Abstract: The HIV-1 protease is one of several common key targets of combination drug therapies for human immunodeficiency virus infection and acquired immunodeficiency syndrome (HIV/AIDS). During the progression of the disease, some individual patients acquire drug resistance due to mutational hotspots on the viral proteins targeted by combination drug therapies. It has recently been discovered that drug-resistant mutations accumulate on the ‘flap region' of the HIV-1 protease, which is a critical dynamic region involved in non-specific polypeptide binding during invasion and infection of the host cell. In this study, we utilize machine learning assisted comparative molecular dynamics, conducted at single amino acid site resolution, to investigate the dynamic changes that occur during functional dimerization, and drug-binding of wild-type and common drug-resistant versions of the main protease. We also use a multi-agent machine learning model to identify conserved dynamics of the HIV-1 main protease that are preserved across simian and feline protease orthologs (SIV and FIV). We find that a key conserved functional site in the flap region, a solvent-exposed isoleucine (Ile50) that controls flap dynamics is functionally targeted by drug-resistance mutations, leading to amplified molecular dynamics affecting the functional ability of the flap region to hold the drugs. We conclude that better long term patient outcomes may be achieved by designing drugs that target protease regions which are less dependent upon single sites with large functional binding effects.
Publisher Site: ScienceDirect
PDF (Requires Sign-In): PDF
Click for Bibtex
@article{rajendran2023evolution, title = {Evolution of drug resistance drives destabilization of flap region dynamics in HIV-1 protease}, author = {Rajendran, Madhusudan and Ferran, Maureen C and Mouli, Leora and Babbitt, Gregory A and Lynch, Miranda L}, journal = {Biophysical Reports}, year = {2023}, publisher = {Elsevier} }
[J-2023-08] Marzano, Chloe and Dholabhai, Pratik P. (2023). “High-Throughput Prediction of Thermodynamic Stabilities of Dopant-Defect Clusters at Misfit Dislocations in Perovskite Oxide Heterostructures.” Journal of Physical Chemistry C.
Abstract: Complex oxide heterostructures and thin films have emerged as promising candidates for diverse applications, wherein interfaces formed by joining two different oxides play a central role in novel properties that are not present in the individual components. Lattice mismatch between the two oxides leads to the formation of misfit dislocations, which often influence vital material properties. In oxides, doping is used as a strategy to improve properties, wherein inclusion of aliovalent dopants leads to formation of oxygen vacancy defects. At low temperatures, these dopants and defects often form stable clusters. In semicoherent perovskite oxide heterostructures, the stability of such clusters at misfit dislocations, while not well understood, is anticipated to impact interface-governed properties. Herein, we report atomistic simulations elucidating the influence of misfit dislocations on the stability of dopant-defect clusters in SrTiO3/BaZrO3 heterostructures. SrO–BaO, SrO-ZrO2, BaO-TiO2, and ZrO2-TiO2 interfaces having dissimilar misfit dislocation structures were considered. High-throughput computing was implemented to predict the thermodynamic stabilities of 275,610 dopant-defect clusters in the vicinity of misfit dislocations. The misfit dislocation structure of the given interface and corresponding atomic layer chemistry play a fundamental role in influencing the thermodynamic stability of geometrically diverse clusters. A stark difference in cluster stability is observed at misfit dislocation lines and intersections as compared to the coherent terraces. These results offer an atomic scale perspective of the complex interplay between dopants, point defects, and extended defects, which is necessary to comprehend the functionalities of the perovskite oxide heterostructures.
Publisher Site: ACS Publications
PDF (Requires Sign-In): PDF
Click for Bibtex
@article{marzano2023high, title = {High-Throughput Prediction of Thermodynamic Stabilities of Dopant-Defect Clusters at Misfit Dislocations in Perovskite Oxide Heterostructures}, author = {Marzano, Chloe and Dholabhai, Pratik P}, journal = {The Journal of Physical Chemistry C}, year = {2023}, publisher = {ACS Publications} }
[J-2023-07] Lyu, Zimeng and Ororbia, Alexander and Desell, Travis. (2023). “Online Evolutionary Neural Architecture Search for Multivariate Non-Stationary Time Series Forecasting.” Applied Soft Computing.
Abstract: Time series forecasting (TSF) is one of the most important tasks in data science. TSF models are usually pre-trained with historical data and then applied on future unseen datapoints. However, real-world time series data is usually non-stationary and models trained offline usually face problems from data drift. Models trained and designed in an offline fashion can not quickly adapt to changes quickly or be deployed in real-time. To address these issues, this work presents the Online NeuroEvolution-based Neural Architecture Search (ONE-NAS) algorithm, which is a novel neural architecture search method capable of automatically designing and dynamically training recurrent neural networks (RNNs) for online forecasting tasks. Without any pre-training, ONE-NAS utilizes populations of RNNs that are continuously updated with new network structures and weights in response to new multivariate input data. ONE-NAS is tested on real-world, large-scale multivariate wind turbine data as well as the univariate Dow Jones Industrial Average (DJIA) dataset. Results demonstrate that ONE-NAS outperforms traditional statistical time series forecasting methods, including online linear regression, fixed long short-term memory (LSTM) and gated recurrent unit (GRU) models trained online, as well as state-of-the-art, online ARIMA strategies. Additionally, results show that utilizing multiple populations of RNNs which are periodically repopulated provide significant performance improvements, allowing this online neural network architecture design and training to be successful.
Publisher Site: ScienceDirect
PDF (Requires Sign-In): PDF
Click for Bibtex
@article{lyu2023online, title = {Online evolutionary neural architecture search for multivariate non-stationary time series forecasting}, author = {Lyu, Zimeng and Ororbia, Alexander and Desell, Travis}, journal = {Applied Soft Computing}, year = {2023}, publisher = {Elsevier} }
[J-2023-06] Keithley, Kimberlee S.M. and Palmerio, Jacob and Escobedo IV, Hector A. and Bartlett, Jordyn and Huang, Henry and Villasmil, Larry A. and Cromer, Michael. (2023). “Role of Shear Thinning in the Flow of Polymer Solutions Around a Sharp Bend.” Rheologica Acta.
Abstract: In flows with re-entrant corners, polymeric fluids can exhibit a recirculation region along the wall upstream from the corner. In general, the formation of these vortices is controlled by both the extensional and shear rheology of the material. More importantly, these regions can only form for sufficiently elastic fluids and are often called "lip vortices". These elastic lip vortices have been observed in the flows of complex fluids in geometries with sharp bends. In this work, we characterize the roles played by elasticity and shear thinning in the formation of the lip vortices. Simulations of the Newtonian, Bird-Carreau, and Oldroyd-B models reveal that elasticity is a necessary element. A systematic study of the White-Metzner, finitely extensible non-linear elastic (FENE-P), Giesekus and Rolie-Poly models shows that the onset and size of the elastic lip vortex is governed by a combination of both the degree of shear thinning and the critical shear rate at which the thinning begins.
Publisher Site: SpringerLink
PDF (Requires Sign-In): PDF
Click for Bibtex
@article{keithley2023role, title = {Role of shear thinning in the flow of polymer solutions around a sharp bend}, author = {Keithley, Kimberlee SM and Palmerio, Jacob and Escobedo IV, Hector A and Bartlett, Jordyn and Huang, Henry and Villasmil, Larry A and Cromer, Michael}, journal = {Rheologica Acta}, pages = {1--15}, year = {2023}, publisher = {Springer} }
[J-2023-05] Nur, Nayma Binte and Bachmann, Charles M. (2023). “Comparison of Soil Moisture Content Retrieval Models Utilizing Hyperspectral Goniometer Data and Hyperspectral Imagery from an Unmanned Aerial System.” Journal of Geophysical Research.
Abstract: To understand surface biogeophysical processes, accurately evaluating the geographical and temporal fluctuations of soil moisture is crucial. It is well known that the surface soil moisture content (SMC) affects soil reflectance at all solar spectrum wavelengths. Therefore, future satellite missions, such as the NASA Surface Biology and Geology (SBG) mission, will be essential for mapping and monitoring global soil moisture changes. Our study compares two widely used moisture retrieval models: the multilayer radiative transfer model of soil reflectance (MARMIT) and the soil water parametric (SWAP)-Hapke model. We evaluated the SMC retrieval accuracy of these models using unmanned aerial systems (UAS) hyperspectral imagery and goniometer hyperspectral data. Laboratory analysis employed hyperspectral goniometer data of sediment samples from four locations reflecting diverse environments, while field validation used hyperspectral UAS imaging and coordinated ground truth collected in 2018 and 2019 from a barrier island beach at the Virginia Coast Reserve Long-Term Ecological Research site. The (SWAP)-Hapke model achieves comparable accuracy to MARMIT using laboratory hyperspectral data but is less accurate when applied to UAS hyperspectral imagery than the MARMIT model. We proposed a modified version of the (SWAP)-Hapke model, which achieves better results than MARMIT when applied to laboratory spectral measurements; however, MARMIT performance is still more accurate when applied to UAS imagery. These results are likely due to differences in the models' descriptions of multiply-scattered light and MARMIT's more detailed description of air-water interactions.
Publisher Site: Wiley
PDF (Requires Sign-In): PDF
Click for Bibtex
@article{nur2023comparison, title = {Comparison of Soil Moisture Content Retrieval Models Utilizing Hyperspectral Goniometer Data and Hyperspectral Imagery from an Unmanned Aerial System}, author = {Nur, Nayma Binte and Bachmann, Charles M}, journal = {Journal of Geophysical Research: Biogeosciences}, year = {2023}, publisher = {Wiley Online Library} }
[J-2023-04] Olatunji, Isaac and Cui, Feng. (2023). “Multimodal AI for Prediction of Distant Metastasis in Carcinoma Patients.” Frontiers in Bioinformatics.
Abstract: Metastasis of cancer is directly related to death in almost all cases, however a lot is yet to be understood about this process. Despite advancements in the available radiological investigation techniques, not all cases of Distant Metastasis (DM) are diagnosed at initial clinical presentation. Also, there are currently no standard biomarkers of metastasis. Early, accurate diagnosis of DM is however crucial for clinical decision making, and planning of appropriate management strategies. Previous works have achieved little success in attempts to predict DM from either clinical, genomic, radiology, or histopathology data. In this work we attempt a multimodal approach to predict the presence of DM in cancer patients by combining gene expression data, clinical data and histopathology images. We tested a novel combination of Random Forest (RF) algorithm with an optimization technique for gene selection, and investigated if gene expression pattern in the primary tissues of three cancer types (Bladder Carcinoma, Pancreatic Adenocarcinoma, and Head and Neck Squamous Carcinoma) with DM are similar or different. Gene expression biomarkers of DM identified by our proposed method outperformed Differentially Expressed Genes (DEGs) identified by the DESeq2 software package in the task of predicting presence or absence of DM. Genes involved in DM tend to be more cancer type specific rather than general across all cancers. Our results also indicate that multimodal data is more predictive of metastasis than either of the three unimodal data tested, and genomic data provides the highest contribution by a wide margin. The results re-emphasize the importance for availability of sufficient image data when a weakly supervised training technique is used. Code is made available at: https://github.com/rit-cuilab/Multimodal-AI-for-Prediction-of-Distant-Metastasis-in-Carcinoma-Patients.
Publisher Site: PubMed Central
PDF (Requires Sign-In): PDF
Click for Bibtex
@article{olatunji2023multimodal, title = {Multimodal AI for prediction of distant metastasis in carcinoma patients}, author = {Olatunji, Isaac and Cui, Feng}, journal = {Frontiers in Bioinformatics}, volume = {3}, year = {2023}, publisher = {Frontiers Media SA} }
[J-2023-03] Childs, Megan Rowan and Wong, Tony E. (2023). “Assessing Parameter Sensitivity in a University Campus COVID-19 Model with Vaccination.” Journal of Infectious Disease Modelling.
Abstract: From the beginning of the COVID-19 pandemic, universities have experienced unique challenges due to their dual nature as a place of education and residence. Current research has explored non-pharmaceutical approaches to combating COVID-19, including representing in models different categories such as age groups. One key area not currently well represented in models is the effect of pharmaceutical preventative measures, specifically vaccinations, on COVID-19 spread on college campuses. There remain key questions on the sensitivity of COVID-19 infection rates on college campuses to potentially time-varying vaccine immunity. Here we introduce a compartment model that decomposes a campus population into constituent subpopulations and implements vaccinations with time-varying efficacy. We use this model to represent a campus population with both vaccinated and unvaccinated individuals, and we analyze this model using two metrics of interest: maximum isolation population and symptomatic infection. We demonstrate a decrease in symptomatic infections occurs for vaccinated individuals when the frequency of testing for unvaccinated individuals is increased. We find that the number of symptomatic infections is insensitive to the frequency of testing of the unvaccinated subpopulation once about 80% or more of the population is vaccinated. Through a Sobol' global sensitivity analysis, we characterize the sensitivity of modeled infection rates to these uncertain parameters. We find that in order to manage symptomatic infections and the maximum isolation population campuses must minimize contact between infected and uninfected individuals, promote high vaccine protection at the beginning of the semester, and minimize the number of individuals developing symptoms.
Publisher Site: ScienceDirect
PDF (Requires Sign-In): PDF
Click for Bibtex
@article{childs2023assessing, title = {Assessing parameter sensitivity in a university campus COVID-19 model with vaccinations}, author = {Childs, Meghan Rowan and Wong, Tony E}, journal = {Infectious Disease Modelling}, year = {2023}, publisher = {Elsevier} }
[J-2023-02] Bergstrom, Austin C. and Messinger, David W. (2023). “Image Quality and Computer Vision Performance: Assessing the Effects of Image Distortions and Modeling Performance Relationships Using the General Image Quality Equation.” Journal of Electronic Imaging.
Abstract: Substantial research has explored methods to optimize convolutional neural networks (CNNs) for tasks such as image classification and object detection, but research into the image quality drivers of computer vision performance has been limited. Additionally, there are indications that image degradations such as blur and noise affect human visual interpretation and machine interpretation differently. The general image quality equation (GIQE) predicts overhead image quality for human analysis using the National Image Interpretability Rating Scale, but no such model exists to predict image quality for interpretation by CNNs. Here, we assess the relationship between image quality variables and CNN performance. Specifically, we examine the impacts of resolution, blur, and noise on CNN performance for models trained with in-distribution and out-of-distribution distortions. Using two datasets, we observe that while generalization remains a significant challenge for CNNs faced with out-of-distribution image distortions, CNN performance against low visual quality images remains strong with appropriate training, indicating the potential to expand the design trade space for sensors providing data to computer vision systems. Additionally, we find that CNN performance predictions using the functional form of the GIQE can predict CNN performance as a function of image degradation, but we observe that the legacy form of the GIQE (from GIQE versions 3 and 4) does a better job of modeling the impact of blur/relative edge response in our experiments.
Publishier Site: SPIE
PDF (Requires Sign-In): PDF
Click for Bibtex
@article{bergstrom2023image, title = {Image quality and computer vision performance: assessing the effects of image distortions and modeling performance relationships using the general image quality equation}, author = {Bergstrom, Austin C and Messinger, David W}, journal = {Journal of Electronic Imaging}, volume = {32}, number = {2}, pages = {023018}, year = {2023}, publisher = {SPIE} }
[J-2023-01] Shipkowski, S.P. and Perez-Reya, I. (2023). “Precise and Analytical Calculation of Interface Surface Area in Sharp Interfaces and Multiphase Modeling.” International Journal of Heat and Mass Transfer.
Abstract: Various kinds of Piecewise Linear Interface Calculations (PLIC) are commonly used in Computational Fluid Dynamics (CFD) for multiphase flows. Here, a new method is proposed to calculate the interface size for CFD simulations. This PLIC method with the modifier, Analytic Size Based [method], (PLIC-ASB), being that the calculation requires a symbolic equation and no approximation or numerical methods. The primary advantage of the PLIC-ASB is maintaining a sharp interface and providing for physics-based (not iterative or empirically derived factors) mass transfer due to the cell-by-cell accuracy. Along with the sharp interface, this method leads to precise interface displacements and suppresses parasitic velocities; altogether, this means an accurate model of the temperature distribution near the interface can be achieved. The PLIC-ASB method was compared directly against the VOF gradient method (most prevalent PLIC in multiphase simulations). Static testing for accuracy on various geometric shapes expressed as the color function on representative grids of cells. The PLIC-ASB had a better result than the VOF gradient method, with an average relative error of 3% and 13%, respectively. Spherical bubble growth simulations were performed with customized ANSYS-Fluent. Both interface calculation methods were tested. Foremost, the PLIC-ASB method produced experimentally and theoretically anticipated results. Parasitic velocities were problematic in the VOF gradient method simulations but only minor with the use of PLIC-ASB. Further, a trend of accuracy improving with reduced cell size was found with the PLIC-ASB, whereas no trend was present with the VOF-gradient method. The PLIC-ASB method has the characteristic limitations of any PLIC method, most notably requiring a straight-line approximation to suitably represent the multiphase interface; however, the straightforward implementation and accuracy make broad adoption reasonable.
Publisher Site: ScienceDirect
PDF (Requires Sign-In): PDF
Click for Bibtex
@article{shipkowski2023precise, title = {Precise and analytical calculation of interface surface area in sharp interfaces and multiphase modeling}, author = {Shipkowski, SP and Perez-Raya, I}, journal = {International Journal of Heat and Mass Transfer}, volume = {202}, pages = {123683}, year = {2023}, publisher = {Elsevier} }
[J-2022-14] Rajendran, Madhusudan and Babbitt, Gregory A. (2022). “Persistent Cross-Species SARS-CoV-2 Variant Infectivity Predicted via Comparative Molecular Dynamics Simulation.” Royal Society of Open Science.
Abstract: Widespread human transmission of SARS-CoV-2 highlights the substantial public health, economic and societal consequences of virus spillover from wildlife and also presents a repeated risk of reverse spillovers back to naive wildlife populations. We employ comparative statistical analyses of a large set of short-term molecular dynamic (MD) simulations to investigate the potential human-to-bat (genus Rhinolophus) cross-species infectivity allowed by the binding of SARS-CoV-2 receptor-binding domain (RBD) to angiotensin-converting enzyme 2 (ACE2) across the bat progenitor strain and emerging human strain variants of concern (VOC). We statistically compare the dampening of atom motion across protein sites upon the formation of the RBD/ACE2 binding interface using various bat versus human target receptors (i.e. bACE2 and hACE2). We report that while the bat progenitor viral strain RaTG13 shows some pre-adaption binding to hACE2, it also exhibits stronger affinity to bACE2. While early emergent human strains and later VOCs exhibit robust binding to both hACE2 and bACE2, the delta and omicron variants exhibit evolutionary adaption of binding to hACE2. However, we conclude there is a still significant risk of mammalian cross-species infectivity of human VOCs during upcoming waves of infection as COVID-19 transitions from a pandemic to endemic status.
Publisher Site: The Royal Society
PDF (Requires Sign-In): PDF
Click for Bibtex
@article{rajendran2022persistent, title = {Persistent cross-species SARS-CoV-2 variant infectivity predicted via comparative molecular dynamics simulation}, author = {Rajendran, Madhusudan and Babbitt, Gregory A}, journal = {Royal Society Open Science}, volume = {9}, number = {11}, pages = {220600}, year = {2022}, publisher = {The Royal Society} }
[J-2022-13] Medlar, Michael and Hensel, Edward. (2022). “Transient 3-D Thermal Simulation of a Fin-FET Transistor with Electron-Phonon Heat Generation, Three Phonon Scattering, and Drift with Periodic Switching.” Journal of Heat Transfer.
Abstract: The International Roadmap for Devices and Systems shows FinFET array transistors as the mainstream structure for logic devices. Traditional methods of Fourier heat transfer analysis that are typical of Technology Computer Aided Design (TCAD) software are invalid at the length and time scales associated with these devices. Traditional models for phonon transport modeling have not demonstrated the ability to accurately model 3-d, transient transistor thermal responses. An engineering design tool is needed to accurately predict the thermal response of FinFET transistor arrays. The Statistical Phonon Transport Model (SPTM) was applied in a 3-d, transient manner to predict non-equilibrium phonon transport in an SOI-FinFET array transistor with a 60 nm long fin and a 20 nm channel length. A heat generation profile from electron-phonon scattering was applied in a transient manner to model switching. Simulation results indicated an excess build-up of up to 17% optical phonons giving rise to transient local temperature hot spots of 37 Kelvin in the drain region. The local build-up of excess optical phonons in the drain region has implications on performance and reliability. The SPTM is a valid engineering design tool for evaluating the thermal performance of emergent proposed FinFET transistor designs. The phonon fidelity of the SPTM is greater than Monte Carlo and the Boltzmann Transport Equation and the length scale and time scale fidelity of the SPTM is better than Direct Atomic Simulation.
Publisher Site: ASME Digital Collection
PDF (Requires Sign-In): PDF
Click for Bibtex
@article{medlar2022transient, title = {Transient 3-D Thermal Simulation of a Fin-FET Transistor with Electron-Phonon Heat Generation, Three Phonon Scattering, and Drift with Periodic Switching}, ISSN = {1528-8943}, url = {http://dx.doi.org/10.1115/1.4056002}, DOI = {10.1115/1.4056002}, journal = {Journal of Heat Transfer}, publisher = {ASME International}, author = {Medlar, Michael and Hensel, Edward}, year = {2022}, month = {Oct} }
[J-2022-12] Uche, Obioma U. and Sidorick, Micah. (2022). “A Comparative Analysis of the Vibrational and Structural Properties of Nearly Incommensurate Overlayer Systems.” Surfance Science.
Abstract: Using molecular dynamics simulations based on embedded atom method potentials, we have investigated the vibrational properties of the following three systems of nearly hexagonal noble metal monolayers adsorbed on substrates of square symmetry: Ag on Cu(001), Ag on Ni(001) and Au on Ni(001). The corresponding local phonon density of states, mean square amplitudes, and substrate interlayer relaxation of the above systems are compared to identify similarities and differences. A key finding is that the Ag monolayers have a stronger influence on both phonon spectra and vibrational amplitudes of the topmost layer of the Cu(001) surface than that of the nickel substrates. Furthermore, the vibrational amplitudes of the overlayer atoms are shown to be anisotropic and dominant along the incommensurate direction for the three systems. Temperature effects on the vibrational and structural properties are also discussed.
Publisher Site: ScienceDirect
PDF (Requires Sign-In): PDF
Click for Bibtex
@article{uche2022comparative, title = {A comparative analysis of the vibrational and structural properties of nearly incommensurate overlayer systems}, author = {Uche, Obioma U and Sidorick, Micah}, journal = {Surface Science}, volume = {717}, pages = {121989}, year = {2022}, publisher = {Elsevier} }
[J-2022-11] Sankaran, Prashant and McConky, Katie and Sudit, Moises and Ortiz-Peña, Héctor. (2022). “GAMMA: Graph Attention Model for Multiple Agents to Solve Team Orienteering Problem With Multiple Depots.” IEEE Transactions on Neural Networks and Learning Systems.
Abstract: In this work, we present an attention-based encoder-decoder model to approximately solve the team orienteering problem with multiple depots (TOPMD). The TOPMD instance is an NP-hard combinatorial optimization problem that involves multiple agents (or autonomous vehicles) and not purely Euclidean (straight line distance) graph edge weights. In addition, to avoid tedious computations on dataset creation, we provide an approach to generate synthetic data on the fly for effectively training the model. Furthermore, to evaluate our proposed model, we conduct two experimental studies on the multi-agent reconnaissance mission planning problem formulated as TOPMD. First, we characterize the model based on the training configurations to understand the scalability of the proposed approach to unseen configurations. Second, we evaluate the solution quality of the model against several baselines-heuristics, competing machine learning (ML), and exact approaches, on several reconnaissance scenarios. The experimental results indicate that training the model with a maximum number of agents, a moderate number of targets (or nodes to visit), and moderate travel length, performs well across a variety of conditions. Furthermore, the results also reveal that the proposed approach offers a more tractable and higher quality (or competitive) solution in comparison with existing attention-based models, stochastic heuristic approach, and standard mixed-integer programming solver under the given experimental conditions. Finally, the different experimental evaluations reveal that the proposed data generation approach for training the model is highly effective.
Publisher Site: IEEE Xplore
PDF (Requires Sign-In): PDF
Click for Bibtex
@article{sankaran2022gamma, title = {GAMMA: Graph Attention Model for Multiple Agents to Solve Team Orienteering Problem With Multiple Depots}, author = {Sankaran, Prashant and McConky, Katie and Sudit, Moises and Ortiz-Pe{\~n}a, H{\'e}ctor}, journal = {IEEE Transactions on Neural Networks and Learning Systems}, year = {2022}, publisher = {IEEE} }
[J-2022-10] Rajendran, Madhusudan and Ferran, Maureen C. and Babbitt, Gregory A. (2022). “Identifying Vaccine Escape Sites via Statistical Comparisons of Short-term Molecular Dynamics.” Biophysical Reports.
Abstract: The identification of viral mutations that confer escape from antibodies is crucial for understanding the interplay between immunity and viral evolution. We describe a molecular dynamics (MD) based approach that goes beyond contact mapping, scales well to a desktop computer with a modern graphics processor, and enables the user to identify functional protein sites that are prone to vaccine escape in a viral antigen. We first implement our MD pipeline to employ site-wise calculation of Kullback-Leibler divergence in atom fluctuation over replicate sets of short-term MD production runs thus enabling a statistical comparison of the rapid motion of influenza hemagglutinin (HA) in both the presence and absence of three well-known neutralizing antibodies. Using this simple comparative method applied to motions of viral proteins, we successfully identified in silico all previously empirically confirmed sites of escape in influenza HA, predetermined via selection experiments and neutralization assays. Upon the validation of our computational approach, we then surveyed potential hot spot residues in the receptor binding domain of the SARS-CoV-2 virus in the presence of COVOX-222 and S2H97 antibodies. We identified many single sites in the antigen-antibody interface that are similarly prone to potential antibody escape and that match many of the known sites of mutations arising in the SARS-CoV-2 variants of concern. In the omicron variant, we find only minimal adaptive evolutionary shifts in the functional binding profiles of both antibodies. In summary, we provide an inexpensive and accurate computational method to monitor hot spots of functional evolution in antibody binding footprints.
Publisher Site: ScienceDirect
PDF (Requires Sign-In): PDF
Click for Bibtex
@article{rajendran2022identifying, title = {Identifying vaccine escape sites via statistical comparisons of short-term molecular dynamics}, author = {Rajendran, Madhusudan and Ferran, Maureen C and Babbitt, Gregory A}, journal = {Biophysical reports}, volume = {2}, number = {2}, pages = {100056}, year = {2022}, publisher = {Elsevier} }
[J-2022-09] Peters, Jared I. and Tang, Lisa and Uche, Obioma U. (2022). “Influence of Size and Composition on the Transformation Mechanics of Gold-Silver Core-Shell Nanoparticles.” The Journal of Physical Chemistry C.
Abstract: Bimetallic nanoparticles occupy a unique space in the field of materials science as they display physical and optoelectronic properties that are absent in their monometallic counterparts. These attributes increase their applicability in niche processes where they can perform distinct functions from those achievable with the corresponding pure-element particles. In the current work, we explore the feasibility of performing structural characterization of gold-silver core-shell nanoclusters as well as analyze the transformation between cuboctahedral and icosahedral geometries using a combination of atomistic simulations and electronic structure calculations. We find that size may be a limiting factor in distinguishing between the above two geometries when theoretical vibrational densities of states are employed in characterizing the nanoparticles under consideration. The results from our density functional theory calculations also reveal that the transformation between cuboctahedral and icosahedral geometries occurs via a martensitic, symmetric mechanism for the 147-atom and 309-atom nanoclusters. In addition, the associated transformation barriers for the bimetallic core-shell particles are strongly size-dependent and typically increase with the composition of silver in the nanocluster.
Publisher Site: ACS Publications
PDF (Requires Sign-In): PDF
Click for Bibtex
@article{peters2022influence, title = {Influence of Size and Composition on the Transformation Mechanics of Gold--Silver Core--Shell Nanoparticles}, author = {Peters, Jared I and Tang, Lisa and Uche, Obioma U}, journal = {The Journal of Physical Chemistry C}, volume = {126}, number = {15}, pages = {6612--6618}, year = {2022}, publisher = {ACS Publications} }
[J-2022-08] Meyers, Benjamin S. and Almassari, Sultan Fahad and Keller, Brandon N. and Meneely, Andrew. (2022). “Examining Penetration Tester Behavior in the Collegiate Penetration Testing Competition.” ACM Transactions on Software Engineering and Methodology (TOSEM).
Abstract: Penetration testing is a key practice toward engineering secure software. Malicious actors have many tactics at their disposal, and software engineers need to know what tactics attackers will prioritize in the first few hours of an attack. Projects like MITRE ATT&CK(TM) provide knowledge, but how do people actually deploy this knowledge in real situations? A penetration testing competition provides a realistic, controlled environment with which to measure and compare the efficacy of attackers. In this work, we examine the details of vulnerability discovery and attacker behavior with the goal of improving existing vulnerability assessment processes using data from the 2019 Collegiate Penetration Testing Competition (CPTC). We constructed 98 timelines of vulnerability discovery and exploits for 37 unique vulnerabilities discovered by 10 teams of penetration testers. We grouped related vulnerabilities together by mapping to Common Weakness Enumerations and MITRE ATT&CK(TM). We found that (1) vulnerabilities related to improper resource control (e.g., session fixation) are discovered faster and more often, as well as exploited faster, than vulnerabilities related to improper access control (e.g., weak password requirements), (2) there is a clear process followed by penetration testers of discovery/collection to lateral movement/pre-attack. Our methodology facilitates quicker analysis of vulnerabilities in future CPTC events.
Publisher Site: ACM Digital Library
PDF (Requires Sign-In): PDF
Click for Bibtex
@article{meyers2022examining, title = {Examining penetration tester behavior in the collegiate penetration testing competition}, author = {Meyers, Benjamin S and Almassari, Sultan Fahad and Keller, Brandon N and Meneely, Andrew}, journal = {ACM Transactions on Software Engineering and Methodology (TOSEM)}, volume = {31}, number = {3}, pages = {1--25}, year = {2022}, publisher = {ACM New York, NY} }
[J-2022-07] Kothari, Rakshit S. and Bailey, Reynold J. and Kanan, Christopher and Pelz, Jeff B. and Diaz, Gabriel J. (2022). “EllSeg-Gen, Towards Domain Generalization for Head-mounted Eyetracking.” Proceedings of the ACM on Human-Computer Interaction.
Abstract: The study of human gaze behavior in natural contexts requires algorithms for gaze estimation that are robust to a wide range of imaging conditions. However, algorithms often fail to identify features such as the iris and pupil centroid in the presence of reflective artifacts and occlusions. Previous work has shown that convolutional networks excel at extracting gaze features despite the presence of such artifacts. However, these networks often perform poorly on data unseen during training. This work follows the intuition that jointly training a convolutional network with multiple datasets learns a generalized representation of eye parts. We compare the performance of a single model trained with multiple datasets against a pool of models trained on individual datasets. Results indicate that models tested on datasets in which eye images exhibit higher appearance variability benefit from multiset training. In contrast, dataset-specific models generalize better onto eye images with lower appearance variability.
Publisher Site: ACM Digital Library
PDF (Requires Sign-In): PDF
Click for Bibtex
@article{kothari2022ellseg, title = {EllSeg-Gen, towards Domain Generalization for head-mounted eyetracking}, author = {Kothari, Rakshit S and Bailey, Reynold J and Kanan, Christopher and Pelz, Jeff B and Diaz, Gabriel J}, journal = {Proceedings of the ACM on Human-Computer Interaction}, volume = {6}, number = {ETRA}, pages = {1--17}, year = {2022}, publisher = {ACM New York, NY, USA} }
[J-2022-06] Grant, Michael and Kunz, M. Ross and Iyer, Krithika and Held, Leander I. and Tasdizen, Tolga and Aguiar, Jeffery A. and Dholabhai, Pratik P. (2022). “Integrating Atomistic Simulations and Machine Learning to Design Multi-principal Element Alloys with Superior Elastic Modulus.” Journal of Materials Research.
Abstract: Multi-principal element, high entropy alloys (HEAs) are an emerging class of materials that have found applications across the board. Owing to the multitude of possible candidate alloys, exploration and compositional design of HEAs for targeted applications is challenging since it necessitates a rational approach to identify compositions exhibiting enriched performance. Here, we report an innovative framework that integrates molecular dynamics and machine learning to explore a large chemical-configurational space for evaluating elastic modulus of equiatomic and non-equiatomic HEAs along primary crystallographic directions. Vital thermodynamic properties and machine learning features have been incorporated to establish fundamental relationships correlating Young's modulus with Gibbs free energy, valence electron concentration, and atomic size difference. In HEAs, as the number of elements increases, interactions between the elastic modulus values and features become increasingly nested, but tractable as long as non-linearity is accounted. Basic design principles are offered to predict HEAs with enhanced mechanical attributes.
Publisher Site: SpringerLink
PDF (Requires Sign-In): PDF
Click for Bibtex
@article{grant2022integrating, title = {Integrating atomistic simulations and machine learning to design multi-principal element alloys with superior elastic modulus}, author = {Grant, Michael and Kunz, M Ross and Iyer, Krithika and Held, Leander I and Tasdizen, Tolga and Aguiar, Jeffery A and Dholabhai, Pratik P}, journal = {Journal of Materials Research}, volume = {37}, number = {8}, pages = {1497--1512}, year = {2022}, publisher = {Springer} }
[J-2022-05] Dholabhai, Pratik P. (2022). “Oxygen Vacancy Formation and Interface Charge Transfer at Misfit Dislocations in Gd-Doped CeO2/MgO Heterostructures.” The Journal of Physical Chemistry C.
Abstract: Among numerous functionalities of mismatched complex oxide thin films and heterostructures, their application as next-generation electrolytes in solid oxide fuel cells has shown remarkable promise. In thin-film oxide electrolytes, although misfit dislocations ubiquitous at interfaces play a critical role in ionic transport, fundamental understanding of their influence on oxygen vacancy formation and passage is nevertheless lacking. Herein, we report first-principles density functional theory calculations to elucidate the atomic and electronic structures of misfit dislocations in the CeO 2 /MgO heterostructure for the experimentally observed epitaxial relationship. Thermodynamic stability of the structure corroborates recent results demonstrating that the 45 degree rotation of the CeO 2 thin film eliminates the surface dipole, resulting in experimentally observed epitaxy. The energetics and electronic structures of oxygen vacancy formation near gadolinium dopants at misfit dislocations are evaluated, which demonstrate complex tendencies as compared to the grain interior and surfaces of ceria. The interface charge transfer mechanism is studied for defect-free and defective interfaces. Since the atomic and electronic structures of misfit dislocations at complex oxide interfaces and their influence on interface charge transfer and oxygen vacancy defect formation have not been studied in the past, this work offers new opportunities to unravel the untapped potential of oxide heterostructures.
Publisher Site: ACS Publications
PDF (Requires Sign-In): PDF
Click for Bibtex
@article{dholabhai2022oxygen, title = {Oxygen Vacancy Formation and Interface Charge Transfer at Misfit Dislocations in Gd-Doped CeO2/MgO Heterostructures}, author = {Dholabhai, Pratik P}, journal = {The Journal of Physical Chemistry C}, volume = {126}, number = {28}, pages = {11735--11750}, year = {2022}, publisher = {ACS Publications} }
[J-2022-04] Chaudhary, Aayush K. and Nair, Nitinraj and Bailey, Reynold J. and Pelz, Jeff B. and Talathi, Sachin S. and Diaz, Gabriel J. (2022). “Temporal RIT-Eyes: From Real Infrared Eye-Images to Synthetic Sequences of Gaze Behavior.” IEEE Transactions on Visualization and Computer Graphics.
Abstract: Current methods for segmenting eye imagery into skin, sclera, pupil, and iris cannot leverage information about eye motion. This is because the datasets on which models are trained are limited to temporally non-contiguous frames. We present Temporal RIT-Eyes , a Blender pipeline that draws data from real eye videos for the rendering of synthetic imagery depicting natural gaze dynamics. These sequences are accompanied by ground-truth segmentation maps that may be used for training image-segmentation networks. Temporal RIT-Eyes relies on a novel method for the extraction of 3D eyelid pose (top and bottom apex of eyelids/eyeball boundary) from raw eye images for the rendering of gaze-dependent eyelid pose and blink behavior. The pipeline is parameterized to vary in appearance, eye/head/camera/illuminant geometry, and environment settings (indoor/outdoor). We present two open-source datasets of synthetic eye imagery: sGiW is a set of synthetic-image sequences whose dynamics are modeled on those of the Gaze in Wild dataset, and sOpenEDS2 is a series of temporally non-contiguous eye images that approximate the OpenEDS-2019 dataset. We also analyze and demonstrate the quality of the rendered dataset qualitatively and show significant overlap between latent-space representations of the source and the rendered datasets.
Publisher Site: IEEE Xplore
PDF (Requires Sign-In): PDF
Click for Bibtex
@article{chaudhary2022temporal, title = {Temporal RIT-Eyes: From Real Infrared Eye-Images to Synthetic Sequences of Gaze Behavior}, author = {Chaudhary, Aayush K. and Nair, Nitinraj and Bailey, Reynold J. and Pelz, Jeff B. and Talathi, Sachin S. and Diaz, Gabriel J.}, journal = {IEEE Transactions on Visualization and Computer Graphics}, volume = {}, number = {}, pages = {1-11}, year = {2022}, publisher = {IEEE} doi = {10.1109/TVCG.2022.3203100} }
[J-2022-03] Butler, Victoria L. and Feder, Richard M. and Daylan, Tansu and Mantz, Adam B. and Mercado, Dale and Montaña, Alfredo and Portillo, Stephen K.N. and Sayers, Jack and Vaughan, Benjamin J. and Zemcov, Michael and Others. (2022). “Measurement of the Relativistic Sunyaev-Zeldovich Correction in RX J1347.5-1145.” The Astrophysical Journal.
Abstract: We present a measurement of the relativistic corrections to the thermal Sunyaev-Zel'dovich (SZ) effect spectrum, the rSZ effect, toward the massive galaxy cluster RX J1347.5-1145 by combining submillimeter images from Herschel-SPIRE with millimeter wavelength Bolocam maps. Our analysis simultaneously models the SZ effect signal, the population of cosmic infrared background galaxies, and the galactic cirrus dust emission in a manner that fully accounts for their spatial and frequency-dependent correlations. Gravitational lensing of background galaxies by RX J1347.5-1145 is included in our methodology based on a mass model derived from the Hubble Space Telescope observations. Utilizing a set of realistic mock observations, we employ a forward modeling approach that accounts for the non-Gaussian covariances between the observed astrophysical components to determine the posterior distribution of SZ effect brightness values consistent with the observed data. We determine a maximum a posteriori (MAP) value of the average Comptonization parameter of the intracluster medium (ICM) within R_2500 to be <y>_2500 = 1.56 x 10-4, with corresponding 68% credible interval [1.42, 1.63] x 10-4, and a MAP ICM electron temperature of <T_sz>_2500 = 22.4 keV with 68% credible interval spanning [10.4, 33.0] keV. This is in good agreement with the pressure-weighted temperature obtained from Chandra X-ray observations, <T_x,pw>_2500 = 17.4 +/- 2.3 keV. We aim to apply this methodology to comparable existing data for a sample of 39 galaxy clusters, with an estimated uncertainty on the ensemble mean <T_sz>_2500 at the ~= 1 keV level, sufficiently precise to probe ICM physics and to inform X-ray temperature calibration.
Publisher Site: IOP Science
PDF (Requires Sign-In): PDF
Click for Bibtex
@article{butler2022measurement, title = {Measurement of the Relativistic Sunyaev--Zeldovich Correction in RX J1347. 5-1145}, author = {Butler, Victoria L and Feder, Richard M and Daylan, Tansu and Mantz, Adam B and Mercado, Dale and Monta{\~n}a, Alfredo and Portillo, Stephen KN and Sayers, Jack and Vaughan, Benjamin J and Zemcov, Michael and others}, journal = {The Astrophysical Journal}, volume = {932}, number = {1}, pages = {55}, year = {2022}, publisher = {IOP Publishing} }
[J-2022-02] Buchanan, Natalie and Provenzano, Joules and Padmanabhan, Poornima. (2022). “A Tunable, Particle-Based Model for the Diverse Conformations Exhibited by Chiral Homopolymers.” Macromolecules.
Abstract: Chiral block copolymers capable of hierarchical self-assembly can also exhibit chirality transfer the transfer of chirality at the monomer or conformational scale to the self-assembly. Prior studies focused on experimental and theoretical methods that are unable to fully decouple the thermodynamic origins of chirality transfer and necessitate the development of particle-based models that can be used to quantify intrachain, interchain, and entropic contributions. With this goal in mind, in this work, we developed a parametrized coarse-grained model of a chiral homopolymer and extensively characterized the resulting conformations. Specifically, the energetic parameter in the angular and dihedral potentials, the angular set point, and the dihedral set point are systematically varied to produce a wide range of conformations from a random coil to a nearly ideal helix. The average helicity, pitch, persistence length, and end-to-end distance are measured, and correlations between the model parameters and resulting conformations are obtained. Using available experimental data on model polypeptoid-based chiral polymers, we back out the required parameters that produce similar pitch and persistence length ratios reported in the experiments. The conformations for the experimentally matched chains appear to be somewhat flexible, exhibiting some helical turns. Our model is versatile and can be used to perform molecular dynamics simulations of chiral block copolymers and even sequence-specific polypeptides to study their self-assembly and to gain thermodynamic insights.
Publisher Site: ACS Publications
PDF (Requires Sign-In): PDF
Click for Bibtex
@article{buchanan2022tunable, title = {A Tunable, Particle-Based Model for the Diverse Conformations Exhibited by Chiral Homopolymers}, author = {Buchanan, Natalie and Provenzano, Joules and Padmanabhan, Poornima}, journal = {Macromolecules}, year = {2022}, publisher = {ACS Publications} }
[J-2022-01] Brakensiek, Joshua and Heule, Marijn and Mackey, John and Narváez, David. (2022). “The Resolution of Keller’s Conjecture.” Journal of Automated Reasoning.
Abstract: We consider three graphs, G7,3, G7,4, and G7,6, related to Keller's conjecture in dimension 7. The conjecture is false for this dimension if and only if at least one of the graphs contains a clique of size 2 7 = 128. We present an automated method to solve this conjecture by encoding the existence of such a clique as a propositional formula. We apply satisfiability solving combined with symmetry-breaking techniques to determine that no such clique exists. This result implies that every unit cube tiling of R7 contains a facesharing pair of cubes. Since a faceshare-free unit cube tiling of R8 exists (which we also verify), this completely resolves Keller's conjecture.
Publisher Site: SpringerLink
PDF (Requires Sign-In): PDF
Click for Bibtex
@article{brakensiek2022resolution, title = {The resolution of Keller's conjecture}, author = {Brakensiek, Joshua and Heule, Marijn and Mackey, John and Narv{\'a}ez, David}, journal = {Journal of Automated Reasoning}, pages = {1--24}, year = {2022}, publisher = {Springer} }
[J-2021-09] Buchanan, Natalie and Browka, Krysia and Ketcham, Lianna and Le, Hillary and Padmanabhan, Poornima. (2021). “Conformational and Topological Correlations in Non-Frustated Triblock Copolymers with Homopolymers.” Soft Matter.
Abstract: The phase behavior of non-frustrated ABC block copolymers polymers, modeling poly(isoprene-b-styrene-b-ethylene oxide) (ISO), is studied using dissipative particle dynamic (DPD) simulations. The phase diagram showed a wide composition range for the alternating gyroid morphology, which can be transformed to a chiral metamaterial. A quantitative analysis of topology was developed, that correlates the location of a block relative to the interface with the block's end-to-end distance. This analysis showed that the A-blocks stretched as they were located deeper in the A-rich region. To further expand the stability of the alternating gyroid phase, A-selective homopolymers of different lengths were co-assembled with the ABC copolymer at several compositions. Topological analysis showed that homopolymers with lengths shorter than or equal to the A-block length filled the middle of the networks, decreasing packing frustration and stabilizing them, while longer homopolymers stretched across the network but allowed for the formation of stable, novel morphologies. Adding homopolymers to triblock copolymer melts increases tunability of the network, offering greater control over the final stable phase and bridging two separate regions in the phase diagram.
Publisher Site: RSC.org
PDF (Requires Sign-In): PDF
Click for Bibtex
@article{buchanan2021conformational, title = {Conformational and topological correlations in non-frustated triblock copolymers with homopolymers}, author = {Buchanan, Natalie and Browka, Krysia and Ketcham, Lianna and Le, Hillary and Padmanabhan, Poornima}, journal = {Soft Matter}, volume = {17}, number = {3}, pages = {758--768}, year = {2021}, publisher = {Royal Society of Chemistry} }
[J-2021-08] Uche, Obioma U. and Le, Han G. and Brunner, Logan B. (2021). “Size-selective, Rapid Dynamics of Large, Hetero-epitaxial Islands on fcc(0 0 1) Surfaces.” Computational Materials Science.
Abstract: In this work, we use molecular dynamics simulations to investigate the diffusion of two-dimensional, hexagonal silver islands on copper and nickel substrates below room temperature. Our results indicate that certain sized islands diffuse orders of magnitude faster than other islands and even single atoms under similar conditions. An analysis of several low-energy pathways reveals two governing processes: a rapid, glide-centric process and a slower, vacancy-assisted one. The relative magnitude of the energies required to nucleate a vacancy plays a role in determining the size-selective diffusion of these islands. In addition, we propose a model which can be used to predict magic-sized islands in related systems. These findings should provide insight to future experimental research on the size distribution and shapes observed during the growth of metal-on-metal thin films.
Publisher Site: ScienceDirect
PDF (Requires Sign-In): PDF
Click for Bibtex
@article{uche2021size, title = {Size-selective, rapid dynamics of large, hetero-epitaxial islands on fcc (0 0 1) surfaces}, author = {Uche, Obioma U and Le, Han G and Brunner, Logan B}, journal = {Computational Materials Science}, volume = {188}, pages = {110225}, year = {2021}, publisher = {Elsevier} }
[J-2021-07] Symons, Teresa and Zemcov, Michael and Bock, James and Cheng, Yun-Ting and Crill, Brendan and Hirata, Christopher and Venuto, Stephanie. (2021). “Superresolution Reconstruction of Severely Undersampled Point-spread Functions Using Point-source Stacking and Deconvolution.” The Astrophysical Journal Supplement Series.
Abstract: Point-spread function (PSF) estimation in spatially undersampled images is challenging because large pixels average fine-scale spatial information. This is problematic when fine-resolution details are necessary, as in optimal photometry where knowledge of the illumination pattern beyond the native spatial resolution of the image may be required. Here, we introduce a method of PSF reconstruction where point sources are artificially sampled beyond the native resolution of an image and combined together via stacking to return a finely sampled estimate of the PSF. This estimate is then deconvolved from the pixel-gridding function to return a superresolution kernel that can be used for optimally weighted photometry. We benchmark against the <1% photometric error requirement of the upcoming SPHEREx mission to assess performance in a concrete example. We find that standard methods like Richardson-Lucy deconvolution are not sufficient to achieve this stringent requirement. We investigate a more advanced method with significant heritage in image analysis called iterative back-projection (IBP) and demonstrate it using idealized Gaussian cases and simulated SPHEREx images. In testing this method on real images recorded by the LORRI instrument on New Horizons, we are able to identify systematic pointing drift. Our IBP-derived PSF kernels allow photometric accuracy significantly better than the requirement in individual SPHEREx exposures. This PSF reconstruction method is broadly applicable to a variety of problems and combines computationally simple techniques in a way that is robust to complicating factors such as severe undersampling, spatially complex PSFs, noise, crowded fields, or limited source numbers.
Publisher Site: IOP Science
PDF (Requires Sign-In): PDF
Click for Bibtex
@article{symons2021superresolution, title = {Superresolution Reconstruction of Severely Undersampled Point-spread Functions Using Point-source Stacking and Deconvolution}, author = {Symons, Teresa and Zemcov, Michael and Bock, James and Cheng, Yun-Ting and Crill, Brendan and Hirata, Christopher and Venuto, Stephanie}, journal = {The Astrophysical Journal Supplement Series}, volume = {252}, number = {2}, pages = {24}, year = {2021}, publisher = {IOP Publishing} }
[J-2021-06] Santos, Zackary and Dholabhai, Pratik P. (2021). “Thermodynamic Stability of Defects in Hybrid MoS2/InAs Heterostructures.” Computational Materials Science.
Abstract: Vertical van der Waals heterostructures created from two chemically different two-dimensional (2D) materials have made significant headway in the current electronic manufacturing landscape since they demonstrate enhanced attributes. Recently, among the wide variety of possible van der Waals heterostructures, a 2D molybdenum disulfide (MoS_2) thin film deposited on a 3D indium arsenide (InAs) semiconductor substrate has demonstrated promising properties. To realize the true potential of these hybrid heterostructures, it is imperative to understand their structural stability, as well as the thermodynamic stabilities of various defects at the heterointerface. We report first principles-based electronic structure calculations to study MoS_2(1 0 0)/InAs(1 1 1) heterostructures with two different terminations of the substrate. We present relative stabilities of arsenic-terminated and indium-terminated heterostructures, and shed light on the thermodynamic stabilities of arsenic, indium, and sulfur vacancy defects at the heterointerface. Atomic-scale structure and electronic structure of defects is detailed along with changes in band gaps due to inclusion of vacancy defects. In general, defects are found to be energetically favorable to occur at the interface as compared to the bulk. We offer new insights into the fundamental role of interfaces in hybrid van der Waals heterostructures and their influence on defects, with potential implications for future design of next-generation semiconductors.
Publisher Site: ScienceDirect
PDF (Requires Sign-In): PDF
Click for Bibtex
@article{santos2021thermodynamic, title = {Thermodynamic stability of defects in hybrid MoS2/InAs heterostructures}, author = {Santos, Zackary and Dholabhai, Pratik P}, journal = {Computational Materials Science}, volume = {194}, pages = {110426}, year = {2021}, publisher = {Elsevier} }
[J-2021-05] Meyers, Benjamin S. and Meneely, Andrew. (2021). “An Automated Post-mortem Analysis of Vulnerability Relationships Using Natural Language Word Embeddings.” Procedia Computer Science.
Abstract: The daily activities of cybersecurity experts of and software engineers--code reviews, vulnerability reporting--are constantly contributing to a massive wealth security-specific natural language. In the issue case tracking, of vulnerabilities, understanding their constantly contributing to a massive wealth of security-specific natural language. In the case of vulnerabilities, understanding their causes, consequences, and mitigations is essential to learning from past mistakes and writing better, more secure code in the future. causes, consequences, and mitigations is essential to learning from past mistakes and writing better, more secure code in the future. Many existing vulnerability assessment methodologies, like CVSS, rely on categorization and numerical metrics to glean insights Many existing vulnerability methodologies, CVSS, complexities rely on categorization and numerical to glean because insights into vulnerabilities, but these assessment tools are unable to capture like the subtle and relationships between metrics vulnerabilities into tools natural are unable to capture the subtle complexities and relationships between vulnerabilities they vulnerabilities, do not examine but the these nuanced language artifacts left behind by developers. In this work, we want to discover because unex- they do relationships not examine the nuanced natural language behind by developers. In practices this work, for we post-mortem want to discover unex- pected between vulnerabilities with artifacts the goal left of improving upon current analysis of pected relationships between with the goal of improving upon of current practices for post-mortem analysis of vulnerabilities. To that end, we vulnerabilities trained word embedding models on two corpora vulnerability descriptions from Common Vul- vulnerabilities. To that end, (CVE) we trained word embedding History models Project on two corpora vulnerability descriptions from Common Vul- nerabilities and Exposures and the Vulnerability (VHP), of performed hierarchical agglomerative clustering nerabilities and Exposures and the History Project performed hierarchical agglomerative on word embedding vectors (CVE) representing the Vulnerability overall semantic meaning of (VHP), vulnerability descriptions, and derived insights clustering from vul- on word embedding vectors representing the overall semantic meaning descriptions, and consequences derived insights nerability clusters based on their most common bigrams. We found that of (1) vulnerability vulnerabilities with similar and from based vul- on nerability clusters based on their most common We found (1) vulnerabilities similar consequences and based on similar weaknesses are often clustered together, bigrams. (2) clustering word that embeddings identified with vulnerabilities that need more detailed similar weaknesses are often clustered together, (2) clustering word embeddings identified vulnerabilities that need more detailed descriptions, and (3) clusters rarely contained vulnerabilities from a single software project. Our methodology is automated and descriptions, (3) to clusters rarely contained from all a single methodology is automated and can be easily and applied other natural language vulnerabilities corpora. We release of the software corpora, project. models, Our and code used in our work. can be easily applied to other natural language corpora. We release all of the corpora, models, and code used in our work.
Publisher Site: ScienceDirect
PDF (Requires Sign-In): PDF
Click for Bibtex
@article{meyers2021automated, title = {An automated post-mortem analysis of vulnerability relationships using natural language word embeddings}, author = {Meyers, Benjamin S and Meneely, Andrew}, journal = {Procedia Computer Science}, volume = {184}, pages = {953--958}, year = {2021}, publisher = {Elsevier} }
[J-2021-04] Kothari, Rakshit S. and Chaudhary, Aayush K. and Bailey, Reynold J. and Pelz, Jeff B. and Diaz, Gabriel J. (2021). “EllSeg: An Ellipse Segmentation Framework for Robust Gaze Tracking.” IEEE Transactions on Visualization and Computer Graphics.
Abstract: Ellipse fitting, an essential component in pupil or iris tracking based video oculography, is performed on previously segmented eye parts generated using various computer vision techniques. Several factors, such as occlusions due to eyelid shape, camera position or eyelashes, frequently break ellipse fitting algorithms that rely on well-defined pupil or iris edge segments. In this work, we propose training a convolutional neural network to directly segment entire elliptical structures and demonstrate that such a framework is robust to occlusions and offers superior pupil and iris tracking performance (at least 10% and 24% increase in pupil and iris center detection rate respectively within a two-pixel error margin) compared to using standard eye parts segmentation for multiple publicly available synthetic segmentation datasets.
Publisher Site: IEEE Xplore
PDF (Requires Sign-In): PDF
Click for Bibtex
@article{kothari2021ellseg, title = {Ellseg: An ellipse segmentation framework for robust gaze tracking}, author = {Kothari, Rakshit S and Chaudhary, Aayush K and Bailey, Reynold J and Pelz, Jeff B and Diaz, Gabriel J}, journal = {IEEE Transactions on Visualization and Computer Graphics}, volume = {27}, number = {5}, pages = {2757--2767}, year = {2021}, publisher = {IEEE} }
[J-2021-03] Kishan, K.C. and Subramanya, Sridevi K. and Li, Rui and Cui, Feng. (2021). “Machine Learning Predicts Nucleosome Binding Modes of Transcription Factors.” BMC Bioinformatics.
Abstract: Background: Most transcription factors (TFs) compete with nucleosomes to gain access to their cognate binding sites. Recent studies have identified several TF-nucleosome interaction modes including end binding (EB), oriented binding, periodic binding, dyad binding, groove binding, and gyre spanning. However, there are substantial experimental challenges in measuring nucleosome binding modes for thousands of TFs in different species. Results: We present a computational prediction of the binding modes based on TF protein sequences. With a nested cross-validation procedure, our model outperforms several fine-tuned off-the-shelf machine learning (ML) methods in the multi-label classification task. Our binary classifier for the EB mode performs better than these ML methods with the area under precision-recall curve achieving 75%. The end preference of most TFs is consistent with low nucleosome occupancy around their binding site in GM12878 cells. The nucleosome occupancy data is used as an alternative dataset to confirm the superiority of our EB classifier. Conclusions: We develop the first ML-based approach for efficient and comprehensive analysis of nucleosome binding modes of TFs.
Publisher Site: BMC Bioinformatics
PDF (Requires Sign-In): PDF
Click for Bibtex
@article{kishan2021machine, title = {Machine learning predicts nucleosome binding modes of transcription factors}, author = {Kishan, KC and Subramanya, Sridevi K and Li, Rui and Cui, Feng}, journal = {BMC bioinformatics}, volume = {22}, number = {1}, pages = {1--19}, year = {2021}, publisher = {BioMed Central} }
[J-2021-02] Freewoman, Julia M. and Snape, Rajiv and Cui, Feng. (2021). “Temporal Gene Regulation by p53 is Associated with the Rotational Setting of its Binding Sites in Nucleosomes.” Cell Cycle.
Abstract: The tumor suppressor protein p53 is a DNA-binding transcription factor (TF) that, once activated, coordinates the expression of thousands of target genes. Increased p53 binding to gene promoters occurs shortly after p53 activation. Intriguingly, gene transcription exhibits differential kinetics with some genes being induced early (early genes) and others being induced late (late genes). To understand pre-binding factors contributing to the temporal gene regulation by p53, we performed time-course RNA sequencing experiments in human colon cancer cell line HCT116 treated with fluorouracil to identify early and late genes. Published p53 ChIP fragments co-localized with the early or late genes were used to uncover p53 binding sites (BS). We demonstrate that the BS associated with early genes are clustered around gene starts with decreased nucleosome occupancy. DNA analysis shows that these BS are likely exposed on nucleosomal surface if wrapped into nucleosomes, thereby facilitating stable interactions with and fast induction by p53. By contrast, p53 BS associated with late genes are distributed uniformly across the genes with increased nucleosome occupancy. Predicted rotational settings of these BS show limited accessibility. We therefore propose a hypothetical model in which the BS are fully, partially or not accessible to p53 in the nucleosomal context. The partial accessibility of the BS allows subunits of a p53 tetramer to bind, but the resulting p53-DNA complex may not be stable enough to recruit cofactors, which leads to delayed induction. Our work highlights the importance of DNA conformations of p53 BS in gene expression dynamics.
Publisher Site: Taylor & Francis
PDF (Requires Sign-In): PDF
Click for Bibtex
@article{freewoman2021temporal, title = {Temporal gene regulation by p53 is associated with the rotational setting of its binding sites in nucleosomes}, author = {Freewoman, Julia M and Snape, Rajiv and Cui, Feng}, journal = {Cell Cycle}, volume = {20}, number = {8}, pages = {792--807}, year = {2021}, publisher = {Taylor \& Francis} }
[J-2021-01] Eon, Rehman S. and Bachmann, Charles M. (2021). “Mapping Barrier Island Soil Moisture Using a Radiative Transfer Model of Hyperspectral Imagery from an Unmanned Aerial System.” Scientific Reports.
Abstract: The advent of remote sensing from unmanned aerial systems (UAS) has opened the door to more affordable and effective methods of imaging and mapping of surface geophysical properties with many important applications in areas such as coastal zone management, ecology, agriculture, and defense. We describe a study to validate and improve soil moisture content retrieval and mapping from hyperspectral imagery collected by a UAS system. Our approach uses a recently developed model known as the multilayer radiative transfer model of soil reflectance (MARMIT). MARMIT partitions contributions due to water and the sediment surface into equivalent but separate layers and describes these layers using an equivalent slab model formalism. The model water layer thickness along with the fraction of wet surface become parameters that must be optimized in a calibration step, with extinction due to water absorption being applied in the model based on equivalent water layer thickness, while transmission and reflection coefficients follow the Fresnel formalism. In this work, we evaluate the model in both field settings, using UAS hyperspectral imagery, and laboratory settings, using hyperspectral spectra obtained with a goniometer. Sediment samples obtained from four different field sites representing disparate environmental settings comprised the laboratory analysis while field validation used hyperspectral UAS imagery and coordinated ground truth obtained on a barrier island shore during field campaigns in 2018 and 2019. Analysis of the most significant wavelengths for retrieval indicate a number of different wavelengths in the short-wave infra-red (SWIR) that provide accurate fits to measured soil moisture content in the laboratory with normalized root mean square error (NRMSE)< 0.145, while independent evaluation from sequestered test data from the hyperspectral UAS imagery obtained during the field campaign obtained an average NRMSE = 0.169 and median NRMSE = 0.152 in a bootstrap analysis.
Publisher Site: Nature
PDF (Requires Sign-In): PDF
Click for Bibtex
@article{eon2021mapping, title = {Mapping barrier island soil moisture using a radiative transfer model of hyperspectral imagery from an unmanned aerial system}, author = {Eon, Rehman S and Bachmann, Charles M}, journal = {Scientific Reports}, volume = {11}, number = {1}, pages = {1--11}, year = {2021}, publisher = {Nature Publishing Group} }
[J-2020-04] Shah, Ekta A. and Kartaltepe, Jeyhan S. and Magagnoli, Christina T. and Cox, Isabella G. and Wetherell, Caleb T. and Vanderhoof, Brittany N. and Calabro, Antonello and Chartab, Nima and Conselice, Christopher J. and Croton, Darren J. and Others. (2020). “Investigating the Effect of Galaxy Interactions on the Enhancement of Active Galactic Nuclei at 0.5 < z < 3.0.” The Astrophysical Journal.
Abstract: Galaxy interactions and mergers are thought to play an important role in the evolution of galaxies. Studies in the nearby universe show a higher fraction of active galactic nuclei (AGNs) in interacting and merging galaxies than in their isolated counterparts, indicating that such interactions are important contributors to black hole growth. To investigate the evolution of this role at higher redshifts, we have compiled the largest known sample of major spectroscopic galaxy pairs (2381 with ΔV < 5000 km s-1 ) at 0.5 < z < 3.0 from observations in the COSMOS and CANDELS surveys. We identify X-ray and IR AGNs among this kinematic pair sample, a visually identified sample of mergers and interactions, and a mass-, redshift-, and environment-matched control sample for each in order to calculate AGN fractions and the level of AGN enhancement as a function of relative velocity, redshift, and X-ray luminosity. While we see a slight increase in AGN fraction with decreasing projected separation, overall, we find no significant enhancement relative to the control sample at any separation. In the closest projected separation bin (< 25 kpc, ΔV < 1000 km s-1), we find enhancements of a factor of 0.94+0.21-0.16 and 1.00+0.58-0.31 for X-ray and IRselected AGNs, respectively. While we conclude that galaxy interactions do not significantly enhance AGN activity on average over 0.5 < z < 3.0 at these separations, given the errors and the small sample size at the closest projected separations, our results would be consistent with the presence of low-level AGN enhancement.
Publisher Site: IOP Science
PDF (Requires Sign-In): PDF
Click for Bibtex
@article{shah2020investigating, title = {Investigating the Effect of Galaxy Interactions on the Enhancement of Active Galactic Nuclei at 0.5< z< 3.0}, author = {Shah, Ekta A and Kartaltepe, Jeyhan S and Magagnoli, Christina T and Cox, Isabella G and Wetherell, Caleb T and Vanderhoof, Brittany N and Calabro, Antonello and Chartab, Nima and Conselice, Christopher J and Croton, Darren J and others}, journal = {The Astrophysical Journal}, volume = {904}, number = {2}, pages = {107}, year = {2020}, publisher = {IOP Publishing} }
[J-2020-03] Kothari, Rakshit and Yang, Zhizhuo and Kanan, Christopher and Bailey, Reynold and Pelz, Jeff B. and Diaz, Gabriel J. (2020). “Gaze-in-wild: A Dataset for Studying Eye and Head Coordination in Everyday Activities.” Scientific Reports.
Abstract: The study of gaze behavior has primarily been constrained to controlled environments in which the head is fixed. Consequently, little effort has been invested in the development of algorithms for the categorization of gaze events (e.g. fixations, pursuits, saccade, gaze shifts) while the head is free, and thus contributes to the velocity signals upon which classification algorithms typically operate. Our approach was to collect a novel, naturalistic, and multimodal dataset of eye + head movements when subjects performed everyday tasks while wearing a mobile eye tracker equipped with an inertial measurement unit and a 3D stereo camera. This Gaze-in-the-Wild dataset (GW) includes eye + head rotational velocities (deg/s), infrared eye images and scene imagery (RGB + D). A portion was labelled by coders into gaze motion events with a mutual agreement of 0.74 sample based Cohen's kappa. This labelled data was used to train and evaluate two machine learning algorithms, Random Forest and a Recurrent Neural Network model, for gaze event classification. Assessment involved the application of established and novel event based performance metrics. Classifiers achieve ~87% human performance in detecting fixations and saccades but fall short (50%) on detecting pursuit movements. Moreover, pursuit classification is far worse in the absence of head movement information. A subsequent analysis of feature significance in our best performing model revealed that classification can be done using only the magnitudes of eye and head movements, potentially removing the need for calibration between the head and eye tracking systems. The GW dataset, trained classifiers and evaluation metrics will be made publicly available with the intention of facilitating growth in the emerging area of head-free gaze event classification.
Publisher Site: Nature
PDF (Requires Sign-In): PDF
Click for Bibtex
@article{kothari2020gaze, title = {Gaze-in-wild: A dataset for studying eye and head coordination in everyday activities}, author = {Kothari, Rakshit and Yang, Zhizhuo and Kanan, Christopher and Bailey, Reynold and Pelz, Jeff B and Diaz, Gabriel J}, journal = {Scientific reports}, volume = {10}, number = {1}, pages = {1--18}, year = {2020}, publisher = {Nature Publishing Group} }
[J-2020-02] Gancio, Guillermo and Lousto, Carlos Oscar and Combi, Luciano and del Palacio, Santiago and Armengol, F.G. López and Combi, Jorge Ariel and García, Federico and Kornecki, Paula and Müller, Ana Laura and Gutiérrez, E. and Others. (2020). “Upgraded Antennas for Pulsar Observations in the Argentine Institute of Radio Astronomy.” Astronomy & Astrophysics.
Abstract: Context. The Argentine Institute of Radio astronomy (IAR) is equipped with two single-dish 30 m radio antennas capable of performing daily observations of pulsars and radio transients in the southern hemisphere at 1.4 GHz. Aims. We aim to introduce to the international community the upgrades performed and to show that the IAR observatory has become suitable for investigations in numerous areas of pulsar radio astronomy, such as pulsar timing arrays, targeted searches of continuous gravitational waves sources, monitoring of magnetars and glitching pulsars, and studies of a short time scale interstellar scintillation. Methods. We refurbished the two antennas at IAR to achieve high-quality timing observations. We gathered more than 1 000 hours of observations with both antennas in order to study the timing precision and sensitivity they can achieve. Results. We introduce the new developments for both radio telescopes at IAR. We present daily observations of the millisecond pulsar J0437-4715 with timing precision better than 1 microseconds. We also present a follow-up of the reactivation of the magnetar XTE J1810-197 and the measurement and monitoring of the latest (Feb. 1, 2019) glitch of the Vela pulsar (J0835-4510). Conclusions. We show that IAR is capable of performing pulsar monitoring in the 1.4 GHz radio band for long periods of time with a daily cadence. This opens up the possibility of pursuing several goals in pulsar science, including coordinated multi-wavelength observations with other observatories. In particular, daily observations of the millisecond pulsar J0437-4715 would increase the sensitivity of pulsar timing arrays. We also show IAR's great potential for studying targets of opportunity and transient phenomena, such as magnetars, glitches, and fast-radio-burst sources.
Publisher Site: EDP Sciences
PDF (Requires Sign-In): PDF
Click for Bibtex
@article{gancio2020upgraded, title = {Upgraded antennas for pulsar observations in the Argentine Institute of Radio astronomy}, author = {Gancio, Guillermo and Lousto, Carlos Oscar and Combi, Luciano and del Palacio, Santiago and Armengol, FG L{\'o}pez and Combi, Jorge Ariel and Garc{\'\i}a, Federico and Kornecki, Paula and M{\"u}ller, Ana Laura and Guti{\'e}rrez, E and others}, journal = {Astronomy \& Astrophysics}, volume = {633}, pages = {A84}, year = {2020}, publisher = {EDP Sciences} }
[J-2020-01] Blakeslee, Bryan and Savakis, Andreas. (2020). “LambdaNet: A Fully Convolutional Architecture for Directional Change Detection.” Electronic Imaging.
Abstract: Change detection in image pairs has traditionally been a binary process, reporting either "Change" or "No Change." In this paper, we present LambdaNet, a novel deep architecture for performing pixel-level directional change detection based on a four class classification scheme. LambdaNet successfully incorporates the notion of "directional change" and identifies differences between two images as "Additive Change" when a new object appears, "Subtractive Change" when an object is removed, "Exchange" when different objects are present in the same location, and "No Change." To obtain pixel annotated change maps for training, we generated directional change class labels for the Change Detection 2014 dataset. Our tests illustrate that LambdaNet would be suitable for situations where the type of change is unstructured, such as change detection scenarios in satellite imagery.
Publisher Site: Electronic Imaging
PDF (Requires Sign-In): PDF
Click for Bibtex
@article{blakeslee2020lambdanet, title = {Lambdanet: A fully convolutional architecture for directional change detection}, author = {Blakeslee, Bryan and Savakis, Andreas}, journal = {Electronic Imaging}, volume = {2020}, number = {8}, pages = {114--1}, year = {2020}, publisher = {Society for Imaging Science and Technology} }
[J-2019-01] Narde, Rounak Singh and Venkataraman, Jayanti and Ganguly, Amlan and Puchades, Ivan. (2019). “Intra- and Inter-Chip Transmission of Millimeter-Wave Interconnects in NoC-Based Multi-Chip Systems.” IEEE Access.
Abstract: The primary objective of this paper is to investigate the communication capabilities of short-range millimeter-wave (mmWave) communication among network-on-chip (NoC)-based multi-core processors integrated on a substrate board. This paper presents the characterization of transmission between on-chip antennas for both intra- and inter-chip communication in multi-chip computing systems, such as server blades or embedded systems. Through simulation at 30 GHz, we have characterized the inter-chip transmission and studied the electric field distribution to explain the transmission characteristics. It is shown that the antenna radiation efficiency reduces with a decrease in the resistivity of silicon. The simulation results have been validated with fabricated antennas in different orientations on silicon dies that can communicate with inter-chip transmission coefficients ranging from -45 to -60 dB while sustaining bandwidths up to 7 GHz. Using measurements, a large-scale log-normal channel model is derived, which can be used for system-level architecture design. Using the same simulation environment, we perform design and analysis at 60 GHz to provide another non-interfering frequency channel for inter-chip communication in order to increase the physical bandwidth of the interconnection architecture. Furthermore, densely packed multilayer copper wires in NoCs have been modeled in this paper to study their impact on the wireless transmission for both intra- and inter-chip links. The dense orthogonal multilayer wires are shown to be equivalent to copper sheets. In addition, we have shown that the antenna radiation efficiency reduces in the presence of these densely packed wires placed in the close proximity of the antenna elements. Using this model, the reduction of inter-chip transmission is quantified to be about 20 dB compared with a system with no wires. Furthermore, the transmission characteristics of the antennas resonating at 60 GHz in a flip-chip packaging environment are also presented.
Publisher Site: IEEE Xplore
PDF (Requires Sign-In): PDF
Click for Bibtex
@article{narde2019intra, title = {Intra-and inter-chip transmission of millimeter-wave interconnects in NoC-based multi-chip systems}, author = {Narde, Rounak Singh and Venkataraman, Jayanti and Ganguly, Amlan and Puchades, Ivan}, journal = {IEEE Access}, volume = {7}, pages = {112200--112215}, year = {2019}, publisher = {IEEE} }
[J-2017-01] Munaiah, Nuthan and Kroh, Steven and Cabrey, Craig and Nagappan, Meiyappan. (2017). “Curating GitHub for Engineered Software Projects.” Empirical Software Engineering.
Abstract: Software forges like GitHub host millions of repositories. Software engineering researchers have been able to take advantage of such a large corpora of potential study subjects with the help of tools like GHTorrent and Boa. However, the simplicity in querying comes with a caveat: there are limited means of separating the signal (e.g. repositories containing engineered software projects) from the noise (e.g. repositories containing home work assignments). The proportion of noise in a random sample of repositories could skew the study and may lead to researchers reaching unrealistic, potentially inaccurate, conclusions. We argue that it is imperative to have the ability to sieve out the noise in such large repository forges. We propose a framework, and present a reference implementation of the framework as a tool called reaper, to enable researchers to select GitHub repositories that contain evidence of an engineered software project. We identify software engineering practices (called dimensions) and propose means for validating their existence in a GitHub repository. We used reaper to measure the dimensions of 1,857,423 GitHub repositories. We then used manually classified data sets of repositories to train classifiers capable of predicting if a given GitHub repository contains an engineered software project. The performance of the classifiers was evaluated using a set of 200 repositories with known ground truth classification. We also compared the performance of the classifiers to other approaches to classification (e.g. number of GitHub Stargazers) and found our classifiers to outperform existing approaches. We found stargazers-based classifier (with 10 as the threshold for number of stargazers) to exhibit high precision (97%) but an inversely proportional recall (32%). On the other hand, our best classifier exhibited a high precision (82%) and a high recall (86%). The stargazer-based criteria offers precision but fails to recall a significant portion of the population.
Publisher Site: SpringerLink
PDF (Requires Sign-In): PDF
Click for Bibtex
@article{munaiah2017curating, title = {Curating github for engineered software projects}, author = {Munaiah, Nuthan and Kroh, Steven and Cabrey, Craig and Nagappan, Meiyappan}, journal = {Empirical Software Engineering}, volume = {22}, pages = {3219--3253}, year = {2017}, publisher = {Springer} }
[J-2016-01] Shamim, Md Shahriar and Mansoor, Naseef and Narde, Rounak Singh and Kothandapani, Vignesh and Ganguly, Amlan and Venkataraman, Jayanti. (2016). “A Wireless Interconnection Framework for Seamless Inter and Intra-Chip Communication in Multichip Systems.” IEEE Transactions on Computers.
Abstract: Computing modules in typical data center nodes or server racks consist of several multicore chips either on a board or in a System-in-Package (SiP) environment. State-of-the-art inter-chip communication over wireline channels require data signals to travel from internal nets to the peripheral I/O ports and then get routed over the inter-chip channels to the I/O port of the destination chip. Following this, the data is finally routed from the I/O to internal nets of the destination chip over a wireline interconnect fabric. This multihop communication increases energy consumption while decreasing data bandwidth in a multichip system. Also, traditional I/O does not scale well with technology generations due to limitations of pitch. Moreover, intra-chip and inter-chip communication protocol within such a multichip system is often decoupled to facilitate design flexibility. However, a seamless interconnection between on-chip and off-chip data transfer can improve the communication efficiency significantly. Here, we propose the design of a seamless hybrid wired and wireless interconnection network for multichip systems with dimensions spanning up to tens of centimeters with on-chip wireless transceivers. We demonstrate with cycle accurate simulations that such a design increases the bandwidth and reduces the energy consumption in comparison to state-of-the-art wireline I/O based multichip communication.
Publisher Site: IEEE Xplore
PDF (Requires Sign-In): PDF
Click for Bibtex
@article{shamim2016wireless, title = {A wireless interconnection framework for seamless inter and intra-chip communication in multichip systems}, author = {Shamim, Md Shahriar and Mansoor, Naseef and Narde, Rounak Singh and Kothandapani, Vignesh and Ganguly, Amlan and Venkataraman, Jayanti}, journal = {IEEE Transactions on Computers}, volume = {66}, number = {3}, pages = {389--402}, year = {2016}, publisher = {IEEE} }
Conference Papers
[C-2024-08] Nau, Calvin and Sankaranb, Prashant and McConkya, Katie and Suditb, Moises and Khazaelpoura, Payam and Velasquezc, Alvaro. (2024). “A Flexible and Synthetic Transplant Kidney Exchange Problem (FASTKEP) Data Generator.” IISE Annual Conference.
Abstract:The Kidney Exchange Problem (KEP) optimizes a pool of incompatible patient-donor pairs and non-directed donors to determine the optimal cycles and chains of length-N that maximize transplants. A direct impact of KEP optimization is an improved quality of life for transplant recipients at a lower cost to the healthcare system (as opposed to dialysis treatments). To improve this process, synthetic data is commonly used to test and develop KEP algorithms. The canonical KEP data generation process is referred to as the Saidman Generator. The generator utilizes attributes of the donors and recipients that determine compatibility, such as blood type and protein compatibility, alongside known data distributions, including the percentage of different blood types comprising an exchange, to create datasets that aim to mirror the real world. Prior published Java implementations of this generator exist. However, in this work, an open-source data generation package implemented in Python is proposed for the KEP which mirrors the Saidman generator's output. The proposed implementation is compared to a common Java implementation for scalability and efficiency. This generator allows for future extensions that integrate additional recipient and donor features that produce data better matching real-world exchanges. The result is an open-source Python implementation of a KEP data generator. The efficient creation of directed exchange datasets enables future work, including the use of the presented data generation process by ML/AI researchers in application to large-scale machine learning model training.
Publisher Site: ProQuest
PDF (Requires Sign-In): PDF
Click for Bibtex
@inproceedings{naua2024flexible, title = {A Flexible and Synthetic Transplant Kidney Exchange Problem (FASTKEP) Data Generator}, author = {Nau, Calvin and Sankaranb, Prashant and McConkya, Katie and Suditb, Moises and Khazaelpoura, Payam and Velasquezc, Alvaro}, booktitle = {IISE Annual Conference. Proceedings}, pages = {1--6}, year = {2024}, organization = {Institute of Industrial and Systems Engineers (IISE)} }
[C-2024-07] Rao, Aishwarya and Krishnan, Narayanan Asuri and Rivero, Carlos R. (2024). “Using Model Calibration to Evaluate Link Prediction in Knowledge Graphs.” ACM on Web.
Abstract:Link prediction models assign scores to predict new, plausible edges to complete knowledge graphs. In link prediction evaluation, the score of an existing edge (positive) is ranked w.r.t. the scores of its synthetically corrupted counterparts (negatives). An accurate model ranks positives higher than negatives, assuming ascending order. Since the number of negatives are typically large for a single positive, link prediction evaluation is computationally expensive. As far as we know, only one approach has proposed to replace rank aggregations by a distance between sample positives and negatives. Unfortunately, the distance does not consider individual ranks, so edges in isolation cannot be assessed. In this paper, we propose an alternative protocol based on posterior probabilities of positives rather than ranks. A calibration function assigns posterior probabilities to edges that measure their plausibility. We propose to assess our alternative protocol in various ways, including whether expected semantics are captured when using different strategies to synthetically generate negatives. Our experiments show that posterior probabilities and ranks are highly correlated. Also, the time reduction of our alternative protocol is quite significant: more than 77% compared to rank-based evaluation. We conclude that link prediction evaluation based on posterior probabilities is viable and significantly reduces computational costs.
Publisher Site: ACM Digital Library
PDF (Requires Sign-In): PDF
Click for Bibtex
@inproceedings{rao2024using, title = {Using Model Calibration to Evaluate Link Prediction in Knowledge Graphs}, author = {Rao, Aishwarya and Krishnan, Narayanan Asuri and Rivero, Carlos R}, booktitle = {Proceedings of the ACM on Web Conference 2024}, pages = {2042--2051}, year = {2024} }
[C-2024-06] Krishnan, Narayanan Asuri and Rivero, Carlos R. (2024). “A Method for Assessing Inference Patterns Captured by Embedding Models in Knowledge Graphs.” ACM on Web.
Abstract:Various methods embed knowledge graphs with the goal of predicting missing edges. Inference patterns are the logical relationships that occur in a graph. To make proper predictions, models trained by embedding methods must capture inference patterns. There are several theoretical analyses studying pattern-capturing capabilities. Unfortunately, these analyses are challenging and many embedding methods remain unstudied. Also, they do not quantify how accurately a pattern is captured in real-world datasets. Existing empirical studies have studied a small subset of simple inference patterns, and the analysis methods used have varied depending on the models evaluated. In this paper, we present a model-agnostic method to empirically quantify how patterns are captured by trained embedding models. We collect the most plausible predictions to form a new graph, and use it to globally assess pattern-capturing capabilities. For a given pattern, we study positive and negative evidence, i.e., edges that the pattern deems correct and incorrect based on the partial completeness assumption. As far as we know, it is the first time negative evidence is analyzed. Our experiments show that several models effectively capture the positive evidence of inference patterns. However, the performance is poor for negative evidence, which entails that models fail to learn the partial completeness assumption. We also identify new inference patterns not studied before. Surprisingly, models generally achieve better performance in these new patterns that we introduce.
Publisher Site: ACM Digital Library
PDF (Requires Sign-In): PDF
Click for Bibtex
@inproceedings{krishnan2024method, title = {A Method for Assessing Inference Patterns Captured by Embedding Models in Knowledge Graphs}, author = {Krishnan, Narayanan Asuri and Rivero, Carlos R}, booktitle = {Proceedings of the ACM on Web Conference 2024}, pages = {2030--2041}, year = {2024} }
[C-2024-05] Ranjan, Navin and Savakis, Andreas. (2024). “Vision Transformer Quantization with Multi-Step Knowledge Distillation.” Signal Processing, Sensor/Information Fusion, and Target Recognition.
Abstract:Vision Transformers (ViTs) have demonstrated remarkable performance in various visual tasks, but they suffer from expensive computational and memory challenges, which hinder their practical application in the real world. Model quantization methods reduce the model computation and memory requirements through low-bit representations. Knowledge distillation is used to guide the quantized student network to imitate the performance of its full-precision counterpart teacher network. However, for ultra-low bit quantization, the student networks experience a noticeable performance drop. This is primarily due to the limited learning capacity of the smaller network to capture the knowledge of the full-precision teacher, especially when the representation gaps between the student and the teacher networks are significant. In this paper, we introduce a multi-step knowledge distillation approach, utilizing intermediate-quantized networks with varying bit precision. This multi-step knowledge distillation approach enables an ultra-low bit quantized student network to effectively bridge the gap with the teacher network by gradually reducing the model’s bit representation. We progressively teach each TA network to learn by distilling the knowledge from higher-bit quantized teacher networks from the previous step. The target student network learns from the combined knowledge of the teacher assistants and the full-precision teacher network, resulting in improved learning capacity even when faced with significant knowledge gaps. We evaluate our methods using the DeiT vision transformer for both ground level and aerial image classification tasks.
Publisher Site: SPIE Digital Library
PDF (Requires Sign-In): PDF
Click for Bibtex
@inproceedings{ranjan2024vision, title = {Vision transformer quantization with multi-step knowledge distillation}, author = {Ranjan, Navin and Savakis, Andreas}, booktitle = {Signal Processing, Sensor/Information Fusion, and Target Recognition XXXIII}, volume = {13057}, pages = {283--292}, year = {2024}, organization = {SPIE} }
[C-2024-04] Sahay, Rajat and Savakis, Andreas. (2024). “On Aligning SAM to Remote Sensing Data.” Geospatial Informatics.
Abstract:The Segment Anything Model (SAM) has demonstrated exceptional capabilities for object segmentation in various settings. In this work, we focus on the remote sensing domain and examine whether SAM’s performance can be improved for overhead imagery and geospatial data. Our evaluation indicates that directly applying the pretrained SAM model to aerial imagery does not yield satisfactory performance due to the domain gap between natural and aerial images. To bridge this gap, we utilize three parameter-efficient fine-tuning strategies and evaluate SAM’s performance across a set of diverse benchmarks. Our results show that while a vanilla SAM model lacks the intrinsic ability to generate accurate masks for smaller objects often found in overhead imagery, fine-tuning greatly improves performance and produces results comparable to current state-of-the-art techniques.
Publisher Site: SPIE Digital Library
PDF (Requires Sign-In): PDF
Click for Bibtex
@inproceedings{sahay2024aligning, title = {On aligning SAM to remote sensing data}, author = {Sahay, Rajat and Savakis, Andreas}, booktitle = {Geospatial Informatics XIV}, volume = {13037}, pages = {10--18}, year = {2024}, organization = {SPIE} }
[C-2024-03] Kundu, Bipasha and Yang, Zixin and Simon, Richard and Linte, Cristian. (2024). “Comparative Analysis of Non-Rigid Registration Techniques for Liver Surface Registration.” Medical Imaging.
Abstract: Non-rigid surface-based soft tissue registration is crucial for surgical navigation systems, but its adoption still faces several challenges due to the large number of degrees of freedom and the continuously varying and complex surface structures present in the intra-operative data. By employing non-rigid registration, surgeons can integrate the pre-operative images into the intra-operative guidance environment, providing real-time visualization of the patient's complex pre- and intra-operative anatomy in a common coordinate system to improve navigation accuracy. However, many of the existing registration methods, including those for liver applications, are inaccessible to the broader community. To address this limitation, we present a comparative analysis of several open-source, non-rigid surface-based liver registration algorithms, with the overall goal of contrasting their strength and weaknesses and identifying an optimal solution. We compared the robustness of three optimization-based and one data-driven nonrigid registration algorithms in response to a reduced visibility ratio (reduced partial views of the surface) and to an increasing deformation level (mean displacement), reported as the root mean square error (RMSE) between the pre-and intra-operative liver surface meshed following registration. Our results indicate that the Gaussian Mixture Model - Finite Element Model (GMM-FEM) method consistently yields a lower post-registration error than the other three tested methods in the presence of both reduced visibility ratio and increased intra-operative surface displacement, therefore offering a potentially promising solution for pre- to intra-operative nonrigid liver surface registration.
Publisher Site: SPIE
PDF (Requires Sign-In): PDF
Click for Bibtex
@inproceedings{kundu2024comparative, title = {Comparative analysis of non-rigid registration techniques for liver surface registration}, author = {Kundu, Bipasha and Yang, Zixin and Simon, Richard and Linte, Cristian}, booktitle = {Medical Imaging 2024: Image-Guided Procedures, Robotic Interventions, and Modeling}, volume = {12928}, pages = {520--526}, year = {2024}, organization = {SPIE} }
[C-2024-02] Meyers, Benjamin S. and Meneely, Andrew. (2024). “Taxonomy-Based Human Error Assessment for Senior Software Engineering Students.” ACM Technical Symposium on Computer Science Education.
Abstract: Software engineering (SE) is a complex symphony of development activities spanning multiple engineering phases. Despite best efforts, software engineers experience human errors. Human error theory from psychology has been studied in the context of SE, but human error assessment has yet to be adopted as part of typical post-mortem activities in SE. Our goal in this work is to evaluate an existing Taxonomy of Human Errors in Software Engineering (T.H.E.S.E.) as a learning tool for software engineering students. We conducted a user study involving five SE students at the Rochester Institute of Technology (RIT). In two experimental phases (17 weeks total), participants self-reported 162 human errors that they experienced during software development. Participants' feedback collected via surveys indicates that T.H.E.S.E. is clear, simple to use, and general to all phases of SE. Participants also indicated that human error assessment guided by T.H.E.S.E. (1) would benefit other students and professional software engineers and (2) enhanced their understanding of human errors in SE and of their own human errors. These are promising results indicating that human error assessment facilitated by T.H.E.S.E. is a valuable learning experience for software engineering students. We release anonymized survey responses and reported human errors. Future work should examine T.H.E.S.E. as a learning tool for professional software developers and examine other SE artifacts to identify categories of human error that are not presently captured by T.H.E.S.E.
Publisher Site: ACM Digital Library
PDF (Requires Sign-In): PDF
Click for Bibtex
@inproceedings{meyers2024taxonomy, titlei = {Taxonomy-Based Human Error Assessment for Senior Software Engineering Students}, author = {Meyers, Benjamin S. and Meneely, Andrew}, booktitle = {Proceedings of the 55th ACM Technical Symposium on Computer Science Education V. 1}, pages = {840--846}, year = {2024} }
[C-2024-01] Que, Xiaofan and Yu, Qi. (2024). “Dual-Level Curriculum Meta-Learning for Noisy Few-Shot Learning Tasks.” AAAI Conference on Artificial Intelligence.
Abstract: Few-shot learning (FSL) is essential in many practical applications. However, the limited training examples make the models more vulnerable to label noise, which can lead to poor generalization capability. To address this critical challenge, we propose a curriculum meta-learning model that employs a novel dual-level class-example sampling strategy to create a robust curriculum for adaptive task distribution formulation and robust model training. The dual-level framework proposes a heuristic class sampling criterion that measures pairwise class boundary complexity to form a class curriculum; it uses effective example sampling through an under-trained proxy model to form an example curriculum. By utilizing both class-level and example-level information, our approach is more robust to handle limited training data and noisy labels that commonly occur in few-shot learning tasks. The model has efficient convergence behavior, which is verified through rigorous convergence analysis. Additionally, we establish a novel error bound through a hierarchical PAC-Bayesian analysis for curriculum meta-learning under noise. We conduct extensive experiments that demonstrate the effectiveness of our framework in outperforming existing noisy few-shot learning methods under various few-shot classification benchmarks. Our code is available at https://github.com/ritmininglab/DCML.
Publisher Site: AAAI
PDF (Requires Sign-In): PDF
Click for Bibtex
@inproceedings{que2024dual, title = {Dual-Level Curriculum Meta-Learning for Noisy Few-Shot Learning Tasks}, author = {Que, Xiaofan and Yu, Qi}, booktitle = {Proceedings of the AAAI Conference on Artificial Intelligence}, volume = {38}, number = {13}, pages = {14740--14748}, year = {2024} }
[C-2023-08] Thakur, Aditya Shankar and Awari, Akshar Bajrang and Lyu, Zimeng and Desell, Travis. (2023). “Efficient Neuroevolution Using Island Repopulation and Simplex Hyperparameter Optimization.” IEEE Symposium Series on Computational Intelligence.
Abstract: Recent studies have shown that the performance of evolutionary neural architecture search (i.e., neuroevolution) algorithms can be significantly improved by the use of island based strategies which periodically experience extinction and repopulation events. Further, it has been shown that the simplex hyperparameter optimization (SHO) method can also improve neuroevolution (NE) performance by optimizing neural network training hyperparameters while the NE algorithm also trains and designs neural networks. This work provides an extensive examination of combining island repopulation events with five different island-based variations of SHO. These methods are evaluated for the evolution of recurrent neural networks for the challenging problem of multivariate time series forecasting on two real world datasets. We show with statistical significance that adding repopulation to the SHO variants in almost every case improves performance, and for those that does there is no statistical difference. In addition, we find that one variant in particular, multi-island, random island best genome (MIRIB) performs the best across all experiment types.
Publisher Site: IEEE Xplore
PDF (Requires Sign-In): PDF
Click for Bibtex
@inproceedings{thakur2023efficient, title = {Efficient Neuroevolution Using Island Repopulation and Simplex Hyperparameter Optimization}, author = {Thakur, Aditya Shankar and Awari, Akshar Bajrang and Lyu, Zimeng and Desell, Travis}, booktitle = {2023 IEEE Symposium Series on Computational Intelligence (SSCI)}, pages = {1837--1842}, year = {2023}, organization = {IEEE} }
[C-2023-07] Krishnan, Narayanan Asuri and Rivero, Carlos R. (2023). “A Model-Agnostic Method to Interpret Link Prediction Evaluation of Knowledge Graph Embeddings.” ACM International Conference on Information and Knowledge Management.
Abstract: In link prediction evaluation, an embedding model assigns plausibility scores to unseen triples in a knowledge graph using an input partial triple. Performance metrics like mean rank are useful to compare models side by side, but do not shed light on their behavior. Interpreting link prediction evaluation and comparing models based on such interpretation are appealing. Current interpretation methods have mainly focused on single predictions or other tasks different from link prediction. Since knowledge graph embedding methods are diverse, interpretation methods that are applicable only to certain machine learning approaches cannot be used. In this paper, we propose a model-agnostic method for interpreting link prediction evaluation as a whole. The interpretation consists of Horn rules mined from the knowledge graph containing the triples a model deems plausible. We combine precision and recall measurements of mined rules using Fβ score to quantify interpretation accuracy. To maximize interpretation accuracy when comparing models, we study two approximations to the hard problem of merging rules. Our quantitative study shows that interpretation accuracy serves to compare diverse models side by side, and that these comparisons are different from those using ranks. Our qualitative study shows that several models globally capture expected semantics, and that models make a common set of predictions despite of redundancy reduction.
Publisher Site: ACM Digital Library
PDF (Requires Sign-In): PDF
Click for Bibtex
@inproceedings{krishnan2023model, title = {A Model-Agnostic Method to Interpret Link Prediction Evaluation of Knowledge Graph Embeddings}, author = {Krishnan, Narayanan Asuri and Rivero, Carlos R}, booktitle = {Proceedings of the 32nd ACM International Conference on Information and Knowledge Management}, pages = {1107--1116}, year = {2023} }
[C-2023-06] Jimerson, Robert and Liu, Zoey and Prud’hommeaux, Emily. (2023). “An (Unhelpful) Guide to Selecting the Best ASR Architecture for Your Under-Resourced Language.” Association for Computational Linguistics.
Abstract: Advances in deep neural models for automatic speech recognition (ASR) have yielded dramatic improvements in ASR quality for resource-rich languages, with English ASR now achieving word error rates comparable to that of human transcribers. The vast majority of the world's languages, however, lack the quantity of data necessary to approach this level of accuracy. In this paper we use four of the most popular ASR toolkits to train ASR models for eleven languages with limited ASR training resources: eleven widely spoken languages of Africa, Asia, and South America, one endangered language of Central America, and three critically endangered languages of North America. We find that no single architecture consistently outperforms any other. These differences in performance so far do not appear to be related to any particular feature of the datasets or characteristics of the languages. These findings have important implications for future research in ASR for under-resourced languages. ASR systems for languages with abundant existing media and available speakers may derive the most benefit simply by collecting large amounts of additional acoustic and textual training data. Communities using ASR to support endangered language documentation efforts, who cannot easily collect more data, might instead focus on exploring multiple architectures and hyperparameterizations to optimize performance within the constraints of their available data and resources.
Publisher Site: ACL Anthology
PDF (Requires Sign-In): PDF
Click for Bibtex
@inproceedings{jimerson2023unhelpful, title = {An (unhelpful) guide to selecting the best ASR architecture for your under-resourced language}, author = {Jimerson, Robert and Liu, Zoey and Prud'hommeaux, Emily}, booktitle = {Proceedings of the 61st Annual Meeting of the Association for Computational Linguistics (Volume 2: Short Papers)}, pages = {1008--1016}, year = {2023} }
[C-2023-05] Regmi, Paribesh and Li, Rui. (2023). “AdaVAE: Bayesian Structural Adaptation for Variational Autoencoders.” Conference on Neural Information Processing Systems.
Abstract: The neural network structures of generative models and their corresponding infenrence models paired in variational autoencoders (VAEs) play a critical role in the models' generative performance. However, powerful VAE network structures are hand-crafted and fixed prior to training, resulting in a one-size-fits-all approach that requires heavy computation to tune for given data. Moreover, existing VAE regularization methods largely overlook the importance of network structures and fail to prevent overfitting in deep VAE models with cascades of hidden layers. To address these issues, we propose a Bayesian inference framework that automatically adapts VAE network structures to data and prevent overfitting as they grow deeper. We model the number of hidden layers with a beta process to infer the most plausible encoding/decoding network depths warranted by data and perform layer-wise dropout regularization with a conjugate Bernoulli process. We develop a scalable estimator that performs joint inference on both VAE network structures and latent variables. Our experiments show that the inference framework effectively prevents overfitting in both shallow and deep VAE models, yielding state-of-the-art performance. We demonstrate that our framework is compatible with different types of VAE backbone networks and can be applied to various VAE variants, further improving their performance.
Publisher Site: OpenReview
PDF (Requires Sign-In): PDF
Click for Bibtex
@inproceedings{regmi2023adavae, title = {AdaVAE: Bayesian Structural Adaptation for Variational Autoencoders}, author = {Regmi, Paribesh and Li, Rui}, booktitle = {Thirty-seventh Conference on Neural Information Processing Systems}, year = {2023} }
[C-2023-04] Young, Michael and Yang, Zixin and Simon, Richard and Linte, Cristian A. (2023). “Investigating Transformer Encoding Techniques to Improve Data-Driven Volume-to-Surface Liver Registration for Image-Guided Navigation.” Workshop on Data Engineering in Medical Imaging.
Abstract: Due to limited direct organ visualization, minimally invasive interventions rely extensively on medical imaging and image guidance to ensure accurate surgical instrument navigation and target tissue manipulation. In the context of laparoscopic liver interventions, intra-operative video imaging only provides a limited field-of-view of the liver surface, with no information of any internal liver lesions identified during diagnosis using pre-procedural imaging. Hence, to enhance intra-procedural visualization and navigation, the registration of pre-procedural, diagnostic images and anatomical models featuring target tissues to be accessed or manipulated during surgery entails a sufficient accurate registration of the pre-procedural data into the intra-operative setting. Prior work has demonstrated the feasibility of neural network-based solutions for nonrigid volume-to-surface liver registration. However, view occlusion, lack of meaningful feature landmarks, and liver deformation between the pre- and intra-operative settings all contribute to the difficulty of this registration task. In this work, we leverage some of the state-of-the-art deep learning frameworks to implement and test various network architecture modifications toward improving the accuracy and robustness of volume-to-surface liver registration. Specifically, we focus on the adaptation of a transformer-based segmentation network for the task of better predicting the optimal displacement field for nonrigid registration. Our results suggest that one particular transformer-based network architecture—UTNet—led to significant improvements over baseline performance, yielding a mean displacement error on the order of 4 mm across a variety of datasets.
Publisher Site: SpringerLink
PDF (Requires Sign-In): PDF
Click for Bibtex
@inproceedings{young2023investigating, title = {Investigating Transformer Encoding Techniques to Improve Data-Driven Volume-to-Surface Liver Registration for Image-Guided Navigation}, author = {Young, Michael and Yang, Zixin and Simon, Richard and Linte, Cristian A}, booktitle = {MICCAI Workshop on Data Engineering in Medical Imaging}, pages = {91--101}, year = {2023}, organization = {Springer} }
[C-2023-03] Lin, Huawei and Chung, Jun woo and Lao, Yingjie and Zhao, Weijie. (2023). “Machine Unlearning in Gradient Boosting Decision Trees.” Conference on Knowledge Desciovery and Data Mining.
Abstract: Various machine learning applications take users' data to train the models. Recently enforced legislation requires companies to remove users' data upon requests, i.e.,the right to be forgotten. In the context of machine learning, the trained model potentially memorizes the training data. Machine learning algorithms have to be able to unlearn the user data that are requested to delete to meet the requirement. Gradient Boosting Decision Trees (GBDT) is a widely deployed model in many machine learning applications. However, few studies investigate the unlearning on GBDT. This paper proposes a novel unlearning framework for GBDT. To the best of our knowledge, this is the first work that considers machine unlearning on GBDT. It is not straightforward to transfer the unlearning methods of DNN to GBDT settings. We formalized the machine unlearning problem and its relaxed version. We propose an unlearning framework that efficiently and effectively unlearns a given collection of data without retraining the model from scratch. We introduce a collection of techniques, including random split point selection and random partitioning layers training, to the training process of the original tree models to ensure that the trained model requires few subtree retrainings during the unlearning. We investigate the intermediate data and statistics to store as an auxiliary data structure during the training so that we can immediately determine if a subtree is required to be retrained without touching the original training dataset. Furthermore, a lazy update technique is proposed as a trade-off between unlearning time and model functionality. We experimentally evaluate our proposed methods on public datasets. The empirical results confirm the effectiveness of our framework.
Publisher Site: ACM Digital Library
PDF (Requires Sign-In): PDF
Click for Bibtex
@inproceedings{lin2023machine, title = {Machine Unlearning in Gradient Boosting Decision Trees}, author = {Lin, Huawei and Chung, Jun Woo and Lao, Yingjie and Zhao, Weijie}, booktitle = {Proceedings of the 29th ACM SIGKDD Conference on Knowledge Discovery and Data Mining}, pages = {1374--1383}, year = {2023} }
[C-2023-02] Kini, Amit Dilip and Yadav, Swaraj Sambhaji and Thakur, Aditya Shankar and Awari, Akshar Bajrang and Lyu, Zimeng and Desell, Travis. (2023). “Co-Evolving Recurrent Neural Networks and their Hyperparameters with Simplex Hyperparameter Optimization.” Companion Conference on Genetic and Evolutionary Computation.
Abstract: Designing machine learning models involves determining not only the network architecture, but also non-architectural elements such as training hyperparameters. Further confounding this problem, different architectures and datasets will perform more optimally with different hyperparameters. This problem is exacerbated for neuroevolution (NE) and neural architecture search (NAS) algorithms, which can generate and train architectures with a wide variety of architectures in order to find optimal architectures. In such algorithms, if hyperparameters are fixed, then suboptimal architectures can be found as they will be biased towards the fixed parameters. This paper evaluates the use of the simplex hyperparameter optimization (SHO) method, which allows co-evolution of hyperparameters over the course of a NE algorithm, allowing the NE algorithm to simultaneously optimize both network architectures and hyperparameters. SHO has been previously shown to be able to optimize hyperparameters for convolutional neural networks using traditional stochastic gradient descent with Nesterov momentum, and this work extends on this to evaluate SHO for evolving recurrent neural networks with additional modern weight optimizers such as RMSProp and Adam. Results show that incorporating SHO into the neuroevolution process not only enables finding better performing architectures but also faster convergence to optimal architectures across all datasets and optimization methods tested.
Publisher Site: ACM Digital Library
PDF (Requires Sign-In): PDF
Click for Bibtex
@inproceedings{kini2023co, title = {Co-evolving Recurrent Neural Networks and their Hyperparameters with Simplex Hyperparameter Optimization}, author = {Kini, Amit Dilip and Yadav, Swaraj Sambhaji and Thakur, Aditya Shankar and Awari, Akshar Bajrang and Lyu, Zimeng and Desell, Travis}, booktitle = {Proceedings of the Companion Conference on Genetic and Evolutionary Computation}, pages = {1639--1647}, year = {2023} }
[C-2023-01] Mathew, Roshan and Dannels, Wendy A. and Parker, Aaron J. (2023). “An Augmented Reality Based Approach for Optimization of Language Access Services in Healthcare for Deaf Patients.” International Conference on Human-Computer Interaction.
Abstract: Deaf adults often rely on sign language interpreters and real-time captioners for language access during healthcare consultations. While deaf adults generally prefer that these access services be offered in person, previous studies have described that many healthcare providers frequently resort to employing video remote interpreting (VRI) or remote captioning services because of the lack of locally available qualified interpreters or captioners and because of a shorter turnaround time for availing these services. VRI equipment typically consists of a tablet mounted on a utility cart, whereas captions are usually displayed on handheld tablets. These approaches present visibility and cognitive challenges for deaf adults who then need to divide their attention between the interpreting or captioning display and the healthcare provider, thereby affecting their ability to thoroughly access health information shared during consultations. This study proposes augmented reality (AR) smart glasses as an alternative to traditional VRI and remote captioning services for optimizing communication access in healthcare settings. A descriptive study about the perspectives of deaf adults (N = 62), interpreters (N = 25), and captioners (N = 22) on using a smart glasses application for language access in healthcare and biomedical settings are discussed. Results showed that deaf adults who primarily use sign language interpreting and interpreters prefer onsite language access services but identified the benefits of implementing a smart glasses application whenever onsite access services are unavailable or not feasible. Additionally, deaf adults who rely primarily on captioning and captioners prefer the smart glasses application over traditional captioning methods.
Publisher Site: SpringerLink
PDF (Requires Sign-In): PDF
Click for Bibtex
@inproceedings{mathew2023augmented, title = {An Augmented Reality Based Approach for Optimization of Language Access Services in Healthcare for Deaf Patients}, author = {Mathew, Roshan and Dannels, Wendy A. and Parker, Aaron J.}, booktitle = {International Conference on Human-Computer Interaction}, pages = {29-52}, year = {2023}, organization = {Springer} }
[C-2022-01] Hemaspaandra, Edith and Narváez, David E. (2022). “Formal Methods for NFA Equivalence: QBFs, Witness Extraction, and Encoding Verification.” International Conference on Intelligent Computer Mathematics.
Abstract: Nondeterministic finite automata (NFAs) are an important model of computation. The equivalence problem for NFAs is known to be PSPACE-complete, and several specialized algorithms have been developed to solve this problem. In this paper, we approach the equivalence problem for NFAs by means of another PSPACE-complete problem: quantified satisfiability (QSAT). QSAT asks whether a quantified Boolean formula (QBF) is true. We encode the NFA equivalence problem as a QBF which is true if and only if the input NFAs are not equivalent. In addition to determining the equivalence of NFAs, we are also able to decode strings that witness the inequivalence of two NFAs by looking into the solving certificate. This is a novel application of certified QSAT solving. Lastly, we formally verify key aspects of the encoding in the Isabelle/HOL theorem prover.
Publisher Site: SpringerLink
PDF (Requires Sign-In): PDF
Click for Bibtex
@inproceedings{hemaspaandra2022formal, title = {Formal Methods for NFA Equivalence: QBFs, Witness Extraction, and Encoding Verification}, author = {Hemaspaandra, Edith and Narv{\'a}ez, David E}, booktitle = {International Conference on Intelligent Computer Mathematics}, pages = {241--255}, year = {2022}, organization = {Springer} }
[C-2021-03] Deshpande, Niranjana and Sharma, Naveen and Yu, Qi and Krutz, Daniel E. (2021). “R-CASS: Using Algorithm Selection for Self-Adaptive Service Oriented Systems.” IEEE International Conference on Web Services.
Abstract: In service composition, complex applications are built by combining web services to fulfill user Quality of Service (QoS) and business requirements. To meet these requirements, applications are composed by evaluating all possible web service combinations using search algorithms. These algorithms need to be accurate and inexpensive to evaluate a large number of possible service combinations and services' fluctuating QoS attributes while meeting the constraints of limited computational resources. Recent research has shown that different search algorithms can outperform others on specific instances of a problem domain, in terms of solution quality and computational resource usage. Problematically, current service composition approaches ignore this property, leading to inefficient compositions. To address these limitations, we propose a composition algorithm selection framework which selects an algorithm per composition task at runtime, R-CASS. Our evaluations demonstrate that R-CASS leads to more efficient compositions, reducing composition time by 55.1% and memory by 37.5%.
Publisher Site: IEEE Xplore
PDF (Requires Sign-In): PDF
Click for Bibtex
@inproceedings{deshpande2021r, title = {R-CASS: Using algorithm selection for self-adaptive service oriented systems}, author = {Deshpande, Niranjana and Sharma, Naveen and Yu, Qi and Krutz, Daniel E}, booktitle = {2021 IEEE International Conference on Web Services (ICWS)}, pages = {61--72}, year = {2021}, organization = {IEEE} }
[C-2021-02] Taufique, Abu Md Niamul and Savakis, Andreas and Braun, Michael and Kubacki, Daniel and Dell, Ethan and Qian, Lei and O’Rourke, Sean. (2021). “SIAM-REID: Confuser Aware Siamese Tracker with Re-identification Feature.” Applications of Machine Learning.
Abstract: Siamese deep-network trackers have received significant attention in recent years due to their real-time speed and state-of-the-art performance. However, Siamese trackers suffer from similar looking confusers,that are prevalent in aerial imagery and create challenging conditions due to prolonged occlusions where the tracker object re-appears under different pose and illumination. Our work proposes SiamReID, a novel re-identification framework for Siamese trackers, that incorporates confuser rejection during prolonged occlusions and is well-suited for aerial tracking. The re-identification feature is trained using both triplet loss and a class balanced loss. Our approach achieves state-of-the-art performance in the UAVDT single object tracking benchmark.
Publisher Site: SPIE
PDF (Requires Sign-In): PDF
Click for Bibtex
@inproceedings{taufique2021siam, title = {SIAM-REID: confuser aware Siamese tracker with re-identification feature}, author = {Taufique, Abu Md Niamul and Savakis, Andreas and Braun, Michael and Kubacki, Daniel and Dell, Ethan and Qian, Lei and O'Rourke, Sean}, booktitle = {Applications of Machine Learning 2021}, volume = {11843}, pages = {292--298}, year = {2021}, organization = {SPIE} }
[C-2021-01] ElSaid, AbdElRahman and Karns, Joshua and Lyu, Zimeng and Ororbia, Alexander G and Desell, Travis. (2021). “Continuous Ant-Based Neural Topology Search.” International Conference on the Applications of Evolutionary Computation.
Abstract: This work introduces a novel, nature-inspired neural architecture search (NAS) algorithm based on ant colony optimization, Continuous Ant-based Neural Topology Search (CANTS), which utilizes synthetic ants that move over a continuous search space based on the density and distribution of pheromones, is strongly inspired by how ants move in the real world. The paths taken by the ant agents through the search space are utilized to construct artificial neural networks (ANNs). This continuous search space allows CANTS to automate the design of ANNs of any size, removing a key limitation inherent to many current NAS algorithms that must operate within structures with a size predetermined by the user. CANTS employs a distributed asynchronous strategy which allows it to scale to large-scale high performance computing resources, works with a variety of recurrent memory cell structures, and makes use of a communal weight sharing strategy to reduce training time. The proposed procedure is evaluated on three real-world, time series prediction problems in the field of power systems and compared to two state-of-the-art algorithms. Results show that CANTS is able to provide improved or competitive results on all of these problems, while also being easier to use, requiring half the number of user-specified hyper-parameters.
Publisher Site: SpringerLink
PDF (Requires Sign-In): PDF
Click for Bibtex
@inproceedings{elsaid2021continuous, title = {Continuous Ant-Based Neural Topology Search}, author = {ElSaid, AbdElRahman and Karns, Joshua and Lyu, Zimeng and Ororbia, Alexander G and Desell, Travis}, booktitle = {International Conference on the Applications of Evolutionary Computation (Part of EvoStar)}, pages = {291--306}, year = {2021}, organization = {Springer} }
[C-2020-01] Bansal, Iti and Tiwari, Sudhanshu and Rivero, Carlos R. (2020). “The Impact of Negative Triple Generation Strategies and Anomalies on Knowledge Graph Completion.” ACM International Conference on Information & Knowledge Management.
Abstract: Even though knowledge graphs have proven very useful for several tasks, they are marked by incompleteness. Completion algorithms aim to extend knowledge graphs by predicting missing (subject, predicate, object) triples, usually by training a model to discern between correct (positive) and incorrect (negative) triples. However, under the open-world assumption in which a missing triple is not negative but unknown, negative triple generation is challenging. Although negative triples are known to drive the accuracy of completion models, its impact has not been thoroughly examined yet. To evaluate accuracy, test triples are considered positive and negative triples are derived from them. The evaluation protocol is thus impacted by the generation of negative triples, which remains to be analyzed. Another issue is that the knowledge graphs available for evaluation contain anomalies like severe redundancy, and it is unclear how anomalies affect the accuracy of completion models. In this paper, we analyze the impact of negative triple generation during both training and testing on translation-based completion models. We examine four negative triple generation strategies, which are also used to evaluate the models when anomalies in the test split are included and discarded. In addition to previously-studied anomalies like near-same predicates, we include another anomaly: knowledge present in the test that is missing from the training split. Our main conclusion is that the most common strategy for negative triple generation (local-closed world assumption) can be mimicked by a combination of a naive and a immediate neighborhood strategies. This result suggests that completion models can be learned independently for certain subgraphs, which would render completion models useful in the context of knowledge graph evolution. Although anomalies are considered harmful since they artificially increase the accuracy of completion models, our results show otherwise for certain knowledge graphs, which calls for further research efforts.
Publisher Site: ACM Digital Library
PDF (Requires Sign-In): PDF
Click for Bibtex
@inproceedings{bansal2020impact, title = {The impact of negative triple generation strategies and anomalies on knowledge graph completion}, author = {Bansal, Iti and Tiwari, Sudhanshu and Rivero, Carlos R}, booktitle = {Proceedings of the 29th ACM International Conference on Information \& Knowledge Management}, pages = {45--54}, year = {2020} }
[C-2019-04] ElSaid, AbdElRahman and Benson, Steven and Patwardhan, Shuchita and Stadem, David and Desell, Travis. (2019). “Evolving Recurrent Neural Networks for Time Series Data Prediction of Coal Plant Parameters.” Applications of Evolutionary Computation.
Abstract: This paper presents the Evolutionary eXploration of Augmenting LSTM Topologies (EXALT) algorithm and its use in evolving recurrent neural networks (RNNs) for time series data prediction. It introduces a new open data set from a coal-fired power plant, consisting of 10 days of per minute sensor recordings from 12 different burners at the plant. This large scale real world data set involves complex dependencies between sensor parameters and makes for challenging data to predict. EXALT provides interesting new techniques for evolving neural networks, including epigenetic weight initialization, where child neural networks re-use parental weights as a starting point to backpropagation, as well as node-level mutation operations which can improve evolutionary progress. EXALT has been designed with parallel computation in mind to further improve performance. Preliminary results were gathered predicting the Main Flame Intensity data parameter, with EXALT strongly outperforming five traditional neural network architectures on the best, average and worst cases across 10 repeated training runs per test case; and was only slightly behind the best trained Elman recurrent neural networks while being significantly more reliable (i.e., much better average and worst case results). Further, EXALT achieved these results 2 to 10 times faster than the traditional methods, in part due to its scalability, showing strong potential to beat traditional architectures given additional runtime.
Publisher Site: SpringerLink
PDF (Requires Sign-In): PDF
Click for Bibtex
@inproceedings{elsaid2019evolving, title = {Evolving recurrent neural networks for time series data prediction of coal plant parameters}, author = {ElSaid, AbdElRahman and Benson, Steven and Patwardhan, Shuchita and Stadem, David and Desell, Travis}, booktitle = {Applications of Evolutionary Computation: 22nd International Conference, EvoApplications 2019, Held as Part of EvoStar 2019, Leipzig, Germany, April 24--26, 2019, Proceedings 22}, pages = {488--503}, year = {2019}, organization = {Springer} }
[C-2019-03] Meyers, Benjamin S. and Munaiah, Nuthan and Meneely, Andrew and Prud’hommeaux, Emily. (2019). “Pragmatic Characteristics of Security Conversations: An Exploratory Linguistic Analysis.” International Workshop on Cooperative and Human Aspects of Software Engineering.
Abstract: Experts suggest that engineering secure software requires a defensive mindset to be ingrained in developer culture, which could be reflected in conversation. But what does a conversation about software security in a real project look like? Linguists analyze a wide array of characteristics: lexical, syntactic, semantic, and pragmatic. Pragmatics focus on identifying the style and tone of the author's language. If security requires a different mindset, then perhaps this would be reflected in the conversations' pragmatics. Our goal is to characterize the pragmatic features of conversations about security so that developers can be more informed about communication strategies regarding security concerns. We collected and annotated a corpus of conversations from 415,041 bug reports in the Chromium project. We examined five linguistic metrics related to pragmatics: formality, informativeness, implicature, politeness, and uncertainty. Our initial exploration into these data show that pragmatics plays a role, however small, in security conversations. These results indicate that the area of linguistic analysis shows promise in automatically identifying effective security communication strategies.
Publisher Site: IEEE Xplore
PDF (Requires Sign-In): PDF
Click for Bibtex
@inproceedings{meyers2019pragmatic, title = {Pragmatic characteristics of security conversations: an exploratory linguistic analysis}, author = {Meyers, Benjamin S and Munaiah, Nuthan and Meneely, Andrew and Prud'hommeaux, Emily}, booktitle = {2019 IEEE/ACM 12th International Workshop on Cooperative and Human Aspects of Software Engineering (CHASE)}, pages = {79--82}, year = {2019}, organization = {IEEE} }
[C-2019-02] Marin, Victor J. and Rivero, Carlos R. (2019). “Clustering Recurrent and Semantically Cohesive Program Statements in Introductory Programming Assignments.” International Conference on Information and Knowledge Management.
Abstract: Students taking introductory programming courses are typically required to complete assignments and expect timely feedback to advance their learning. With the current popularity of these courses in both traditional and online versions, graders are seeing themselves overwhelmed by the sheer amount of student programs they have to handle, and the quality of the educational experience provided is often compromised for promptness. Thus, there is a need for automated approaches to effectively increase grading productivity. Existing approaches in this context fail to support flexible grading schemes and customization based on the assignment at hand. This paper presents a data-driven approach for clustering recurrent program statements performing similar but not exact semantics across student programs, which we refer to as core statements. We rely on structural graph clustering over the program dependence graph representations of student programs. Such clustering is performed over the graph resulting from the pairwise approximate graph alignments of programs. Core statements help graders understand solution variations at a glance and, since they group program statements present in individual student programs, can be used to propagate feedback, thus increasing grading productivity. Our experimental results show that, on average, we discover core statements covering more than 50% of individual student programs, and that program statements grouped by core statements are semantically cohesive, which ensures effective grading.
Publisher Site: ACM Digital Library
PDF (Requires Sign-In): PDF
Click for Bibtex
@inproceedings{marin2019clustering, title = {Clustering recurrent and semantically cohesive program statements in introductory programming assignments}, author = {Marin, Victor J and Rivero, Carlos R}, booktitle = {Proceedings of the 28th ACM International Conference on Information and Knowledge Management}, pages = {911--920}, year = {2019} }
[C-2019-01] Chaudhary, Aayush K. and Kothari, Rakshit and Acharya, Manoj and Dangi, Shusil and Nair, Nitinraj and Bailey, Reynold and Kanan, Christopher and Diaz, Gabriel and Pelz, Jeff B. (2019). “RITnet: Real-time Semantic Segmentation of the Eye for Gaze Tracking.” International Conference on Computer Vision Workshop.
Abstract: Accurate eye segmentation can improve eye-gaze estimation and support interactive computing based on visual attention; however, existing eye segmentation methods suffer from issues such as person-dependent accuracy, lack of robustness, and an inability to be run in real-time. Here, we present the RITnet model, which is a deep neural net- work that combines U-Net and DenseNet. RITnet is under 1 MB and achieves 95.3% accuracy on the 2019 OpenEDS Semantic Segmentation challenge. Using a GeForce GTX 1080 Ti, RITnet tracks at > 300Hz, enabling real-time gaze tracking applications. Pre-trained models and source code are available.
Publisher Site: IEEE Xplore
PDF (Requires Sign-In): PDF
Click for Bibtex
@inproceedings{chaudhary2019ritnet, title = {Ritnet: Real-time semantic segmentation of the eye for gaze tracking}, author = {Chaudhary, Aayush K and Kothari, Rakshit and Acharya, Manoj and Dangi, Shusil and Nair, Nitinraj and Bailey, Reynold and Kanan, Christopher and Diaz, Gabriel and Pelz, Jeff B}, booktitle = {2019 IEEE/CVF International Conference on Computer Vision Workshop (ICCVW)}, pages = {3698--3702}, year = {2019}, organization = {IEEE} }
[C-2018-04] Narde, Rounak Singh and Mansoor, Naseef and Ganguly, Amlan and Venkataraman, Jayanti. (2018). “On-Chip Antennas for Inter-Chip Wireless Interconnections: Challenges and Opportunities.” Institution of Engineering and Technology.
Abstract: Wireless interconnects have emerged as a solution to the problem of metallic interconnections for both intra and inter-chip communications. Multi GHz bandwidths of millimeter-wave (mm-wave) antennas coupled with appropriate system-level interconnection fabric design enables low power and high-speed communication between multiple many-core chips. In this paper, we discuss the design and transmission characteristics of an mm-wave antenna for multi-chip wireless interconnection systems. We investigate the challenges and opportunities for antenna design in this domain. We present a specific antenna design and its performance in terms of its transmission characteristics for inter-chip communication in a server node. Lastly, we explore the impact of antenna design on system-level design choices pertaining to Medium Access Control (MAC), topology and modulation techniques suitable for multi-chip systems.
Publisher Site: IET Digital Library
PDF (Requires Sign-In): Unavailable
Click for Bibtex
@inproceedings{narde2018chip, title = {On-chip antennas for inter-chip wireless interconnections: Challenges and opportunities}, author = {Narde, Rounak Singh and Mansoor, Naseef and Ganguly, Amlan and Venkataraman, Jayanti}, booktitle = {Institution of Engineering and Technology Proceedings}, year = {2018}, publisher = {IET} }
[C-2018-03] Cahill, Nathan D. and Hayes, Tyler L. and Meinhold, Renee T. and Hamilton, John F. (2018). “Compassionately Conservative Balanced Cuts for Image Segmentation.” IEEE Conference on Computer Vision and Pattern Recognition.
Abstract: The Normalized Cut (NCut) objective function, widely used in data clustering and image segmentation, quantifies the cost of graph partitioning in a way that biases clusters or segments that are balanced towards having lower values than unbalanced partitionings. However, this bias is so strong that it avoids any singleton partitions, even when vertices are very weakly connected to the rest of the graph. Motivated by the Bühler-Hein family of balanced cut costs, we propose the family of Compassionately Conservative Balanced (CCB) Cut costs, which are indexed by a parameter that can be used to strike a compromise between the desire to avoid too many singleton partitions and the notion that all partitions should be balanced. We show that CCB-Cut minimization can be relaxed into an orthogonally constrained lt-minimization problem that coincides with the problem of computing Piecewise Flat Embeddings (PFE) for one particular index value, and we present an algorithm for solving the relaxed problem by iteratively minimizing a sequence of reweighted Rayleigh quotients (IRRQ). Using images from the BSDS500 database, we show that image segmentation based on CCB-Cut minimization provides better accuracy with respect to ground truth and greater variability in region size than NCut-based image segmentation.
Publisher Site: IEEE Xplore
PDF (Requires Sign-In): PDF
Click for Bibtex
@inproceedings{cahill2018compassionately, title = {Compassionately conservative balanced cuts for image segmentation}, author = {Cahill, Nathan D and Hayes, Tyler L and Meinhold, Renee T and Hamilton, John F}, booktitle = {Proceedings of the IEEE Conference on Computer Vision and Pattern Recognition}, pages = {1683--1691}, year = {2018} }
[C-2018-02] Immel, Poppy G. and Cahill, Nathan D. (2018). “Semi-Supervised Normalized Embeddings for Land-Use Classification from Multiple View Data.” Algorithms and Technologies for Multispectral, Hyperspectral, and Ultraspectral Imagery.
Abstract: Land-use classification from multiple data sources is an important problem in remote sensing. Data fusion algorithms like Semi-Supervised Manifold Alignment (SSMA) and Manifold Alignment with Schroedinger Eigenmaps (SEMA) use spectral and/or spatial features from multispectral, multimodal imagery to project each data source into a common latent space in which classification can be performed. However, in order for these algorithms to be well-posed, they require an expert user to either directly identify pairwise dissimilarities in the data or to identify class labels for a subset of points from which pairwise dissimilarities can be derived. In this paper, we propose a related data fusion technique, which we refer to as Semi-Supervised Normalized Embeddings (SSNE). SSNE is defined by modifying the SSMA/SEMA objective functions to incorporate an extra normalization term that enables a latent space to be well-defined even when no pairwise-dissimilarities are provided. Using publicly available data from the 2017 IEEE GRSS Data Fusion Contest, we show that SSNE enables similar land-use classification performance to SSMA/SEMA in scenarios where pairwise dissimilarities are available, but that unlike SSMA/SEMA, it also enables land-use classification in other scenarios.
Publisher Site: SPIE
PDF (Requires Sign-In): Unavailable
Click for Bibtex
@inproceedings{immel2018semi, title = {Semi-supervised normalized embeddings for land-use classification from multiple view data}, author = {Immel, Poppy G and Cahill, Nathan D}, booktitle = {Algorithms and Technologies for Multispectral, Hyperspectral, and Ultraspectral Imagery XXIV}, volume = {10644}, pages = {62--74}, year = {2018}, organization = {SPIE} }
[C-2018-01] Meyers, Benjamin S. and Munaiah, Nuthan and Prud’hommeaux, Emily and Meneely, Andrew and Wolff, Josephine and Alm, Cecilia Ovesdotter and Murukannaiah, Pradeep. (2018). “A Dataset for Identifying Actionable Feedback in Collaborative Software Development.” Association for Computational Linguistics.
Abstract: Software developers and testers have long struggled with how to elicit proactive responses from their coworkers when reviewing code for security vulnerabilities and errors. For a code review to be successful, it must not only identify potential problems but also elicit an active response from the colleague responsible for modifying the code. To understand the factors that contribute to this outcome, we analyze a novel dataset of more than one million code reviews for the Google Chromium project, from which we extract linguistic features of feedback that elicited responsive actions from coworkers. Using a manually-labeled subset of reviewer comments, we trained a highly accurate classifier to identify acted-upon comments (AUC = 0.85). Our results demonstrate the utility of our dataset, the feasibility of using NLP for this new task, and the potential of NLP to improve our understanding of how communications between colleagues can be authored to elicit positive, proactive responses.
Publisher Site: ACL Anthology
PDF (Requires Sign-In): PDF
Click for Bibtex
@inproceedings{meyers2018dataset, title = {A dataset for identifying actionable feedback in collaborative software development}, author = {Meyers, Benjamin S and Munaiah, Nuthan and Prud'hommeaux, Emily and Meneely, Andrew and Wolff, Josephine and Alm, Cecilia Ovesdotter and Murukannaiah, Pradeep}, booktitle = {Proceedings of the 56th Annual Meeting of the Association for Computational Linguistics (Volume 2: Short Papers)}, pages = {126--131}, year = {2018} }
[C-2017-03] Narde, Rounak Singh and Venkataraman, Jayanti and Ganguly, Amlan. (2017). “Feasibility Study of Transmission Between Wireless Interconnects in Multichip Multicore Systems.” IEEE International Symposium on Antennas and Propagation.
Abstract: The paper presents a feasibility study of transmission between wireless interconnects in Multichip Multicore (MCMC) systems. The MCMC model consists of four IC's, each with four cores that are placed at the corners. For this work, 4 electrically small monopole antennas are considered as wireless interconnects, placed on 3 IC's. Simulation is performed using ANSYS Electronics suite. As expected, transmission decreases with the distance between the antennas. Further, distortions of the radiation pattern due to the lossy silicon layer and the ground plane, significantly affect the transmission between the antennas. For experimental validation, zigzag antennas have been fabricated on silicon wafers. Measurements and analysis for a wireless channel modeling is under progress.
Publisher Site: IEEE Xplore
PDF (Requires Sign-In): PDF
Click for Bibtex
@inproceedings{narde2017feasibility, title = {Feasibility study of transmission between wireless interconnects in multichip multicore systems}, author = {Narde, Rounak Singh and Venkataraman, Jayanti and Ganguly, Amlan}, booktitle = {2017 IEEE International Symposium on Antennas and Propagation \& USNC/URSI National Radio Science Meeting}, pages = {1821--1822}, year = {2017}, organization = {IEEE} }
[C-2017-02] Hayes, Tyler L. and Meinhold, Renee T. and Hamilton Jr, John F. and Cahill, Nathan D. (2017). “Piecewise Flat embeddings for Hyperspectral Image Analysis.” Algorithms and Technologies for Multispectral, Hyperspectral, and Ultraspectral Imagery.
Abstract: Graph-based dimensionality reduction techniques such as Laplacian Eigenmaps (LE), Local Linear Embedding (LLE), Isometric Feature Mapping (ISOMAP), and Kernel Principal Components Analysis (KPCA) have been used in a variety of hyperspectral image analysis applications for generating smooth data embeddings. Recently, Piecewise Flat Embeddings (PFE) were introduced in the computer vision community as a technique for generating piecewise constant embeddings that make data clustering / image segmentation a straightforward process. In this paper, we show how PFE arises by modifying LE, yielding a constrained l1-minimization problem that can be solved iteratively. Using publicly available data, we carry out experiments to illustrate the implications of applying PFE to pixel-based hyperspectral image clustering and classification.
Publisher Site: SPIE
PDF (Requires Sign-In): PDF
Click for Bibtex
@inproceedings{hayes2017piecewise, title = {Piecewise flat embeddings for hyperspectral image analysis}, author = {Hayes, Tyler L and Meinhold, Renee T and Hamilton Jr, John F and Cahill, Nathan D}, booktitle = {Algorithms and Technologies for Multispectral, Hyperspectral, and Ultraspectral Imagery XXIII}, volume = {10198}, pages = {252--262}, year = {2017}, organization = {SPIE} }
[C-2017-01] Munaiah, Nuthan and Meyers, Benjamin S. and Alm, Cecilia O. and Meneely, Andrew and Murukannaiah, Pradeep K. and Prud’hommeaux, Emily and Wolff, Josephine and Yu, Yang. (2017). “Natural Language Insights from Code Reviews that Missed a Vulnerability.” International Symposium on Engineering Secure Software and Systems.
Abstract: Engineering secure software is challenging. Software development organizations leverage a host of processes and tools to enable developers to prevent vulnerabilities in software. Code reviewing is one such approach which has been instrumental in improving the overall quality of a software system. In a typical code review, developers critique a proposed change to uncover potential vulnerabilities. Despite best efforts by developers, some vulnerabilities inevitably slip through the reviews. In this study, we characterized linguistic features-inquisitiveness, sentiment and syntactic complexity-of conversations between developers in a code review, to identify factors that could explain developers missing a vulnerability. We used natural language processing to collect these linguistic features from 3,994,976 messages in 788,437 code reviews from the Chromium project. We collected 1,462 Chromium vulnerabilities to empirically analyze the linguistic features. We found that code reviews with lower inquisitiveness, higher sentiment, and lower complexity were more likely to miss a vulnerability. We used a Naive Bayes classifier to assess if the words (or lemmas) in the code reviews could differentiate reviews that are likely to miss vulnerabilities. The classifier used a subset of all lemmas (over 2 million) as features and their corresponding TF-IDF scores as values. The average precision, recall, and F-measure of the classifier were 14%, 73%, and 23%, respectively. We believe that our linguistic characterization will help developers identify problematic code reviews before they result in a vulnerability being missed.
Publisher Site: SpringerLink
PDF (Requires Sign-In): PDF
Click for Bibtex
@inproceedings{munaiah2017natural, title = {Natural language insights from code reviews that missed a vulnerability}, author = {Munaiah, Nuthan and Meyers, Benjamin S and Alm, Cecilia O and Meneely, Andrew and Murukannaiah, Pradeep K and Prud'hommeaux, Emily and Wolff, Josephine and Yu, Yang}, booktitle = {International Symposium on Engineering Secure Software and Systems}, pages = {70--86}, year = {2017}, organization = {Springer} }
[C-2016-01] Munaiah, Nuthan and Meneely, Andrew. (2016). “Beyond the Attack Surface: Assessing Security Risk with Random Walks on Call Graphs.” ACM Workshop on Software Protection.
Abstract: When reasoning about software security, researchers and practitioners use the phrase ``attack surface'' as a metaphor for risk. Enumerate and minimize the ways attackers can break in then risk is reduced and the system is better protected, the metaphor says. But software systems are much more complicated than their surfaces. We propose function- and file-level attack surface metrics---proximity and risky walk---that enable fine-grained risk assessment. Our risky walk metric is highly configurable: we use PageRank on a probability-weighted call graph to simulate attacker behavior of finding or exploiting a vulnerability. We provide evidence-based guidance for deploying these metrics, including an extensive parameter tuning study. We conducted an empirical study on two large open source projects, FFmpeg and Wireshark, to investigate the potential correlation between our metrics and historical post-release vulnerabilities. We found our metrics to be statistically significantly associated with vulnerable functions/files with a small-to-large Cohen's d effect size. Our prediction model achieved an increase of 36% (in FFmpeg) and 27% (in Wireshark) in the average value of F{2}-measure over a base model built with SLOC and coupling metrics. Our prediction model outperformed comparable models from prior literature with notable improvements: 58% reduction in false negative rate, 81% reduction in false positive rate, and 548% increase in F{2}-measure. These metrics advance vulnerability prevention by [(a)] being flexible in terms of granularity, performing better than vulnerability prediction literature, and being tunable so that practitioners can tailor the metrics to their products and better assess security risk.
Publisher Site: ACM Digital Library
PDF (Requires Sign-In): PDF
Click for Bibtex
@inproceedings{munaiah2016beyond, title = {Beyond the attack surface: Assessing security risk with random walks on call graphs}, author = {Munaiah, Nuthan and Meneely, Andrew}, booktitle = {Proceedings of the 2016 ACM Workshop on Software PROtection}, pages = {3--14}, year = {2016} }
Ph.D. Dissertations
[D-2023-30] S. M. Kamrul Hasan. (2023). “From Fully-Supervised Single-Task to Semi-Supervised Multi-Task Deep Learning Architectures for Segmentation in Medical Imaging Applications.” Rochester Institute of Technology.
Abstract: Medical imaging is routinely performed in clinics worldwide for the diagnosis and treatment of numerous medical conditions in children and adults. With the advent of these medical imaging modalities, radiologists can visualize both the structure of the body as well as the tissues within the body. However, analyzing these high-dimensional (2D/3D/4D) images demands a significant amount of time and effort from radiologists. Hence, there is an ever-growing need for medical image computing tools to extract relevant information from the image data to help radiologists perform efficiently. Image analysis based on machine learning has pivotal potential to improve the entire medical imaging pipeline, providing support for clinical decision-making and computer-aided diagnosis. To be effective in addressing challenging image analysis tasks such as classification, detection, registration, and segmentation, specifically for medical imaging applications, deep learning approaches have shown significant improvement in performance. While deep learning has shown its potential in a variety of medical image analysis problems including segmentation, motion estimation, etc., generalizability is still an unsolved problem and many of these successes are achieved at the cost of a large pool of datasets. For most practical applications, getting access to a copious dataset can be very difficult, often impossible. Annotation is tedious and time-consuming. This cost is further amplified when annotation must be done by a clinical expert in medical imaging applications. Additionally, the applications of deep learning in the real-world clinical setting are still limited due to the lack of reliability caused by the limited prediction capabilities of some deep learning models. Moreover, while using a CNN in an automated image analysis pipeline, it's critical to understand which segmentation results are problematic and require further manual examination. To this extent, the estimation of uncertainty calibration in a semi-supervised setting for medical image segmentation is still rarely reported. This thesis focuses on developing and evaluating optimized machine learning models for a variety of medical imaging applications, ranging from fully-supervised, single-task learning to semi-supervised, multi-task learning that makes efficient use of annotated training data. The contributions of this dissertation are as follows: (1) developing a fully-supervised, single-task transfer learning for the surgical instrument segmentation from laparoscopic images; and (2) utilizing supervised, single-task, transfer learning for segmenting and digitally removing the surgical instruments from endoscopic/laparoscopic videos to allow the visualization of the anatomy being obscured by the tool. The tool removal algorithms use a tool segmentation mask and either instrument-free reference frames or previous instrument-containing frames to fill in (inpaint) the instrument segmentation mask; (3) developing fully-supervised, single-task learning via efficient weight pruning and learned group convolution for accurate left ventricle (LV), right ventricle (RV) blood pool and myocardium localization and segmentation from 4D cine cardiac MR images; (4) demonstrating the use of our fully-supervised memory-efficient model to generate dynamic patient-specific right ventricle (RV) models from cine cardiac MRI dataset via an unsupervised learning-based deformable registration field; and (5) integrating a Monte Carlo dropout into our fully-supervised memory-efficient model with inherent uncertainty estimation, with the overall goal to estimate the uncertainty associated with the obtained segmentation and error, as a means to flag regions that feature less than optimal segmentation results; (6) developing semi-supervised, single-task learning via self-training (through meta pseudo-labeling) in concert with a Teacher network that instructs the Student network by generating pseudo-labels given unlabeled input data; (7) proposing largely-unsupervised, multi-task learning to demonstrate the power of a simple combination of a disentanglement block, variational autoencoder (VAE), generative adversarial network (GAN), and a conditioning layer-based reconstructor for performing two of the foremost critical tasks in medical imaging — segmentation of cardiac structures and reconstruction of the cine cardiac MR images; (8) demonstrating the use of 3D semi-supervised, multi-task learning for jointly learning multiple tasks in a single backbone module – uncertainty estimation, geometric shape generation, and cardiac anatomical structure segmentation of the left atrial cavity from 3D Gadolinium-enhanced magnetic resonance (GE-MR) images. This dissertation summarizes the impact of the contributions of our work in terms of demonstrating the adaptation and use of deep learning architectures featuring different levels of supervision to build a variety of image segmentation tools and techniques that can be used across a wide spectrum of medical image computing applications centered on facilitating and promoting the wide-spread computer-integrated diagnosis and therapy data science.
Publisher Site: RIT Repository
PDF (Requires Sign-In): PDF
Click for Bibtex
@book{hasan2023from, title = {From Fully-Supervised Single-Task to Semi-Supervised Multi-Task Deep Learning Architectures for Segmentation in Medical Imaging Applications}, author = {Hasan, S. M. Kamrul}, year = {2023}, school = {Rochester Institute of Technology} }
[D-2023-29] Roshan Reddy Upendra. (2023). “A Deep Learning-based Pipeline to Generate Patient-specific Anatomical Models of the Heart Using Cardiac MRI.” Rochester Institute of Technology.
Abstract: Image-based patient-specific anatomical models of the heart have the potential to be used in a variety of clinical scenarios such as diagnosis and prognosis of various cardiovascular diseases (CVDs), including cardiac resynchronization therapy (CRT), ablation therapy, risk stratification, and minimally invasive cardiac interventions. Cardiac magnetic resonance imaging (MRI) provides images with high-resolution and superior soft tissue contrast, rendering it as the gold standard modality for imaging cardiac anatomy. To obtain meaningful information from such image-based personalized anatomical models of the heart, it is crucial to combine the geometric models of the cardiac chambers extracted from cine cardiac MRI and the scar anatomy from the late gadolinium enhanced (LGE) MRI. There are several challenges to be tackled to generate patient-specific anatomical models of the heart from the cardiac MRI data. Firstly, accurate and robust automated segmentation of the cardiac chambers from the cine cardiac MRI data is essential to estimate cardiac function indices. Secondly, it is important to estimate cardiac motion from 4D cine MRI data to assess the kinematic and contractile properties of the myocardium. Thirdly, accurate registration of the LGE MRI images with their corresponding cine MRI images is crucial to assess myocardial viability. In addition to the above-mentioned segmentation and registration tasks, it is also crucial to computationally super-resolve the anisotropic (high in-plane and low through-plane resolution) cardiac MRI images, while maintaining the structural integrity of the tissues. With the advent of deep learning, medical image segmentation and registration have immensely benefited. In this work, we present a deep learning-based framework to generate personalized cardiac anatomical models using cardiac MRI data. Firstly, we segment the cardiac chambers from an open-source cine cardiac MRI data using an adversarial deep learning framework. We evaluate the viability of the proposed adversarial framework by assessing its effect on the clinical cardiac parameters. Secondly, we propose a convolutional neural network (CNN) based 4D deformable registration algorithm for cardiac motion estimation from an open-source 4D cine cardiac MRI dataset. We extend this proposed CNN-based 4D deformable registration algorithm to develop dynamic patient-specific geometric models of the left ventricle (LV) myocardium and right ventricle (RV) endocardium. Thirdly, we present a deep learning framework for registration of cine and LGE MRI images, and assess the registration performance of the proposed method on an open source dataset. Finally, we present a 3D CNN-based framework with structure preserving gradient guidance to generate super-resolution cardiac MRI images, and assess this proposed super-resolution algorithm on an open-source LGE MRI dataset. Furthermore, we investigate the effect of the proposed super-resolution algorithm on downstream segmentation task.
Publisher Site: RIT Repository
PDF (Requires Sign-In): PDF
Click for Bibtex
@book{upendra2023a, title = {A Deep Learning-based Pipeline to Generate Patient-specific Anatomical Models of the Heart Using Cardiac MRI}, author = {Upendra, Roshan Reddy}, year = {2023}, school = {Rochester Institute of Technology} }
[D-2023-28] Omar Abdul-Latif. (2023). “End-To-End Network Slicing Using Hypergraph Theory.” Rochester Institute of Technology.
Abstract: Network slicing is the practice of implementing multiple virtualized and independent architectures on a single physical network infrastructure, in a way that allows for multiplexing and efficient use of resources. Network slicing is based on the concept of network virtualization and, when it reaches maturity, is expected to result in complete softwarization of 5G, Beyond-5G (B5G) and 6G networks. This means that future networks will only need minimal physical infrastructure upgrades (mostly in the frontend of the network). Network slicing is identified as one of the key enablers of next generation wireless mobile networks due to its ability to multiplex virtualized and independent architectures on the same physical network infrastructure. The virtual architectures instantiated through network slicing can be tailored to the technical requirements of specific verticals or applications. However, there is still the challenge of providing traffic-specific mechanism to generate and provision the virtual networks (i.e. network slices) that are tailor-made for specific applications. This challenge is currently an active research topic in the field of wireless communication networks. In this research work, three end-to-end network slicing provisioning frameworks are proposed and investigated. We started with an existing complex-network-based framework and devised an improvement scheme that utilized the more fitting Dijkstra's and A* algorithms to linearize the provisioning time needed to process the number of network slice requests (NSR). Next, a new hypergraph-based framework utilizing the generalization feature of hypergraphs is proposed to optimize the resource scheduling and bandwidth allocation procedures. The hypergraph-game-based framework employs two altruistic games, which are used for the resources and bandwidth selection operations. Lastly, spiking neural networks (SNN) are utilized to implement a novel hypergraph-SNN-based framework that reduces provisioning time by an order of magnitude, while optimizing resource scheduling and performance of the network. The performance of the frameworks was assessed in terms of resource utilization and acceptance ratios while maintaining near optimum provisioning time requirement. The simulation results of the proposed complex-network framework showed linearization and significant reduction in the processing time of the network slicing provisioning as a function of the number of nodes in both the physical infrastructure and the virtual network slices. The hypergraph-game-based frameworks produced better-quality results when compared to other methods presented in the literature. Lastly, the hypergraph-SNN-based framework produced superior results that addressed the main challenges of minimizing the execution time while maintaining high resource efficiency and acceptance ratio in the provisioning process of NSRs.
Publisher Site: RIT Repository
PDF (Requires Sign-In): PDF
Click for Bibtex
@book{abdul-latif2023end-to-end, title = {End-To-End Network Slicing Using Hypergraph Theory}, author = {Abdul-Latif, Omar}, year = {2023}, school = {Rochester Institute of Technology} }
[D-2023-27] Akhter Al Amin. (2023). “Investigating the Importance of Non-Textual Caption Properties from Deaf and Hard of Hearing Viewers’ Perspective.” Rochester Institute of Technology.
Abstract: Deaf and hard-of-hearing (DHH) viewers rely on captioning to perceive auditory information while watching live television programming. Captioning ensures that viewers can receive textual and non-textual information that is crucial to comprehend the content of the video. However, no research has investigated how non-textual properties of captioning, such as caption placement and the presentation of current speakers, influence DHH viewers' ability to comprehend video content and their judgement of a captioned video's quality. Thus, this work aims to understand the effect of non-textual properties of captioning on DHH viewers' live captioned video watching experiences; these findings can inform a better design of existing live captioning guidelines and caption quality evaluation methods to benefit DHH viewers who employ captioning while watching live TV programming. This dissertation offers the following three major contributions: 1. Understanding Perspective of DHH Users' while Watching Live Captioned TV Programming. While captioning is a long-standing medium of disseminating auditory information, live captioning is relatively newer phenomenon. It is still unknown how current practices related to captioning properties and appearance affect DHH viewers' ability to comprehend the content of TV programming. Furthermore, it is also unknown what challenges DHH viewers encounter with traditional captioning and what methods of presenting captions they prefer in a live context. Thus, the first part of this dissertation focuses on investigating the perspectives of DHH viewers regarding caption appearance in live TV programming. 2. The Creation and Evaluation of Occlusion Severity-Based Caption Evaluation Methods. Prior literature has identified challenges encountered by DHH viewers when captions overlap some of the visual information on the screen. However, no research has quantified how much information captions occlude and which information affects DHH viewers' subjective assessments of the quality of captioned videos. In light of this, in this part, we present research that aims to identify the information regions that are crucial to DHH viewers. Based on users' subjective priority on these information regions, we developed and evaluated metrics that can estimate the quality of captioning when captions occlude various information regions from the perspective of DHH viewers. 3. The Effect of Speaker Identifier Methods on DHH Viewers' Subjective Assessments of a Captioned Video's Quality. This part explores another facet of caption attributes, namely the ability to identify the current speaker on the screen. Prior literature has proposed several advanced speaker identification methods that somewhat improved viewers' ability to comprehend who is speaking. However, due to the challenges involved in live captioning, including captioners' time and resource limitations, several in-text speaker identification methods are more commonly used live contexts, yet no prior research has evaluated how these methods would perform when multiple people appear on the screen. Therefore, we investigate whether DHH viewers' preferences regarding speaker identification methods change in the presence of various numbers of onscreen speakers.
Publisher Site: RIT Repository
PDF (Requires Sign-In): PDF
Click for Bibtex
@book{amin2023investigating, title = {Investigating the Importance of Non-Textual Caption Properties from Deaf and Hard of Hearing Viewers' Perspective}, author = {Amin, Akhter Al}, year = {2023}, school = {Rochester Institute of Technology} }
[D-2023-26] Victoria Butler. (2023). “A Study of Large-Scale Structure Evolution Through Sunyaev-Zel’Dovich Corrections in Massive Clusters.” Rochester Institute of Technology.
Abstract: The evolution of large-scale structure is traced by galaxy clusters, which are groups of galaxies gravitationally bound by a shared envelope of dark matter on scales of 1-10 Mpc. They are filled with an intra-cluster medium (ICM) consisting of a diffuse hot plasma with characteristic temperature $10^7K. While ICM models match reasonably well with observations, smaller-scale astrophysical processes in clusters can complicate interpretations of derived thermodynamic properties. These can include cluster-cluster mergers, feedback from active black holes, and accretion, among several others. The complex relationship between these processes and the properties of the ICM must be understood to use clusters as a proxy for structure formation over cosmic history. My work uses two different instruments to constrain large-scale structure evolution and cluster astrophysics through observations of the diffuse ICM. The first is Herschel-SPIRE, which I used to measure cluster temperatures with the relativistic Sunyaev-Zel'dovich effect. The second is the Tomographic Ionized-carbon Mapping Experiment, which will perform observations of cluster peculiar velocities using the kinetic Sunyaev-Zel'dovich effect. These measurements will provide insight into the connection between clusters and the cosmic web of structure that influences their growth. This thesis will describe the creation and testing of an analysis pipeline to derive cluster ICM temperatures using archival SPIRE data, as well as the hardware and software development I performed for the TIME project, and my contributions during our two instrument deployments.
Publisher Site: RIT Repository
PDF (Requires Sign-In): PDF
Click for Bibtex
@book{butler2023a, title = {A Study of Large-Scale Structure Evolution Through Sunyaev-Zel'Dovich Corrections in Massive Clusters}, author = {Butler, Victoria}, year = {2023}, school = {Rochester Institute of Technology} }
[D-2023-25] Zhiyuan Li. (2023). “Unsupervised Progressive and Continual Learning of Disentangled Representations.” Rochester Institute of Technology.
Abstract: Unsupervised representation learning is an important task in machine learning that identifies and models underlying explanatory factors hidden in the observed data. In recent years, unsupervised representation learning has been attracting increasing attention for its abilities to improve interpretability, extract useful features without expert annotations, and enhance downstream tasks, which has been successful in many machine learning topics, such as Computer Vision, Natural Language Processing, and Anomaly Detection. Unsupervised representation learning has many desirable abilities, including disentangling generative factors, generalization between different domains, and incremental knowledge accumulation. However, existing works had faced two critical challenges. First, the unsupervised representation learning models were often designed to learn and disentangle all representations of data at the same time, which obstructed the models from learning representations in a more progressive and reasonable way (like from easy to hard), resulting in bad (often blurry) generation quality with the loss of detailed information. Second, when it comes to a more realistic problem setting, continual unsupervised representation learning, existing works tended to suffer from catastrophic forgetting, including forgetting learned representations and how to disentangle them. The continual disentangling problem was very difficult without modeling the relationship between data environments while the forgetting problem was often alleviated by generative-reply. In this dissertation, we are interested in developing advanced unsupervised repre- sentation learning methods based on answering three research questions: (1) how to progressively learn representations such that it can improve the quality and the disentanglement of representations, (2) how to continually learn and accumulate the knowledge of representations from different data environments, and (3) how to continually reuse the existing representations to facilitate learning and disentangling representations given new data environments. We first established a novel solution for resolving the first research question: progres- sively learn and disentangle representations and demonstrated the performance in a typical static data environment. And then, for answering the rest two research questions, we extended to study a more challenging and under-investigated set- ting: unsupervised continual learning and disentangling representations of dynamic data environments, where the proposed model is capable of not only remembering old representations but also reusing them to facilitate learning and disentangling representations in a sequential data stream. In summary, in this dissertation, we proposed several novel unsupervised repre- sentation learning methods and their applications by drawing ideas from different well-studied areas such as auto-encoders, variational inference, mixture distribu- tion, and self-organizing map. We demonstrated the presented methods on various benchmarks, such as dSprites, 3DShape, MNIST, Fashion-MNIST, and CelebA, to provide the quantitative and qualitative evaluation of the learned representations. We concluded by identifying the limitations of the proposed methods and discussing future research directions.
Publisher Site: RIT Repository
PDF (Requires Sign-In): PDF
Click for Bibtex
@book{li2023unsupervised, title = {Unsupervised Progressive and Continual Learning of Disentangled Representations}, author = {Li, Zhiyuan}, year = {2023}, school = {Rochester Institute of Technology} }
[D-2023-24] Pancy T. Lwin. (2023). “Emergent Properties of Cells and Tissues in Health and Disease.” Rochester Institute of Technology.
Abstract: This dissertation uses statistical physics based mathematical models to investigate and predict the emergent properties of biological systems in both healthy and diseased states. Three projects are presented, each exploring a different aspect of biological systems. The first project focuses on modeling cartilage-like tissue systems as a double network consisting of two interconnected networks. By using rigidity percolation theory, we study the tunable mechanics and fracture resistance of such biological tissues in healthy and degraded states. Our findings show that the secondary network density can be tuned to facilitate stress relaxation, leading to robust tissue properties when the primary network density is just above its rigidity percolation threshold. However, when the primary network is very dense, the double network becomes stiff and brittle. In the second project, we develop active double network models to investigate the interplay of actin-microtubule interactions and actomyosin dynamics in the cytoskeleton, an active network of proteins in cells. Our study reveals that the rigidity of the composite depends on the interplay between myosin-dependent crosslinking and contraction and the proximity of the actin or microtubule networks to the rigidity percolation threshold. The third project employs a viral quasispecies dynamics model to investigate the effectiveness of antiviral strategies that target different aspects of the lifecycle of cold or flu-like viruses, including COVID-19. Our results demonstrate that antivirals targeting fecundity and reproduction rates decrease the viral load linearly and via a power law, respectively. However, antivirals targeting the infection rate cause a non-monotonic change in the viral load, initially increasing and then decreasing as the infection rate is decreased, particularly for individuals with low immunity. In summary, the findings of these projects underscore the importance of understanding the underlying mechanisms behind the properties of biological cells and tissues. They also provide insights into the development of tunable, resilient, and adaptive biomaterials and the effectiveness of different antiviral strategies for COVID-19 and similar viral diseases.
Publisher Site: RIT Repository
PDF (Requires Sign-In): PDF
Click for Bibtex
@book{lwin2023emergent, title = {Emergent Properties of Cells and Tissues in Health and Disease}, author = {Lwin, Pancy T.}, year = {2023}, school = {Rochester Institute of Technology} }
[D-2023-23] Simon Peter Shipkowski. (2023). “A Novel Analytic Linear Interface Calculation and Its Use in Computational Fluid Dynamics for Enhanced Multiphase Modeling.” Rochester Institute of Technology.
Abstract: Interfacial modeling is of widespread interest in manufacturing, imaging, and scientific applications. Presented here is an interfacial reconstruction method designed for use in Computational Fluid Dynamics (CFD) simulations of nucleate boiling, yet it is more broadly applicable. Nucleate boiling is vital in current and emerging technologies due to the high heat transfer at low temperature. A challenge for multiphase CFD simulation with phase change mass flux is the competing interests of quality mass transfer conservation, resolution of interfaces, and computational time/power. This Piecewise Linear Interface Calculation-Analytic Size Based (PLIC-ASB) method was developed to mitigate this problem in the volume of fluid simulation method so that all three ends could be achieved simultaneously. Research consisted of a cyclical process of testing in an expanding parameter space to ensure improvement to the PLIC-ASB, the CFD simulation, or the testing itself. This parameter space includes data from: theoretical, numerical, and empirical sources; interface methods, and op- tions (e.g. gradient methods); integration routine sensitivity analysis, and alternate software instantiation. The main findings are: (1) the PLIC-ASB yields high levels of accuracy that converge to theoretical and empirical results as the mesh size is refined. The relative error reduction tends to be over 70% compared to the relevant industry standard. (2) The PLIC-ASB exceeded the design parameters. Symbolic formulation converts easily to code and its exactness permits a sharp interface. (3) The PLIC-ASB was less prone to error due to gradient method changes.
Publisher Site: RIT Repository
PDF (Requires Sign-In): PDF
Click for Bibtex
@book{shipkowski2023a, title = {A Novel Analytic Linear Interface Calculation and Its Use in Computational Fluid Dynamics for Enhanced Multiphase Modeling}, author = {Shipkowski, Simon Peter}, year = {2023}, school = {Rochester Institute of Technology} }
[D-2023-22] Andrew Greeley. (2023). “Vibration Powder Dispensing and Gravity-Fed Powder Delivery for Directed Energy Deposition Additive Manufacturing Systems.” Rochester Institute of Technology.
Abstract: Directed energy deposition is a class of additive manufacturing processes where focused thermal energy melts and fuses material being deposited on a part. This type of process is useful for making high performance parts, remanufacturing and repair, and for making multi-material parts. However, in systems where powder feedstock is used, only a fraction of the powder ends up in the part and the rest is usually wasted. Increasing the amount of powder captured in powder-fed directed energy deposition systems is the focus of this work. The proposed solution is to combine vibration powder dispensing and gravity-fed powder delivery systems to give very high levels of powder capture. Vibration powder dispensing involves positioning a capillary tube at the bottom of a powder hopper that will not allow flow under static conditions, then applying suitable vibration to dispense powder. Experimental and discrete element method simulation work will be performed to assess the effects of capillary size, hopper incline and vibration parameters on the outlet mass flow rate of 316L stainless steel powder. Gravity-fed powder delivery uses an inclined surface or tube to direct the powder from the dispensing system to its desired location. Experimental and discrete element method simulation work will be performed to assess the effects of tube size, tube length, inclination and mass flow rate on the trajectory and spread of 316L stainless steel powder. Desired outcomes of these experiments are a mass flow rate range of at least 1−5 g/min on a single vibrating capillary, 95% or better powder capture, and a reduced time to turn the powder flow on and off compared to a conventional system. Results show that the system is capable of consistent mass flow rates between 0.6 and 7.9 g/min, 95% powder capture efficiency and lower on and off times than the conventional system, meeting all research goals.
Publisher Site: RIT Repository
PDF (Requires Sign-In): PDF
Click for Bibtex
@book{greeley2023vibration, title = {Vibration Powder Dispensing and Gravity-Fed Powder Delivery for Directed Energy Deposition Additive Manufacturing Systems}, author = {Greeley, Andrew}, year = {2023}, school = {Rochester Institute of Technology} }
[D-2023-21] Jakob D. Hamilton. (2023). “Cast Iron Repair Through Directed Energy Deposition and Ancillary Processing Modes.” Rochester Institute of Technology.
Abstract: A cast iron repair process that restores above 80% of the original tensile strength without thermal pre-conditioning is presented. Cast iron, an integral alloy to transportation and agricultural sectors, is a difficult alloy to repair owing to the presence of discrete graphite flakes and the tendency to form brittle iron-carbide phases and porosity. Preheating and post-process annealing assist in preventing premature fracturing but are economically and environmentally impractical for large castings. Thus, methods that circumvent these limitations while avoiding defect formation are crucial. Laser-based directed energy deposition (DED) offers a highly controllable method of remanufacturing cast iron and high carbon steel components. Lower thermal input and dilution in DED contribute to less porosity and smaller thermal gradients compared to welding processes. The result is that, under a narrow range of process parameters (laser power, scanning speed, etc.), defects can be avoided. This study expands the DED processing window of cast iron and steel through ancillary processing strategies, namely, varying filler material composition, wire feeding, and melt pool vibration. Using nickel-based filler material, 82% of the cast iron ultimate tensile strength may be restored without auxiliary preheating and annealing cycles. In-situ high speed imaging complements this work by quantifying process stability under various processing conditions (laser power, scanning speed, etc.). Using low thermal input conditions, the repair strength may be maximized while reducing gas generation and escapement during melting. Wire feeding was also found to maximize process stability by reducing the amount of graphite that was vaporized or oxidized during laser exposure. A novel method of localized ultrasonic melt pool vibration was shown to refine the microstructure in deposited stainless steel 316L and promote equiaxed grain formation. The use of these technologies enhances the robustness of DED techniques, expanding the application scope and lowering the cost to entry for iron and steel repair. As society aims toward technologies that reduce greenhouse gas emissions and energy consumption, this work provides a foundation for laser-based DED restoration of cast iron and dissimilar material structures.
Publisher Site: RIT Repository
PDF (Requires Sign-In): PDF
Click for Bibtex
@book{hamilton2023cast, title = {Cast Iron Repair Through Directed Energy Deposition and Ancillary Processing Modes}, author = {Hamilton, Jakob D.}, year = {2023}, school = {Rochester Institute of Technology} }
[D-2023-20] Nibesh Shrestha. (2023). “Efficient Synchronous Byzantine Consensus.” Rochester Institute of Technology.
Abstract: With the emergence of decentralized technologies such as Blockchains, Byzantine consensus protocols have become a fundamental building block as they provide a consistent service despite some malicious and arbitrary process failures. While the Byzantine consensus problem has been extensively studied for over four decades under various settings, many challenges and open problems still exist. Improving the communication complexity and the latency or round complexity are the two key challenges in the design of efficient and scalable solutions for the Byzantine consensus problem. This thesis focuses on improving the communication complexity and the round complexity of the synchronous Byzantine consensus problem under various setup assumptions. In this thesis, I will first present OptSync, a new paradigm to achieve optimistic responsiveness that allows a consensus protocol to commit with the best-possible latency under all conditions. A lower bound that relates to the commit latencies for an optimistically responsive protocol and matching upper bound protocols with optimal commit latency under all conditions will be presented. Then, I will discuss consensus protocols in the absence of threshold setup; this setting supports efficient reconfiguration of participating parties. In this setting, I will present two efficient consensus protocols that incur quadratic communication per decision and optimistically responsive latency during optimistic conditions. Next, I will discuss the design of communication and round efficient protocols for distributed key generation (DKG). I will present a new framework to solve the DKG problem and present two new constructions following the framework. The first protocol incurs cubic communication in expectation and expected constant rounds, while the second protocol incurs cubic communication in the worst-case and linear round complexity. Improved constructions for several useful primitives such as gradecast and multi-valued validated Byzantine agreement will also be presented. Finally, I will present communication and round efficient protocols for parallel broadcast where all parties wish to broadcast their input. A generic reduction from parallel broadcast to graded parallel broadcast and validated Byzantine consensus will be presented along with improved constructions for gradecast with multiple grades and multi-valued validated Byzantine agreement.
Publisher Site: RIT Repository
PDF (Requires Sign-In): PDF
Click for Bibtex
@book{shrestha2023efficient, title = {Efficient Synchronous Byzantine Consensus}, author = {Shrestha, Nibesh}, year = {2023}, school = {Rochester Institute of Technology} }
[D-2023-19] Kimberly A. Dautel. (2023). “Development and Validation of Epidemiological Models for Informing Intervention Strategies and Preventing Critical Resource Shortages.” Rochester Institute of Technology.
Abstract: Epidemiological models have been used to measure, understand, and respond to infectious disease outbreaks. Both model development and model validation are important when utilizing models to help inform public health responses. The goal of this work is to advance mathematical modeling efforts of infectious diseases of current worldwide importance through model development and validation. The model development portion focuses on modeling interventions strategies for two infectious diseases. The first model formulated captures education campaign as the collective effort aimed at educating the public about the nature of the Ebola virus, its transmission routes, and the importance of early detection and seeking medical assistance. The basic reproduction number is computed and used to analyze the equilibria of the model for stability. Simulations of the model successfully capture the dynamics of the 2014 West Africa and 2018 Democratic Republic of Congo outbreaks. Then we developed an age-structured model for COVID-19 using contact matrices that accounts for varying contact among different ages and used it to analyze the impact vaccinations have on COVID-19 transmission by running simulations under various vaccine regimens. Model predictions aligned with the Centers for Disease Control and Prevention reports, capturing COVID-19 dynamics among various age groups with the implementation of vaccinations. Next, we developed a validation framework for epidemiological models focused on predictive capability of quantities relevant to decision-making end-users. We applied this framework to evaluate a range of COVID-19 models. We found that when predicting the date of peak deaths, the most accurate model had errors of approximately 15 days or less for releases 3-6 weeks in advance of the peak. Death peak magnitude relative errors were generally in the 50% range 3-6 weeks before peak. All models were highly variable in predictive accuracy across regions. Lastly, we evaluated the reliability of epidemiological-based medical resource demand models. We analyzed epidemiological models that predicted hospitalizations and admissions as these predictions are typically inputs into demand calculators. We also analyzed models that predicted demand for critical resources including ventilators, personal protective equipment, and testing diagnostics. We estimated errors in these models and provided recommendations to advance this nascent field.
Publisher Site: RIT Repository
PDF (Requires Sign-In): PDF
Click for Bibtex
@book{dautel2023development, title = {Development and Validation of Epidemiological Models for Informing Intervention Strategies and Preventing Critical Resource Shortages}, author = {Dautel, Kimberly A.}, year = {2023}, school = {Rochester Institute of Technology} }
[D-2023-18] Matthew van Niekerk. (2023). “Design and Optimization of Devices and Architectures for Neuromorphic Silicon Photonics.” Rochester Institute of Technology.
Abstract: Neuromorphic photonics is an exciting field at the intersection of neuroscience, integrated photonics, and microelectronics. To realize large-scale neural network-inspired systems, we must have a toolbox of linear and nonlinear operators. Here, we review state of the art for integrated photonic linear operators, which can perform linear operations using interference or wavelength-division-multiplexing (WDM) techniques, and nonlinear operators, which perform nonlinear operations with a combination of photodetection and modulation. We present some devices that fit into the needed toolbox. A new type of directional coupler, using skin depth engineering of electromagnetic waves, suppresses optical crosstalk and eliminates the need for waveguide bends. An optimized high extinction ratio microring modulator, with which we demonstrate a high operating modulation frequency while having a large extinction ratio > 25 dB. We develop and improve a technique for wafer-scale thermal isolation of optical components, allowing the demonstration of a highly efficient thermo-optic phase shifter which exhibits a measured ∼ 30x improvement on the power efficient and ∼ 25x improvement on thermal parasitic cross talk. In addition to device-level optimization and design, we create a new neuromorphic photonics architecture that combines interference with WDM to create a massively scalable, wavelength-diverse integrated photonic linear neuron. This architecture enables dramatic parallelism and physical footprint reduction, resulting in a highly scalable design system. We demonstrate this architecture with devices we optimized, showing single wavelength operation of a full neural network algorithm that adapts to three logic gates (AND, OR, XOR) with reconfiguration, achieving on-chip accuracies of 96.8%, 99%, and 98.5%, respectively. We also demonstrate this architecture by simultaneously implementing four separate logic gates (OR, AND, XOR, NAND), projecting the outputs at four distinct wavelengths, and achieving on-chip accuracies of 99.87%, 99.05%, 98.05% and 99.73%, respectively. Finally, we developed packages and implemented packaging techniques to address these increasingly complicated circuits like wire bonding, printed circuit board design, and flip-chip thermo-compression bonding. These efforts represent progress towards fully integrated neuromorphic photonic systems.
Publisher Site: RIT Repository
PDF (Requires Sign-In): PDF
Click for Bibtex
@book{van niekerk2023design, title = {Design and Optimization of Devices and Architectures for Neuromorphic Silicon Photonics}, author = {van Niekerk, Matthew}, year = {2023}, school = {Rochester Institute of Technology} }
[D-2023-17] Gaurav Shivaji Wagh. (2023). “A Privacy Safeguarding Framework for the Smart Grid.” Rochester Institute of Technology.
Abstract: The smart grid is an outcome of integrating communication technologies with traditional electrical systems. This enables the collection of granular metering data from the customer domain for providing grid and billing functionalities. However, the data collection process exposes the grid to various cyberattacks, posing a significant threat to customer privacy. This is a critical concern for the smart grid community and has hindered the global adoption of the smart grid technology. Although aggregation-based frameworks show promise for sharing metering data with the Electrical Service Provider while maintaining customer privacy, existing aggregation-based frameworks have several limitations. Some of these limitations include a high computational overhead on resource-constrained smart meters, susceptibility to single points of compromise due to dependency on a centralized entity, lack of support for dynamic billing functionality, and the absence of integrity verification capabilities for spatial and temporal metering data. To address the aforementioned limitations, we propose a distributed privacy-preserving framework for the smart grid that utilizes secret sharing, commitments, and secure multiparty computation. The framework consists of smart meters employing secret sharing and commitments to outsource their data to multiple aggregating entities, known as Dedicated Aggregators. These Dedicated Aggregators utilize secure multiparty computation to perform spatial aggregation in a privacy-preserving manner and report the aggregated readings to the Electrical Service Provider. By offloading most computations to the Dedicated Aggregators, our framework ensures that it remains lightweight for the smart meters. The introduction of multiple Dedicated Aggregators also aids in mitigating concerns associated with single points of compromise. Additionally, we have adapted the framework to support temporal aggregation, enabling dynamic billing functionalities while preserving customer privacy. The temporal aggregation process is integrated with the spatial aggregation process, thus imposing no additional computational overhead on the smart meters. The framework is designed to cater to both semi-honest and malicious adversarial settings, and works even in the presence of a majority of dishonest Dedicated Aggregators. In the event that some Dedicated Aggregators deviate from the normal execution of computing spatial and/or temporal aggregation by making modifications to metering data, the Electrical Service Provider can detect and respond to such modifications in a privacy-preserving manner. This dissertation presents our proposed framework and conducts a comprehensive analysis of it under different configurations. We develop a proof of concept to illustrate the practicality of implementing our framework in a real-world setting. We also compare its performance with other related works in the literature, evaluating the end-to-end delay for spatial aggregation. Additionally, we analyze the computational overhead on the smart meters in an embedded environment for various framework designs. The resilience of our proposed framework is analyzed against security and privacy threats. Finally, we identify future research directions to extend the capabilities of our framework.
Publisher Site: RIT Repository
PDF (Requires Sign-In): PDF
Click for Bibtex
@book{wagh2023a, title = {A Privacy Safeguarding Framework for the Smart Grid}, author = {Wagh, Gaurav Shivaji}, year = {2023}, school = {Rochester Institute of Technology} }
[D-2023-16] Samantha Emma Sarles. (2023). “Biomimetic Aerosol Exposure System for In Vitro Human Airway Exposure Studies.” Rochester Institute of Technology.
Abstract: Tobacco use remains the number one cause of preventable death in the United States, disproportionately affecting residents of rural areas, people who are financially disadvantaged, and adults who identify as gay, lesbian, or bisexual. The impact that electronic cigarettes will have on public health is not yet fully understood. Combinations of electronic cigarette products, human airway geometries, and user behaviors create barriers to understanding products' interaction with the human airway and their health effects. Current in vitro emissions systems, also called smoking robots, lack biomimicry, use unrealistic flow conditions, produce unrealistic aerosol dose, and provide inaccurate bio-mechanical cues to cell cultures, limiting ability to correlate in vitro outcomes with in vivo health effects. A Biomimetic Aerosol Exposure System, which includes an electronic cigarette adapter, an Oral Cavity Module, and a Bifurcated Exposure Chamber, was designed, manufactured, and characterized. Mass distribution, flow rate, and cell viability were studied as a function of puffing and respiration topography and system location. Accuracy of flow rate throughout the system was within 5% of programmed flow rate. Mass deposition was significantly different between the Puff Only condition and topography profiles that include ambient air inhalation cycles between puffs. Proof of concept cell culture in the system was performed with 3T3 fibroblasts cultured at an air-liquid interface and exposed to transverse aerosol flow in the Bifurcated Exposure Chamber. The Biomimetic Aerosol Exposure System was developed to increase biomimicry at three levels: the systems level, the macroscale level, and the cellular level. BAES achieves biomimicry at the systems level with the ability to perform puffing and ambient air inhalation between puffs, mimicking how a human uses an electronic cigarette. At the macroscopic level, the flow path to emissions/characterization and biological exposure subsystems utilize geometries that mimic the human airway, including surface topographies, turns, and a bifurcation. At the cellular level, the free stream angle of aerosol induces fluid shear on cells, mimicking physiological conditions. This system may broaden the utility of an emissions system to generate physiologically relevant in vitro models of exposure to inhaled aerosols.
Publisher Site: RIT Repository
PDF (Requires Sign-In): PDF
Click for Bibtex
@book{sarles2023biomimetic, title = {Biomimetic Aerosol Exposure System for In Vitro Human Airway Exposure Studies}, author = {Sarles, Samantha Emma}, year = {2023}, school = {Rochester Institute of Technology} }
[D-2023-15] Austin Bergstrom. (2023). “Understanding Image Quality for Deep Learning-Based Computer Vision.” Rochester Institute of Technology.
Abstract: Extensive research has gone into optimizing convolutional neural network (CNN) architectures for tasks such as image classification and object detection, but research to date on the relationship between input image quality and CNN prediction performance has been relatively limited. Additionally, while CNN generalization against out-of-distribution image distortions persists as a significant challenge and a focus of substantial research, a range of studies have suggested that CNNs can be be made robust to low visual quality images when the distortions are predictable. In this research, we systematically study the relationships between image quality and CNN performance on image classification and detection tasks. We find that while generalization remains a significant challenge for CNNs faced with out-of-distribution image distortions, CNN performance against low visual quality images remains strong with appropriate training, indicating the potential to expand the design trade space for sensors providing data to computer vision systems. We find that the functional form of the GIQE can predict CNN performance as a function of image degradation, but we observe that the legacy form of the GIQE does a better job of modeling the impact of blur/relative edge response in some scenarios. Additionally, we evaluate other image quality models that lack the pedigree of the GIQE and find that they generally work as well or better than the functional form of the GIQE in modeling computer vision performance on distorted images. We observe that object detector performance is qualitatively very similar to image classifier performance in the presence of image distortion. Finally, we observe that computer vision performance tends to exhibit relatively smooth, monotonic variation with blur and noise, but we find that performance is relatively insensitive to resolution under a range of conditions.
Publisher Site: RIT Repository
PDF (Requires Sign-In): PDF
Click for Bibtex
@book{bergstrom2023understanding, title = {Understanding Image Quality for Deep Learning-Based Computer Vision}, author = {Bergstrom, Austin}, year = {2023}, school = {Rochester Institute of Technology} }
[D-2023-14] Caitlin Rose. (2023). “A Morphological Analysis of High Redshift Galaxy Mergers Using Machine Learning.” Rochester Institute of Technology.
Abstract: Galaxy mergers play an important role in the formation and evolution of galaxies. However, identifying mergers can be difficult, especially at high redshift, due to effects such as: cosmological surface brightness dimming, poor resolution of images, the shifting of optical light to the infrared, and the inherently more irregular morphologies of younger galaxies. The advent of JWST and new deep, high-resolution near-infrared NIRCam images from the Cosmic Evolution Early Release Science Survey (CEERS) will help mitigate some of these problems to better detect high redshift merger features. Simultaneously, sophisticated machine learning analysis techniques have the po- tential to more accurately identify mergers by exploiting complex multidimensional data (whether directly from multi-band images or from pre-computed quantitative measurements). In this dissertation, we investigate the use of machine learning techniques (random forests and convolutional neural networks) to identify high redshift galaxy mergers. We create simulated JWST CEERS NIRCam images in six filters and HST CANDELS/Wide WFC3/ACS images in four filters from IllustrisTNG and the Santa Cruz SAM. We use these simulated data to train the algorithms. We calculate morphology parameters for galaxies in those images using Galapagos-2 and statmorph, which are used as inputs for the random forests. We also cut stamps of uniform size for those galaxies, which are used as inputs to the convolutional neural networks. The input labels for the simulated galaxies were derived from Illustris merger history catalogs, such that "mergers" are galaxies that have experienced or will experience a merger within ±250 Myr and "non-mergers" are those that will not experience a merger within that time frame. We train random forests on simulated CEERS galaxies from 0.5
Publisher Site: RIT Repository
PDF (Requires Sign-In): PDF
Click for Bibtex
@book{rose2023a, title = {A Morphological Analysis of High Redshift Galaxy Mergers Using Machine Learning}, author = {Rose, Caitlin}, year = {2023}, school = {Rochester Institute of Technology} }
[D-2023-13] David Saroff. (2023). “A Search of M31 for Giant Radio Pulses from Radio Pulsars and Magnetars.” Rochester Institute of Technology.
Abstract: We present results of a 40-hour survey of the nearby galaxy M31 for giant radio pulses (GRPs) from radio pulsars using the Green Bank Radio telescope (GBT) in the 300 MHz to 400 MHz band. We note that GRPs from known pulsars in the Milky Way or its nearest companion galaxies, the Magellanic clouds, if relocated to M31, would not be detectable with the GBT in the 300 MHz to 400 MHz band. However, the brightest GRPs from a pulsar in M31, like the Crab pulsar B0531+21, are within a factor of 2 of detectability. Our observations cover a projected surface area of 8000 kpc2 at the distance of M31 including the Galactic bulge and most of the stellar disk. M32 is included, but M110 is not. The dwarf galaxy AND III is partially sampled. We analyze sources of interference including meteor head scattering of military and civilian air traffic control channels into the GBT beam during the 2015 Orionid and Taurid meteor showers coincident with our observing time. We report our methods for interference mitigation and methods for visualizing and exploring the data. Dispersion measures (DM) from 30 pc cm−3 to 500 pc cm−3 were searched using the PRESTO library. There are no detections of pulses which repeated regularly during our 2.3-minute individual pointings. However, there are detections of candidate events which are either isolated in time or have one or two repetitions during our observations. These candidates have dispersion measures which are plausible values for M31. We detect a candidate event which has a dispersion measure of 54.7 pc cm−3 which is the value of the 6 events reported by [Rubio-Herrera et al., 2013]. Our observations are consistent with a search for pulses in M31 with LOFAR. If any of our candidates are real events, there must be a population of objects in M31 which are more luminous than the Crab pulsar and which emit very luminous pulses intermittently.
Publisher Site: RIT Repository
PDF (Requires Sign-In): PDF
Click for Bibtex
@book{saroff2023a, title = {A Search of M31 for Giant Radio Pulses from Radio Pulsars and Magnetars}, author = {Saroff, David}, year = {2023}, school = {Rochester Institute of Technology} }
[D-2023-12] Prashant Sankaran. (2023). “Deep Reinforcement Learning and Hybrid Approaches to Solve Multi-Vehicle Combinatorial Optimization Problems.” Rochester Institute of Technology.
Abstract: Combinatorial optimization problems are an important class of problems often encountered in the real world involving a combinatorially growing set of feasible solutions as the problem size increases. Since exact approaches can be computationally expensive, practitioners often use approximate approaches such as metaheuristics. However, sophisticated approximate methods that yield high-quality solutions require expert help to handcraft or fine-tune the solution process to suit a given problem distribution. In recent years, artificial intelligence (AI) approaches that involve learning from data without being explicitly programmed have shown tremendous success at various challenging tasks, like natural language processing and autonomous driving. Therefore, solving combinatorial optimization problems is an ideal use case for AI approaches. In this dissertation, we find answers to two key questions considering recent AI developments. 1) How to use deep reinforcement learning (DRL) approaches to solve complex multi-vehicle combinatorial optimization problems. 2) Can combining machine learning, metaheuristics, and mixed integer-linear optimization solvers under a hybrid framework help quickly obtain certifiable high-quality solutions for combinatorial optimization problems? The answer to these questions broadly builds on two key directions: DRL and hybrid approaches to tackle challenging multi-vehicle combinatorial optimization problems considering the recent advancements, gaps, and drawbacks. Specifically, in Part I of this dissertation, DRL-based approximate approaches are developed to learn from complex edge features, reason over uncertain edges, and handle multi-vehicle decoding and collaboration to solve complex multi-vehicle combinatorial optimization problems. Additionally, we develop approaches to generate large-scale complex data on the fly for training. Upon experimental evaluation, we learn that DRL-based approaches can quickly generate high-quality solutions to complex scenarios and, in the best case, yields a minimum improvement of 3.44% and, in the worst case, at par with traditional and other baseline DRL methods. Next, in Part II of this dissertation, a hybrid-learning optimization framework (HyLOS), combining machine learning, metaheuristics, and mixed integer-linear optimization, is developed to solve challenging combinatorial optimization problems quickly. Experimental studies reveal that the HyLOS framework can collaboratively and effectively utilize metaheuristics, machine learning, and MILP approaches to generate high-quality solutions quickly and, on average, yield a minimum improvement of 48% against all individuals and subsets of solvers.
Publisher Site: RIT Repository
PDF (Requires Sign-In): PDF
Click for Bibtex
@book{sankaran2023deep, title = {Deep Reinforcement Learning and Hybrid Approaches to Solve Multi-Vehicle Combinatorial Optimization Problems}, author = {Sankaran, Prashant}, year = {2023}, school = {Rochester Institute of Technology} }
[D-2023-11] Anna Starynska. (2023). “Text Restoration in Palimpsested Historical Manuscripts Using Deep Learning Methods.” Rochester Institute of Technology.
Abstract: Historical palimpsests are manuscripts that have more than one layer of text, where the original text was erased, and subsequent layers overwritten. For many centuries, palimpsested text of ancient manuscripts was considered impossible to recover. Nowadays, with new technology, such as multispectral imaging, under some portion of electromagnetic spectrum illumination, the overwritten text can become visible again. This peculiar property of faded ink and some traditional signal processing methods had already helped recover text from numerous manuscripts. Nevertheless, even showing the best performance, existing methods can only enhance the visibility of the text but not eliminate the effects of occlusion from the overlapping text, stains, and fading of aged parchment. The dependency of the performance of these methods on material variation caused by aging, ink composition, and the text-erasing process, is not well understood. This factors makes their performance very inconsistent and parameter tuning very labor-intensive. As a solutions, we suggest reconstructing the palimpsest text using deep neural networks that have already proved to be the leading technology in the field of image reconstruction, in-painting, and super-resolution. Additionally, we present a method that combines spectral information from a multispectral imaging system with spatial statistics of palimpsest text.
Publisher Site: RIT Repository
PDF (Requires Sign-In): PDF
Click for Bibtex
@book{starynska2023text, title = {Text Restoration in Palimpsested Historical Manuscripts Using Deep Learning Methods}, author = {Starynska, Anna}, year = {2023}, school = {Rochester Institute of Technology} }
[D-2023-10] Lauren Melcher. (2023). “Equilibrium and Active Self-Assembly and Collective Properties of Network-Based Biomaterials.” Rochester Institute of Technology.
Abstract: Biological systems have the unique ability to self-organize, respond to environmental stimuli, and generate autonomous motion and work. The fields of biomaterials and synthetic biology seek to recapitulate these remarkable properties of biological systems in in-vitro reconstitutions of biological systems, and in abiotic or biotic-abiotic hybrid materials to better understand the underlying rules of life and to predict the design principles of biomimetic smart materials. Motivated by this, we create bottom-up mathematical models, rooted in experiments, of network-based synthetic and biological materials. These encompass two-dimensional colloidal networks connected by rhythmic crosslinkers, and three-dimensional biopolymer networks assembled via depletion interactions, physical crosslinking, and adhesive proteins. We use Langevin Dynamics simulations to investigate the spatiotemporal evolution of these systems, probing and characterizing their emergent collective dynamics and structural properties. We refine our models by comparing our results with experimental data when available and make predictions of mechanisms driving the observed collective behavior and possibilities for modulation. By providing insights on what kinds of behavior to expect in different areas of the phase space, we use results from our model studies to help inform the rational design of bio-inspired soft materials with desired properties.
Publisher Site: RIT Repository
PDF (Requires Sign-In): PDF
Click for Bibtex
@book{melcher2023equilibrium, title = {Equilibrium and Active Self-Assembly and Collective Properties of Network-Based Biomaterials}, author = {Melcher, Lauren}, year = {2023}, school = {Rochester Institute of Technology} }
[D-2023-09] Benjamin S. Meyers. (2023). “Human Error Assessment in Software Engineering.” Rochester Institute of Technology.
Abstract: Software engineers work under strict constraints, balancing a complex, multi-phase development process on top of user support and professional development. Despite their best efforts, software engineers experience human errors, which manifest as software defects. While some defects are simple bugs, others can be costly security vulnerabilities. Practices such as defect tracking and vulnerability disclosure help software engineers reflect on the outcomes of their human errors (i.e. software failures), and even the faults that led to those failures, but not the underlying human behaviors. While human error theory from psychology research has been studied and applied to medical, industrial, and aviation accidents, researchers are only beginning to systematically reflect on software engineers' human errors. Some software engineering research has used human error theories from psychology to help developers identify and organize their human errors (mistakes) during requirements engineering activities, but developers need an improved and systematic way to reflect on their human errors during other phases of software development. The goal of this dissertation is to help software engineers confront and reflect on their human errors by creating a process to document, organize, and analyze human errors. To that end, our research comprises three phases: (1) systematization (i.e. identification and taxonomization) of software engineers' human errors from literature and development artifacts into a Taxonomy of Human Errors in Software Engineering (T.H.E.S.E.), (2) evaluation and refinement of T.H.E.S.E. based on software engineers' perceptions and natural language insights, and (3) creation of a human error informed micro post-mortem process and the Human Error Reflection Engine (H.E.R.E.), a proof-of-concept GitHub workflow facilitating human error reflection. In demonstrating the utility of T.H.E.S.E. and our micro post-mortem process, the software development community will be closer to inculcating the wisdom of historical developer human errors, enabling them to engineer higher quality and more secure software.
Publisher Site: RIT Repository
PDF (Requires Sign-In): PDF
Click for Bibtex
@book{meyers2023human, title = {Human Error Assessment in Software Engineering}, author = {Meyers, Benjamin S.}, year = {2023}, school = {Rochester Institute of Technology} }
[D-2023-08] Hitesh Sapkota. (2023). “Robust Weakly Supervised Learning for Real-World Anomaly Detection.” Rochester Institute of Technology.
Abstract: Anomaly detection has attracted increasing attentions from diverse domains, including medicine, public safety, and military operations. Despite its wide applicability, anomaly detection is inherently challenging as abnormal activities are usually rare and unbounded in nature. This makes it difficult and expensive to identify all potential anomalous events and label them during the training phase so that a detection model can provide robust prediction on unseen event data. Unsupervised and semi-supervised learning models have been explored, which achieve a decent detection performance with no or much less annotations. However, these models can be highly sensitive to outliers (\ie normal samples that look different from other normal ones) or multimodal scenarios (\ie existence of multiple types of anomalies), leading to much worse detection performance under these situations. The imbalanced class distribution of the normal data samples poses a further challenge as the model may be confused between the normal samples from a minority class and true anomalies. To systematically address the key challenges outlined above, this dissertation contributes the first Robust Weakly Supervised Learning (RWSL) framework that provides fundamental support for real-world anomaly detection using only weak and/or sparse learning signals. The proposed RWSL framework offers a principled learning paradigm to deal with the rare and unbounded nature of real-world anomalies, which allows a statistical learning model to be robustly trained using only high-level supervised signals while generalizing well in few-shot settings. The framework is comprised of three interconnected components. The first research component integrates Robust Distributionally Optimization (DRO) with Bayesian learning, leading to a novel Bayesian DRO model that achieves robust detection performance using weak learning signals coupled with outliers and multimodal anomalies. The Bayesian DRO model is further augmented with non-parametric submodular optimization and active instance sampling to improve both the reliability and accuracy of the detection performance. The second research component leverages the evidential theory and its fine-grained uncertainty formulation to tackle anomaly detection coupled with imbalanced class distribution of normal data samples. An adaptive Distributionally Robust Evidential Optimization (DREO) training process is developed to boost the anomaly detection performance by accurately differentiating minority class samples and true anomalies using evidential uncertainty. Evidential learning is further integrated with a transformer architecture, leading to an Evidential Meta Transformer (MET) for reliable anomaly detection in the few-short setting. Finally, the third research component aims to ensure an unbiased (\ie fair) and better-calibrated model with improved anomaly detection performance by avoiding the overconfidence predictions stemming from the memorization effect seen in deep neural networks. To achieve this, the Distributionally Robust Ensemble (DRE) is proposed that learns multiple diverse and complementary sparse sub-networks through the utilization of DRO properties. By facilitating these sparse sub-networks to capture different data distributions across varying levels of complexity, they naturally complement each other resulting in improved model calibration with enhanced anomaly detection capability.
Publisher Site: RIT Repository
PDF (Requires Sign-In): PDF
Click for Bibtex
@book{sapkota2023robust, title = {Robust Weakly Supervised Learning for Real-World Anomaly Detection}, author = {Sapkota, Hitesh}, year = {2023}, school = {Rochester Institute of Technology} }
[D-2023-07] Seyed Hamed Fatemi Langroudi. (2023). “Tapered-Precision Numerical Formats for Deep Learning Inference and Training.” Rochester Institute of Technology.
Abstract: The demand to deploy deep learning models on edge devices has recently increased due to their pervasiveness, in applications ranging from healthcare to precision agriculture. However, a major challenge with current deep learning models, is their computational complexity. One approach to address this limitation is to compress the deep learning models by employing low-precision numerical formats. Such low-precision models often suffer from degraded inference or training accuracy. This lends itself to the question, which low-precision numerical format can meet the objective of high training accuracy with minimal resources? This research introduces tapered-precision numerical formats for deep learning inference and training. These formats have inherent capability to match the distribution of deep learning parameters by expressing values in unequal-magnitude spacing such that the density of values is maximum near zero and is tapered towards the maximum representable number. We develop low-precision arithmetic frameworks, that utilize tapered precision numerical formats to enhance the performance of deep learning inference and training. Further, we develop a software/hardware co-design framework to identify the right format for inference based on user-defined constraints through integer linear programming optimization. Third, novel adaptive low-precision algorithms are proposed that match the tapered-precision numerical format configuration to best represent the layerwise dynamic range and distribution of parameters within a deep learning model. Finally, a numerical analysis approach and signal-to-quantization-noise ratio equation for tapered-precision numerical formats are proposed that uses a metric to select the appropriate numerical format configuration. The efficacy of the proposed approaches is demonstrated on various benchmarks. Results assert that the accuracy and hardware cost trade-off of low-precision deep neural networks using tapered precision numerical formats outperform other well-known numerical formats, including floating point and fixed-point.
Publisher Site: RIT Repository
PDF (Requires Sign-In): PDF
Click for Bibtex
@book{fatemi langroudi2023tapered-precision, title = {Tapered-Precision Numerical Formats for Deep Learning Inference and Training}, author = {Fatemi Langroudi, Seyed Hamed}, year = {2023}, school = {Rochester Institute of Technology} }
[D-2023-06] Emily Kessler-Lewis. (2023). “Demonstration of a Hybrid Electroabsorption Modulator/Photovoltaic Device for Space-Based Free Space Optical Communication and Power Generation.” Rochester Institute of Technology.
Abstract: The emergence of proliferated low-Earth orbit (pLEO) constellations using small satellites has resulted in significant interest in lowering the size, weight, and power (SWaP) of mission critical subsystems. III-V semiconductors are the class of materials typically used for photovoltaic (PV) arrays for satellites due to their exceptional efficiencies of upwards of 35 % and ability to be made thin, flexible, and lightweight. This is contrasted with the high SWaP communication system, which utilizes a radio frequency (RF) transceiver in order to communicate with a ground station. A lower SWaP alternative to the RF transceiver is to transmit data using free space optical (FSO) communication. FSO communication at 1.55 µm is of particular interest due to the wavelength being inherently eye safe and existing infrastructure from the telecommunications industry. This research presents on a hybrid power generation/data communication device using III-V PV as the power generating component and an InP-based multiple quantum well (MQW) electroabsorption modulator (EAM) targeted for operation at 1.55 µm as the data communication component. A MQW EAM utilizes the quantum confined Stark effect (QCSE) to shift the absorption coefficient of the material; using the QCSE amplitude modulation of a signal is possible over FSO communication. To be hybridized with a PV device, a surface normal EAM is required. While it is advantageous to have a large area to aid pointing accuracy with FSO communication, the EAM suffers from decreased bandwidth due to area-dominated capacitive effects. These capacitive effects were extensively studied, and data rates ranging from 0.25 to 1 Mbps were demonstrated. Additionally, in order to accurately target device operation at 1.55 µm, the thickness of the InGaAs quantum well region and the InAlAs barriers were investigated through both simulation and experimental work. Two device architectures were investigated; a four-terminal, mechanically bonded hybrid device and a three-terminal, monolithically integrated device. The four-terminal, mechanically bonded device allows growth of the PV device to be conducted on a GaAs substrate, enabling higher power conversion efficiencies compared to growth on InP. A dual junction InGaP/GaAs photovoltaic device with an AM0 power conversion efficiency of 23 % was mechanically bonded to an InP-based EAM in a 0.5 U form factor module. Using a segmented modulator design, a data rate for the module of 0.5 Mbps was demonstrated. The three-terminal device, all grown monolithically on InP, has the advantage of only requiring one growth and one fabrication. In addition, the monolithically integrated device further reduces the SWAP of the hybrid device by 50 % as only one substrate is required. This design required careful consideration of the shared contact layer between the PV device and EAM, needing to balance fractional power loss in the PV device and parasitic absorption of 1.55 µm light. A single junction InP PV device was grown a top of an InGaAs/InAlAs EAM, demonstrating simultaneous power generation and data transmission, and to our best knowledge demonstrated the first hybrid, monolithically integrated device to be successfully fabricated. These hybrid devices have applications that extend beyond satellites, such as unmanned aerial vehicles, orbital debris tagging, and implantable medical devices.
Publisher Site: RIT Repository
PDF (Requires Sign-In): PDF
Click for Bibtex
@book{kessler-lewis2023demonstration, title = {Demonstration of a Hybrid Electroabsorption Modulator/Photovoltaic Device for Space-Based Free Space Optical Communication and Power Generation}, author = {Kessler-Lewis, Emily}, year = {2023}, school = {Rochester Institute of Technology} }
[D-2023-05] Krishna Prasad Neupane. (2023). “Learning to Learn from Sparse User Interactions.” Rochester Institute of Technology.
Abstract: The ability to learn from user interactions provides an effective means to understand user intent through their behaviors that is instrumental to improve user engagement, incorporate user feedback, and gauge user satisfaction. By leveraging important cues embedded in such interactions, a learning system can collect key evidence to uncover users' cognitive, affective, and behavioral factors, all of which are critical to maintain and/or increase its user base. However, in practical settings, interactive systems are still challenged by sparse user interactions that are also dynamic, noisy, and highly heterogeneous. As a result, both traditional statistical learning methods and contemporary deep neural networks (DNNs) may not be properly trained to learn meaningful patterns from sparse, noisy, and constantly changing learning signals. In recent years, the learning to learn (L2L) (or meta-learning) paradigm has been increasingly leveraged in diverse application domains, such as computer vision, gaming, and healthcare to improve the learning capacity from sparse data. L2L tries to mimic the way how humans learn from many tasks that allows them to generalize often from extremely few examples. Inspired by such an attractive learning paradigm, this dissertation aims to contribute a novel L2L framework that is able to effectively learn and generalize well from sparse user interactions to collectively address the challenges of learning from sparse user interactions. The L2L framework is comprised of four interconnected components. The first component focuses on learning from sparse interactions that constantly change over time. For this, we introduce a Dynamic Meta Learning (DML) model that integrates a meta learning module with a sequential learning module, where data sparsity is tackled by the first module and the later module captures constantly changing user interaction behaviors. The second component focuses on dealing with noisy interactions to detect true user intent through uncertainty quantification. This component integrates evidential learning with meta learning, in which the former quantifies the uncertainty by leveraging evidence of each interaction and the later tackles sparse interactions. Furthermore, the concept of evidence is utilized to guide the predictive model to find more important and informative interactions in sparse data to enhance model training. The third component emphasizes dealing with dynamic and heterogeneous user behavior in sparse interactions. This component aims to ensure long-term user satisfaction by combining reinforcement learning, which handles constantly evolving user behaviors and evidential learning, which leverages evidence-based exploration to tackle data heterogeneity. The last component aims to advance the contemporary dynamic models which require to partition the time into arbitrary intervals to support model training and inference. We develop a novel Neural Stochastic Differential Equation (NSDE) model in the L2L setting that captures continuously changing user behavior and integrates with evidential theory to achieve evidence-guided learning. This method leverages the power of a NSDE solver to capture user's continuously evolving preferences over time which results in richer user representation than previous discrete dynamic methods. Furthermore, we derive a mathematical relationship between the interaction time gap and model uncertainty to provide effective recommendations.
Publisher Site: RIT Repository
PDF (Requires Sign-In): PDF
Click for Bibtex
@book{neupane2023learning, title = {Learning to Learn from Sparse User Interactions}, author = {Neupane, Krishna Prasad}, year = {2023}, school = {Rochester Institute of Technology} }
[D-2023-04] Masoud Abdollahi. (2023). “A Comprehensive Machine Learning Approach to Assess Fall Risk in Stroke Survivors: Integrating Common Clinical Tests, Motor-Cognitive Dual-Tasks, Wearable Motion Sensors, and Detailed Motion Analysis.” Rochester Institute of Technology.
Abstract: This PhD dissertation focuses on developing improved methods for assessing and reducing fall risk in stroke survivors. Falls are a major concern in this population as they occur more frequently and can lead to catastrophic injuries due to factors like hemiparesis, spasticity, impaired balance and cognitive deficits. The dissertation reviews fall risk factors (FRFs) identified in prior studies and finds balance, gait and mobility metrics to be most significant. It notes current assessment methods rely on subjective scales and lack detailed motion analysis. Dual-task testing is also overlooked despite revealing subtle deficits. To address these gaps, wearable sensors and machine learning are proposed to objectively quantify fall risk. The dissertation first compares outcomes of clinical tests like Timed Up and Go (TUG), Sit-to-Stand (STS), balance and 10 Meter Walk Test (10MWT) between stroke survivors and controls under single and dual-task conditions. Stroke survivors show impaired performance exacerbated by dual-tasking, indicating utility of this approach. The next study instruments the tests with inertial sensors to enable precise motion analysis. Key deficits in turning, transitions and gait are identified in stroke survivors using features like trunk sway and velocities. Dual-tasking disproportionately worsens their performance, stressing limited cognitive-motor resources. A machine learning model using a test battery of instrumented clinical tasks under single and dual-task conditions is then developed to classify fall risk. Random forest model with two features related to medio-lateral sway during dual-task balance and gait speed achieves 91% accuracy. Analysis of sensor configurations finds a single thorax sensor effectively captures fall risk biomechanics. The model could be applied to assess rehabilitation exercises, demonstrating feasibility of tracking risk over time. In conclusion, the dissertation presents wearable sensor-based movement analysis and machine learning models as a quantitative framework for objective fall risk assessment in stroke survivors. Dual-task testing enhances sensitivity. A single thorax sensor capturing key metrics during clinical tests can efficiently evaluate risk. This could assist clinicians in prescribing interventions and tracking rehabilitation progress. With further validation, the approach may be translated to point-of-care screening and remote monitoring to improve prevention and quality of life after stroke.
Publisher Site: RIT Repository
PDF (Requires Sign-In): PDF
Click for Bibtex
@book{abdollahi2023a, title = {A Comprehensive Machine Learning Approach to Assess Fall Risk in Stroke Survivors: Integrating Common Clinical Tests, Motor-Cognitive Dual-Tasks, Wearable Motion Sensors, and Detailed Motion Analysis}, author = {Abdollahi, Masoud}, year = {2023}, school = {Rochester Institute of Technology} }
[D-2023-03] Alireza Abrand. (2023). “Catalyst-Free Epitaxy of Core-Shell Nanowire Arrays for Wavelength-Selective and Substrate-Free Optoelectronic Devices.” Rochester Institute of Technology.
Abstract: This research is dedicated to advancing catalyst-free epitaxial growth methods for indium arsenide-based nanowires (NWs) on foreign substrates, including silicon and two-dimensional (2D) materials. The findings presented here demonstrate new methods for low-cost III-V semiconductor NW synthesis, manipulation of NW optical properties, and advanced techniques for modulation of NW geometry and composition during selective-area epitaxy (SAE). The first part of this work explores the growth of InAs NWs with sub-lithographic dimensions on reusable Si (111), toward NW membrane-based optoelectronic devices such as infrared photodetectors (IR PDs). A SiO2 masking template is first patterned using i-line photolithography, yielding hexagonal arrays of nanopores. InAs NW arrays are then grown through a novel localized self-assembly (LSA) method using metalorganic chemical vapor deposition (MOCVD). The yield of NWs is optimized through the introduction of a two-step flowrate-modulated growth technique. The NW arrays are embedded in a polymer membrane, delaminated, and transferred to carrier substrates. The process achieves ~100% transfer yields, fully preserving NW position and orientation during transfer. The starting SiO2-templated Si (111) substrates are then reused for LSA growth of subsequent NW generations, demonstrating reproducible global yields of > 85% over wafer-scale areas. This substrate recycling approach aims to reduce the manufacturing costs of III-V NW-based membranes for large-area, flexible, and wearable optoelectronic devices. Next, the influence of an integrated backside reflector on the optical properties of NW-based membranes is investigated. Simulations performed using the rigorous coupled-wave analysis (RCWA) technique are employed to demonstrate significant tunability of the IR absorption spectra of coaxially heterostructured NW arrays, where InAs core segments are partially encapsulated by GaAs0.1Sb0.9 shell layers. The integration of Au backside contact layers creates periodic evanescent fields between adjacent NWs, dependent on the NW core segment diameter and incident light wavelength. By introducing partial GaAs0.1Sb0.9 shell layers with dimensions aligned to the evanescent field, selective absorption of otherwise decoupled light in the 2 to 3 µm range is realized within the InAs NW core segments. This site-specific absorption, which can be engineered as a function of the NW shell segment geometry, offers new opportunities for the development of tunable IR photodetector device structures. Lastly, we introduce a method to locally modulate the effective precursor flowrates during SAE growth by MOCVD in order to manipulate NW dimensions and compositions. Our findings demonstrate a significant growth rate modulation effect, resulting in an eight-fold volumetric enhancement ratio compared to control samples. This technique allows for the fabrication of III-V NWs with adjustable geometries on the same substrate, using only a single nanopore masking pattern. Furthermore, by leveraging the growth rate enhancement effect, we demonstrate the ability to alter the composition of adjacent AlxIn(1-x)As NW arrays within the 0.11 ≤ x ≤ 0.54 range during a single SAE run. This provides new approaches for the growth and fabrication of NW-based optoelectronic devices, particularly beneficial for multispectral photodetector applications.
Publisher Site: RIT Repository
PDF (Requires Sign-In): PDF
Click for Bibtex
@book{abrand2023catalyst-free, title = {Catalyst-Free Epitaxy of Core-Shell Nanowire Arrays for Wavelength-Selective and Substrate-Free Optoelectronic Devices}, author = {Abrand, Alireza}, year = {2023}, school = {Rochester Institute of Technology} }
[D-2023-02] Luke Hellwig. (2023). “Novel Psychophysics of Cognitive Color Appearance Phenomena.” Rochester Institute of Technology.
Abstract: Color appearance models can be used as an experimental design and analysis tool to better study high-level color perception involving brightness and chromatic adaptation. We investigate the experimental basis for the Helmholtz-Kohlrausch effect, the contribution of chromatic intensity to our perception of brightness. A new experimental method for measuring the brightness of chromatic colors leads to a model of the Helmholtz-Kohlrausch effect, which we use to extend CIECAM16, the color appearance model recommended by the Commission Internationale de l'Eclairage. The model is tested on high-dynamic-range images. The process of building this model also leads to several improvements in CIECAM16 itself. We then investigate how color appearance models can similarly be used to design experiments and model cognitive mechanisms of discounting the color of illumination. Two different experimental modalities are used to separately measure sensory and cognitive mechanisms of chromatic adaptation to heterochromatic lighting conditions. The results provide insight into this cognitive phenomenon while also setting new benchmark processes for studies that use asymmetric color matching.
Publisher Site: RIT Repository
PDF (Requires Sign-In): PDF
Click for Bibtex
@book{hellwig2023novel, title = {Novel Psychophysics of Cognitive Color Appearance Phenomena}, author = {Hellwig, Luke}, year = {2023}, school = {Rochester Institute of Technology} }
[D-2023-01] Nicholas Soures. (2023). “Lifelong Learning in Spiking Neural Networks Through Neural Plasticity.” Rochester Institute of Technology.
Abstract: Lifelong learning, the ability to learn from continually changing data distributions in real-time, is a significant challenge in artificial intelligence. The central issue is that new learning tends to interfere with previously acquired memories, a phenomenon known as catastrophic forgetting. Surrounding this are several other challenges associated with this, such as knowledge transfer and adaptation, few-shot learning, and processing of noisy data. Since humans do not seem to suffer from this problem, researchers have applied biologically inspired techniques to address it, including metaplasticity, synaptic consolidation and memory replay. Although these approaches have seen some success in traditional neural networks, there is limited exploration of how to support lifelong learning in spiking networks. In general, spiking neural networks are efficient for resource-constrained environments due to inherent sparse, asynchronous, and low-precision computation. However, spiking networks require a different set of learning rules from traditional rate-based models. Few works highlight that the application of simple Hebbian rules can support aspects of lifelong learning. However, there is a significant gap in understanding how spiking networks can solve complex tasks experienced in a lifelong learning setting. We propose to address this by using compositional biological mechanisms, where these networks can overcome the limitations of simple Hebbian models. In this work, we present NACHOS, a model that integrates multiple biologically inspired mechanisms to promote lifelong learning at both the synapse-level and the network-level. At the synapse-level, NACHOS uses regularization and homeostatic mechanisms to protect the information learned over time. At the network level, NACHOS introduces heterogeneous learning rules and dynamic architecture to form distributed processing across tasks without impeding learning. These mechanisms work in tandem to significantly boost the performance of baseline spiking networks by 3x in a lifelong learning scenario. Unlike Hebbian approaches, they are also scalable beyond a single-layer. The key features of NACHOS are that (a) it operates without task knowledge, (b) it is evaluated on online continual learning, (c) it does not grow over time, and (d) it has better energy - accuracy trade-offs compared to existing rate-based models. This enables the model to be deployed in the wild, where the AI system is not aware of task switching. NACHOS is demonstrated on several lifelong learning scenarios, where it matches performance of state-of-the-art non-spiking lifelong learning models. In summary, we explore the role of spiking networks in lifelong learning and provide a blueprint for adoption into resource-constrained environments.
Publisher Site: RIT Repository
PDF (Requires Sign-In): PDF
Click for Bibtex
@book{soures2023lifelong, title = {Lifelong Learning in Spiking Neural Networks Through Neural Plasticity}, author = {Soures, Nicholas}, year = {2023}, school = {Rochester Institute of Technology} }
[D-2022-15] Olivia Young. (2022). “CLEAN Deconvolution of Radio Pulsar Pulses.” Rochester Institute of Technology.
Abstract: Broadband radio waves emitted from pulsars are distorted as they propagate toward Earth due to interactions with the free electrons that comprise the interstellar medium (ISM). Irregularities in the ISM cause multipath propagation of the wavefronts along the line of sight toward Earth, with lower radio frequencies being more greatly impacted than higher frequencies. These delays result in later times of arrival for the lower frequencies and cause the observed pulse to arrive with a broadened tail, which can be described using a pulse broadening function. CLEAN deconvolution, as outlined in Bhat et al. (2003) for use in pulsar scattering measurements, can be employed to recover both the intrinsic pulse shape and pulse broadening function of radio pulsar pulse profiles, thus quantifying the effect the ISM has on radio pulsar emission. This work expands upon that done by Bhat et al. (2003) by developing a more robust CLEAN deconvolution algorithm in Python, parameterizing the algorithm via comparison to recent cyclic spectroscopy methods outlined in Dolch et al. (2021), and the deployment of the algorithm on observational data of the highly scattered millisecond pulsar J1903+0327.
Publisher Site: RIT Repository
PDF (Requires Sign-In): PDF
Click for Bibtex
@book{young2022clean, title = {CLEAN Deconvolution of Radio Pulsar Pulses}, author = {Young, Olivia}, year = {2022}, school = {Rochester Institute of Technology} }
[D-2022-14] Abu Niamul Taufique. (2022). “Deep Feature Learning and Adaptation for Computer Vision.” Rochester Institute of Technology.
Abstract: We are living in times when a revolution of deep learning is taking place. In general, deep learning models have a backbone that extracts features from the input data followed by task-specific layers, e.g. for classification. This dissertation proposes various deep feature extraction and adaptation methods to improve task-specific learning, such as visual re-identification, tracking, and domain adaptation. The vehicle re-identification (VRID) task requires identifying a given vehicle among a set of vehicles under variations in viewpoint, illumination, partial occlusion, and background clutter. We propose a novel local graph aggregation module for feature extraction to improve VRID performance. We also utilize a class-balanced loss to compensate for the unbalanced class distribution in the training dataset. Overall, our framework achieves state-of-the-art (SOTA) performance in multiple VRID benchmarks. We further extend our VRID method for visual object tracking under occlusion conditions. We motivate visual object tracking from aerial platforms by conducting a benchmarking of tracking methods on aerial datasets. Our study reveals that the current techniques have limited capabilities to re-identify objects when fully occluded or out of view. The Siamese network based trackers perform well compared to others in overall tracking performance. We utilize our VRID work in visual object tracking and propose Siam-ReID, a novel tracking method using a Siamese network and VRID technique. In another approach, we propose SiamGauss, a novel Siamese network with a Gaussian Head for improved confuser suppression and real time performance. Our approach achieves SOTA performance on aerial visual object tracking datasets. A related area of research is developing deep learning based domain adaptation techniques. We propose continual unsupervised domain adaptation, a novel paradigm for domain adaptation in data constrained environments. We show that existing works fail to generalize when the target domain data are acquired in small batches. We propose to use a buffer to store samples that are previously seen by the network and a novel loss function to improve the performance of continual domain adaptation. We further extend our continual unsupervised domain adaptation research for gradually varying domains. Our method outperforms several SOTA methods even though they have the entire domain data available during adaptation.
Publisher Site: RIT Repository
PDF (Requires Sign-In): PDF
Click for Bibtex
@book{taufique2022deep, title = {Deep Feature Learning and Adaptation for Computer Vision}, author = {Taufique, Abu Niamul}, year = {2022}, school = {Rochester Institute of Technology} }
[D-2022-13] Aayush Kumar Chaudhary. (2022). “Deep into the Eyes: Applying Machine Learning to Improve Eye-Tracking.” Rochester Institute of Technology.
Abstract: Eye-tracking has been an active research area with applications in personal and behav- ioral studies, medical diagnosis, virtual reality, and mixed reality applications. Improving the robustness, generalizability, accuracy, and precision of eye-trackers while maintaining privacy is crucial. Unfortunately, many existing low-cost portable commercial eye trackers suffer from signal artifacts and a low signal-to-noise ratio. These trackers are highly depen- dent on low-level features such as pupil edges or diffused bright spots in order to precisely localize the pupil and corneal reflection. As a result, they are not reliable for studying eye movements that require high precision, such as microsaccades, smooth pursuit, and ver- gence. Additionally, these methods suffer from reflective artifacts, occlusion of the pupil boundary by the eyelid and often require a manual update of person-dependent parame- ters to identify the pupil region. In this dissertation, I demonstrate (I) a new method to improve precision while maintaining the accuracy of head-fixed eye trackers by combin- ing velocity information from iris textures across frames with position information, (II) a generalized semantic segmentation framework for identifying eye regions with a further extension to identify ellipse fits on the pupil and iris, (III) a data-driven rendering pipeline to generate a temporally contiguous synthetic dataset for use in many eye-tracking ap- plications, and (IV) a novel strategy to preserve privacy in eye videos captured as part of the eye-tracking process. My work also provides the foundation for future research by addressing critical questions like the suitability of using synthetic datasets to improve eye-tracking performance in real-world applications, and ways to improve the precision of future commercial eye trackers with improved camera specifications.
Publisher Site: RIT Repository
PDF (Requires Sign-In): PDF
Click for Bibtex
@book{chaudhary2022deep, title = {Deep into the Eyes: Applying Machine Learning to Improve Eye-Tracking}, author = {Chaudhary, Aayush Kumar}, year = {2022}, school = {Rochester Institute of Technology} }
[D-2022-12] Sulabh Kumra. (2022). “Learning Multi-Step Robotic Manipulation Tasks Through Visual Planning.” Rochester Institute of Technology.
Abstract: Multi-step manipulation tasks in unstructured environments are extremely challenging for a robot to learn. Such tasks interlace high-level reasoning that consists of the expected states that can be attained to achieve an overall task and low-level reasoning that decides what actions will yield these states. A model-free deep reinforcement learning method is proposed to learn multi-step manipulation tasks. This work introduces a novel Generative Residual Convolutional Neural Network (GR-ConvNet) model that can generate robust antipodal grasps from n-channel image input at real-time speeds (20ms). The proposed model architecture achieved a state-of-the-art accuracy on three standard grasping datasets. The adaptability of the proposed approach is demonstrated by directly transferring the trained model to a 7 DoF robotic manipulator with a grasp success rate of 95.4% and 93.0% on novel household and adversarial objects, respectively. A novel Robotic Manipulation Network (RoManNet) is introduced, which is a vision-based model architecture, to learn the action-value functions and predict manipulation action candidates. A Task Progress based Gaussian (TPG) reward function is defined to compute the reward based on actions that lead to successful motion primitives and progress towards the overall task goal. To balance the ratio of exploration/exploitation, this research introduces a Loss Adjusted Exploration (LAE) policy that determines actions from the action candidates according to the Boltzmann distribution of loss estimates. The effectiveness of the proposed approach is demonstrated by training RoManNet to learn several challenging multi-step robotic manipulation tasks in both simulation and real-world. Experimental results show that the proposed method outperforms the existing methods and achieves state-of-the-art performance in terms of success rate and action efficiency. The ablation studies show that TPG and LAE are especially beneficial for tasks like multiple block stacking.
Publisher Site: RIT Repository
PDF (Requires Sign-In): PDF
Click for Bibtex
@book{kumra2022learning, title = {Learning Multi-Step Robotic Manipulation Tasks Through Visual Planning}, author = {Kumra, Sulabh}, year = {2022}, school = {Rochester Institute of Technology} }
[D-2022-11] Bruno Artacho. (2022). “Multi-Scale Architectures for Human Pose Estimation.” Rochester Institute of Technology.
Abstract: In this dissertation we present multiple state-of-the-art deep learning methods for computer vision tasks using multi-scale approaches for two main tasks: pose estimation and semantic segmentation. For pose estimation, we introduce a complete framework expanding the fields-of-view of the network through a multi-scale approach, resulting in a significant increasing the effectiveness of conventional backbone architectures, for several pose estimation tasks without requiring a larger network or postprocessing. Our multi-scale pose estimation framework contributes to research on methods for single-person pose estimation in both 2D and 3D scenarios, pose estimation in videos, and the estimation of multiple people's pose in a single image for both top-down and bottom-up approaches. In addition to the enhanced capability of multi-person pose estimation generated by our multi-scale approach, our framework also demonstrates a superior capacity to expanded the more detailed and heavier task of full-body pose estimation, including up to 133 joints per person. For segmentation, we present a new efficient architecture for semantic segmentation, based on a "Waterfall" Atrous Spatial Pooling architecture, that achieves a considerable accuracy increase while decreasing the number of network parameters and memory footprint. The proposed Waterfall architecture leverages the efficiency of progressive filtering in the cascade architecture while maintaining multi-scale fields-of-view comparable to spatial pyramid configurations. Additionally, our method does not rely on a postprocessing stage with conditional random fields, which further reduces complexity and required training time.
Publisher Site: RIT Repository
PDF (Requires Sign-In): PDF
Click for Bibtex
@book{artacho2022multi-scale, title = {Multi-Scale Architectures for Human Pose Estimation}, author = {Artacho, Bruno}, year = {2022}, school = {Rochester Institute of Technology} }
[D-2022-10] Bruno Alves-Maciel. (2022). “Optimizing Vaccine Procurement for Affordability and Profits.” Rochester Institute of Technology.
Abstract: This thesis presents methods to increase savings in the global vaccine market without compromising its sustainability. Considering a hypothetically coordinated vaccine market (HCVM), where one or more coordinating entities are responsible for negotiating quantities and prices of vaccines on behalf of countries with different purchasing powers, this work explores four different and complementary issues related to market configurations impacting global affordability and profits: (1) the level of cooperation among coordinating entities; (2) optimal country assignment to coordinating entities; (3) benefits in procuring vaccines through tenders of formularies rather than purchasing doses individually; and (4) the value of a dollar saved in different countries. Additionally, these studies incorporate prescriptive and descriptive analytics with economic theory exploring the incentives that could bring the global vaccine market closer to the HCVM. The findings presented in this thesis contribute both to the literature and to the sustainability of the global vaccine market with specific recommendations to improve affordability for low-income countries with minimal impact to other market segments or to the vaccine producers.
Publisher Site: RIT Repository
PDF (Requires Sign-In): PDF
Click for Bibtex
@book{alves-maciel2022optimizing, title = {Optimizing Vaccine Procurement for Affordability and Profits}, author = {Alves-Maciel, Bruno}, year = {2022}, school = {Rochester Institute of Technology} }
[D-2022-09] Sanketh Satyanarayana Moudgalya. (2022). “Cochlear Compartments Segmentation and Pharmacokinetics Using Micro Computed Tomography Images.” Rochester Institute of Technology.
Abstract: Local drug delivery to the inner ear via micropump implants has the potential to be much more effective than oral drug delivery for treating patients with sensorineural hearing loss and to protect hearing from ototoxic insult due to noise exposure. Delivering appropriate concentrations of drugs to the necessary cochlear compartments is of paramount importance; however, directly measuring local drug concentrations over time throughout the cochlea is not possible. Indirect measurement using otoacoustic emissions and auditory brainstem response are ineffective as they only provide an estimate of concentration and are susceptible to non-linear sensitivity effects. Imaging modalities such as MRI with infused gadolinium contrast agent are limited due to the high spatial resolution requirement for pharmacokinetic analysis, especially in mice with cochlear length in the micron scale. We develop an intracochlear pharmacokinetic model using micro-computed tomography imaging of the cochlea during in vivo infusion of a contrast agent at the basal end of scala tympani through a cochleostomy. This approach requires accurately segmenting the main cochlear compartments: scala tympani (ST), scala media (SM) and scala vestibuli (SV). Each scan was segmented using 1) atlas-based deformable registration, and 2) V-Net, a encoder-decoder style convolutional neural network. The segmentation of these cochlear regions enable concentrations to be extracted along the length of each scala. These spatio-temporal concentration profiles are used to learn a concentration dependent diffusion coefficient, and transport parameters between the major scalae and to clearance. The pharmacokinetic model results are comparable to the current state of the art model, and can simulate concentrations for cases involving different infusion molecules and drug delivery protocols. While our model shows promising results, to extend the approach to larger animals and to generate accurate further experimental data, computational constraints, and time requirements of previous segmentation methods need to be mitigated. To this end, we extended the V-Net architecture with inclusion of spatial attention. Moreover, to enable segmentation in hardware restricted environments, we designed a 3D segmentation network using Capsule Networks that can provide improved segmentation performance along with 90% reduction in trainable parameters. Finally, to demonstrate the effectiveness of these networks, we test them on multiple public datasets. They are also tested on the cochlea dataset and pharmacokinetic model simulations will be validated against existing results.
Publisher Site: RIT Repository
PDF (Requires Sign-In): PDF
Click for Bibtex
@book{moudgalya2022cochlear, title = {Cochlear Compartments Segmentation and Pharmacokinetics Using Micro Computed Tomography Images}, author = {Moudgalya, Sanketh Satyanarayana}, year = {2022}, school = {Rochester Institute of Technology} }
[D-2022-08] Gordon Werner. (2022). “R-CAD: Rare Cyber Alert Signature Relationship Extraction Through Temporal Based Learning.” Rochester Institute of Technology.
Abstract: The large number of streaming intrusion alerts make it challenging for security analysts to quickly identify attack patterns. This is especially difficult since critical alerts often occur too rarely for traditional pattern mining algorithms to be effective. Recognizing the attack speed as an inherent indicator of differing cyber attacks, this work aggregates alerts into attack episodes that have distinct attack speeds, and finds attack actions regularly co-occurring within the same episode. This enables a novel use of the constrained SPADE temporal pattern mining algorithm to extract consistent co-occurrences of alert signatures that are indicative of attack actions that follow each other. The proposed Rare yet Co-occurring Attack action Discovery (R-CAD) system extracts not only the co-occurring patterns but also the temporal characteristics of the co-occurrences, giving the `strong rules' indicative of critical and repeated attack behaviors. Through the use of a real-world dataset, we demonstrate that R-CAD helps reduce the overwhelming volume and variety of intrusion alerts to a manageable set of co-occurring strong rules. We show specific rules that reveal how critical attack actions follow one another and in what attack speed.
Publisher Site: RIT Repository
PDF (Requires Sign-In): PDF
Click for Bibtex
@book{werner2022r-cad:, title = {R-CAD: Rare Cyber Alert Signature Relationship Extraction Through Temporal Based Learning}, author = {Werner, Gordon}, year = {2022}, school = {Rochester Institute of Technology} }
[D-2022-07] Weishi Shi. (2022). “Active Learning from Knowledge-Rich Data.” Rochester Institute of Technology.
Abstract: With the ever-increasing demand for the quality and quantity of the training samples, it is difficult to replicate the success of modern machine learning models in knowledge-rich domains, where the labeled data for training is scarce and labeling new data is expensive. While machine learning and AI have achieved significant progress in many common domains, the lack of large-scale labeled data samples poses a grand challenge for the wide application of advanced statistical learning models in key knowledge-rich domains, such as medicine, biology, physical science, and more. Active learning (AL) offers a promising and powerful learning paradigm that can significantly reduce the data-annotation stress by allowing the model to only sample the informative objects to learn from human experts. Previous AL models leverage simple criteria to explore the data space and achieve fast convergence of AL. However, those active sampling methods are less effective in exploring knowledge-rich data spaces and result in slow convergence of AL. In this thesis, we propose novel AL methods to address knowledge-rich data exploration challenges with respect to different types of machine learning tasks. Specifically, for multi-class tasks, we propose three approaches that leverage different types of sparse kernel machines to better capture the data covariance and use them to guide effective data exploration in a complex feature space. For multi-label tasks, it is essential to capture label correlations, and we model them in three different approaches to guide effective data exploration in a large and correlated label space. For data exploration in a very high-dimension feature space, we present novel uncertainty measures to better control the exploration behavior of deep learning models and leverage a uniquely designed regularizer to achieve effective exploration in high-dimension space. Our proposed models not only exhibit a good behavior of exploration for different types of knowledge-rich data but also manage to achieve an optimal exploration-exploitation balance with strong theoretical underpinnings. In the end, we study active learning in a more realistic scenario where human annotators provide noisy labels. We propose a re-sampling paradigm that leverages the machine's awareness to reduce the noise rate. We theoretically prove the effectiveness of the re-sampling paradigm and design a novel spatial-temporal active re-sampling function by leveraging the critical spatial and temporal properties of the maximum-margin kernel classifiers.
Publisher Site: RIT Repository
PDF (Requires Sign-In): PDF
Click for Bibtex
@book{shi2022active, title = {Active Learning from Knowledge-Rich Data}, author = {Shi, Weishi}, year = {2022}, school = {Rochester Institute of Technology} }
[D-2022-06] Peter Jackson. (2022). “On Uncertainty Propagation in Image-Guided Renal Navigation: Exploring Uncertainty Reduction Techniques through Simulation and In Vitro Phantom Evaluation.” Rochester Institute of Technology.
Abstract: Image-guided interventions (IGIs) entail the use of imaging to augment or replace direct vision during therapeutic interventions, with the overall goal is to provide effective treatment in a less invasive manner, as an alternative to traditional open surgery, while reducing patient trauma and shortening the recovery time post-procedure. IGIs rely on pre-operative images, surgical tracking and localization systems, and intra-operative images to provide correct views of the surgical scene. Pre-operative images are used to generate patient-specific anatomical models that are then registered to the patient using the surgical tracking system, and often complemented with real-time, intra-operative images. IGI systems are subject to uncertainty from several sources, including surgical instrument tracking / localization uncertainty, model-to-patient registration uncertainty, user-induced navigation uncertainty, as well as the uncertainty associated with the calibration of various surgical instruments and intra-operative imaging devices (i.e., laparoscopic camera) instrumented with surgical tracking sensors. All these uncertainties impact the overall targeting accuracy, which represents the error associated with the navigation of a surgical instrument to a specific target to be treated under image guidance provided by the IGI system. Therefore, understanding the overall uncertainty of an IGI system is paramount to the overall outcome of the intervention, as procedure success entails achieving certain accuracy tolerances specific to individual procedures. This work has focused on studying the navigation uncertainty, along with techniques to reduce uncertainty, for an IGI platform dedicated to image-guided renal interventions. We constructed life-size replica patient-specific kidney models from pre-operative images using 3D printing and tissue emulating materials and conducted experiments to characterize the uncertainty of both optical and electromagnetic surgical tracking systems, the uncertainty associated with the virtual model-to-physical phantom registration, as well as the uncertainty associated with live augmented reality (AR) views of the surgical scene achieved by enhancing the pre-procedural model and tracked surgical instrument views with live video views acquires using a camera tracked in real time. To better understand the effects of the tracked instrument calibration, registration fiducial configuration, and tracked camera calibration on the overall navigation uncertainty, we conducted Monte Carlo simulations that enabled us to identify optimal configurations that were subsequently validated experimentally using patient-specific phantoms in the laboratory. To mitigate the inherent accuracy limitations associated with the pre-procedural model-to-patient registration and their effect on the overall navigation, we also demonstrated the use of tracked video imaging to update the registration, enabling us to restore targeting accuracy to within its acceptable range. Lastly, we conducted several validation experiments using patient-specific kidney emulating phantoms using post-procedure CT imaging as reference ground truth to assess the accuracy of AR-guided navigation in the context of in vitro renal interventions. This work helped find answers to key questions about uncertainty propagation in image-guided renal interventions and led to the development of key techniques and tools to help reduce optimize the overall navigation / targeting uncertainty.
Publisher Site: RIT Repository
PDF (Requires Sign-In): PDF
Click for Bibtex
@book{jackson2022on, title = {On Uncertainty Propagation in Image-Guided Renal Navigation: Exploring Uncertainty Reduction Techniques through Simulation and In Vitro Phantom Evaluation}, author = {Jackson, Peter}, year = {2022}, school = {Rochester Institute of Technology} }
[D-2022-05] Teresa Symons. (2022). “The View from 50 AU: Measuring the Cosmic Optical Background with New Horizons.” Rochester Institute of Technology.
Abstract: The extragalactic background light (EBL) is the sum of the light emitted by sources beyond the Milky Way throughout the history of the universe. While the EBL is present at all wavelengths, at optical wavelengths it is largely sourced by the light from stars and galaxies and is referred to as the cosmic optical background (COB). Direct photometric measurements of the COB provide an important comparison to population models of galaxy formation and evolution. Such measurements therefore provide a cosmic consistency test with the potential to reveal additional diffuse sources of emission. However, the COB has been difficult to measure from Earth due to the difficulty of isolating it from the diffuse light scattered from interplanetary dust in our Solar System, Zodiacal Light (ZL). In this dissertation, I present a pipeline to measure the COB using data taken by the Long-Range Reconnaissance Imager (LORRI) on NASA's New Horizons mission. Because of New Horizons' location in the outer Solar System, the ZL is negligible compared to measurements taken from 1 AU. To measure the COB, I characterize and remove structured and diffuse astrophysical foregrounds including bright stars, the integrated starlight from faint unresolved sources, and diffuse galactic light. Dark current and other instrument systematics are also accounted for, including various sources of scattered light. I present a measurement of the COB derived from a set of LORRI images encompassing New Horizons' journey from the inner Solar System to Pluto and beyond. I discuss sources of systematic error, including astrophysical, calibration, and instrumental sources affecting each foreground component, and put this measurement in the context of other estimates of the COB and EBL.
Publisher Site: RIT Repository
PDF (Requires Sign-In): PDF
Click for Bibtex
@book{symons2022the, title = {The View from 50 AU: Measuring the Cosmic Optical Background with New Horizons}, author = {Symons, Teresa}, year = {2022}, school = {Rochester Institute of Technology} }
[D-2022-04] Celal Savur. (2022). “A Physiological Computing System to Improve Human-Robot Collaboration by Using Human Comfort Index.” Rochester Institute of Technology.
Abstract: Fluent human-robot collaboration requires a robot teammate to understand, learn, and adapt to the human's psycho-physiological state. Such collaborations require a physiological computing system that monitors human biological signals during human-robot collaboration (HRC) to quantitatively estimate a human's level of comfort, which we have termed in this research as comfortability index (CI) and uncomfortability index (UnCI). We proposed a human comfort index estimation system (CIES) that uses biological signals and subjective metrics. Subjective metrics (surprise, anxiety, boredom, calmness, and comfortability) and physiological signals were collected during a human-robot collaboration experiment that varied the robot's behavior. The emotion circumplex model is adapted to calculate the CI from the participant's quantitative data as well as physiological data. This thesis developed a physiological computing system that estimates human comfort levels from physiological by using the circumplex model approach. The data was collected from multiple experiments and machine learning models trained, and their performance was evaluated. As a result, a subject-independent model was tested to determine the robot behavior based on human comfort level. The results from multiple experiments indicate that the proposed CIES model improves human comfort by providing feedback to the robot. In conclusion, physiological signals can be used for personalized robots, and it has the potential to improve safety for humans and increase the fluency of collaboration.
Publisher Site: RIT Repository
PDF (Requires Sign-In): PDF
Click for Bibtex
@book{savur2022a, title = {A Physiological Computing System to Improve Human-Robot Collaboration by Using Human Comfort Index}, author = {Savur, Celal}, year = {2022}, school = {Rochester Institute of Technology} }
[D-2022-03] Julia Rae D’Rozario. (2022). “Light Management in III-V Thin-Film Photovoltaics and Micro-LEDs.” Rochester Institute of Technology.
Abstract: Light management is essential to improve the performance of optoelectronic devices as they depend on the interaction between photons and device design. This research demonstrates novel approaches to enhance the light absorption in thin-film III-V photovoltaics (PV) and light emission from micrometer-scale light-emitting diodes (μLED). The high power conversion efficiency (PCE) realized in III-V PV makes them attractive power generation sources, especially for off-the-grid space-related missions. Thin-film PV (
Publisher Site: RIT Repository
PDF (Requires Sign-In): PDF
Click for Bibtex
@book{d'rozario2022light, title = {Light Management in III-V Thin-Film Photovoltaics and Micro-LEDs}, author = {D'Rozario, Julia Rae}, year = {2022}, school = {Rochester Institute of Technology} }
[D-2022-02] Mayur Dhanaraj. (2022). “Dynamic Algorithms and Asymptotic Theory for Lp-Norm Data Analysis.” Rochester Institute of Technology.
Abstract: The focus of this dissertation is the development of outlier-resistant stochastic algorithms for Principal Component Analysis (PCA) and the derivation of novel asymptotic theory for Lp-norm Principal Component Analysis (Lp-PCA). Modern machine learning and signal processing applications employ sensors that collect large volumes of data measurements that are stored in the form of data matrices, that are often massive and need to be efficiently processed in order to enable machine learning algorithms to perform effective underlying pattern discovery. One such commonly used matrix analysis technique is PCA. Over the past century, PCA has been extensively used in areas such as machine learning, deep learning, pattern recognition, and computer vision, just to name a few. PCA's popularity can be attributed to its intuitive formulation on the L2-norm, availability of an elegant solution via the singular-value-decomposition (SVD), and asymptotic convergence guarantees. However, PCA has been shown to be highly sensitive to faulty measurements (outliers) because of its reliance on the outlier-sensitive L2-norm. Arguably, the most straightforward approach to impart robustness against outliers is to replace the outlier-sensitive L2-norm by the outlier-resistant L1-norm, thus formulating what is known as L1-PCA. Exact and approximate solvers are proposed for L1-PCA in the literature. On the other hand, in this big-data era, the data matrix may be very large and/or the data measurements may arrive in streaming fashion. Traditional L1-PCA algorithms are not suitable in this setting. In order to efficiently process streaming data, while being resistant against outliers, we propose a stochastic L1-PCA algorithm that computes the dominant principal component (PC) with formal convergence guarantees. We further generalize our stochastic L1-PCA algorithm to find multiple components by propose a new PCA framework that maximizes the recently proposed Barron loss. Leveraging Barron loss yields a stochastic algorithm with a tunable robustness parameter that allows the user to control the amount of outlier-resistance required in a given application. We demonstrate the efficacy and robustness of our stochastic algorithms on synthetic and real-world datasets. Our experimental studies include online subspace estimation, classification, video surveillance, and image conditioning, among other things. Last, we focus on the development of asymptotic theory for Lp-PCA. In general, Lp-PCA for pHowever, unlike PCA, Lp-PCA is perceived as a ``robust heuristic'' by the research community due to the lack of theoretical asymptotic convergence guarantees. In this work, we strive to shed light on the topic by developing asymptotic theory for Lp-PCA. Specifically, we show that, for a broad class of data distributions, the Lp-PCs span the same subspace as the standard PCs asymptotically and moreover, we prove that the Lp-PCs are specific rotated versions of the PCs. Finally, we demonstrate the asymptotic equivalence of PCA and Lp-PCA with a wide variety of experimental studies.
Publisher Site: RIT Repository
PDF (Requires Sign-In): PDF
Click for Bibtex
@book{dhanaraj2022dynamic, title = {Dynamic Algorithms and Asymptotic Theory for Lp-Norm Data Analysis}, author = {Dhanaraj, Mayur}, year = {2022}, school = {Rochester Institute of Technology} }
[D-2022-01] Aneesh Prasad Rangnekar. (2022). “Learning Representations in the Hyperspectral Domain in Aerial Imagery.” Rochester Institute of Technology.
Abstract: We establish two new datasets with baselines and network architectures for the task of hyperspectral image analysis. The first dataset, AeroRIT, is a moving camera static scene captured from a flight and contains per pixel labeling across five categories for the task of semantic segmentation. The second dataset, RooftopHSI, helps design and interpret learnt features on hyperspectral object detection on scenes captured from an university rooftop. This dataset accounts for static camera, moving scene hyperspectral imagery. We further broaden the scope of our understanding of neural networks with the development of two novel algorithms - S4AL and S4AL+. We develop these frameworks on natural (color) imagery, by combining semi-supervised learning and active learning, and display promising results for learning with limited amount of labeled data, which can be extended to hyperspectral imagery. In this dissertation, we curated two new datasets for hyperspectral image analysis, significantly larger than existing datasets and broader in terms of categories for classification. We then adapt existing neural network architectures to function on the increased channel information, in a smart manner, to leverage all hyperspectral information. We also develop novel active learning algorithms on natural (color) imagery, and discuss the hope for expanding their functionality to hyperspectral imagery.
Publisher Site: RIT Repository
PDF (Requires Sign-In): PDF
Click for Bibtex
@book{rangnekar2022learning, title = {Learning Representations in the Hyperspectral Domain in Aerial Imagery}, author = {Rangnekar, Aneesh Prasad}, year = {2022}, school = {Rochester Institute of Technology} }
[D-2021-17] Eloy Yague-Spaude. (2021). “Computational Design, Simulation of Meshing, and Stress Analysis of Strain Wave Gear Drives.” Rochester Institute of Technology.
Abstract: Strain wave gear (SWG) drives were patented in 1959 by C. Walton Musser as a coaxial, compact, and lightweight gear drive providing remarkably large gear ratios without backlash. This outstanding performance requires the use of a flexible gear, as well as a meshing process with two regions of tooth contact as opposed to traditional gear drives, which only mesh in a single region. The latter drives have been studied for centuries under the principles of solid mechanics whereas SWG drives lack strong bodies of literature, principles, and computational tools for their design. SWG drives include three parts: wave generator, flexible spline, and ring gear. Typically, the wave generator is an elliptical cam surrounded by a flexible race ball bearing which, inserted inside the flexible spline, provides the input motion to the drive. The flexible spline is a cup-shaped spring with external teeth on the open-end which, deflected by the wave generator, mesh with the internal teeth of the ring gear in two regions along the major axis of the drive. This thesis dissertation focuses on understanding the influence of the geometries of the wave generator and tooth profiles of the flexible spline and the ring gear over stresses throughout the operation of SWG drives. In doing so, computational tools have been developed and design recommendations have been formulated. The wave generator geometries simplified, elliptical, and four roller have been implemented based on previous geometries, while an additional geometry called parabolic is newly proposed. For the tooth profiles, the involute, as a generated profile, and the double and quadruple circular arc geometries, as directly-defined profiles, have been implemented. Two- and three-dimensional finite element models have been developed in a custom-made software to generate fully parameterized models based on the design and manufacturing processes of SWG drives. The models are analyzed and the resulting stresses for each design are compared to determine which geometries and micro-geometry modifications are the most influential over the mechanical performance of this type of gear drive. Significant improvement has been achieved by modifying the geometries of the wave generator and the tooth flanks of the flexible spline and the ring gear. Simplified and parabolic wave generator geometries proved similarly advantageous and the elliptical geometry resulted in the lowest compressive stresses, while the four roller geometry was discarded due to large stresses. The involute tooth profile was proven unsuitable while the directly-defined tooth profiles showed similarly beneficial outcomes when the root geometry was reinforced. The three-dimensional model evidenced the complex state of deflection of the flexible spline due to the cup-shaped spring and the need for micro-geometry modifications to further improve the behavior of SWG drives. Crowning and slope micro-geometry modifications on the wave generator and crowning on the tooth flanks of the flexible spline have been implemented. When combined, these modifications eliminated the areas of stress concentration due to the deflection of the flexible spline and allowed the contact pattern to move closer to the center of the teeth. These improvements resulted in remarkably lower stresses which serve to increase the overall mechanical performance of SWG drives.
Publisher Site: RIT Repository
PDF (Requires Sign-In): PDF
Click for Bibtex
@book{yague-spaude2021computational, title = {Computational Design, Simulation of Meshing, and Stress Analysis of Strain Wave Gear Drives}, author = {Yague-Spaude, Eloy}, year = {2021}, school = {Rochester Institute of Technology} }
[D-2021-16] M. Meraj Ahmed. (2021). “Architecting a One-to-Many Traffic-Aware and Secure Millimeter-Wave Wireless Network-in-Package Interconnect for Multichip Systems.” Rochester Institute of Technology.
Abstract: With the aggressive scaling of device geometries, the yield of complex Multi Core Single Chip(MCSC) systems with many cores will decrease due to the higher probability of manufacturing defects especially, in dies with a large area. Disintegration of large System-on-Chips(SoCs) into smaller chips called chiplets has shown to improve the yield and cost of complex systems. Therefore, platform-based computing modules such as embedded systems and micro-servers have already adopted Multi Core Multi Chip (MCMC) architectures overMCSC architectures. Due to the scaling of memory intensive parallel applications in such systems, data is more likely to be shared among various cores residing in different chips resulting in a significant increase in chip-to-chip traffic, especially one-to-many traffic. This one-to-many traffic is originated mainly to maintain cache-coherence between many cores residing in multiple chips. Besides, one-to-many traffics are also exploited by many parallel programming models, system-level synchronization mechanisms, and control signals. How-ever, state-of-the-art Network-on-Chip (NoC)-based wired interconnection architectures do not provide enough support as they handle such one-to-many traffic as multiple unicast trafficusing a multi-hop MCMC communication fabric. As a result, even a small portion of such one-to-many traffic can significantly reduce system performance as traditional NoC-basedinterconnect cannot mask the high latency and energy consumption caused by chip-to-chipwired I/Os. Moreover, with the increase in memory intensive applications and scaling of MCMC systems, traditional NoC-based wired interconnects fail to provide a scalable inter-connection solution required to support the increased cache-coherence and synchronization generated one-to-many traffic in future MCMC-based High-Performance Computing (HPC) nodes. Therefore, these computation and memory intensive MCMC systems need an energy-efficient, low latency, and scalable one-to-many (broadcast/multicast) traffic-aware interconnection infrastructure to ensure high-performance. Research in recent years has shown that Wireless Network-in-Package (WiNiP) architectures with CMOS compatible Millimeter-Wave (mm-wave) transceivers can provide a scalable, low latency, and energy-efficient interconnect solution for on and off-chip communication. In this dissertation, a one-to-many traffic-aware WiNiP interconnection architecture with a starvation-free hybrid Medium Access Control (MAC), an asymmetric topology, and a novel flow control has been proposed. The different components of the proposed architecture are individually one-to-many traffic-aware and as a system, they collaborate with each other to provide required support for one-to-many traffic communication in a MCMC environment. It has been shown that such interconnection architecture can reduce energy consumption and average packet latency by 46.96% and 47.08% respectively for MCMC systems. Despite providing performance enhancements, wireless channel, being an unguided medium, is vulnerable to various security attacks such as jamming induced Denial-of-Service (DoS), eavesdropping, and spoofing. Further, to minimize the time-to-market and design costs, modern SoCs often use Third Party IPs (3PIPs) from untrusted organizations. An adversary either at the foundry or at the 3PIP design house can introduce a malicious circuitry, to jeopardize an SoC. Such malicious circuitry is known as a Hardware Trojan (HT). An HTplanted in the WiNiP from a vulnerable design or manufacturing process can compromise a Wireless Interface (WI) to enable illegitimate transmission through the infected WI resulting in a potential DoS attack for other WIs in the MCMC system. Moreover, HTs can be used for various other malicious purposes, including battery exhaustion, functionality subversion, and information leakage. This information when leaked to a malicious external attackercan reveals important information regarding the application suites running on the system, thereby compromising the user profile. To address persistent jamming-based DoS attack in WiNiP, in this dissertation, a secure WiNiP interconnection architecture for MCMC systems has been proposed that re-uses the one-to-many traffic-aware MAC and existing Design for Testability (DFT) hardware along with Machine Learning (ML) approach. Furthermore, a novel Simulated Annealing (SA)-based routing obfuscation mechanism was also proposed toprotect against an HT-assisted novel traffic analysis attack. Simulation results show that,the ML classifiers can achieve an accuracy of 99.87% for DoS attack detection while SA-basedrouting obfuscation could reduce application detection accuracy to only 15% for HT-assistedtraffic analysis attack and hence, secure the WiNiP fabric from age-old and emerging attacks.
Publisher Site: RIT Repository
PDF (Requires Sign-In): PDF
Click for Bibtex
@book{ahmed2021architecting, title = {Architecting a One-to-Many Traffic-Aware and Secure Millimeter-Wave Wireless Network-in-Package Interconnect for Multichip Systems}, author = {Ahmed, M. Meraj}, year = {2021}, school = {Rochester Institute of Technology} }
[D-2021-15] Dimitris G. Chachlakis. (2021). “Theory and Algorithms for Reliable Multimodal Data Analysis, Machine Learning, and Signal Processing.” Rochester Institute of Technology.
Abstract: Modern engineering systems collect large volumes of data measurements across diverse sensing modalities. These measurements can naturally be arranged in higher-order arrays of scalars which are commonly referred to as tensors. Tucker decomposition (TD) is a standard method for tensor analysis with applications in diverse fields of science and engineering. Despite its success, TD exhibits severe sensitivity against outliers —i.e., heavily corrupted entries that appear sporadically in modern datasets. We study L1-norm TD (L1-TD), a reformulation of TD that promotes robustness. For 3-way tensors, we show, for the first time, that L1-TD admits an exact solution via combinatorial optimization and present algorithms for its solution. We propose two novel algorithmic frameworks for approximating the exact solution to L1-TD, for general N-way tensors. We propose a novel algorithm for dynamic L1-TD —i.e., efficient and joint analysis of streaming tensors. Principal-Component Analysis (PCA) (a special case of TD) is also outlier responsive. We consider Lp-quasinorm PCA (Lp-PCA) for p
Publisher Site: RIT Repository
PDF (Requires Sign-In): PDF
Click for Bibtex
@book{chachlakis2021theory, title = {Theory and Algorithms for Reliable Multimodal Data Analysis, Machine Learning, and Signal Processing}, author = {Chachlakis, Dimitris G.}, year = {2021}, school = {Rochester Institute of Technology} }
[D-2021-14] Nicole Hill. (2021). “Translating Macroscale Concepts to Microfluidic Devices.” Rochester Institute of Technology.
Abstract: Electrokinetics represents an extremely versatile family of techniques that can be used to manipulate particles and fluid in microfluidic devices. This dissertation focused on taking techniques commonly used on a macroscale and developing microscale equivalents utilizing electrokinetics to effectively manipulate and separate microparticles. This analysis focuses on chromatography and separation trains as macroscale techniques translated to microfluidic insulator-based electrokinetic devices. The geometries of insulating post arrays embedded in microchannels were optimized using a combination of mathematical simulations and experimentally derived correction factors. Two particle separations were experimentally demonstrated: a separation based on differences in particle size and a separation based on differences in particle charge. The introduction of nonlinear electrophoresis into the electrokinetics paradigm prompted the creation of an empirical electrokinetic equilibrium condition, an experimentally derived, geometry independent value unique to different particles. This term takes into account particle-particle interactions and the presence of an electric field gradient to help simulate the impact of nonlinear electrophoresis on particle motion and provide an estimate trapping voltages for particles using similar suspending media. Finally, a cascade device design was presented as a type of separation train, built to filter larger contaminants from complex particle suspensions. Sample purification for a scheme involving manual device transferring of sample versus the cascade device, which required no manual transfer, demonstrated a notably lower sample loss in the cascade device. Bacteriophages were effectively enriched using the cascade scheme, demonstrating the potential use of this technique for purifying valuable biological samples.
Publisher Site: RIT Repository
PDF (Requires Sign-In): PDF
Click for Bibtex
@book{hill2021translating, title = {Translating Macroscale Concepts to Microfluidic Devices}, author = {Hill, Nicole}, year = {2021}, school = {Rochester Institute of Technology} }
[D-2021-13] Aniket Mohan Rishi. (2021). “Development of Highly Functional, Surface Tunable, and Efficient Composite Coatings for Pool Boiling Heat Transfer Enhancement.” Rochester Institute of Technology.
Abstract: Rapid growth and advancements in high-power electronic devices, IC chips, electric vehicles, and lithium-ion batteries have compelled the development of efficient and novel thermal management solutions. Currently used air and liquid cooling systems are unable to remove the heat efficiently due to significant pressure drops, temperature differences, and limited heat-carrying capacities. In contrast, phase-change cooling techniques can remove the larger amount of heat with higher efficiency while maintaining safer operational temperature ranges. Pool boiling heat transfer is a type of phase-change cooling technique in which vapor bubbles generated on the boiling surface carry away the heat. This pool boiling performance is limited by the maximum heat dissipation capacity, quantified by the Critical Heat Flux (CHF), and efficiency of the boiling surface, quantified by the Heat Transfer Coefficient (HTC). This work emphasizes on improving both CHF and HTC by developing highly surface functional and tunable microporous coatings using sintering and electrodeposition techniques. Initially, graphene nanoplatelets/copper (GNP/Cu)-based composite coatings were developed using a multi-step electrodeposition technique. And 2% GNP/Cu coating rendered the highest reported CHF of 286 W/cm² and HTC of 204 kW/m²-°C with increased bond strength. To further enhance the cohesive and adhesive bond strength of the electrodeposited coatings, a novel multi-step electrodeposition technique was developed and tested on copper-based coatings. This technique dramatically improved the overall functionality, pool boiling performance, and durability of the coatings. Later, a sintering technique was used to develop the coatings using GNP and copper particles. Uniform spreading of GNP over the coatings was obtained via ball milling technique. This technique yielded a CHF of 239 W/cm² and the HTC of 285 kW/m²-°C (~91% and ~438% higher than a plain copper surface, respectively). A novel approach of salt-templated sintering was developed in the final part to attain a better control on porosity and wicking properties of the sintered coatings. This generated interconnected porous networks with a higher nucleating activity, and attained record-breaking CHF of 289 W/cm² and the HTC of 1,314 kW/m²-°C.
Publisher Site: RIT Repository
PDF (Requires Sign-In): PDF
Click for Bibtex
@book{rishi2021development, title = {Development of Highly Functional, Surface Tunable, and Efficient Composite Coatings for Pool Boiling Heat Transfer Enhancement}, author = {Rishi, Aniket Mohan}, year = {2021}, school = {Rochester Institute of Technology} }
[D-2021-12] Michael P. Medlar. (2021). “An Enhanced Statistical Phonon Transport Model for Nanoscale Thermal Transport and Design.” Rochester Institute of Technology.
Abstract: Managing thermal energy generation and transfer within the nanoscale devices (transistors) of modern microelectronics is important as it limits speed, carrier mobility, and affects reliability. Application of Fourier's Law of Heat Conduction to the small length and times scales associated with transistor geometries and switching frequencies doesn't give accurate results due to the breakdown of the continuum assumption and the assumption of local thermodynamic equilibrium. Heat conduction at these length and time scales occurs via phonon transport, including both classical and quantum effects. Traditional methods for phonon transport modeling are lacking in the combination of computational efficiency, physical accuracy, and flexibility. The Statistical Phonon Transport Model (SPTM) is an engineering design tool for predicting non-equilibrium phonon transport. The goal of this work has been to enhance the models and computational algorithms of the SPTM to elevate it to have a high combination of accuracy and flexibility. Four physical models of the SPTM were enhanced. The lattice dynamics calculation of phonon dispersion relations was extended to use first and second nearest neighbor interactions, based on published interatomic force constants computed with first principles Density Functional Theory (DFT). The computation of three phonon scattering partners (that explicitly conserve energy and momentum) with the inclusion of the three optical phonon branches was applied using scattering rates computed from Fermi's Golden Rule. The prediction of phonon drift was extended to three dimensions within the framework of the previously established methods of the SPTM. Joule heating as a result of electron-phonon scattering in nanoscale electronic devices was represented using a modal specific phonon source that can be varied in space and time. Results indicate the use of first and second nearest neighbor lattice dynamics better predicted dispersion when compared to experimental results and resulted in a higher fidelity representation of phonon group velocities and three phonon scattering partners in an anisotropic manner. Three phonon scattering improvements resulted in enhanced fidelity in the prediction of phonon modal decay rates across the wavevector space and thus better representation of non-equilibrium behavior. Comparisons to the range of phonon transport modeling approaches from literature verify that the SPTM has higher phonon fidelity than Boltzmann Transport Equation and Monte Carlo and higher length scale and time scale fidelity than Direct Atomic Simulation. Additional application of the SPTM to both a 1-d silicon nanowire transistor and a 3-d FinFET array transistor in a transient manner illustrate the design capabilities. Thus, the SPTM has been elevated to fill the gap between lower phonon fidelity Monte Carol (MC) models and high fidelity, inflexible direct quantum simulations (or Direct Atomic Simulations (DAS)) within the field of phonon transport modeling for nanoscale electronic devices. The SPTM has produced high fidelity device level non-equilibrium phonon information in a 3-d, transient manner where Joule heating occurs. This information is required due to the fact that effective lattice temperatures are not adequate to describe the local thermal conditions. Knowledge of local phonon distributions, which can't be determined from application of Fourier's law, is important because of effects on electron mobility, device speed, leakage, and reliability.
Publisher Site: RIT Repository
PDF (Requires Sign-In): PDF
Click for Bibtex
@book{medlar2021an, title = {An Enhanced Statistical Phonon Transport Model for Nanoscale Thermal Transport and Design}, author = {Medlar, Michael P.}, year = {2021}, school = {Rochester Institute of Technology} }
[D-2021-11] Ekta Arjunkumar Shah. (2021). “The Effect of Galaxy Interactions on Star Formation and AGN Activity at 0.5 < z < 3.0.” Rochester Institute of Technology.
Abstract: Galaxy interactions and mergers play an important role in the hierarchical formation and evolution of galaxies. Studies in the nearby universe show a higher star formation rate (SFR) and active galactic nuclei (AGN) fraction in interacting and merging galaxies than in their isolated counterparts, indicating that such interactions are important contributors to star formation and black hole growth. A large fraction of massive galaxies is thought to be affected by galaxy mergers at high redshifts because the galaxy merger rate increases with redshift. We use deep observations and cosmological simulations to study the role of galaxy mergers and interactions in enhancing SFR and AGN activity in galaxies at $0.5
Publisher Site: RIT Repository
PDF (Requires Sign-In): PDF
Click for Bibtex
@book{shah2021the, title = {The Effect of Galaxy Interactions on Star Formation and AGN Activity at 0.5 < z < 3.0}, author = {Shah, Ekta Arjunkumar}, year = {2021}, school = {Rochester Institute of Technology} }
[D-2021-10] Manoj Meda. (2021). “Direct Writing of Printed Electronics through Molten Metal Jetting.” Rochester Institute of Technology.
Abstract: This research proposes a novel approach to printed electronics manufacturing via molten metal droplet jetting (MMJ). Large scale experimental work is presented to establish suitable jetting parameters for fabrication of high quality conductive electronic traces. Following process optimization, resistivity values as low as 4.49 µΩ-cm for printed 4043 aluminum alloy traces have achieved. This essentially matches the electrical resistivity of the bulk alloy, and it represents a significant improvement over results obtained with printed nanoparticle ink electronics. Electrical resistivity of printed traces that undergo static and cyclical flexing is included in the analysis. The cross-sectional area of traces printed with this approach is orders of magnitude larger than those achieved with nanoparticle inks, hence the traces essentially behave like solid-core metal wire capable of carrying very high currents. The relationship between jetting parameters and the equivalent wire gauge of the uniform printed traces is presented. Early experimental trials revealed the formation of large pinholes inside droplets deposited onto room temperature polyimide substrates. This was hypothesized to be due to the release of adsorbed moisture from the polyimide into the solidifying droplet. Subsequent experiments revealed that heating the polyimide substrate drives off the moisture and eliminates moisture-induced porosity. A multi-physics Ansys process model is also presented to understand behavior of molten metal droplets as they impinge upon a temperature sensitive polymer substrate, spread out, cool down, and solidify. The process model allows the study of many process conditions without expensive and time-consuming physical experimentation.
Publisher Site: RIT Repository
PDF (Requires Sign-In): PDF
Click for Bibtex
@book{meda2021direct, title = {Direct Writing of Printed Electronics through Molten Metal Jetting}, author = {Meda, Manoj}, year = {2021}, school = {Rochester Institute of Technology} }
[D-2021-09] Danielle Nicole Gonzalez. (2021). “The State of Practice for Security Unit Testing: Towards Data Driven Strategies to Shift Security into Developer’s Automated Testing Workflows.” Rochester Institute of Technology.
Abstract: The pressing need to "shift security left" in the software development lifecycle has motivated efforts to adapt the iterative and continuous process models used in practice today. Security unit testing is praised by practitioners and recommended by expert groups, usually in the context of DevSecOps and achieving "continuous security". In addition to vulnerability testing and standards adherence, this technique can help developers verify that security controls are implemented correctly, i.e. functional security testing. Further, the means by which security unit testing can be integrated into developer workflows is unique from other standalone tools as it is an adaptation of practices and infrastructure developers are already familiar with. Yet, software engineering researchers have so far failed to include this technique in their empirical studies on secure development and little is known about the state of practice for security unit testing. This dissertation is motivated by the disconnect between promotion of security unit testing and the lack of empirical evidence on how it is and can be applied. The goal of this work was to address the disconnect towards identifying actionable strategies to promote wider adoption and mitigate observed challenges. Three mixed-method empirical studies were conducted wherein practitioner-authored unit test code, Q&A posts, and grey literature were analyzed through three lenses: Practices (what they do), Perspectives and Guidelines (what and how they think it should be done), and Pain Points (what challenges they face) to incorporate both technical and human factors of this phenomena. Accordingly, this work contributes novel and important insights into how developers write functional unit tests for at least nine security controls, including a taxonomy of 53 authentication unit test cases derived from real code and a detailed analysis of seven unique pain points that developers seek help with from peers on Q&A sites. Recommendations given herein for conducting and adopting security unit testing, including mitigating challenges and addressing gaps between available and needed support, are grounded in the guidelines and perspectives on the benefits, limitations, use cases, and integration strategies shared in grey literature authored by practitioners.
Publisher Site: RIT Repository
PDF (Requires Sign-In): PDF
Click for Bibtex
@book{gonzalez2021the, title = {The State of Practice for Security Unit Testing: Towards Data Driven Strategies to Shift Security into Developer's Automated Testing Workflows}, author = {Gonzalez, Danielle Nicole}, year = {2021}, school = {Rochester Institute of Technology} }
[D-2021-08] Aranya Chauhan. (2021). “High Heat Flux Dissipation Using Innovative Dual Tapered Manifold in Pool Boiling, and Thermosiphon Loop for CPU Cooling in Data Centers.” Rochester Institute of Technology.
Abstract: The current trend of electronics miniaturization presents thermal challenges which limit the performance of processors. The high heat fluxes in CPUs are affecting the reliability and processing ability of the servers. Due to these thermal limitations, large amount of energy is required to cool the servers in data centers. The advanced two-phase boiling heat transfer systems are significantly more efficient than currently used single phase coolers. In the current work, a novel approach is adopted by using a dual tapered microgap over the heated boiling surface for enhanced heat transfer. The theoretical work has identified the role of two-phase pressure recovery effect induced by the expanding bubbles in the tapered microgap configuration that leads to a self-sustained flow over the heater surface. This effectively transforms the pool boiling into a pumpless flow boiling system. Additionally, the tapered microgap introduces a bubble squeezing effect that pushes the liquid along the expanding taper direction. High fluid velocities are generated through this mechanism thus creating the pumpless flow boiling process in a conventional pool boiling system. Using water as the working fluid, a critical heat flux (CHF) of 288 W/cm2 was achieved at a wall superheat of 24.1°C. The baseline configuration without any tapered manifold resulted in a CHF of 124 W/cm2 at a wall superheat of 23.8°C. This represents the largest enhancement ever reported for water on a plain surface without incorporating any surface modifications during pool boiling. For dielectric liquid as the working fluid, the dual tapered micogap obtained ~2X enhancement in the heat transfer coefficient (HTC) compared to the configuration with the baseline configuration. This dual tapered microgap design is also implemented in a thermosiphon loop for CPU cooling where no pumping power is required for fluid circulation in a closed loop. The loop contains an evaporator with a dual tapered microgap and was able to dissipate heat from an actual CPU (i7-930 processor, TDP 130W) more efficiently as compared to air, or water-based coolers during thermal stress tests. This dual taper evaporator configuration will be able to mitigate the hotspots generated on the CPU surface and improve the efficiency and mechanical integrity of the processor. Such boiling heat transfer systems can significantly reduce the cooling water temperature requirement thereby reducing the operational cost of data centers.
Publisher Site: RIT Repository
PDF (Requires Sign-In): PDF
Click for Bibtex
@book{chauhan2021high, title = {High Heat Flux Dissipation Using Innovative Dual Tapered Manifold in Pool Boiling, and Thermosiphon Loop for CPU Cooling in Data Centers}, author = {Chauhan, Aranya}, year = {2021}, school = {Rochester Institute of Technology} }
[D-2021-07] Collin Taylor Burkhart. (2021). “Coplanar Electrowetting-Induced Droplet Ejection for 3D Digital Microfluidic Devices.” Rochester Institute of Technology.
Abstract: Digital microfluidics is a promising fluid processing technology used in lab-on-achip applications to perform chemical synthesis, particle filtration, immunoassays, and other biological protocols. Traditional digital microfluidic (DMF) devices consist of a 2D grid of coated electrodes over which droplets are manipulated. Selective activation of the electrodes results in an electrowetting effect that deforms the droplets and can move them around the electrode grid. More recently, electrowetting on dielectric has also been used to eject droplets and transfer them between opposing surfaces. This has given rise to new 3D DMF devices capable of more sophisticated routing patterns that can minimize crosscontamination between different biological reagents used during operation. A better understanding of electrowetting-induced droplet ejection is critical for the future development of efficient 3D DMF devices. The focus of this work was to better predict electrowetting-induced droplet ejection and to determine how droplet selection and electrode design influence the process. An improved model of droplet gravitational potential and interfacial energy throughout ejection was developed that predicts a critical electrowetting number necessary for successful detachment. Predictions using the new model agreed more closely with experimentally observed thresholds than previous models, especially for larger droplet volumes. Droplet ejection experiments were also performed with a variety of coplanar electrode designs featuring different numbers of electrode pieces and different spacings between features. The critical voltage for ejection was observed to be approximately the same for all designs, despite the poor predicted performance for the case with the widest spacing (200 𝜇𝑚) where nearly 25% of the area beneath the droplet was dead space. Findings indicated that a critical electrowetting for ejection must be achieved at the contact line of a droplet rather than over the entire droplet region. Droplets were also ejected for the first time from devices with inkjet-printed electrodes, demonstrating the feasibility of future low-cost 3D DMF systems.
Publisher Site: RIT Repository
PDF (Requires Sign-In): PDF
Click for Bibtex
@book{burkhart2021coplanar, title = {Coplanar Electrowetting-Induced Droplet Ejection for 3D Digital Microfluidic Devices}, author = {Burkhart, Collin Taylor}, year = {2021}, school = {Rochester Institute of Technology} }
[D-2021-06] Anastasiia Fedorenko. (2021). “Advanced Photovoltaic Devices Enabled by Lattice-Mismatched Epitaxy.” Rochester Institute of Technology.
Abstract: Thin-film III-V semiconductor-based photovoltaic (PV) devices, whose light conversion efficiency is primarily limited by the minority carrier lifetimes, are commonly designed to minimize the formation of crystalline defects (threading dislocations or, in extreme cases, fractures) that can occur, in particular, due to a mismatch in lattice constants of the epitaxial substrate and of the active film. At the same time, heteroepitaxy using Si or metal foils instead of costly III-V substrates is a pathway to enabling low-cost thin-film III-V-based PV and associated devices, yet it requires to either use metamorphic buffers or lateral confinement either by substrate patterning or by growing high aspect ratio structures. Mismatched epitaxy can be used for high-efficiency durable III-V space PV systems by incorporation of properly engineered strained quantum confined structures into the solar cells that can enable bandgap engineering and enhanced radiation tolerance. One of the major topics covered in this work is optical and optoelectronic modeling and physics of the triple-junction solar cell featuring planar Si middle sub-cell and GaAs0.73P0.27 and InAs0.85P0.15 periodic nanowire (NW) top and bottom sub-cells, respectively. In particular, the dimensions of the NW arrays that would enable near-unity broad-band absorption for maximum generated current were identified. For the top cell, the planarized array dimensions corresponding to maximum generated current and current matching with the underlying Si sub-cell were found to be 350 nm for NW diameter and 450 – 500 nm for NW spacing. For the GaAs0.73P0.27, resonant coupling was the main factor driving the absorption, yet addressing the coupling of IR light in the transmission mode in the InAs0.85P0.15 nanoscale arrays was challenging and unique. Given the nature of the Si and bottom NW interface, the designs of high refractive index encapsulation materials and conformal reflectors were proposed to enable the use of thin NWs (300 – 400 nm) for sufficient IR absorption. A novel co-simulation tool combining RSoft DiffractMOD® and Sentaurus Device® was established and utilized to design the p-i-n 3D junction and thin conformal GaP passivation coating for maximum GaAs0.73P0.27 NW sub-cell efficiency (16.5%) mainly impacted by the carrier surface annihilation. Development of a highly efficient GaAs solar cell enhanced with InxGa1-xAs/GaAsyP1-y quantum wells (QWs) is also demonstrated as one of the key parts of the dissertation. The optimizations including design of GaAsP strain balancing that would support efficient thermal (here, 17 nm-thick GaAs0.90P0.10 for 9.2 nm-thick In0.10Ga0.90As QWs) and/or tunneling (4.9 nm-thick GaAs0.68P0.32) carrier escape out of the QW while maintaining a consistent morphology of the QW layers in extended QW superlattices were performed using the principles of strain energy minimization and by tuning the growth parameters. The fundamental open-circuit voltage (V¬oc) restraints in radiative and non-radiative recombination-limited regimes in the QW solar cells were studied for a variety of InxGa1-xAs compositions (x=6%, 8%, 10%, and 14%) and number of QWs using spectroscopic and dark current analysis and modeling. Additionally, the design and use of distributed Bragg reflectors for targeted up to 90% QW absorption enhancement is demonstrated resulting in an absolute QW solar cell efficiency increase by 0.4% due to nearly doubled current from the QWs and 0.1% enhancement relatively to the optically-thick baseline device with no QWs.
Publisher Site: RIT Repository
PDF (Requires Sign-In): PDF
Click for Bibtex
@book{fedorenko2021advanced, title = {Advanced Photovoltaic Devices Enabled by Lattice-Mismatched Epitaxy}, author = {Fedorenko, Anastasiia}, year = {2021}, school = {Rochester Institute of Technology} }
[D-2021-05] Joanna Cecilia da Silva Santos. (2021). “Understanding and Identifying Vulnerabilities Related to Architectural Security Tactics.” Rochester Institute of Technology.
Abstract: To engineer secure software systems, software architects elicit the system's security requirements to adopt suitable architectural solutions. They often make use of architectural security tactics when designing the system's security architecture. Security tactics are reusable solutions to detect, resist, recover from, and react to attacks. Since security tactics are the building blocks of a security architecture, flaws in the adoption of these tactics, their incorrect implementation, or their deterioration during software maintenance activities can lead to vulnerabilities, which we refer to as "tactical vulnerabilities". Although security tactics and their correct adoption/implementation are crucial elements to achieve security, prior works have not investigated the architectural context of vulnerabilities. Therefore, this dissertation presents a research work whose major goals are: (i) to identify common types of tactical vulnerabilities, (ii) to investigate tactical vulnerabilities through in-depth empirical studies, and (iii) to develop a technique that detects tactical vulnerabilities caused by object deserialization. First, we introduce the Common Architectural Weakness Enumeration (CAWE), which is a catalog that enumerates 223 tactical vulnerability types. Second, we use this catalog to conduct an empirical study using vulnerability reports from large-scale open-source systems. Among our findings, we observe that "Improper Input Validation" was the most reoccurring vulnerability type. This tactical vulnerability type is caused by not properly implementing the "Validate Inputs" tactic. Although prior research focused on devising automated (or semi-automated) techniques for detecting multiple instances of improper input validation (e.g., SQL Injection and Cross-Site Scripting) one of them got neglected, which is the untrusted deserialization of objects. Unlike other input validation problems, object deserialization vulnerabilities exhibit a set of characteristics that are hard to handle for effective vulnerability detection. We currently lack a robust approach that can detect untrusted deserialization problems. Hence, this dissertation introduces DODO untrusteD ObjectDeserialization detectOr), a novel program analysis technique to detect deserialization vulnerabilities. DODO encompasses a sound static analysis of the program to extract potentially vulnerable paths, an exploit generation engine, and a dynamic analysis engine to verify the existence of untrusted object deserialization. Our experiments showed that DODO can successfully infer possible vulnerabilities that could arise at runtime during object deserialization.
Publisher Site: RIT Repository
PDF (Requires Sign-In): PDF
Click for Bibtex
@book{da silva santos2021understanding, title = {Understanding and Identifying Vulnerabilities Related to Architectural Security Tactics}, author = {da Silva Santos, Joanna Cecilia}, year = {2021}, school = {Rochester Institute of Technology} }
[D-2021-04] Tejaswini Ananthanarayana. (2021). “A Comprehensive Approach to Automated Sign Language Translation.” Rochester Institute of Technology.
Abstract: Many sign languages are bonafide natural languages with grammatical rules and lexicons, hence can benefit from neural machine translation methods. As significant advances are being made in natural language processing (specifically neural machine translation) and in computer vision processes, specifically image and video captioning, related methods can be further researched to boost automated sign language understanding. This is an especially challenging AI research area due to the involvement of a continuous visual-spatial modality, where meaning is often derived from context. To this end, this thesis is focused on the study and development of new computational methods and training mechanisms to enhance sign language translation in two directions, signs to texts and texts to signs. This work introduces a new, realistic phrase-level American Sign Language dataset (ASL/ ASLing), and investigates the role of different types of visual features (CNN embeddings, human body keypoints, and optical flow vectors) in translating ASL to spoken American English. Additionally, the research considers the role of multiple features for improved translation, via various fusion architectures. As an added benefit, with continuous sign language being challenging to segment, this work also explores the use of overlapping scaled visual segments, across the video, for simultaneously segmenting and translating signs. Finally, a quintessential interpreting agent not only understands sign language and translates to text, but also understands the text and translates to signs. Hence, to facilitate two-way sign language communication, i.e. visual sign to spoken language translation and spoken to visual sign language translation, a dual neural machine translation model, SignNet, is presented. Various training paradigms are investigated for improved translation, using SignNet. By exploiting the notion of similarity (and dissimilarity) of visual signs, a metric embedding learning process proved most useful in training SignNet. The resulting processes outperformed their state-of-the-art counterparts by showing noteworthy improvements in BLEU 1 - BLEU 4 scores.
Publisher Site: RIT Repository
PDF (Requires Sign-In): PDF
Click for Bibtex
@book{ananthanarayana2021a, title = {A Comprehensive Approach to Automated Sign Language Translation}, author = {Ananthanarayana, Tejaswini}, year = {2021}, school = {Rochester Institute of Technology} }
[D-2021-03] Rakshit S. Kothari. (2021). “Towards Robust Gaze Estimation and Classification in Naturalistic Conditions.” Rochester Institute of Technology.
Abstract: Eye movements help us identify when and where we are fixating. The location under fixation is a valuable source of information in decoding a person's intent or as an input modality for human-computer interaction. However, it can be difficult to maintain fixation under motion unless our eyes compensate for body movement. Humans have evolved compensatory mechanisms using the vestibulo-ocular reflex pathway which ensures stable fixation under motion. The interaction between the vestibular and ocular system has primarily been studied in controlled environments, with comparatively few studies during natural tasks that involve coordinated head and eye movements under unrestrained body motion. Moreover, off-the-shelf tools for analyzing gaze events perform poorly when head movements are allowed. To address these issues we developed algorithms for gaze event classification and collected the Gaze-in-Wild (GW) dataset. However, reliable inference of human behavior during in-the-wild activities depends heavily on the quality of gaze data extracted from eyetrackers. State of the art gaze estimation algorithms can be easily affected by occluded eye features, askew eye camera orientation and reflective artifacts from the environments - factors commonly found in unrestrained experiment designs. To inculcate robustness to reflective artifacts, our efforts helped develop RITNet, a convolutional encoder-decoder neural network which successfully segments eye images into semantic parts such as pupil, iris and sclera. Well chosen data augmentation techniques and objective functions combat reflective artifacts and helped RITNet achieve first place in OpenEDS'19, an international competition organized by Facebook Reality Labs. To induce robustness to occlusions, our efforts resulted in a novel eye image segmentation protocol, EllSeg. EllSeg demonstrates state of the art pupil and iris detection despite the presence of reflective artifacts and occlusions. While our efforts have shown promising results in developing a reliable and robust gaze feature extractor, convolutional neural networks are prone to overfitting and do not generalize well beyond the distribution of data it was optimized on. To mitigate this limitation and explore the generalization capacity of EllSeg, we acquire a wide distribution of eye images sourced from multiple publicly available datasets to develop EllSeg-Gen, a domain generalization framework for segmenting eye imagery. EllSeg-Gen proposes four tests which allow us to quantify generalization. We find that jointly training with multiple datasets improves generalization for eye images acquired outdoors. In contrast, specialized dataset specific models are better suited for indoor domain generalization.
Publisher Site: RIT Repository
PDF (Requires Sign-In): PDF
Click for Bibtex
@book{kothari2021towards, title = {Towards Robust Gaze Estimation and Classification in Naturalistic Conditions}, author = {Kothari, Rakshit S.}, year = {2021}, school = {Rochester Institute of Technology} }
[D-2021-02] Stephen Frank Moskal. (2021). “HeAt PATRL: Network-Agnostic Cyber Attack Campaign Triage with Pseudo-Active Transfer Learning.” Rochester Institute of Technology.
Abstract: SOC (Security Operation Center) analysts historically struggled to keep up with the growing sophistication and daily prevalence of cyber attackers. To aid in the detection of cyber threats, many tools like IDS's (Intrusion Detection Systems) are utilized to monitor cyber threats on a network. However, a common problem with these tools is the volume of the logs generated is extreme and does not stop, further increasing the chance for an adversary to go unnoticed until it's too late. Typically, the initial evidence of an attack is not an isolated event but a part of a larger attack campaign describing prior events that the attacker took to reach their final goal. If an analyst can quickly identify each step of an attack campaign, a timely response can be made to limit the impact of the attack or future attacks. In this work, we ask the question "Given IDS alerts, can we extract out the cyber-attack kill chain for an observed threat that is meaningful to the analyst?" We present HeAT-PATRL, an IDS attack campaign extractor that leverages multiple deep machine learning techniques, network-agnostic feature engineering, and the analyst's knowledge of potential threats to extract out cyber-attack campaigns from IDS alert logs. HeAT-PATRL is the culmination of two works. Our first work "PATRL" (Pseudo-Active Transfer Learning), translates the complex alert signature description to the Action-Intent Framework (AIF), a customized set of attack stages. PATRL employs a deep language model with cyber security texts (CVE's, C-Sec Blogs, etc.) and then uses transfer learning to classify alert descriptions. To further leverage the cyber-context learned in the language model, we develop Pseudo-Active learning to self-label unknown unlabeled alerts to use as additional training data. We show PATRL classifying the entire Suricata database (~70k signatures) with a top-1 of 87\% and top-3 of 99\% with less than 1,200 manually labeled signatures. The final work, HeAT (Heated Alert Triage), captures the analyst's domain knowledge and opinion of the contribution of IDS events to an attack campaign given a critical IoC (indicator of compromise). We developed network-agnostic features to characterize and generalize attack campaign contributions so that prior triages can aid in identifying attack campaigns for other attack types, new attackers, or network infrastructures. With the use of cyber-attack competition data (CPTC) and data from a real SOC operation, we demonstrate that the HeAT process can identify campaigns reflective of the analysts thinking while greatly reducing the number of actions to be assessed by the analyst. HeAT has the unique ability to uncover attack campaigns meaningful to the analyst across drastically different network structures while maintaining the important attack campaign relationships defined by the analyst.
Publisher Site: RIT Repository
PDF (Requires Sign-In): PDF
Click for Bibtex
@book{moskal2021heat, title = {HeAt PATRL: Network-Agnostic Cyber Attack Campaign Triage with Pseudo-Active Transfer Learning}, author = {Moskal, Stephen Frank}, year = {2021}, school = {Rochester Institute of Technology} }
[D-2021-01] Maojia Patrick Li. (2021). “Task Assignment and Path Planning for Autonomous Mobile Robots in Stochastic Warehouse Systems.” Rochester Institute of Technology.
Abstract: The material handling industry is in the middle of a transformation from manual operations to automation due to the rapid growth in e-commerce. Autonomous mobile robots (AMRs) are being widely implemented to replace manually operated forklifts in warehouse systems to fulfil large shipping demand, extend warehouse operating hours, and mitigate safety concerns. Two open questions in AMR management are task assignment and path planning. This dissertation addresses the task assignment and path planning (TAPP) problem for autonomous mobile robots (AMR) in a warehouse environment. The goals are to maximize system productivity by avoiding AMR traffic and reducing travel time. The first topic in this dissertation is the development of a discrete event simulation modeling framework that can be used to evaluate alternative traffic control rules, task assignment methods, and path planning algorithms. The second topic, Risk Interval Path Planning (RIPP), is an algorithm designed to avoid conflicts among AMRs considering uncertainties in robot motion. The third topic is a deep reinforcement learning (DRL) model that is developed to solve task assignment and path planning problems, simultaneously. Experimental results demonstrate the effectiveness of these methods in stochastic warehouse systems.
Publisher Site: RIT Repository
PDF (Requires Sign-In): PDF
Click for Bibtex
@book{li2021task, title = {Task Assignment and Path Planning for Autonomous Mobile Robots in Stochastic Warehouse Systems}, author = {Li, Maojia Patrick}, year = {2021}, school = {Rochester Institute of Technology} }
[D-2020-26] Devarth Parikh. (2020). “Gaze Estimation Based on Multi-view Geometric Neural Networks.” Rochester Institute of Technology.
Abstract: Gaze and head pose estimation can play essential roles in various applications, such as human attention recognition and behavior analysis. Most of the deep neural network-based gaze estimation techniques use supervised regression techniques where features are extracted from eye images by neural networks and regress 3D gaze vectors. I plan to apply the geometric features of the eyes to determine the gaze vectors of observers relying on the concepts of 3D multiple view geometry. We develop an end to-end CNN framework for gaze estimation using 3D geometric constraints under semi-supervised and unsupervised settings and compare the results. We explore the mathematics behind the concepts of Homography and Structure-from- Motion and extend it to the gaze estimation problem using the eye region landmarks. We demonstrate the necessity of the application of 3D eye region landmarks for implementing the 3D geometry-based algorithms and address the problem when lacking the depth parameters in the gaze estimation datasets. We further explore the use of Convolutional Neural Networks (CNNs) to develop an end-to-end learning-based framework, which takes in sequential eye images to estimate the relative gaze changes of observers. We use a depth network for performing monocular image depth estimation of the eye region landmarks, which are further utilized by the pose network to estimate the relative gaze change using view synthesis constraints of the iris regions. We further explore CNN frameworks to estimate the relative changes in homography matrices between sequential eye images based on the eye region landmarks to estimate the pose of the iris and hence determine the relative change in the gaze of the observer. We compare and analyze the results obtained from mathematical calculations and deep neural network-based methods. We further compare the performance of the proposed CNN scheme with the state-of-the-art regression-based methods for gaze estimation. Future work involves extending the end-to-end pipeline as an unsupervised framework for gaze estimation in the wild.
Publisher Site: RIT Repository
PDF (Requires Sign-In): PDF
Click for Bibtex
@book{parikh2020gaze, title = {Gaze Estimation Based on Multi-view Geometric Neural Networks}, author = {Parikh, Devarth}, year = {2020}, school = {Rochester Institute of Technology} }
[D-2020-25] Elisabeth L. McClure. (2020). “Optimization and Characterization of Low-Cost Substrates for III-V Photovoltaics.” Rochester Institute of Technology.
Abstract: The highest achieved photovoltaic power conversion efficiency (approximately 47% under concentration) is available from III-V multijunction solar cells made from subcells of descending bandgap that optimize light collection from the solar spectrum. Unfortunately, both III-V multijunction and single-junction solar cells are expensive, limiting their use to niche concentration or space power applications and precluding their competitiveness in the terrestrial flat-plate market. The majority of the III-V solar cell cost is attributed to the thick, monocrystalline substrates that are used as a platform for epitaxial growth, and to the throughput, precursors, and utilization of those precursors associated with traditional growth reactors. Significant cost reduction to approach $1/W for total photovoltaic system cost is imperative to realize III-V solar cells that are cost-competitive with incumbent silicon solar cells, and can include techniques to develop inexpensive substrates directly; enable multiple reuses of a pristine, expensive substrate without the need for polishing; and enhance the throughput by increasing the semiconductor growth rate during epitaxy. This dissertation explores two main techniques to achieve low-cost substrates for III-V photovoltaics: aluminum-induced crystallization to create polycrystalline germanium thin films, and remote epitaxy through graphene to enable monocrystalline substrate reuse without polishing. This dissertation also demonstrates record III-V growth rates exceeding 0.5 mm/h using a potentially lower-cost III-V growth technique, which would increase throughput in production reactors. The ability to reduce the costs associated with both substrates and epitaxy will be imperative to decreasing the total system cost of III-V PV.
Publisher Site: RIT Repository
PDF (Requires Sign-In): PDF
Click for Bibtex
@book{mcclure2020optimization, title = {Optimization and Characterization of Low-Cost Substrates for III-V Photovoltaics}, author = {McClure, Elisabeth L.}, year = {2020}, school = {Rochester Institute of Technology} }
[D-2020-24] Kushal Kafle. (2020). “Advancing Multi-modal Deep Learning: Towards Language-grounded Visual Understanding.” Rochester Institute of Technology.
Abstract: Using deep learning, computer vision now rivals people at object recognition and detection, opening doors to tackle new challenges in image understanding. Among these challenges, understanding and reasoning about language grounded visual content is of fundamental importance to advancing artificial intelligence. Recently, multiple datasets and algorithms have been created as proxy tasks towards this goal, with visual question answering (VQA) being the most widely studied. In VQA, an algorithm needs to produce an answer to a natural language question about an image. However, our survey of datasets and algorithms for VQA uncovered several sources of dataset bias and sub-optimal evaluation metrics that allowed algorithms to perform well by merely exploiting superficial statistical patterns. In this dissertation, we describe new algorithms and datasets that address these issues. We developed two new datasets and evaluation metrics that enable a more accurate measurement of abilities of a VQA model, and also expand VQA to include new abilities, such as reading text, handling out-of-vocabulary words, and understanding data-visualization. We also created new algorithms for VQA that have helped advance the state-of-the-art for VQA, including an algorithm that surpasses humans on two different chart question answering datasets about bar-charts, line-graphs and pie charts. Finally, we provide a holistic overview of several yet-unsolved challenges in not only VQA but vision and language research at large. Despite enormous progress, we find that a robust understanding and integration of vision and language is still an elusive goal, and much of the progress may be misleading due to dataset bias, superficial correlations and flaws in standard evaluation metrics. We carefully study and categorize these issues for several vision and language tasks and outline several possible paths towards development of safe, robust and trustworthy AI for language-grounded visual understanding.
Publisher Site: RIT Repository
PDF (Requires Sign-In): PDF
Click for Bibtex
@book{kafle2020advancing, title = {Advancing Multi-modal Deep Learning: Towards Language-grounded Visual Understanding}, author = {Kafle, Kushal}, year = {2020}, school = {Rochester Institute of Technology} }
[D-2020-23] Michael Larry Fanto. (2020). “Nonlinear Optics in Photonic Ultrawide-Bandgap Circuits.” Rochester Institute of Technology.
Abstract: Ultrawide-bandgap semiconductors have an important role for many applications ranging from power electronics to photonics. The semiconductor material with the largest bandgap is aluminum nitride with a maximum bandgap of 6.28 eV, corresponding to a wavelength transparency extending to 197 nm. The large transparency window makes aluminum nitride a promising optical material for applications that span from the infrared to ultraviolet. Along with these properties, aluminum nitride is a non-centrosymmetric crystal allowing exploitation of the second order nonlinearity and the electro-optic effect. This thesis will cover the synthesis of the material, a number of characterization techniques that verify the quality of the material, and conclude with applications for the synthesized material. To date we have demonstrated an ultrawide-bandgap semiconductor photonics platform based on nanocrystalline aluminum nitride (AlN) on sapphire. This photonics platform guides light at low loss from the ultraviolet (UV) to the visible spectrum, though is transparent from 200 -15,000 nm. We measured ring resonators with intrinsic quality factor (Q) exceeding 170,000 at 638 nm and Q >20,000 down to 369.5 nm, which shows a promising path for low-loss integrated photonics in UV and visible spectrum. The nonlinear, electro-optic, and piezo-electric properties of AlN make it a promising active material for controlling and connecting atomic and atom-like quantum memories, coherent qubit transduction, entangled photon generation, and frequency conversion.
Publisher Site: RIT Repository
PDF (Requires Sign-In): PDF
Click for Bibtex
@book{fanto2020nonlinear, title = {Nonlinear Optics in Photonic Ultrawide-Bandgap Circuits}, author = {Fanto, Michael Larry}, year = {2020}, school = {Rochester Institute of Technology} }
[D-2020-22] Jwala Dhamala. (2020). “Bayesian Active Learning for Personalization and Uncertainty Quantification in Cardiac Electrophysiological Model.” Rochester Institute of Technology.
Abstract: Cardiacvascular disease is the top death causing disease worldwide. In recent years, high-fidelity personalized models of the heart have shown an increasing capability to supplement clinical cardiology for improved patient-specific diagnosis, prediction, and treatment planning. In addition, they have shown promise to improve scientific understanding of a variety of disease mechanisms. However, model personalization by estimating the patient-specific tissue properties that are in the form of parameters of a physiological model is challenging. This is because tissue properties, in general, cannot be directly measured and they need to be estimated from measurements that are indirectly related to them through a physiological model. Moreover, these unknown tissue properties are heterogeneous and spatially varying throughout the heart volume presenting a difficulty of high-dimensional (HD) estimation from indirect and limited measurement data. The challenge in model personalization, therefore, summarizes to solving an ill-posed inverse problem where the unknown parameters are HD and the forward model is complex with a non-linear and computationally expensive physiological model. In this dissertation, we address the above challenge with following contributions. First, to address the concern of a complex forward model, we propose the surrogate modeling of the complex target function containing the forward model – an objective function in deterministic estimation or a posterior probability density function in probabilistic estimation – by actively selecting a set of training samples and a Bayesian update of the prior over the target function. The efficient and accurate surrogate of the expensive target function obtained in this manner is then utilized to accelerate either deterministic or probabilistic parameter estimation. Next, within the framework of Bayesian active learning we enable active surrogate learning over a HD parameter space with two novel approaches: 1) a multi-scale optimization that can adaptively allocate higher resolution to heterogeneous tissue regions and lower resolution to homogeneous tissue regions; and 2) a generative model from low-dimensional (LD) latent code to HD tissue properties. Both of these approaches are independently developed and tested within a parameter optimization framework. Furthermore, we devise a novel method that utilizes the surrogate pdf learned on an estimated LD parameter space to improve the proposal distribution of Metropolis Hastings for an accelerated sampling of the exact posterior pdf. We evaluate the presented methods on estimating local tissue excitability of a cardiac electrophysiological model in both synthetic data experiments and real data experiments. Results demonstrate that the presented methods are able to improve the accuracy and efficiency in patient-specific model parameter estimation in comparison to the existing approaches used for model personalization.
Publisher Site: RIT Repository
PDF (Requires Sign-In): PDF
Click for Bibtex
@book{dhamala2020bayesian, title = {Bayesian Active Learning for Personalization and Uncertainty Quantification in Cardiac Electrophysiological Model}, author = {Dhamala, Jwala}, year = {2020}, school = {Rochester Institute of Technology} }
[D-2020-21] Nuthan Munaiah. (2020). “Toward Data-Driven Discovery of Software Vulnerabilities.” Rochester Institute of Technology.
Abstract: Over the years, Software Engineering, as a discipline, has recognized the potential for engineers to make mistakes and has incorporated processes to prevent such mistakes from becoming exploitable vulnerabilities. These processes span the spectrum from using unit/integration/fuzz testing, static/dynamic/hybrid analysis, and (automatic) patching to discover instances of vulnerabilities to leveraging data mining and machine learning to collect metrics that characterize attributes indicative of vulnerabilities. Among these processes, metrics have the potential to uncover systemic problems in the product, process, or people that could lead to vulnerabilities being introduced, rather than identifying specific instances of vulnerabilities. The insights from metrics can be used to support developers and managers in making decisions to improve the product, process, and/or people with the goal of engineering secure software. Despite empirical evidence of metrics' association with historical software vulnerabilities, their adoption in the software development industry has been limited. The level of granularity at which the metrics are defined, the high false positive rate from models that use the metrics as explanatory variables, and, more importantly, the difficulty in deriving actionable intelligence from the metrics are often cited as factors that inhibit metrics' adoption in practice. Our research vision is to assist software engineers in building secure software by providing a technique that generates scientific, interpretable, and actionable feedback on security as the software evolves. In this dissertation, we present our approach toward achieving this vision through (1) systematization of vulnerability discovery metrics literature, (2) unsupervised generation of metrics-informed security feedback, and (3) continuous developer-in-the-loop improvement of the feedback. We systematically reviewed the literature to enumerate metrics that have been proposed and/or evaluated to be indicative of vulnerabilities in software and to identify the validation criteria used to assess the decision-informing ability of these metrics. In addition to enumerating the metrics, we implemented a subset of these metrics as containerized microservices. We collected the metric values from six large open-source projects and assessed metrics' generalizability across projects, application domains, and programming languages. We then used an unsupervised approach from literature to compute threshold values for each metric and assessed the thresholds' ability to classify risk from historical vulnerabilities. We used the metrics' values, thresholds, and interpretation to provide developers natural language feedback on security as they contributed changes and used a survey to assess their perception of the feedback. We initiated an open dialogue to gain an insight into their expectations from such feedback. In response to developer comments, we assessed the effectiveness of an existing vulnerability discovery approach—static analysis—and that of vulnerability discovery metrics in identifying risk from vulnerability contributing commits.
Publisher Site: RIT Repository
PDF (Requires Sign-In): PDF
Click for Bibtex
@book{munaiah2020toward, title = {Toward Data-Driven Discovery of Software Vulnerabilities}, author = {Munaiah, Nuthan}, year = {2020}, school = {Rochester Institute of Technology} }
[D-2020-20] Shitij P. Kumar. (2020). “Dynamic Speed and Separation Monitoring with On-Robot Ranging Sensor Arrays for Human and Industrial Robot Collaboration.” Rochester Institute of Technology.
Abstract: This research presents a flexible and dynamic implementation of Speed and Separation Monitoring (SSM) safety measure that optimizes the productivity of a task while ensuring human safety during Human-Robot Collaboration (HRC). Unlike the standard static/fixed demarcated 2D safety zones based on 2D scanning LiDARs, this research presents a dynamic sensor setup that changes the safety zones based on the robot pose and motion. The focus of this research is the implementation of a dynamic SSM safety configuration using Time-of-Flight (ToF) laser-ranging sensor arrays placed around the centers of the links of a robot arm. It investigates the viability of on-robot exteroceptive sensors for implementing SSM as a safety measure. Here the implementation of varying dynamic SSM safety configurations based on approaches of measuring human-robot separation distance and relative speeds using the sensor modalities of ToF sensor arrays, a motion-capture system, and a 2D LiDAR is shown. This study presents a comparative analysis of the dynamic SSM safety configurations in terms of safety, performance, and productivity. A system of systems (cyber-physical system) architecture for conducting and analyzing the HRC experiments was proposed and implemented. The robots, objects, and human operators sharing the workspace are represented virtually as part of the system by using a digital-twin setup. This system was capable of controlling the robot motion, monitoring human physiological response, and tracking the progress of the collaborative task. This research conducted experiments with human subjects performing a task while sharing the robot workspace under the proposed dynamic SSM safety configurations. The experiment results showed a preference for the use of ToF sensors and motion capture rather than the 2D LiDAR currently used in the industry. The human subjects felt safe and comfortable using the proposed dynamic SSM safety configuration with ToF sensor arrays. The results for a standard pick and place task showed up to a 40% increase in productivity in comparison to a 2D LiDAR.
Publisher Site: RIT Repository
PDF (Requires Sign-In): PDF
Click for Bibtex
@book{kumar2020dynamic, title = {Dynamic Speed and Separation Monitoring with On-Robot Ranging Sensor Arrays for Human and Industrial Robot Collaboration}, author = {Kumar, Shitij P.}, year = {2020}, school = {Rochester Institute of Technology} }
[D-2020-19] Viraj Reddy Adduru. (2020). “Automated Brain Segmentation Methods for Clinical Quality MRI and CT Images.” Rochester Institute of Technology.
Abstract: Alzheimer's disease (AD) is a progressive neurodegenerative disorder associated with brain tissue loss. Accurate estimation of this loss is critical for the diagnosis, prognosis, and tracking the progression of AD. Structural magnetic resonance imaging (sMRI) and X-ray computed tomography (CT) are widely used imaging modalities that help to in vivo map brain tissue distributions. As manual image segmentations are tedious and time-consuming, automated segmentation methods are increasingly applied to head MRI and head CT images to estimate brain tissue volumes. However, existing automated methods can be applied only to images that have high spatial resolution and their accuracy on heterogeneous low-quality clinical images has not been tested. Further, automated brain tissue segmentation methods for CT are not available, although CT is more widely acquired than MRI in the clinical setting. For these reasons, large clinical imaging archives are unusable for research studies. In this work, we identify and develop automated tissue segmentation and brain volumetry methods that can be applied to clinical quality MRI and CT images. In the first project, we surveyed the current MRI methods and validated the accuracy of these methods when applied to clinical quality images. We then developed CTSeg, a tissue segmentation method for CT images, by adopting the MRI technique that exhibited the highest reliability. CTSeg is an atlas-based statistical modeling method that relies on hand-curated features and cannot be applied to images of subjects with different diseases and age groups. Advanced deep learning-based segmentation methods use hierarchical representations and learn complex features in a data-driven manner. In our final project, we develop a fully automated deep learning segmentation method that uses contextual information to segment clinical quality head CT images. The application of this method on an AD dataset revealed larger differences between brain volumes of AD and control subjects. This dissertation demonstrates the potential of applying automated methods to large clinical imaging archives to answer research questions in a variety of studies.
Publisher Site: RIT Repository
PDF (Requires Sign-In): PDF
Click for Bibtex
@book{adduru2020automated, title = {Automated Brain Segmentation Methods for Clinical Quality MRI and CT Images}, author = {Adduru, Viraj Reddy}, year = {2020}, school = {Rochester Institute of Technology} }
[D-2020-18] Alexander Fafard. (2020). “Clearing the Clouds: Extracting 3D Information from Amongst the Noise.” Rochester Institute of Technology.
Abstract: Advancements permitting the rapid extraction of 3D point clouds from a variety of imaging modalities across the global landscape have provided a vast collection of high fidelity digital surface models. This has created a situation with unprecedented overabundance of 3D observations which greatly outstrips our current capacity to manage and infer actionable information. While years of research have removed some of the manual analysis burden for many tasks, human analysis is still a cornerstone of 3D scene exploitation. This is especially true for complex tasks which necessitate comprehension of scale, texture and contextual learning. In order to ameliorate the interpretation burden and enable scientific discovery from this volume of data, new processing paradigms are necessary to keep pace. With this context, this dissertation advances fundamental and applied research in 3D point cloud data pre-processing and deep learning from a variety of platforms. We show that the representation of 3D point data is often not ideal and sacrifices fidelity, context or scalability. First ground scanning terrestrial LIght Detection And Ranging (LiDAR) models are shown to have an inherent statistical bias, and present a state of the art method for correcting this, while preserving data fidelity and maintaining semantic structure. This technique is assessed in the dense canopy of Micronesia, with our technique being the best at retaining high levels of detail under extreme down-sampling ( The culmination of these efforts have the potential to enhance the capabilities of automated 3D geospatial processing, substantially lightening the burden of analysts, with implications improving our responses to global security, disaster response, climate change, structural design and extraplanetary exploration.
Publisher Site: RIT Repository
PDF (Requires Sign-In): PDF
Click for Bibtex
@book{fafard2020clearing, title = {Clearing the Clouds: Extracting 3D Information from Amongst the Noise}, author = {Fafard, Alexander}, year = {2020}, school = {Rochester Institute of Technology} }
[D-2020-17] Igor Khokhlov. (2020). “Integrated Framework for Data Quality and Security Evaluation on Mobile Devices.” Rochester Institute of Technology.
Abstract: Data quality (DQ) is an important concept that is used in the design and employment of information, data management, decision making, and engineering systems with multiple applications already available for solving specific problems. Unfortunately, conventional approaches to DQ evaluation commonly do not pay enough attention or even ignore the security and privacy of the evaluated data. In this research, we develop a framework for the DQ evaluation of the sensor originated data acquired from smartphones, that incorporates security and privacy aspects into the DQ evaluation pipeline. The framework provides support for selecting the DQ metrics and implementing their calculus by integrating diverse sensor data quality and security metrics. The framework employs a knowledge graph to facilitate its adaptation in new applications development and enables knowledge accumulation. Privacy aspects evaluation is demonstrated by the detection of novel and sophisticated attacks on data privacy on the example of colluded applications attack recognition. We develop multiple calculi for DQ and security evaluation, such as a hierarchical fuzzy rules expert system, neural networks, and an algebraic function. Case studies that demonstrate the framework's performance in solving real-life tasks are presented, and the achieved results are analyzed. These case studies confirm the framework's capability of performing comprehensive DQ evaluations. The framework development resulted in producing multiple products, and tools such as datasets and Android OS applications. The datasets include the knowledge base of sensors embedded in modern mobile devices and their quality analysis, technological signals recordings of smartphones during the normal usage, and attacks on users' privacy. These datasets are made available for public use and can be used for future research in the field of data quality and security. We also released under an open-source license a set of Android OS tools that can be used for data quality and security evaluation.
Publisher Site: RIT Repository
PDF (Requires Sign-In): PDF
Click for Bibtex
@book{khokhlov2020integrated, title = {Integrated Framework for Data Quality and Security Evaluation on Mobile Devices}, author = {Khokhlov, Igor}, year = {2020}, school = {Rochester Institute of Technology} }
[D-2020-16] Miguel Dominguez. (2020). “High-capacity Directional Graph Networks.” Rochester Institute of Technology.
Abstract: Deep Neural Networks (DNN) have proven themselves to be a useful tool in many computer vision problems. One of the most popular forms of the DNN is the Convolutional Neural Network (CNN). The CNN effectively learns features on images by learning a weighted sum of local neighborhoods of pixels, creating filtered versions of the image. Point cloud analysis seems like it would benefit from this useful model. However, point clouds are much less structured than images. Many analogues to CNNs for point clouds have been proposed in the literature, but they are often much more constrained networks than the typical CNN. This is a matter of necessity: common point cloud benchmark datasets are fairly small and thus require strong regularization to mitigate overfitting. In this dissertation we propose two point cloud network models based on graph structures that achieve the high-capacity modeling capability of CNNs. In addition to showing their effectiveness on point cloud classification and segmentation in typical benchmark scenarios, we also propose two novel point cloud problems: ATLAS Detector segmentation and Computational Fluid Dynamics (CFD) surrogate modeling. We show that our networks are much more effective than others on these new problems because they benefit from deeper networks and extra capacity that other researchers have not pursued. These novel networks and datasets pave the way for future development of deeper, more sophisticated point cloud networks.
Publisher Site: RIT Repository
PDF (Requires Sign-In): PDF
Click for Bibtex
@book{dominguez2020high-capacity, title = {High-capacity Directional Graph Networks}, author = {Dominguez, Miguel}, year = {2020}, school = {Rochester Institute of Technology} }
[D-2020-15] Benjamin David Roth. (2020). “Deciduous Broadleaf Bidirectional Scattering Distribution Function (BSDF): Measurements, Modeling, and Impacts on Waveform Lidar Forest Assessments.” Rochester Institute of Technology.
Abstract: Lidar (light detection and ranging) remote sensing has proven high accuracy/precision for quantification of forest biophysical parameters, many of which are needed for operational and ecological management. Although the significant effect of Bidirectional Scattering Distribution Functions (BSDF) on remote sensing of vegetation is well known, current radiative transfer simulations, designed for the development of remote sensing systems for ecological observation, seldom take leaf BSDF into account. Moreover, leaf directional scattering measurements are almost nonexistent, particularly for transmission. Previous studies have been limited in their electromagnetic spectrum extent, lacked validated models to capture all angles beyond measurements, and did not adequately incorporate transmission scattering. Many current remote sensing simulations assume leaves with Lambertian reflectance, opaque leaves, or apply purely Lambertian transmission, even though the validity of these assumptions and the effect on simulation results are currently unknown. This study captured deciduous broadleaf BSDFs (Norway Maple (Acer platanoides), American Sweetgum (Liquidambar styraciflua), and Northern Red Oak (Quercus rubra)) from the ultraviolet through shortwave infrared spectral regions (350-2500 nm), and accurately modeled the BSDF for extension to any illumination angle, viewing zenith, or azimuthal angle. Relative leaf physical parameters were extracted from the microfacet models delineating the three species. Leaf directional scattering effects on waveform lidar (wlidar) signals and their dependence on wavelength, lidar footprint, view angle, and leaf angle distribution (LAD) were explored using the Digital Imaging and Remote Sensing Image Generation (DIRSIG) model. The greatest effects, compared to Lambertian assumptions, were observed at visible wavelengths, small wlidar footprints, and oblique interrogation angles relative to the mean leaf angle. These effects were attributed to (i) a large specular component of the BSDF in the visible region, (ii) small footprints having fewer leaf angles to integrate over, and (iii) oblique angles causing diminished backscatter due to forward scattering. Opaque leaf assumptions were seen to have the greatest error for near-infrared (NIR) wavelengths with large footprints, due to the increased multi-scatter contribution at these configurations. Armed with the knowledge from this study, researchers are able to select appropriate sensor configurations to account for or limit BSDF effects in forest lidar data.
Publisher Site: RIT Repository
PDF (Requires Sign-In): PDF
Click for Bibtex
@book{roth2020deciduous, title = {Deciduous Broadleaf Bidirectional Scattering Distribution Function (BSDF): Measurements, Modeling, and Impacts on Waveform Lidar Forest Assessments}, author = {Roth, Benjamin David}, year = {2020}, school = {Rochester Institute of Technology} }
[D-2020-14] Alyssa Owens. (2020). “A Patient-Specific Infrared Imaging Technique for Adjunctive Breast Cancer Screening: A Clinical and Simulation - Based Approach.” Rochester Institute of Technology.
Abstract: Breast cancer is currently the most prevalent form of cancer in women with over 266,000 new diagnoses every year. The various methods used for breast cancer screening range in accuracy and cost, however there is no easily reproducible, reliable, low-cost screening method currently available for detecting cancer in breasts, especially with dense tissue. Steady-state Infrared Imaging (IRI) is unaffected by tissue density and has the potential to detect tumors in the breast by measuring and capturing the thermal profile on the breast surface induced by increased blood perfusion and metabolic activity in a rapidly growing malignant tumor. The current work presents a better understanding of IRI as an accurate breast cancer detection modality. A detailed study utilizing IRI-MRI approach with clinical design and validation of an elaborate IRI-Mammo study are presented by considering patient population, clinical study design, image interpretation, and recommended future path. Clinical IRI images are obtained in this study and an ANSYS-based modeling process developed earlier at RIT is used to localize and detect tumor in seven patients without subjective human interpretation. Further, the unique thermal characteristics of tumors that make their signatures distinct from benign conditions are identified. This work is part of an ongoing multidisciplinary collaboration between a team of thermal engineers and numerical modelers at the Rochester Institute of Technology and a team of clinicians at the Rochester General Hospital. The following components were developed to ensure valid experimentation while considering ethical considerations: IRB documentation, patient protocols, an image acquisition system (camera setup and screening table), and the necessary tools needed for image analysis without human interpretation. IRI images in the prone position were obtained and were used in accurately detecting the presence of a cancerous tumor in seven subjects. The size and location of tumor was also confirmed within 7 mm as compared to biopsy-proven pathology information. The study indicates that the IRI-Mammo approach has potential to be a highly effective adjunctive screening tool that can improve the breast cancer detection rates especially for subjects with dense breast tissue. This method is low cost, no-touch, radiation-free and highly portable, making it an attractive candidate as a breast cancer detection modality. Further, the developed method provided insight into infrared features corresponding to other biological images, pathology reports and patient history.
Publisher Site: RIT Repository
PDF (Requires Sign-In): PDF
Click for Bibtex
@book{owens2020a, title = {A Patient-Specific Infrared Imaging Technique for Adjunctive Breast Cancer Screening: A Clinical and Simulation - Based Approach}, author = {Owens, Alyssa}, year = {2020}, school = {Rochester Institute of Technology} }
[D-2020-13] Ali Rouzbeh Kargar. (2020). “On the Use of Rapid-Scan, Low Point Density Terrestrial Laser Scanning (TLS) for Structural Assessment of Complex Forest Environments.” Rochester Institute of Technology.
Abstract: Forests fulfill an important role in natural ecosystems, e.g., they provide food, fiber, habitat, and biodiversity, all of which contribute to stable ecosystems. Assessing and modeling the structure and characteristics in forests can lead to a better understanding and management of these resources. Traditional methods for collecting forest traits, known as "forest inventory", is achieved using rough proxies, such as stem diameter, tree height, and foliar coverage; such parameters are limited in their ability to capture fine-scale structural variation in forest environments. It is in this context that terrestrial laser scanning (TLS) has come to the fore as a tool for addressing the limitations of traditional forest structure evaluation methods. However, there is a need for improving TLS data processing methods. In this work, we developed algorithms to assess the structure of complex forest environments – defined by their stem density, intricate root and stem structures, uneven-aged nature, and variable understory - using data collected by a low-cost, portable TLS system, the Compact Biomass Lidar (CBL). The objectives of this work are listed as follow: 1. Assess the utility of terrestrial lidar scanning (TLS) to accurately map elevation changes (sediment accretion rates) in mangrove forest; 2. Evaluate forest structural attributes, e.g., stems and roots, in complex forest environments toward biophysical characterization of such forests; and 3. Assess canopy-level structural traits (leaf area index; leaf area density) in complex forest environments to estimate biomass in rapidly changing environments. The low-cost system used in this research provides lower-resolution data, in terms of scan angular resolution and resulting point density, when compared to higher-cost commercial systems. As a result, the algorithms developed for evaluating the data collected by such systems should be robust to issues caused by low-resolution 3D point cloud data. The data used in various parts of this work were collected from three mangrove forests on the western Pacific island of Pohnpei in the Federated States of Micronesia, as well as tropical forests in Hawai'i, USA. Mangrove forests underscore the economy of this region, where more than half of the annual household income is derived from these forests. However, these mangrove forests are endangered by sea level rise, which necessitates an evaluation of the resilience of mangrove forests to climate change in order to better protect and manage these ecosystems. This includes the preservation of positive sediment accretion rates, and stimulating the process of root growth, sedimentation, and peat development, all of which are influenced by the forest floor elevation, relative to sea level. Currently, accretion rates are measured using surface elevation tables (SETs), which are posts permanently placed in mangrove sediments. The forest floor is measured annually with respect to the height of the SETs to evaluate changes in elevation (Cahoon et al. 2002). In this work, we evaluated the ability of the CBL system for measuring such elevation changes, to address objective #1. Digital Elevation Models (DEMs) were produced for plots, based on the point cloud resulted from co-registering eight scans, spaced 45 degree, per plot. DEMs are refined and produced using Cloth Simulation Filtering (CSF) and kriging interpolation. CSF was used because it minimizes the user input parameters, and kriging was chosen for this study due its consideration of the overall spatial arrangement of the points using semivariogram analysis, which results in a more robust model. The average consistency of the TLS-derived elevation change was 72%, with and RMSE value of 1.36 mm. However, what truly makes the TLS method more tenable, is the lower standard error (SE) values when compared to manual methods (10-70x lower). In order to achieve our second objective, we assessed structural characteristics of the above-mentioned mangrove forest and also for tropical forests in Hawaii, collected with the same CBL scanner. The same eight scans per plot (20 plots) were co-registered using pairwise registration and the Iterative Closest Point (ICP). We then removed the higher canopy using a normal change rate assessment algorithm. We used a combination of geometric classification techniques, based on the angular orientation of the planes fitted to points (facets), and machine learning 3D segmentation algorithms to detect tree stems and above-ground roots. Mangrove forests are complex forest environments, containing above-ground root mass, which can create confusion for both ground detection and structural assessment algorithms. As a result, we needed to train a supporting classifier on the roots to detect which root lidar returns were classified as stems. The accuracy and precision values for this classifier were assessed via manual investigation of the classification results in all 20 plots. The accuracy and precision for stem classification were found to be 82% and 77%, respectively. The same values for root detection were 76% and 68%, respectively. We simulated the stems using alpha shapes in order to assess their volume in the final step. The consistency of the volume evaluation was found to be 85%. This was obtained by comparing the mean stem volume (m3/ha) from field data and the TLS data in each plot. The reported accuracy is the average value for all 20 plots. Additionally, we compared the diameter-at-breast-height (DBH), recorded in the field, with the TLS-derived DBH to obtain a direct measure of the precision of our stem models. DBH evaluation resulted in an accuracy of 74% and RMSE equaled 7.52 cm. This approach can be used for automatic stem detection and structural assessment in a complex forest environment, and could contribute to biomass assessment in these rapidly changing environments. These stem and root structural assessment efforts were complemented by efforts to estimate canopy-level structural attributes of the tropical Hawai'i forest environment; we specifically estimated the leaf area index (LAI), by implementing a density-based approach. 242 scans were collected using the portable low-cost TLS (CBL), in a Hawaii Volcano National Park (HAVO) flux tower site. LAI was measured for all the plots in the site, using an AccuPAR LP-80 Instrument. The first step in this work involved detection of the higher canopy, using normal change rate assessment. After segmenting the higher canopy from the lidar point clouds, we needed to measure Leaf Area Density (LAD), using a voxel-based approach. We divided the canopy point cloud into five layers in the Z direction, after which each of these five layers were divided into voxels in the X direction. The sizes of these voxels were constrained based on interquartile analysis and the number of points in each voxel. We hypothesized that the power returned to the lidar system from woody materials, like branches, exceeds that from leaves, due to the liquid water absorption of the leaves and higher reflectivity for woody material at the 905 nm lidar wavelength. We evaluated leafy and woody materials using images from projected point clouds and determined the density of these regions to support our hypothesis. The density of points in a 3D grid size of 0.1 m, which was determined by investigating the size of the branches in the lower portion of the higher canopy, was calculated in each of the voxels. Note that "density" in this work is defined as the total number of points per grid cell, divided by the volume of that cell. Subsequently, we fitted a kernel density estimator to these values. The threshold was set based on half of the area under the curve in each of the distributions. The grid cells with a density below the threshold were labeled as leaves, while those cells with a density above the threshold were set as non-leaves. We then modeled the LAI using the point densities derived from TLS point clouds, achieving a R2 value of 0.88. We also estimated the LAI directly from lidar data by using the point densities and calculating leaf area density (LAD), which is defined as the total one-sided leaf area per unit volume. LAI can be obtained as the sum of the LAD values in all the voxels. The accuracy of LAI estimation was found to be 90%. Since the LAI values cannot be considered spatially independent throughout all the plots in this site, we performed a semivariogram analysis on the field-measured LAI data. This analysis showed that the LAI values can be assumed to be independent in plots that are at least 30 m apart. As a result, we divided the data into six subsets, where each of the plots were 30 meter spaced for each subset. LAI model R2 values for these subsets ranged between 0.84 - 0.96. The results bode well for using this method for automatic estimation of LAI values in complex forest environments, using a low-cost, low point density, rapid-scan TLS.
Publisher Site: RIT Repository
PDF (Requires Sign-In): PDF
Click for Bibtex
@book{rouzbeh kargar2020on, title = {On the Use of Rapid-Scan, Low Point Density Terrestrial Laser Scanning (TLS) for Structural Assessment of Complex Forest Environments}, author = {Rouzbeh Kargar, Ali}, year = {2020}, school = {Rochester Institute of Technology} }
[D-2020-12] Mahshad Mahdavi. (2020). “Query-Driven Global Graph Attention Model for Visual Parsing: Recognizing Handwritten and Typeset Math Formulas.” Rochester Institute of Technology.
Abstract: We present a new visual parsing method based on standard Convolutional Neural Networks (CNNs) for handwritten and typeset mathematical formulas. The Query-Driven Global Graph Attention (QD-GGA) parser employs multi-task learning, using a single feature representation for locating, classifying, and relating symbols. QD-GGA parses formulas by first constructing a Line-Of-Sight (LOS) graph over the input primitives (e.g handwritten strokes or connected components in images). Second, class distributions for LOS nodes and edges are obtained using query-specific feature filters (i.e., attention) in a single feed-forward pass. This allows end-to-end structure learning using a joint loss over primitive node and edge class distributions. Finally, a Maximum Spanning Tree (MST) is extracted from the weighted graph using Edmonds' Arborescence Algorithm. The model may be run recurrently over the input graph, updating attention to focus on symbols detected in the previous iteration. QD-GGA does not require additional grammar rules and the language model is learned from the sets of symbols/relationships and the statistics over them in the training set. We benchmark our system against both handwritten and typeset state-of-the-art math recognition systems. Our preliminary results show that this is a promising new approach for visual parsing of math formulas. Using recurrent execution, symbol detection is near perfect for both handwritten and typeset formulas: we obtain a symbol f-measure of over 99.4% for both the CROHME (handwritten) and INFTYMCCDB-2 (typeset formula image) datasets. Our method is also much faster in both training and execution than state-of-the-art RNN-based formula parsers. The unlabeled structure detection of QDGGA is competitive with encoder-decoder models, but QD-GGA symbol and relationship classification is weaker. We believe this may be addressed through increased use of spatial features and global context.
Publisher Site: RIT Repository
PDF (Requires Sign-In): PDF
Click for Bibtex
@book{mahdavi2020query-driven, title = {Query-Driven Global Graph Attention Model for Visual Parsing: Recognizing Handwritten and Typeset Math Formulas}, author = {Mahdavi, Mahshad}, year = {2020}, school = {Rochester Institute of Technology} }
[D-2020-11] Michal Kucer. (2020). “Representations and Representation Learning for Image Aesthetics Prediction and Image Enhancement.” Rochester Institute of Technology.
Abstract: With the continual improvement in cell phone cameras and improvements in the connectivity of mobile devices, we have seen an exponential increase in the images that are captured, stored and shared on social media. For example, as of July 1st 2017 Instagram had over 715 million registered users which had posted just shy of 35 billion images. This represented approximately seven and nine-fold increase in the number of users and photos present on Instagram since 2012. Whether the images are stored on personal computers or reside on social networks (e.g. Instagram, Flickr), the sheer number of images calls for methods to determine various image properties, such as object presence or appeal, for the purpose of automatic image management and curation. One of the central problems in consumer photography centers around determining the aesthetic appeal of an image and motivates us to explore questions related to understanding aesthetic preferences, image enhancement and the possibility of using such models on devices with constrained resources. In this dissertation, we present our work on exploring representations and representation learning approaches for aesthetic inference, composition ranking and its application to image enhancement. Firstly, we discuss early representations that mainly consisted of expert features, and their possibility to enhance Convolutional Neural Networks (CNN). Secondly, we discuss the ability of resource-constrained CNNs, and the different architecture choices (inputs size and layer depth) in solving various aesthetic inference tasks: binary classification, regression, and image cropping. We show that if trained for solving fine-grained aesthetics inference, such models can rival the cropping performance of other aesthetics-based croppers, however they fall short in comparison to models trained for composition ranking. Lastly, we discuss our work on exploring and identifying the design choices in training composition ranking functions, with the goal of using them for image composition enhancement.
Publisher Site: RIT Repository
PDF (Requires Sign-In): PDF
Click for Bibtex
@book{kucer2020representations, title = {Representations and Representation Learning for Image Aesthetics Prediction and Image Enhancement}, author = {Kucer, Michal}, year = {2020}, school = {Rochester Institute of Technology} }
[D-2020-10] Rounak Singh Narde. (2020). “Millimeter-wave Interconnects for Intra- and Inter-chip Transmission and Beam Steering in NoC-based Multi-chip Systems.” Rochester Institute of Technology.
Abstract: The primary objective of this work is to investigate the communication capabilities of short-range millimeter-wave (mm-wave) communication among Network-on-Chip (NoC) based multi-core processors integrated on a substrate board. To address the demand for high-performance multi-chip computing systems, the present work studies the transmission coefficients between the on-chip antennas system for both intra- and inter-chip communication. It addresses techniques for enhancing transmission by using antenna arrays for beamforming. It also explores new and creative solutions to minimize the adverse effects of silicon on electromagnetic wave propagation using artificial magnetic conductors (AMC). The following summarizes the work performed and future work. Intra- and inter-chip transmission between wireless interconnects implemented as antennas on-chip (AoC), in a wire-bonded chip package are studied 30GHz and 60 GHz. The simulations are performed in ANSYS HFSS, which is based on the finite element method (FEM), to study the transmission and to analyze the electric field distribution. Simulation results have been validated with fabricated antennas at 30 GHz arranged in different orientations on silicon dies that can communicate with inter-chip transmission coefficients ranging from -45dB to -60dB while sustaining bandwidths up to 7GHz. The fabricated antennas show a shift in the resonant frequency to 25GHz. This shift is attributed to the Ground-Signal-Ground (GSG) probes used for measurement and to the Short-Open-Load (SOLT) calibration which has anomalies at millimeter-wave frequencies. Using measurements, a large-scale log-normal channel model is derived which can be used for system-level architecture design. Further, at 60 GHz densely packed multilayer copper wires in NoCs have been modeled to study their impact on the wireless transmission between antennas for both intra- and inter-chip links and are shown to be equivalent to copper sheets. It is seen that the antenna radiation efficiency reduces in the presence of these densely packed wires placed close to the antenna elements. Using this model, the reduction of inter-chip transmission is seen to be about 20dB as compared to a system with no wires. Lastly, the transmission characteristics of the antennas resonating at 60GHz in a flip-chip packaging environment are also presented.
Publisher Site: RIT Repository
PDF (Requires Sign-In): PDF
Click for Bibtex
@book{narde2020millimeter-wave, title = {Millimeter-wave Interconnects for Intra- and Inter-chip Transmission and Beam Steering in NoC-based Multi-chip Systems}, author = {Narde, Rounak Singh}, year = {2020}, school = {Rochester Institute of Technology} }
[D-2020-09] Daniel Wysocki. (2020). “Measuring the Population Properties of Merging Compact Binaries with Gravitational Wave Observations.” Rochester Institute of Technology.
Abstract: Since the Laser Interferometer Gravitational-Wave Observatory (LIGO) made the first direct detection of gravitational waves in 2015, the era of gravitational wave astronomy has begun. LIGO and its counterpart Virgo are detecting an ever-growing sample of merging compact binaries: binary black holes, binary neutron stars, and neutron star--black hole binaries. Each individual detection can be compared against simulated signals with known properties, in order to measure the binary's properties. In order to understand the sample of detections as a whole, however, ensemble methods are needed. The properties measured from these binary systems have large measurement errors, and the sensitivity of gravitational wave detectors are highly property-dependent, resulting in large selection biases. This dissertation applies the technique of hierarchical Bayesian modeling in order to constrain the underlying, unbiased population of merging compact binaries. We use a number of models to constrain the merger rate, mass distribution, and spin distribution for binary black holes and binary neutron stars. We also use tidal information present in binary neutron stars in order to self-consistently constrain the nuclear equation of state.
Publisher Site: RIT Repository
PDF (Requires Sign-In): PDF
Click for Bibtex
@book{wysocki2020measuring, title = {Measuring the Population Properties of Merging Compact Binaries with Gravitational Wave Observations}, author = {Wysocki, Daniel}, year = {2020}, school = {Rochester Institute of Technology} }
[D-2020-08] Ryne Roady. (2020). “Open Set Classification for Deep Learning in Large-Scale and Continual Learning Models.” Rochester Institute of Technology.
Abstract: Supervised classification methods often assume the train and test data distributions are the same and that all classes in the test set are present in the training set. However, deployed classifiers require the ability to recognize inputs from outside the training set as unknowns and update representations in near real-time to account for novel concepts unknown during offline training. This problem has been studied under multiple paradigms including out-of-distribution detection and open set recognition; however, for convolutional neural networks, there have been two major approaches: 1) inference methods to separate known inputs from unknown inputs and 2) feature space regularization strategies to improve model robustness to novel inputs. In this dissertation, we explore the relationship between the two approaches and directly compare performance on large-scale datasets that have more than a few dozen categories. Using the ImageNet large-scale classification dataset, we identify novel combinations of regularization and specialized inference methods that perform best across multiple open set classification problems of increasing difficulty level. We find that input perturbation and temperature scaling yield significantly better performance on large-scale datasets than other inference methods tested, regardless of the feature space regularization strategy. Conversely, we also find that improving performance with advanced regularization schemes during training yields better performance when baseline inference techniques are used; however, this often requires supplementing the training data with additional background samples which is difficult in large-scale problems. To overcome this problem we further propose a simple regularization technique that can be easily applied to existing convolutional neural network architectures that improves open set robustness without the requirement for a background dataset. Our novel method achieves state-of-the-art results on open set classification baselines and easily scales to large-scale problems. Finally, we explore the intersection of open set and continual learning to establish baselines for the first time for novelty detection while learning from online data streams. To accomplish this we establish a novel dataset created for evaluating image open set classification capabilities of streaming learning algorithms. Finally, using our new baselines we draw conclusions as to what the most computationally efficient means of detecting novelty in pre-trained models and what properties of an efficient open set learning algorithm operating in the streaming paradigm should possess.
Publisher Site: RIT Repository
PDF (Requires Sign-In): PDF
Click for Bibtex
@book{roady2020open, title = {Open Set Classification for Deep Learning in Large-Scale and Continual Learning Models}, author = {Roady, Ryne}, year = {2020}, school = {Rochester Institute of Technology} }
[D-2020-07] Abdullah M. Zyarah. (2020). “Energy Efficient Neocortex-Inspired Systems with On-Device Learning.” Rochester Institute of Technology.
Abstract: Shifting the compute workloads from cloud toward edge devices can significantly improve the overall latency for inference and learning. On the contrary this paradigm shift exacerbates the resource constraints on the edge devices. Neuromorphic computing architectures, inspired by the neural processes, are natural substrates for edge devices. They offer co-located memory, in-situ training, energy efficiency, high memory density, and compute capacity in a small form factor. Owing to these features, in the recent past, there has been a rapid proliferation of hybrid CMOS/Memristor neuromorphic computing systems. However, most of these systems offer limited plasticity, target either spatial or temporal input streams, and are not demonstrated on large scale heterogeneous tasks. There is a critical knowledge gap in designing scalable neuromorphic systems that can support hybrid plasticity for spatio-temporal input streams on edge devices. This research proposes Pyragrid, a low latency and energy efficient neuromorphic computing system for processing spatio-temporal information natively on the edge. Pyragrid is a full-scale custom hybrid CMOS/Memristor architecture with analog computational modules and an underlying digital communication scheme. Pyragrid is designed for hierarchical temporal memory, a biomimetic sequence memory algorithm inspired by the neocortex. It features a novel synthetic synapses representation that enables dynamic synaptic pathways with reduced memory usage and interconnects. The dynamic growth in the synaptic pathways is emulated in the memristor device physical behavior, while the synaptic modulation is enabled through a custom training scheme optimized for area and power. Pyragrid features data reuse, in-memory computing, and event-driven sparse local computing to reduce data movement by ~44x and maximize system throughput and power efficiency by ~3x and ~161x over custom CMOS digital design. The innate sparsity in Pyragrid results in overall robustness to noise and device failure, particularly when processing visual input and predicting time series sequences. Porting the proposed system on edge devices can enhance their computational capability, response time, and battery life.
Publisher Site: RIT Repository
PDF (Requires Sign-In): PDF
Click for Bibtex
@book{zyarah2020energy, title = {Energy Efficient Neocortex-Inspired Systems with On-Device Learning}, author = {Zyarah, Abdullah M.}, year = {2020}, school = {Rochester Institute of Technology} }
[D-2020-06] David E. Narváez. (2020). “Constraint Satisfaction Techniques for Combinatorial Problems.” Rochester Institute of Technology.
Abstract: The last two decades have seen extraordinary advances in tools and techniques for constraint satisfaction. These advances have in turn created great interest in their industrial applications. As a result, tools and techniques are often tailored to meet the needs of industrial applications out of the box. We claim that in the case of abstract combinatorial problems in discrete mathematics, the standard tools and techniques require special considerations in order to be applied effectively. The main objective of this thesis is to help researchers in discrete mathematics weave through the landscape of constraint satisfaction techniques in order to pick the right tool for the job. We consider constraint satisfaction paradigms like satisfiability of Boolean formulas and answer set programming, and techniques like symmetry breaking. Our contributions range from theoretical results to practical issues regarding tool applications to combinatorial problems. We prove search-versus-decision complexity results for problems about backbones and backdoors of Boolean formulas. We consider applications of constraint satisfaction techniques to problems in graph arrowing (specifically in Ramsey and Folkman theory) and computational social choice. Our contributions show how applying constraint satisfaction techniques to abstract combinatorial problems poses additional challenges. We show how these challenges can be addressed. Additionally, we consider the issue of trusting the results of applying constraint satisfaction techniques to combinatorial problems by relying on verified computations.
Publisher Site: RIT Repository
PDF (Requires Sign-In): PDF
Click for Bibtex
@book{narváez2020constraint, title = {Constraint Satisfaction Techniques for Combinatorial Problems}, author = {Narváez, David E.}, year = {2020}, school = {Rochester Institute of Technology} }
[D-2020-05] Golnaz Jalalahmadi. (2020). “Design of a Comprehensive Modeling, Characterization, Rupture Risk Assessment and Visualization Pipeline for Abdominal Aortic Aneurysms.” Rochester Institute of Technology.
Abstract: Abdominal aortic aneurysms (AAA) is a dilation of the abdominal aorta, typically within the infra-renal segment of the vessel that cause an expansion of at least 1.5 times the normal vessel diameter. It is becoming a leading cause of death in the United States and around the world, and consequentially, in 2009, the Society for Vascular Surgery (SVS) practice guidelines expressed the critical need to further investigate the factors associated with the risk of AAA rupture, along with potential treatment methods. For decades, the maximum diameter (Dmax) was introduced as the main parameter used to assess AAA behavior and its rupture risk. However, it has been shown that three main categories of parameters including geometrical indices, such as the maximum transverse diameter, biomechanical parameters, such as material properties, and historical clinical parameters, such as age, gender, hereditary history and life-style affect AAA and its rupture risk. Therefore, despite all efforts that have been undertaken to study the relationship among different parameters affecting AAA and its rupture, there are still limitations that require further investigation and modeling; the challenges associated with the traditional, clinical quality images represent one class of these limitations. The other limitation is the use of the homogenous hyper-elastic material property model to study the entire AAA, when, in fact, there is evidence that different degrees of degradation of the elastin and collagen network of the AAA wall lead to different regions of the AAA exhibiting different material properties, which, in turn, affect its biomechanical behavior and rupture. Moreover, the effects of all three main categories of parameters need to be considered simultaneously and collectively when studying the AAAs and their rupture, so once again, the field can further benefit from such studies. Therefore, in this work, we describe a comprehensive pipeline consisting of three main components to overcome some of these existing limitations. The first component of the proposed method focuses on the reconstruction and analysis of both synthetic and human subject-specific 3D models of AAA, accompanied by a full geometric parameter analysis and their effects on wall stress and peak wall stress. The second component investigates the effect of various biomechanical parameters, specifically the use of various homogeneous and heterogeneous material properties to model the behavior of the AAA wall. To this extent, we introduce two different patient-specific regional material property models to better mimic the physiological behavior of the AAA wall. Finally, the third component utilizes machine learning methods to develop a comprehensive predictive model that incorporates the effect of the geometrical, biomechanical and historical clinical data to predict the rupture severity of AAA in a patient-specific manner. This is the first comprehensive semi-automated method developed for the assessment of AAA. Our findings illustrate that using a regional material property model that mimics the realistic heterogeneity of the vessel's wall leads to more reliable and accurate predictions of AAA severity and associated rupture risk. Additionally, our results indicate that using only Dmax as an indicator for the rupture risk is insufficient, while a combination of parameters from different sources along with PWS could serve as a more reliable rupture assessment. These methods can help better characterize the severity of AAAs, better predict their associated rupture risk, and, in turn, help clinicians with earlier, patient-customized diagnosis and patient-customized treatment planning approaches, such as stent grafting.
Publisher Site: RIT Repository
PDF (Requires Sign-In): PDF
Click for Bibtex
@book{jalalahmadi2020design, title = {Design of a Comprehensive Modeling, Characterization, Rupture Risk Assessment and Visualization Pipeline for Abdominal Aortic Aneurysms}, author = {Jalalahmadi, Golnaz}, year = {2020}, school = {Rochester Institute of Technology} }
[D-2020-04] Jonathan Miller. (2020). “Low Cost Radiometer Design for Landsat Surface Temperature Validation.” Rochester Institute of Technology.
Abstract: Land Surface Temperature (ST) represents the radiative temperature of the Earth's surface and is used as an input for hydrological, agricultural, and meteorological science applications. Due to the synoptic nature of satellite imaging systems, ST products derived from spaceborne platforms are invaluable for estimating ST at the local, regional, and global scale. Over the past two decades, an emphasis has been placed on the need to develop algorithms necessary to deliver accurate ST products to support the needs of science users. However, corresponding efforts to validate these products are hindered by the availability of quality ground based reference measurements. NOAA's Surface Radiation Budget Network (SURFRAD) is commonly used to support ST-validation efforts, but SURFAD's instrumentation is broadband (4-50 micrometer) and several of their sites lack spatial uniformity, which can lead to large ST calculation errors. To address the apparent deficiencies within existing validation networks, this work discusses a prototype instrument developed to provide ST estimates to support validation efforts for spaceborne thermal sensor products. Specifically, a prototype radiometer was designed, built, calibrated, and utilized to acquire ground reference data to validate ST product(s) derived from Landsat 8 imagery. Field based efforts indicate these radiometers demonstrate agreement to Landsat-derived ST products to within 1.37 K over grass targets. This is an improvement of over 2 K when comparing to the SURFRAD validation network. Additionally, the radiometers proposed in this research were designed to calculate the largest unknown variable used to create Landsat 8 derived ST products: the target emissivity. Algorithms have been developed with the purpose of using Landsat 8's two thermal bands to calculate the ST of a given scene. One popular method is the split window algorithm, which uses at sensor apparent temperatures collected by band 10 and 11 of Landsat, along with atmospheric data to calculate the surface leaving temperatures. A key input into the split window algorithm is the emissivity of the target, which is currently calculated using data from another spaceborne sensor; the Advanced Spaceborne Thermal Emission and Reflection Radiometer (ASTER) data from EOS-1 (Terra). This emissivity calculation is not ideal because, like the ST calculation, the emissivity is also propagated through the atmosphere before being calculated. An ideal approach is to measure and calculate the emissivity close to the surface, thus eliminating any atmospheric compensation errors. To eliminate the reliance on ASTER data and calculate the emissivity of the target before atmospheric effects, four additional response bands in the 8 – 9 micrometer range are added to the radiometer resulting in a six band instrument capable of calculating the ST and emissivity of a ground target. Through a validation effort using a commercial Fourier Transform Infrared Spectrometer (FTIR) the six-band radiometer's ability to calculate the emissivity of a target is in agreement to the FTIR derived emissivity values to within 0.025 for Landsat-like band 10 and 0.022 for Landsat-like band 11 over grass targets. More accurate target emissivity values can decrease the error in the split window ST calculation by as much as 2.4 K over grass targets. The proposed instruments in this research can provide a more accurate validation of the Landsat 8 ST product when comparing to current validation networks, and therefore more accurate target emissivity values. Combining the two capabilities of this proposed radiometer allows the delivery of trusted data to the scientific community for their use in multiple applications.
Publisher Site: RIT Repository
PDF (Requires Sign-In): PDF
Click for Bibtex
@book{miller2020low, title = {Low Cost Radiometer Design for Landsat Surface Temperature Validation}, author = {Miller, Jonathan}, year = {2020}, school = {Rochester Institute of Technology} }
[D-2020-03] AbdElRahman A. ElSaid. (2020). “Nature-Inspired Topology Optimization of Recurrent Neural Networks.” Rochester Institute of Technology.
Abstract: Hand-crafting effective and efficient structures for recurrent neural networks (RNNs) is a difficult, expensive, and time-consuming process. To address this challenge, this work presents three nature-inspired (NI) algorithms for neural architecture search (NAS), introducing the subfield of nature-inspired neural architecture search (NI-NAS). These algorithms, based on ant colony optimization (ACO), progress from memory cell structure optimization, to bounded discrete-space architecture optimization, and finally to unbounded continuous-space architecture optimization. These methods were applied to real-world data sets representing challenging engineering problems, such as data from a coal-fired power plant, wind-turbine power generators, and aircraft flight data recorder (FDR) data. Initial work utilized ACO to select optimal connections inside recurrent long short-term memory (LSTM) cell structures. Viewing each LSTM cell as a graph, ants would choose potential input and output connections based on the pheromones previously laid down over those connections as done in a standard ACO search. However, this approach did not optimize the overall network of the RNN, particularly its synaptic parameters. I addressed this issue by introducing the Ant-based Neural Topology Search (ANTS) algorithm to directly optimize the entire RNN topology. ANTS utilizes a discrete-space superstructure representing a completely connected RNN where each node is connected to every other node, forming an extremely dense mesh of edges and recurrent edges. ANTS can select from a library of modern RNN memory cells. ACO agents (ants), in this thesis, build RNNs from the superstructure determined by pheromones laid out on the superstructure's connections. Backpropagation is then used to train the generated RNNs in an asynchronous parallel computing design to accelerate the optimization process. The pheromone update depends on the evaluation of the tested RNN against a population of best performing RNNs. Several variations of the core algorithm was investigated to test several designed heuristics for ANTS and evaluate their efficacy in the formation of sparser synaptic connectivity patterns. This was done primarily by formulating different functions that drive the underlying pheromone simulation process as well as by introducing ant agents with 3 specialized roles (inspired by real-world ants) to construct the RNN structure. This characterization of the agents enables ants to focus on specific structure building roles. ``Communal intelligence'' was also incorporated, where the best set of weights was across locally-trained RNN candidates for weight initialization, reducing the number of backpropagation epochs required to train each candidate RNN and speeding up the overall search process. However, the growth of the superstructure increased by an order of magnitude, as more input and deeper structures are utilized, proving to be one limitation of the proposed procedure. The limitation of ANTS motivated the development of the continuous ANTS algorithm (CANTS), which works with a continuous search space for any fixed network topology. In this process, ants moving within a (temporally-arranged) set of continuous/real-valued planes based on proximity and density of pheromone placements. The motion of the ants over these continuous planes, in a sense, more closely mimicks how actual ants move in the real world. Ants traverse a 3-dimensional space from the inputs to the outputs and across time lags. This continuous search space frees the ant agents from the limitations imposed by ANTS' discrete massively connected superstructure, making the structural options unbounded when mapping the movements of ants through the 3D continuous space to a neural architecture graph. In addition, CANTS has fewer hyperparameters to tune than ANTS, which had five potential heuristic components that each had their own unique set of hyperparameters, as well as requiring the user to define the maximum recurrent depth, number of layers and nodes within each layer. CANTS only requires specifying the number ants and their pheromone sensing radius. The three applied strategies yielded three important successes. Applying ACO on optimizing LSTMs yielded a 1.34\% performance enhancement and more than 55% sparser structures (which is useful for speeding up inference). ANTS outperformed the NAS benchmark, NEAT, and the NAS state-of-the-art algorithm, EXAMM. CANTS showed competitive results to EXAMM and competed with ANTS while offering sparser structures, offering a promising path forward for optimizing (temporal) neural models with nature-inspired metaheuristics based the metaphor of ants.
Publisher Site: RIT Repository
PDF (Requires Sign-In): PDF
Click for Bibtex
@book{elsaid2020nature-inspired, title = {Nature-Inspired Topology Optimization of Recurrent Neural Networks}, author = {ElSaid, AbdElRahman A.}, year = {2020}, school = {Rochester Institute of Technology} }
[D-2020-02] Tania Kleynhans. (2020). “Automatic Pigment Classification in Painted Works of Art from Diffuse Reflectance Image Data.” Rochester Institute of Technology.
Abstract: Information about artists' materials used in paintings, obtained from the analysis of limited micro-samples, has assisted conservators to better define treatment plans, and provided scholars with basic information about the working methods of the artists. Recently, macro-scale imaging systems such as visible-to-near infrared (VNIR) reflectance hyperspectral imaging (HSI) are being used to provide conservators and art historians with a more comprehensive understanding of a given work of art. However, the HSI analysis process has not been streamlined and currently requires significant manual input by experts. Additionally, HSI systems are often too expensive for small to mid-level museums. This research focused on three main objectives: 1) adapt existing algorithms developed for remote sensing applications to automatically create classification and abundance maps to significantly reduce the time to analyze a given artwork, 2) create an end-to-end pigment identification convolutional neural network to produce pigment maps that may be used directly by conservation scientists without further analysis, and 3) propose and model the expected performance of a low-cost fiber optic single point multispectral system that may be added to the scanning tables already part of many museum conservation laboratories. Algorithms developed for both classification and pigment maps were tested on HSI data collected from various illuminated manuscripts. Results demonstrate the potential of both developed processes. Band selection studies indicates that pigment identification from a small number of bands produces similar results to that of the HSI data sets on a selected number of test artifacts. A system level analysis of the proposed system was conducted with a detailed radiometric model. The system trade study confirmed the viability of using either individual spectral filters or a linear variable filter set-up to collect multispectral data for pigment identification of works of art.
Publisher Site: RIT Repository
PDF (Requires Sign-In): PDF
Click for Bibtex
@book{kleynhans2020automatic, title = {Automatic Pigment Classification in Painted Works of Art from Diffuse Reflectance Image Data}, author = {Kleynhans, Tania}, year = {2020}, school = {Rochester Institute of Technology} }
[D-2020-01] Matt Ronnenberg. (2020). “Evaluating Appearance Differences of Color 3D Printed Objects.” Rochester Institute of Technology.
Abstract: Color 3D printing is a relatively young technology with several exciting applications and challenges yet to be explored. One of those challenges is the effect that three dimensional surface geometries have on appearance. The appearance of 3D objects is complex and can be affected by the interaction between several visual appearance parameters such as color, gloss and surface texture. Since traditional printing is only 2D, several of these challenges have either been solved or never needed to be addressed. Complicating matters further, different color 3D printing technologies and materials come with their own inherent material appearance properties, necessitating the study of these appearance parameters on an individual case by case basis. Neural networks are powerful tools that are finding their way into just about every field imaginable, and the world of color science is no exception. A process described by previous researchers provides a method for picking out color sensitive neurons in a given layer of a convolutional neural network (CNN). Typically, CNNs are used for image classification but can also be used for image comparison. A siamese CNN was built and shown to be a good model for appearance differences using textured color patches designed to simulate the appearance of color 3D printed objects. A direct scaling psychophysical experiment was done to create an interval scale of perceptual appearance between color 3D printed objects printed at different angles. The objects used for this experiment were printed with an HP® Jet Fusion 580 color 3D printer. The objects exhibit print angle dependent surface textures inherent to the layered printing process itself. The preliminary siamese CNN showed that perceptual differences in the prints were likely to exist and could be modeled using a neural network. However, the results of the psychophysical experiment indicated that CIELAB color differences were extremely strong predictors of observer perceptions, even with variable surface texture in uncontrolled lighting conditions.
Publisher Site: RIT Repository
PDF (Requires Sign-In): PDF
Click for Bibtex
@book{ronnenberg2020evaluating, title = {Evaluating Appearance Differences of Color 3D Printed Objects}, author = {Ronnenberg, Matt}, year = {2020}, school = {Rochester Institute of Technology} }
[D-2019-28] Xuewen Zhang. (2019). “Efficient Nonlinear Dimensionality Reductionfor Pixel-Wise Classification of Hyperspectral Imagery.” Rochester Institute of Technology.
Abstract: Classification, target detection, and compression are all important tasks in analyzing hyperspectral imagery (HSI). Because of the high dimensionality of HSI, it is often useful to identify low-dimensional representations of HSI data that can be used to make analysis tasks tractable. Traditional linear dimensionality reduction (DR) methods are not adequate due to the nonlinear distribution of HSI data. Many nonlinear DR methods, which are successful in the general data processing domain, such as Local Linear Embedding (LLE) [1], Isometric Feature Mapping (ISOMAP) [2] and Kernel Principal Components Analysis (KPCA) [3], run very slowly and require large amounts of memory when applied to HSI. For example, applying KPCA to the 512x217 pixel, 204-band Salinas image using a modern desktop computer (AMD FX-6300 Six-Core Processor, 32 GB memory) requires more than 5 days of computing time and 28GB memory! In this thesis, we propose two different algorithms for significantly improving the computational efficiency of nonlinear DR without adversely affecting the performance of classification task: Simple Linear Iterative Clustering (SLIC) superpixels and semi-supervised deep autoencoder networks (SSDAN). SLIC is a very popular algorithm developed for computing superpixels in RGB images that can easily be extended to HSI. Each superpixel includes hundreds or thousands of pixels based on spatial and spectral similarities and is represented by the mean spectrum and spatial position of all of its component pixels. Since the number of superpixels is much smaller than the number of pixels in the image, they can be used as input for nonlinearDR, which significantly reduces the required computation time and memory versus providing all of the original pixels as input. After nonlinear DR is performed using superpixels as input, an interpolation step can be used to obtain the embedding of each original image pixel in the low dimensional space. To illustrate the power of using superpixels in an HSI classification pipeline,we conduct experiments on three widely used and publicly available hyperspectral images: Indian Pines, Salinas and Pavia. The experimental results for all three images demonstrate that for moderately sized superpixels, the overall accuracy of classification using superpixel-based nonlinear DR matches and sometimes exceeds the overall accuracy of classification using pixel-based nonlinear DR, with a computational speed that is two-three orders of magnitude faster. Even though superpixel-based nonlinear DR shows promise for HSI classification, it does have disadvantages. First, it is costly to perform out-of-sample extensions. Second, it does not generalize to handle other types of data that might not have spatial information. Third, the original input pixels cannot approximately be recovered, as is possible in many DR algorithms.In order to overcome these difficulties, a new autoencoder network - SSDAN is proposed. It is a fully-connected semi-supervised autoencoder network that performs nonlinear DR in a manner that enables class information to be integrated. Features learned from SSDAN will be similar to those computed via traditional nonlinear DR, and features from the same class will be close to each other. Once the network is trained well with training data, test data can be easily mapped to the low dimensional embedding. Any kind of data can be used to train a SSDAN,and the decoder portion of the SSDAN can easily recover the initial input with reasonable loss.Experimental results on pixel-based classification in the Indian Pines, Salinas and Pavia images show that SSDANs can approximate the overall accuracy of nonlinear DR while significantly improving computational efficiency. We also show that transfer learning can be use to finetune features of a trained SSDAN for a new HSI dataset. Finally, experimental results on HSI compression show a trade-off between Overall Accuracy (OA) of extracted features and PeakSignal to Noise Ratio (PSNR) of the reconstructed image.
Publisher Site: RIT Repository
PDF (Requires Sign-In): PDF
Click for Bibtex
@book{zhang2019efficient, title = {Efficient Nonlinear Dimensionality Reductionfor Pixel-Wise Classification of Hyperspectral Imagery}, author = {Zhang, Xuewen}, year = {2019}, school = {Rochester Institute of Technology} }
[D-2019-27] Kevan Donlon. (2019). “On Interpixel Capacitive Coupling in Hybridized HgCdTe Arrays: Theory, Characterization and Correction.” Rochester Institute of Technology.
Abstract: Hybridization is a process by which detector arrays and read out circuitry can be independently fabricated and then bonded together, typically using indium bumps. This technique allows for the use of exotic detector materials such as HgCdTe for the desired spectral response while benefiting from established and proven silicon CMOS readout structures. However, the introduction of an intermediate layer composed of conductors (indium) and insulators (epoxy) results in a capacitive interconnect between adjacent pixels. This interpixel capacitance (IPC) results in charge collected on one pixel, giving rise to a change in voltage on the output node of adjacent pixels. In imaging arrays, this capacitance manifests itself as a blur, attenuating high spatial frequency information and causing single pixel events to be spread over a local neighborhood. Due to the nature of the electric fields in proximity to the depletion region of the diodes in the detector array, the magnitude of this capacitance changes as the diode depletes. This change in capacitance manifests itself as a change in fractional coupling. This results in a blur kernel that is non-homogeneous both spatially across the array and temporally from exposure to exposure, varying as a function of charge collected in each pixel. This signal dependent behavior invalidates underlying assumptions key for conventional deconvolution/deblurring techniques such as Weiner filtering or Lucy-Richardson deconvolution. As such, these techniques cannot be relied upon to restore scientific accuracy and appropriately solve this inverse problem. This dissertation uses first principle physics simulations to elucidate the mechanisms of IPC, establishes a data processing technique which allows for characterization of IPC, formalizes and implements a nonlinear deconvolution method by which the effects of IPC can be mitigated, and examines the impact that IPC can have on scientific conclusions if left uncorrected.
Publisher Site: RIT Repository
PDF (Requires Sign-In): PDF
Click for Bibtex
@book{donlon2019on, title = {On Interpixel Capacitive Coupling in Hybridized HgCdTe Arrays: Theory, Characterization and Correction}, author = {Donlon, Kevan}, year = {2019}, school = {Rochester Institute of Technology} }
[D-2019-26] Payap Sirinam. (2019). “Website Fingerprinting using Deep Learning.” Rochester Institute of Technology.
Abstract: Website fingerprinting (WF) enables a local eavesdropper to determine which websites a user is visiting over an encrypted connection. State-of-the-art WF attacks have been shown to be effective even against Tor. Recently, lightweight WF defenses for Tor have been proposed that substantially degrade existing attacks: WTF-PAD and Walkie-Talkie. In this work, we explore the impact of recent advances in deep learning on WF attacks and defenses. We first present Deep Fingerprinting (DF), a new WF attack based on deep learning, and we evaluate this attack against WTF-PAD and Walkie-Talkie. The DF attack attains over 98% accuracy on Tor traffic without defenses, making it the state-of-the-art WF attack at the time of publishing this work. DF is the only attack that is effective against WTF-PAD with over 90% accuracy, and against Walkie-Talkie, DF achieves a top-2 accuracy of 98%. In the more realistic open-world setting, our attack remains effective. These findings highlight the need for defenses that protect against attacks like DF that use advanced deep learning techniques. Since DF requires large amounts of training data that is regularly updated, some may argue that is it is not practical for the weaker attacker model typically assumed in WF. Additionally, most WF attacks make strong assumptions about the testing and training data have similar distributions and being collected from the same type of network at about the same time. Thus, we next examine ways that an attacker could reduce the difficulty of performing an attack by leveraging N-shot learning, in which just a few training samples are needed to identify a given class. In particular, we propose a new WF attack called Triplet Fingerprinting (TF) that uses triplet networks for N-shot learning. We evaluate this attack in challenging settings such as where the training and testing data are from multiple years apart and collected on different networks, and we find that the TF attack remains effective in such settings with 85% accuracy or better. We also show that the TF attack is also effective in the open world and outperforms transfer learning. Finally, in response to the DF and TF attacks, we propose the CAM-Pad defense: a novel WF defense utilizing the Grad-CAM visual explanation technique. Grad-CAM can be used to identify regions of particular sensitivity in the data and provide insight into the features that the model has learned, providing more understanding about how the DF attack makes its prediction. The defense is based on a dynamic flow-padding defense, making it practical for deployment in Tor. The defense can reduce the attacker's accuracy using the DF attack from 98% to 67%, which is much better than the WTF-PAD defense, with a packet overhead of approximately 80%.
Publisher Site: RIT Repository
PDF (Requires Sign-In): PDF
Click for Bibtex
@book{sirinam2019website, title = {Website Fingerprinting using Deep Learning}, author = {Sirinam, Payap}, year = {2019}, school = {Rochester Institute of Technology} }
[D-2019-25] Travis S. Emery. (2019). “Bubble Interactions at Multi-Fluid Interfaces.” Rochester Institute of Technology.
Abstract: Numerous industrial applications and environmental phenomena are centered around bubble interactions at multi-fluid interfaces. These applications range from metallurgical processing to direct contact evaporation and solid shell formation. Environmental phenomena, such as bubble collisions with the sea surface microlayer and the collision of liquid encapsulated bubbles, were also considered as motivators for this work. Although the associated flow dynamics are complex, they play a vital role in governing the related application outcome, be it in terms of mass or heat transfer efficiency, bubble shell production rate, chemical reaction rate, etc. For this reason, a fundamental understanding of the fluid dynamics involved in the bubble interactions are required to aid in optimal system design. In this work, rigorous experimental work was supplemented by in-depth theoretical analysis to unravel the physics behind these bubble interactions. The focus of the present work is to develop an improved understanding of bubble interactions at liquid-liquid and compound interfaces. Extensive testing has been carried out to identify and classify flow regimes associated with single bubble and bubble stream passage through a liquid-liquid interface. Dimensionless numbers were identified and employed to map these regimes and identify transition criteria. The extension of one identified regime, bubble shell formation, to the field of direct contact evaporation was considered through the development of a numerical model to predict bubble growth in an immiscible liquid droplet. Additional dimensional analysis was carried out for the characterization of bubble collisions at solid and free surfaces. Previously developed numerical models were employed to form the relationship between the appropriate dimensionless groups capable of characterizing such collisions. This relationship was then used to describe a practical method for predicting the radial film size formed during the collision. Finally, three numerical models were developed to predict the bubble motion and the spatiotemporal evolution of the film(s) formed during the collision of a bubble with a liquid-liquid, solid-liquid-liquid, and gas-liquid-liquid interface. These models were validated through additional experiments carried out for this work as well as from data found in literature.
Publisher Site: RIT Repository
PDF (Requires Sign-In): PDF
Click for Bibtex
@book{emery2019bubble, title = {Bubble Interactions at Multi-Fluid Interfaces}, author = {Emery, Travis S.}, year = {2019}, school = {Rochester Institute of Technology} }
[D-2019-24] Anton Travinsky. (2019). “Evaluating the Performance of Digital Micromirror Devices for Use as Programmable Slit Masks in Multi-Object Spectrometers.” Rochester Institute of Technology.
Abstract: Multi-object spectrometers are extremely useful astronomical instruments that allow simultaneous spectral observations of large numbers of objects. Studies performed with ground-based multi-object spectrometers (MOSs) in the last four decades helped to place unique constraints on cosmology, large scale structure, galaxy evolution, Galactic structure, and contributed to countless other scientific advances. However, terrestrial MOSs use large discrete components for object selection, which, aside from not transferable to space-based applications, are limited in both minimal slit width and minimal time required accommodate a change of the locations of objects of interest in the field of view. There is a pressing need in remotely addressable and fast-re-configurable slit masks, which would allow for a new class of instruments - spacebased MOS. There are Microelectromechanical System (MEMS) - based technologies under development for use in space-based instrumentation, but currently they are still unreliable, even on the ground. A digital micromirror device (DMD) is a highly capable, extremely reliable, and remotely re-configurable spatial light modulator (SLM) that was originally developed by Texas Instruments Incorporated for projection systems. It is a viable and very promising candidate to serve as slit mask for both terrestrial and space-based MOSs. This work focused on assessing the suitability of DMDs for use as slit masks in space-based astronomical MOSs and developing the necessary calibration procedures and algorithms. Radiation testing to the levels of orbit around the second Lagrangian point (L2) was performed using the accelerated heavy-ion irradiation approach. The DMDs were found to be extremely reliable in such radiation environment, the devices did not experience hard failures and there was no permanent damage. Expected single-event upset (SEU) rate was determined to be about 5.6 micro-mirrors per 24 hours on-orbit for 1-megapixel device. Results of vibration and mechanical shock testing performed according to the National Aeronautics and Space Administration (NASA) General Environmental Verification Standard (GEVS) at NASA Goddard Space Flight Center (GSFC) suggest that commercially available DMDs are mechanically suitable for space-deployment with a very significant safety margin. Series of tests to assess the performance and the behaviour of DMDs in cryogenic temperatures (down to 78 K) were also carried out. There were no failures or malfunctions detected in commercially-available devices. An earlier prototype of a terrestrial DMD-based MOS (Rochester Institute of Technology Multi-Object Spectrometer (RITMOS)) was updated with a newer DMD model, and the performance of the instrument was evaluated. All the experiments performed strongly suggest that DMDs are highly reliable and capable devices that are extremely suitable for use as remotely programmable slit masks in MOS.
Publisher Site: RIT Repository
PDF (Requires Sign-In): PDF
Click for Bibtex
@book{travinsky2019evaluating, title = {Evaluating the Performance of Digital Micromirror Devices for Use as Programmable Slit Masks in Multi-Object Spectrometers}, author = {Travinsky, Anton}, year = {2019}, school = {Rochester Institute of Technology} }
[D-2019-23] Mandy C. Nevins. (2019). “Point Spread Function Determination in the Scanning Electron Microscope and Its Application in Restoring Images Acquired at Low Voltage.” Rochester Institute of Technology.
Abstract: Electron microscopes have the capability to examine specimens at much finer detail than a traditional light microscope. Higher electron beam voltages correspond to higher resolution, but some specimens are sensitive to beam damage and charging at high voltages. In the scanning electron microscope (SEM), low voltage imaging is beneficial for viewing biological, electronic, and other beam-sensitive specimens. However, image quality suffers at low voltage from reduced resolution, lower signal-to-noise, and increased visibility of beam-induced contamination. Most solutions for improving low voltage SEM imaging require specialty hardware, which can be costly or system-specific. Point spread function (PSF) deconvolution for image restoration could provide a software solution that is cost-effective and microscope-independent with the ability to produce image quality improvements comparable to specialty hardware systems. Measuring the PSF (i.e., electron probe) of the SEM has been a notoriously difficult task until now. The goals of this work are to characterize the capabilities and limitations of a novel SEM PSF determination method that uses nanoparticle dispersions to obtain a two-dimensional measurement of the PSF, and to evaluate the utility of the measured PSF for restoration of low voltage SEM images. The presented results are meant to inform prospective and existing users of this technique about its fundamental theory, best operating practices, the expected behavior of output PSFs and image restorations, and factors to be aware of during interpretation of results.
Publisher Site: RIT Repository
PDF (Requires Sign-In): PDF
Click for Bibtex
@book{nevins2019point, title = {Point Spread Function Determination in the Scanning Electron Microscope and Its Application in Restoring Images Acquired at Low Voltage}, author = {Nevins, Mandy C.}, year = {2019}, school = {Rochester Institute of Technology} }
[D-2019-22] Mohammed A. Yousefhussien. (2019). “Deep Learning Methods for 3D Aerial and Satellite Data.” Rochester Institute of Technology.
Abstract: Recent advances in digital electronics have led to an overabundance of observations from electro-optical (EO) imaging sensors spanning high spatial, spectral and temporal resolution. This unprecedented volume, variety, and velocity is overwhelming our capacity to manage and translate that data into actionable information. Although decades of image processing research have taken the human out of the loop for many important tasks, the human analyst is still an irreplaceable link in the image exploitation chain, especially for more complex tasks requiring contextual understanding, memory, discernment, and learning. If knowledge discovery is to keep pace with the growing availability of data, new processing paradigms are needed in order to automate the analysis of earth observation imagery and ease the burden of manual interpretation. To address this gap, this dissertation advances fundamental and applied research in deep learning for aerial and satellite imagery. We show how deep learning---a computational model inspired by the human brain---can be used for (1) tracking, (2) classifying, and (3) modeling from a variety of data sources including full-motion video (FMV), Light Detection and Ranging (LiDAR), and stereo photogrammetry. First we assess the ability of a bio-inspired tracking method to track small targets using aerial videos. The tracker uses three kinds of saliency maps: appearance, location, and motion. Our approach achieves the best overall performance, including being the only method capable of handling long-term occlusions. Second, we evaluate the classification accuracy of a multi-scale fully convolutional network to label individual points in LiDAR data. Our method uses only the 3D-coordinates and corresponding low-dimensional spectral features for each point. Evaluated using the ISPRS 3D Semantic Labeling Contest, our method scored second place with an overall accuracy of 81.6\%. Finally, we validate the prediction capability of our neighborhood-aware network to model the bare-earth surface of LiDAR and stereo photogrammetry point clouds. The network bypasses traditionally-used ground classifications and seamlessly integrate neighborhood features with point-wise and global features to predict a per point Digital Terrain Model (DTM). We compare our results with two widely used softwares for DTM extraction, ENVI and LAStools. Together, these efforts have the potential to alleviate the manual burden associated with some of the most challenging and time-consuming geospatial processing tasks, with implications for improving our response to issues of global security, emergency management, and disaster response.
Publisher Site: RIT Repository
PDF (Requires Sign-In): PDF
Click for Bibtex
@book{yousefhussien2019deep, title = {Deep Learning Methods for 3D Aerial and Satellite Data}, author = {Yousefhussien, Mohammed A.}, year = {2019}, school = {Rochester Institute of Technology} }
[D-2019-21] Yu Kee Ooi. (2019). “Light Extraction Efficiency of Nanostructured III-Nitride Light-Emitting Diodes.” Rochester Institute of Technology.
Abstract: III-nitride materials have been extensively employed in a wide variety of applications attributed to their compact sizes, lower operating voltage, higher energy efficiency and longer lifetime. Although tremendous progress has been reported for III-nitride light-emitting diodes (LEDs), further enhancement in the external quantum effciency (η_EQE), which depends upon internal quantum efficiency, injection efficiency and light extraction efficiency (η_extraction), is essential in realizing next generation high-efficiency ultraviolet (UV) and visible LEDs. Several challenges such as charge separation issue, large threading dislocation density, large refractive index contrast between GaN and air, and anisotropic emission at high Al-composition AlGaN quantum wells in the deep-UV regime have been identified to obstruct the realization of high-brightness LEDs. As a result, novel LED designs and growth methods are highly demanded to address those issues. The objective of this dissertation is to investigate the enhancement of η_extraction for various nanostructured III-nitride LEDs. In the first part, comprehensive studies on the polarization-dependent η_extraction for AlGaN-based flip-chip UV LEDs with microdome-shaped patterned sapphire substrates (PSS) and AlGaN-based nanowire UV LEDs are presented. Results show that the microdome-shaped PSS acts as an extractor for transverse-magnetic (TM)-polarized light where up to ~11.2-times and ~2.6-times improvement in TM-polarized η_extraction can be achieved for 230 nm and 280 nm flip-chip UV LEDs, while as a reflector that limits the extraction of transverse-electric (TE)-polarized light through the sapphire substrate. Analysis for 230 nm UV LEDs with nanowire structure shows up to ~48% TM-polarized η_extraction and ~41% TE-polarized η_extraction as compared to the conventional planar structure (~0.2% for TM-polarized η_extraction and ~2% for TE-polarized η_extraction). Plasmonic green LEDs with nanowire structure have also been investigated for enhancing the LED performance via surface plasmon polaritons. The analysis shows that both η_extraction and Purcell factor for the investigated plasmonic nanowire LEDs are independent of the Ag cladding layer thickness (H_Ag), where a Purcell factor of ~80 and η_extraction of ~65% can be achieved when H_Ag > 60 nm. Nanosphere lithography and KOH-based wet etching process have been developed for the top-down fabrication of III-nitride nanowire LEDs. The second part of this dissertation focuses on alternative approaches to fabricate white LEDs. The integration of three-dimensional (3D) printing technology with LED fabrication is proposed as a straightforward and highly reproducible method to improve η_extraction at the same time to achieve stable white color emission. The use of optically transparent acrylate-based photopolymer with a refractive index of ~1.5 as 3D printed lens on blue LED has exhibited 9% enhancement in the output power at current injection of 4 mA as compared to blue LED without 3D printed lens. Stable white color emission can be achieved with chromaticity coordinates around (0.27, 0.32) and correlated color temperature ~8900 K at current injection of 10 mA by mixing phosphor powder in the 3D printed lens. Novel LED structures employing ternary InGaN substrates are then discussed for realizing high-efficiency monolithic tunable white LEDs. Results show that large output power (~170 mW), high η_EQE (~50%), chromaticity coordinates around (0.30, 0.28), and correlated color temperature ~8200 K can be achieved by engineering the band structures of the InGaN/InGaN LEDs on ternary InGaN substrates.
Publisher Site: RIT Repository
PDF (Requires Sign-In): PDF
Click for Bibtex
@book{ooi2019light, title = {Light Extraction Efficiency of Nanostructured III-Nitride Light-Emitting Diodes}, author = {Ooi, Yu Kee}, year = {2019}, school = {Rochester Institute of Technology} }
[D-2019-20] George Thomas Nelson IV. (2019). “Native and Radiation-induced Defects in III-V Solar Cells and Photodiodes.” Rochester Institute of Technology.
Abstract: Photodiodes made of III-V materials are ubiquitous with applications for telecommunications, photonics, consumer electronics, and spectroscopy. The III-V solar cell, specifically, is a large-area photodiode that is used by the satellite industry for power conversion due to its unrivaled efficiency and wide range of available materials. As a device driven by its minority carrier diffusion length (MCDL), the performance of a photodiode is sensitive to crystallographic defects that create states in the forbidden energy gap. Defects commonly arise during growth of the crystal and during device fabrication, and they accumulate slowly over time when deployed into the damaging environment of space. Defect-assisted carrier recombination leads to lower MCDL, higher dark current, reduced sensitivity and signal-to-noise ratio, and, in the case of solar cells, reduced power conversion efficiency. Consequently, the development of photodiode technology requires techniques for detection, characterization, and mitigation of defects and the inter-bandgap states they create. In this work, III-V material defects are addressed across a variety of materials and devices. The first half of the work makes use of deep-level transient spectroscopy (DLTS) to deduce the energy level, cross-section, and density of traps the InAlAs, InAlAsSb, and InGaAs lattice-matched to InP. An in situ DLTS system that can monitor defects immediately after irradiation was developed and applied to InGaAs photodiodes irradiated by protons. Evidence of trap annealing was found to occur as low as 150 K. The second half begins with development of GaSb solar cells grown by molecular beam epitaxy on GaAs substrate intended for use in lower-cost monolithic multi-junction cells. Defect analysis by microscopy, dark lock-in thermography, and dark current measurement, among others, was performed. The best GaSb-on-GaAs cell achieved state-of-the-art 4.5% efficiency under concentrated solar spectrum. Finally, light management in III-V photodiodes was explored as a possible route for defect mitigation. Textures, diffraction gratings, metallic mirrors, and Bragg reflectors were simulated by finite difference time domain for single- and multi-junction GaAs-based cells with the aim of reducing the amount of absorber material required and to simultaneously reduce MCDL requirements by generating carriers closer to the junction. The results were inputted into a device simulator to predict efficiency. A backside reflective pyramidal-textured grating was simulated to allow a GaAs cell to be thinned by a factor of >30 compared to a conventional cell.
Publisher Site: RIT Repository
PDF (Requires Sign-In): PDF
Click for Bibtex
@book{nelson2019native, title = {Native and Radiation-induced Defects in III-V Solar Cells and Photodiodes}, author = {Nelson, George Thomas, IV}, year = {2019}, school = {Rochester Institute of Technology} }
[D-2019-19] Keegan S. McCoy. (2019). “Methodology for the Integration of Optomechanical System Software Models with a Radiative Transfer Image Simulation Model.” Rochester Institute of Technology.
Abstract: Stray light, any unwanted radiation that reaches the focal plane of an optical system, reduces image contrast, creates false signals or obscures faint ones, and ultimately degrades radiometric accuracy. These detrimental effects can have a profound impact on the usability of collected Earth-observing remote sensing data, which must be radiometrically calibrated to be useful for scientific applications. Understanding the full impact of stray light on data scientific utility is of particular concern for lower cost, more compact imaging systems, which inherently provide fewer opportunities for stray light control. To address these concerns, this research presents a general methodology for integrating point spread function (PSF) and stray light performance data from optomechanical system models in optical engineering software with a radiative transfer image simulation model. This integration method effectively emulates the PSF and stray light performance of a detailed system model within a high-fidelity scene, thus producing realistic simulated imagery. This novel capability enables system trade studies and sensitivity analyses to be conducted on parameters of interest, particularly those that influence stray light, by analyzing their quantitative impact on user applications when imaging realistic operational scenes. For Earth science applications, this method is useful in assessing the impact of stray light performance on retrieving surface temperature, ocean color products such as chlorophyll concentration or dissolved organic matter, etc. The knowledge gained from this model integration also provides insight into how specific stray light requirements translate to user application impact, which can be leveraged in writing more informed stray light requirements. In addition to detailing the methodology's radiometric framework, we describe the collection of necessary raytrace data from an optomechanical system model (in this case, using FRED Optical Engineering Software), and present PSF and stray light component validation tests through imaging Digital Imaging and Remote Sensing Image Generation (DIRSIG) model test scenes. We then demonstrate the integration method's ability to produce quantitative metrics to assess the impact of stray light-focused system trade studies on user applications using a Cassegrain telescope model and a stray light-stressing coastal scene under various system and scene conditions. This case study showcases the stray light images and other detailed performance data produced by the integration method that take into account both a system's stray light susceptibility and a scene's at-aperture radiance profile to determine the stray light contribution of specific system components or stray light paths. The innovative contributions provided by this work represent substantial improvements over current stray light modeling and simulation techniques, where the scene image formation is decoupled from the physical system stray light modeling, and can aid in the design of future Earth-observing imaging systems. This work ultimately establishes an integrated-systems approach that combines the effects of scene content and the optomechanical components, resulting in a more realistic and higher fidelity system performance prediction.
Publisher Site: RIT Repository
PDF (Requires Sign-In): PDF
Click for Bibtex
@book{mccoy2019methodology, title = {Methodology for the Integration of Optomechanical System Software Models with a Radiative Transfer Image Simulation Model}, author = {McCoy, Keegan S.}, year = {2019}, school = {Rochester Institute of Technology} }
[D-2019-18] Kevin Christopher Cooke. (2019). “Magnificent Constructions: The Role of Environment on the Stellar Mass Growth of Massive Galaxies.” Rochester Institute of Technology.
Abstract: To understand how the present day universe came to be, we must understand how the massive structures in which we live formed and evolved over the preceding billions of years. Constraining how galaxies grow are the most massive galaxies, called brightest cluster galaxies (BCGs). These luminous and diffuse elliptical galaxies inhabit relaxed positions within their host cluster's gravitational potentials and provide a look at the high mass extreme of galaxy evolution. The relaxed structure, old stellar populations, and central location within the cluster indicate a high redshift formation scenario, however, star-forming BCGs have been observed at much more recent epochs. Addressing this evolutionary complexity, my dissertation consists of four studies to investigate the growth rates of BCGs over several epochs, and how they relate to the growth of the general galaxy population. In my first paper, I present a multiwavelength (far-ultraviolet to far-infrared) study of BCG star formation rates and stellar masses from 0.2 10^11 M_Solar) from galaxy clusters available in the COSMOS X-ray Group Catalog. I find that star formation is roughly constant in our sample of high mass BCGs from 0.3 2.25. Finally, my fourth paper (Cooke et al. in preparation) places the preceding results in context by measuring the correlation between star formation rate and stellar mass for all galaxies above the COSMOS mass completeness limit from 0
Publisher Site: RIT Repository
PDF (Requires Sign-In): PDF
Click for Bibtex
@book{cooke2019magnificent, title = {Magnificent Constructions: The Role of Environment on the Stellar Mass Growth of Massive Galaxies}, author = {Cooke, Kevin Christopher}, year = {2019}, school = {Rochester Institute of Technology} }
[D-2019-17] Sankaranarayanan Piramanayagam. (2019). “Segmentation and Classification of Multimodal Imagery.” Rochester Institute of Technology.
Abstract: Segmentation and classification are two important computer vision tasks that transform input data into a compact representation that allow fast and efficient analysis. Several challenges exist in generating accurate segmentation or classification results. In a video, for example, objects often change the appearance and are partially occluded, making it difficult to delineate the object from its surroundings. This thesis proposes video segmentation and aerial image classification algorithms to address some of the problems and provide accurate results. We developed a gradient driven three-dimensional segmentation technique that partitions a video into spatiotemporal objects. The algorithm utilizes the local gradient computed at each pixel location together with the global boundary map acquired through deep learning methods to generate initial pixel groups by traversing from low to high gradient regions. A local clustering method is then employed to refine these initial pixel groups. The refined sub-volumes in the homogeneous regions of video are selected as initial seeds and iteratively combined with adjacent groups based on intensity similarities. The volume growth is terminated at the color boundaries of the video. The over-segments obtained from the above steps are then merged hierarchically by a multivariate approach yielding a final segmentation map for each frame. In addition, we also implemented a streaming version of the above algorithm that requires a lower computational memory. The results illustrate that our proposed methodology compares favorably well, on a qualitative and quantitative level, in segmentation quality and computational efficiency with the latest state of the art techniques. We also developed a convolutional neural network (CNN)-based method to efficiently combine information from multisensor remotely sensed images for pixel-wise semantic classification. The CNN features obtained from multiple spectral bands are fused at the initial layers of deep neural networks as opposed to final layers. The early fusion architecture has fewer parameters and thereby reduces the computational time and GPU memory during training and inference. We also introduce a composite architecture that fuses features throughout the network. The methods were validated on four different datasets: ISPRS Potsdam, Vaihingen, IEEE Zeebruges, and Sentinel-1, Sentinel-2 dataset. For the Sentinel-1,-2 datasets, we obtain the ground truth labels for three classes from OpenStreetMap. Results on all the images show early fusion, specifically after layer three of the network, achieves results similar to or better than a decision level fusion mechanism. The performance of the proposed architecture is also on par with the state-of-the-art results.
Publisher Site: RIT Repository
PDF (Requires Sign-In): PDF
Click for Bibtex
@book{piramanayagam2019segmentation, title = {Segmentation and Classification of Multimodal Imagery}, author = {Piramanayagam, Sankaranarayanan}, year = {2019}, school = {Rochester Institute of Technology} }
[D-2019-16] Kamal Jnawali. (2019). “Automatic Cancer Tissue Detection Using Multispectral Photoacoustic Imaging.” Rochester Institute of Technology.
Abstract: Convolutional neural networks (CNNs) have become increasingly popular in recent years because of their ability to tackle complex learning problems such as object detection, and object localization. They are being used for a variety of tasks, such as tissue abnormalities detection and localization, with an accuracy that comes close to the level of human predictive performance in medical imaging. The success is primarily due to the ability of CNNs to extract the discriminant features at multiple levels of abstraction. Photoacoustic (PA) imaging is a promising new modality that is gaining significant clinical potential. The availability of a large dataset of three dimensional PA images of ex-vivo human prostate and thyroid specimens has facilitated this current study aimed at evaluating the efficacy of CNN for cancer diagnosis. In PA imaging, a short pulse of near-infrared laser light is sent into the tissue, but the image is created by focusing the ultrasound waves that are photoacoustically generated due to the absorption of light, thereby mapping the optical absorption in the tissue. By choosing multiple wavelengths of laser light, multispectral photoacoustic (MPA) images of the same tissue specimen can be obtained. The objective of this thesis is to implement deep learning architecture for cancer detection using the MPA image dataset. In this study, we built and examined a fully automated deep learning framework that learns to detect and localize cancer regions in a given specimen entirely from its MPA image dataset. The dataset for this work consisted of samples with size ranging from 12 x 45 x 200 pixels to 64 x 64 x 200 pixels at five wavelengths namely, 760 nm, 800 nm, 850 nm, 930 nm, and 970 nm. The proposed algorithms first extract features using convolutional kernels and then detect cancer tissue using the softmax function, the last layer of the network. The AUC was calculated to evaluate the performance of the cancer tissue detector with a very promising result. To the best of our knowledge, this is one of the first examples of the application of deep 3D CNN to a large cancer MPA dataset for the prostate and thyroid cancer detection. While previous efforts using the same dataset involved decision making using mathematically extracted image features, this work demonstrates that this process can be automated without any significant loss in accuracy. Another major contribution of this work has been to demonstrate that both prostate and thyroid datasets can be combined to produce improved results for cancer diagnosis.
Publisher Site: RIT Repository
PDF (Requires Sign-In): PDF
Click for Bibtex
@book{jnawali2019automatic, title = {Automatic Cancer Tissue Detection Using Multispectral Photoacoustic Imaging}, author = {Jnawali, Kamal}, year = {2019}, school = {Rochester Institute of Technology} }
[D-2019-15] Rehman S. Eon. (2019). “The Characterization of Earth Sediments Using Radiative Transfer Models from Directional Hyperspectral Reflectance.” Rochester Institute of Technology.
Abstract: Remote sensing techniques are continuously being developed to extract physical information about the Earth's surface. Over the years, space-borne and airborne sensors have been used for the characterization of surface sediments. Geophysical properties of a sediment surface such as its density, grain size, surface roughness, and moisture content can influence the angular dependence of spectral signatures, specifically the Bidirectional Reflectance Distribution Function (BRDF). Models based on radiative transfer equations can relate the angular dependence of the reflectance to these geophysical variables. Extraction of these parameters can provide a better understanding of the Earth's surface, and play a vital role in various environmental modeling processes. In this work, we focused on retrieving two of these geophysical properties of earth sediments, the bulk density and the soil moisture content (SMC), using directional hyperspectral reflectance. We proposed a modification to the radiative transfer model developed by Hapke to retrieve sediment bulk density. The model was verified under controlled experiments within a laboratory setting, followed by retrieval of the sediment density from different remote sensing platforms: airborne, space-borne and a ground-based imaging sensor. The SMC was characterized using the physics based multilayer radiative transfer model of soil reflectance or MARMIT. The MARMIT model was again validated from experiments performed in our controlled laboratory setting using several different soil samples across the United States; followed by applying the model in mapping SMC from imagery data collected by an Unmanned Aerial System (UAS) based hyperspectral sensor.
Publisher Site: RIT Repository
PDF (Requires Sign-In): PDF
Click for Bibtex
@book{eon2019the, title = {The Characterization of Earth Sediments Using Radiative Transfer Models from Directional Hyperspectral Reflectance}, author = {Eon, Rehman S.}, year = {2019}, school = {Rochester Institute of Technology} }
[D-2019-14] Tyler R. Peery. (2019). “System Design Considerations for a Low-intensity Hyperspectral Imager of Sensitive Cultural Heritage Manuscripts.” Rochester Institute of Technology.
Abstract: Cultural heritage imaging is becoming more common with the increased availability of more complex imaging systems, including multi- and hyperspectral imaging (MSI and HSI) systems. A particular concern with HSI systems is the broadband source required, regularly including infrared and ultraviolet spectra, which may cause fading or damage to a target. Guidelines for illumination of such objects, even while on display at a museum, vary widely from one another. Standards must be followed to assure the curator to allow imaging and ensure protection of the document. Building trust in the cultural heritage community is key to gaining access to objects of significant import, thus allowing scientists, historians, and the public to view digitally preserved representations of the object, and to allow further discovery of the object through spectral processing and analysis. Imaging was conducted with a light level of 270 lux at variable ground sample distances (GSD's). The light level was chosen to maintain a total dose similar to an hour's display time at a museum, based on the United Kingdom standard for cultural heritage display, PAS 198:2012. The varying GSD was used as a variable to increase signal-to-noise ratios (SNR) or decrease total illumination time on a target. This adjustment was performed both digitally and physically, and typically results in a decrease in image quality, as the spatial resolution of the image decreases. However, a technique called "panchromatic sharpening" was used to recover some of the spatial resolution. This method fuses a panchromatic image with good spatial resolution with a spectral image (either MSI or HSI) with poorer spatial resolution to construct a derivative spectral image with improved spatial resolution. Detector systems and additional methods of data capture to assist in processing of cultural heritage documents are investigated, with specific focus on preserving the physical condition of the potentially sensitive documents.
Publisher Site: RIT Repository
PDF (Requires Sign-In): PDF
Click for Bibtex
@book{peery2019system, title = {System Design Considerations for a Low-intensity Hyperspectral Imager of Sensitive Cultural Heritage Manuscripts}, author = {Peery, Tyler R.}, year = {2019}, school = {Rochester Institute of Technology} }
[D-2019-13] Breton Lawrence Minnehan. (2019). “Deep Grassmann Manifold Optimization for Computer Vision.” Rochester Institute of Technology.
Abstract: In this work, we propose methods that advance four areas in the field of computer vision: dimensionality reduction, deep feature embeddings, visual domain adaptation, and deep neural network compression. We combine concepts from the fields of manifold geometry and deep learning to develop cutting edge methods in each of these areas. Each of the methods proposed in this work achieves state-of-the-art results in our experiments. We propose the Proxy Matrix Optimization (PMO) method for optimization over orthogonal matrix manifolds, such as the Grassmann manifold. This optimization technique is designed to be highly flexible enabling it to be leveraged in many situations where traditional manifold optimization methods cannot be used. We first use PMO in the field of dimensionality reduction, where we propose an iterative optimization approach to Principal Component Analysis (PCA) in a framework called Proxy Matrix optimization based PCA (PM-PCA). We also demonstrate how PM-PCA can be used to solve the general $L_p$-PCA problem, a variant of PCA that uses arbitrary fractional norms, which can be more robust to outliers. We then present Cascaded Projection (CaP), a method which uses tensor compression based on PMO, to reduce the number of filters in deep neural networks. This, in turn, reduces the number of computational operations required to process each image with the network. Cascaded Projection is the first end-to-end trainable method for network compression that uses standard backpropagation to learn the optimal tensor compression. In the area of deep feature embeddings, we introduce Deep Euclidean Feature Representations through Adaptation on the Grassmann manifold (DEFRAG), that leverages PMO. The DEFRAG method improves the feature embeddings learned by deep neural networks through the use of auxiliary loss functions and Grassmann manifold optimization. Lastly, in the area of visual domain adaptation, we propose the Manifold-Aligned Label Transfer for Domain Adaptation (MALT-DA) to transfer knowledge from samples in a known domain to an unknown domain based on cross-domain cluster correspondences.
Publisher Site: RIT Repository
PDF (Requires Sign-In): PDF
Click for Bibtex
@book{minnehan2019deep, title = {Deep Grassmann Manifold Optimization for Computer Vision}, author = {Minnehan, Breton Lawrence}, year = {2019}, school = {Rochester Institute of Technology} }
[D-2019-12] Jie Yang. (2019). “Crime Scene Blood Evidence Detection Using Spectral Imaging.” Rochester Institute of Technology.
Abstract: Blood is the key evidence for forensic investigation because it carries critical information to help reconstruct the crime scene, confirm or exclude a suspect, and analyze the timing of a crime. Conventional bloodstain detection uses chemical methods. Those methods require cautious sample preparations. They are destructive to samples in principle. Some of them are carcinogenic to investigators. They require experienced investigators and constrained conditions. Spectral imaging methods are an emerging technique for bloodstain detection in forensic science. It provides a non-destructive, non-contact, non-toxic, and real-time methodology for presumptive bloodstain searching, either in the field or in the laboratory. This thesis prototyped two crime scene bloodstain detection imaging systems. The first generation crime scene imaging system is a LCTF based visible hyperspectral imaging system. Detection results of a simulated indoor crime scene show that bloodstains can be highlighted. However, this system has some drawbacks. First of all, it only records spectral information at 400 nm to 700 nm spectral range. Bloodstains on some substrates may not be detected. Second, it has a low SNR. This is mainly due to the low transmittance of the LCTF. Third, its FOV is only $pm$7 degrees from normal. Fourth, it cannot work continuously for a long time. The lighting module is attached next to the camera, which emits excessive heat and warms the camera quickly. Fifth, it is not calibrated into physical units such as radiance or reflectance. Therefore, a second generation crime scene imaging system was developed. The second generation crime scene imaging system is a VNIR multispectral imaging system. It uses interference filters to construct the spectral bands. Blood reflectance spectral features were extracted from the comparison of blood and visually similar non-blood substances on various common found substrates. Three spectral features were used to construct the VNIR spectral bands of the multispectral imaging system. A linear regression pixel-wise model was used to enhance the spatial uniformity of the CMOS sensor. The lens falloff was corrected. The transmittance spectra of interference filters with various incident angles were calibrated. The system was calibrated to reflectance with the required 10\% accuracy from first principle based modeling. Two verification tests were carried using the MSI system. The first test is a systematic test where 9 substrates were laid radially symmetric to study the spectral shift effect introduced by the interference filters. Comparing with the reflectance error, the spectral shift is found to be not as influential for bloodstain detection using RBD, RX, and TAD methods. The result from the first principle based modeling agrees with the test. The second test is a semi-realistic indoor crime scene test where different sizes of blood spatters were directly applied to off-white carpet, dark carpet, door, and painted wall under various daytime environmental conditions. The image processing workflow includes noise reduction, bloodstain detection, and data fusion. Bloodstains were detected in a large FoV in the semi-realistic crime scene on most substrates, except for a small spatter on dark color thick carpet. SNR is the major factor impacting the detectability, the 2nd major factor is the extra visible lighting which lacks of near infrared information. The contribution of this work are three aspects: the first is the developing and prototyping an interference filter based multispectral imaging system used at a large FoV for forensic bloodstain detection. The second is the discussion of the spectral shift impact compared with the reflectance calibration error suggests that spectral shift is not as influential as reflectance calibration error. Third, the discussion of the impact of environmental conditions towards bloodstain detection on this MSI system.
Publisher Site: RIT Repository
PDF (Requires Sign-In): PDF
Click for Bibtex
@book{yang2019crime, title = {Crime Scene Blood Evidence Detection Using Spectral Imaging}, author = {Yang, Jie}, year = {2019}, school = {Rochester Institute of Technology} }
[D-2019-11] Lauren L. Taylor. (2019). “Ultrafast Laser Polishing for Optical Fabrication.” Rochester Institute of Technology.
Abstract: Next-generation imaging systems for consumer electronics, AR/VR, and space telescopes require weight, size, and cost reduction while maintaining high optical performance. Freeform optics with rotationally asymmetric surface geometries condense the tasks of several spherical optics onto a single element. They are currently fabricated by ultraprecision sub-aperture tools like diamond turning and magnetorheological finishing, but the final surfaces contain mid-spatial-frequency tool marks and form errors which fall outside optical tolerances. Therefore, there remains a need for disruptive tools to generate optic-quality freeform surfaces. This thesis work investigates a high-precision, flexible, non-contact methodology for optics polishing using femtosecond ultrafast lasers. Femtosecond lasers enable ablation-based material removal on substrates with widely different optical properties owing to their high GW-TW/cm\textsuperscript2 peak intensities. For laser-based polishing, it is imperative to precisely remove material while minimizing the onset of detrimental thermal and structural surface artifacts such as melting and oxidation. However, controlling the laser interaction is a non-trivial task due to the competing influence of nonthermal melting, ablation, electron/lattice thermalization, heat accumulation, and thermal melting phenomena occurring on femtosecond to microsecond timescales. Femtosecond laser-material interaction was investigated from the fundamental theoretical and experimental standpoints to determine a methodology for optic-quality polishing of optical / photonic materials. Numerical heat accumulation and two-temperature models were constructed to simulate femtosecond laser processing and predict material-specific laser parameter combinations capable of achieving ablation with controlled thermal impact. A tunable femtosecond laser polishing system was established. Polishing of germanium substrates was successfully demonstrated using the model-determined laser parameters, achieving controllable material removal while maintaining optical surface quality. The established polishing technique opens a viable path for sub-aperture, optic quality finishing of optical / photonic materials, capable of scaling up to address complex polishing tasks towards freeform optics fabrication.
Publisher Site: RIT Repository
PDF (Requires Sign-In): PDF
Click for Bibtex
@book{taylor2019ultrafast, title = {Ultrafast Laser Polishing for Optical Fabrication}, author = {Taylor, Lauren L.}, year = {2019}, school = {Rochester Institute of Technology} }
[D-2019-10] Ryan Ford. (2019). “Water Quality and Algal Bloom Sensing from Multiple Imaging Platforms.” Rochester Institute of Technology.
Abstract: Harmful cyanobacteria blooms have been increasing in frequency throughout the world resulting in a greater need for water quality monitoring. Traditional methods of monitoring water quality, such as point sampling, are often resource expensive and time consuming in comparison to remote sensing approaches, however the spatial resolution of established water remote sensing satellites is often too coarse (300 m) to resolve smaller inland waterbodies. The fine scale spatial resolution and improved radiometric sensitivity of Landsat satellites (30 m) can resolve these smaller waterbodies, enabling their capability for cyanobacteria bloom monitoring. In this work, the utility of Landsat to retrieve concentrations of two cyanobacteria bloom pigments, chlorophyll-a and phycocyanin, is assessed. Concentrations of these pigments are retrieved using a spectral Look-Up-Table (LUT) matching process, where an exploration of the effects of LUT design on retrieval accuracy is performed. Potential augmentations to the spectral sampling of Landsat are also tested to determine how it can be improved for waterbody constituent concentration retrieval. Applying the LUT matching process to Landsat 8 imagery determined that concentrations of chlorophyll-a, total suspended solids, and color dissolved organic matter were retrieved with a satisfactory accuracy through appropriate choice of atmospheric compensation and LUT design, in agreement with previously reported implementations of the LUT matching process. Phycocyanin proved to be a greater challenge to this process due to its weak effect on waterbody spectrum, the lack of Landsat spectral sampling over its predominant spectral feature, and error from atmospheric compensation. From testing potential enhancements to Landsat spectral sampling, we determine that additional spectral sampling in the yellow and red edge regions of the visible/near-infrared (VNIR) spectrum can lead to improved concentration retrievals. This performance further improves when sampling is added to both regions, and when Landsat is transitioned to a VNIR imaging spectrometer, though this is dependent on band position and spacing. These results imply that Landsat can be used to monitor cyanobacteria blooms through retrieval of chlorophyll-a, and this retrieval performance can be improved in future Landsat systems, even with minor changes to spectral sampling. This includes improvement in retrieval of phycocyanin when implementing a VNIR imaging spectrometer.
Publisher Site: RIT Repository
PDF (Requires Sign-In): PDF
Click for Bibtex
@book{ford2019water, title = {Water Quality and Algal Bloom Sensing from Multiple Imaging Platforms}, author = {Ford, Ryan}, year = {2019}, school = {Rochester Institute of Technology} }
[D-2019-09] Jacob Wirth. (2019). “Point Spread Function and Modulation Transfer Function Engineering.” Rochester Institute of Technology.
Abstract: A novel computational imaging approach to sensor protection based on point spread function (PSF) engineering is designed to suppress harmful laser irradiance without significant loss of image fidelity of a background scene. PSF engineering is accomplished by modifying a traditional imaging system with a lossless linear phase mask at the pupil which diffracts laser light over a large area of the imaging sensor. The approach provides the additional advantage of an instantaneous response time across a broad region of the electromagnetic spectrum. As the mask does not discriminate between the laser and desired scene, a post-processing image reconstruction step is required, which may be accomplished in real time, that both removes the laser spot and improves the image fidelity. This thesis includes significant experimental and numerical advancements in the determination and demonstration of optimized phase masks. Analytic studies of PSF engineering systems and their fundamental limits were conducted. An experimental test-bed was designed using a spatial light modulator to create digitally-controlled phase masks to image a target in the presence of a laser source. Experimental results using already known phase masks: axicon, vortex and cubic are reported. New methods for designing phase masks are also reported including (1) a numeric differential evolution algorithm, (2) a "PSF reverse engineering" method, and (3) a hardware based simulated annealing experiment. Broadband performance of optimized phase masks were also evaluated in simulation. Optimized phase masks were shown to provide three orders of magnitude laser suppression while simultaneously providing high fidelity imaging a background scene.
Publisher Site: RIT Repository
PDF (Requires Sign-In): PDF
Click for Bibtex
@book{wirth2019point, title = {Point Spread Function and Modulation Transfer Function Engineering}, author = {Wirth, Jacob}, year = {2019}, school = {Rochester Institute of Technology} }
[D-2019-08] Sanghui Han. (2019). “Utility Analysis for Optimizing Compact Adaptive Spectral Imaging Systems for Subpixel Target Detection Applications.” Rochester Institute of Technology.
Abstract: Since the development of spectral imaging systems where we transitioned from panchromatic, single band images to multiple bands, we have pursued a way to evaluate the quality of spectral images. As spectral imaging capabilities improved and the bands collected wavelengths outside of the visible spectrum they could be used to gain information about the earth such as material identification that would have been a challenge with panchromatic images. We now have imaging systems capable of collecting images with hundreds of contiguous bands across the reflective portion of the electromagnetic spectrum that allows us to extract information at subpixel levels. Prediction and assessment methods for panchromatic image quality, while well-established are continuing to be improved. For spectral images however, methods for analyzing quality and what this entails have yet to form a solid framework. In this research, we built on previous work to develop a process to optimize the design of spectral imaging systems. We used methods for predicting quality of spectral images and extended the existing framework for analyzing efficacy of miniature systems. We comprehensively analyzed utility of spectral images and efficacy of compact systems for a set of application scenarios designed to test the relationships of system parameters, figures of merit, and mission requirements in the trade space for spectral images collected by a compact imaging system from design to operation. We focused on subpixel target detection to analyze spectral image quality of compact spaceborne systems with adaptive band selection capabilities. In order to adequately account for the operational aspect of exploiting adaptive band collection capabilities, we developed a method for band selection. Dimension reduction is a step often employed in processing spectral images, not only to improve computation time but to avoid errors associated with high dimensionality. An adaptive system with a tunable filter can select which bands to collect for each target so the dimension reduction happens at the collection stage instead of the processing stage. We developed the band selection method to optimize detection probability using only the target reflectance signature. This method was conceived to be simple enough to be calculated by a small on-board CPU, to be able to drive collection decisions, and reduce data processing requirements. We predicted the utility of the selected bands using this method, then validated the results using real images, and cross-validated them using simulated image associated with perfect truth data. In this way, we simultaneously validated the band selection method we developed and the combined use of the simulation and prediction tools used as part of the analytic process to optimize system design. We selected a small set of mission scenarios and demonstrated the use of this process to provide example recommendations for efficacy and utility based on the mission. The key parameters we analyzed to drive the design recommendations were target abundance, noise, number of bands, and scene complexity. We found critical points in the system design trade space, and coupled with operational requirements, formed a set of mission feasibility and system design recommendations. The selected scenarios demonstrated the relationship between the imaging system design and operational requirements based on the mission. We found key points in the spectral imaging trade space that indicated relationships within the spectral image utility trade space that can be used to further solidify the frameworks for compact spectral imaging systems.
Publisher Site: RIT Repository
PDF (Requires Sign-In): PDF
Click for Bibtex
@book{han2019utility, title = {Utility Analysis for Optimizing Compact Adaptive Spectral Imaging Systems for Subpixel Target Detection Applications}, author = {Han, Sanghui}, year = {2019}, school = {Rochester Institute of Technology} }
[D-2019-07] Kamran Binaee. (2019). “Study of Human Hand-eye Coordination Using Machine Learning Techniques in a Virtual Reality Setup.” Rochester Institute of Technology.
Abstract: Theories of visually guided action are characterized as closed-loop control in the presence of reliable sources of visual information, and predictive control to compensate for visuomotor delay and temporary occlusion. However, prediction is not well understood. To investigate, a series of studies was designed to characterize the role of predictive strategies in humans as they perform visually guided actions, and to guide the development of computational models that capture these strategies. During data collection, subjects were immersed in a virtual reality (VR) system and were tasked with using a paddle to intercept a virtual ball. To force subjects into a predictive mode of control, the ball was occluded or made invisible for a portion of its 3D parabolic trajectory. The subjects gaze, hand and head movements were recorded during the performance. To improve the quality of gaze estimation, new algorithms were developed for the measurement and calibration of spatial and temporal errors of an eye tracking system. The analysis focused on the subjects gaze and hand movements reveal that, when the temporal constraints of the task did not allow the subjects to use closed-loop control, they utilized a short-term predictive strategy. Insights gained through behavioral analysis were formalized into computational models of visual prediction using machine learning techniques. In one study, LSTM recurrent neural networks were utilized to explain how information is integrated and used to guide predictive movement of the hand and eyes. In a subsequent study, subject data was used to train an inverse reinforcement learning (IRL) model that captures the full spectrum of strategies from closed-loop to predictive control of gaze and paddle placement. A comparison of recovered reward values between occlusion and no-occlusion conditions revealed a transition from online to predictive control strategies within a single course of action. This work has shed new insights on predictive strategies that guide our eye and hand movements.
Publisher Site: RIT Repository
PDF (Requires Sign-In): PDF
Click for Bibtex
@book{binaee2019study, title = {Study of Human Hand-eye Coordination Using Machine Learning Techniques in a Virtual Reality Setup}, author = {Binaee, Kamran}, year = {2019}, school = {Rochester Institute of Technology} }
[D-2019-06] Baabak G. Mamaghani. (2019). “An Analysis of the Radiometric Quality of Small Unmanned Aircraft System Imagery.” Rochester Institute of Technology.
Abstract: In recent years, significant advancements have been made in both sensor technology and small Unmanned Aircraft Systems (sUAS). Improved sensor technology has provided users with cheaper, lighter, and higher resolution imaging tools, while new sUAS platforms have become cheaper, more stable and easier to navigate both manually and programmatically. These enhancements have provided remote sensing solutions for both commercial and research applications that were previously unachievable. However, this has provided non-scientific practitioners with access to technology and techniques previously only available to remote sensing professionals, sometimes leading to improper diagnoses and results. The work accomplished in this dissertation demonstrates the impact of proper calibration and reflectance correction on the radiometric quality of sUAS imagery. The first part of this research conducts an in-depth investigation into a proposed technique for radiance-to-reflectance conversion. Previous techniques utilized reflectance conversion panels in-scene, which, while providing accurate results, required extensive time in the field to position the panels as well as measure them. We have positioned sensors on board the sUAS to record the downwelling irradiance which then can be used to produce reflectance imagery without the use of these reflectance conversion panels. The second part of this research characterizes and calibrates a MicaSense RedEdge-3, a multispectral imaging sensor. This particular sensor comes pre-loaded with metadata values, which are never recalibrated, for dark level bias, vignette and row-gradient correction and radiometric calibration. This characterization and calibration studies were accomplished to demonstrate the importance of recalibration of any sensors over a period of time. In addition, an error propagation was performed to detect the highest contributors of error in the production of radiance and reflectance imagery. Finally, a study of the inherent reflectance variability of vegetation was performed. In other words, this study attempts to determine how accurate the digital count to radiance calibration and the radiance to reflectance conversion has to be. Can we lower our accuracy standards for radiance and reflectance imagery, because the target itself is too variable to measure? For this study, six Coneflower plants were analyzed, as a surrogate for other cash crops, under different illumination conditions, at different times of the day, and at different ground sample distances (GSDs).
Publisher Site: RIT Repository
PDF (Requires Sign-In): PDF
Click for Bibtex
@book{mamaghani2019an, title = {An Analysis of the Radiometric Quality of Small Unmanned Aircraft System Imagery}, author = {Mamaghani, Baabak G.}, year = {2019}, school = {Rochester Institute of Technology} }
[D-2019-05] Yilong Liang. (2019). “Object Detection in High Resolution Aerial Images and Hyperspectral Remote Sensing Images.” Rochester Institute of Technology.
Abstract: With rapid developments in satellite and sensor technologies, there has been a dramatic increase in the availability of remotely sensed images. However, the exploration of these images still involves a tremendous amount of human interventions, which are tedious, time-consuming, and inefficient. To help imaging experts gain a complete understanding of the images and locate the objects of interest in a more accurate and efficient way, there is always an urgent need for developing automatic detection algorithms. In this work, we delve into the object detection problems in remote sensing applications, exploring the detection algorithms for both hyperspectral images (HSIs) and high resolution aerial images. In the first part, we focus on the subpixel target detection problem in HSIs with low spatial resolutions, where the objects of interest are much smaller than the image pixel spatial resolution. To this end, we explore the detection frameworks that integrate image segmentation techniques in designing the matched filters (MFs). In particular, we propose a novel image segmentation algorithm to identify the spatial-spectral coherent image regions, from which the background statistics were estimated for deriving the MFs. Extensive experimental studies were carried out to demonstrate the advantages of the proposed subpixel target detection framework. Our studies show the superiority of the approach when comparing to state-of-the-art methods. The second part of the thesis explores the object based image analysis (OBIA) framework for geospatial object detection in high resolution aerial images. Specifically, we generate a tree representation of the aerial images from the output of hierarchical image segmentation algorithms and reformulate the object detection problem into a tree matching task. We then proposed two tree-matching algorithms for the object detection framework. We demonstrate the efficiency and effectiveness of the proposed tree-matching based object detection framework. In the third part, we study object detection in high resolution aerial images from a machine learning perspective. We investigate both traditional machine learning based framework and end-to-end convolutional neural network (CNN) based approach for various object detection tasks. In the traditional detection framework, we propose to apply the Gaussian process classifier (GPC) to train an object detector and demonstrate the advantages of the probabilistic classification algorithm. In the CNN based approach, we proposed a novel scale transfer module that generates enhanced feature maps for object detection. Our results show the efficiency and competitiveness of the proposed algorithms when compared to state-of-the-art counterparts.
Publisher Site: RIT Repository
PDF (Requires Sign-In): PDF
Click for Bibtex
@book{liang2019object, title = {Object Detection in High Resolution Aerial Images and Hyperspectral Remote Sensing Images}, author = {Liang, Yilong}, year = {2019}, school = {Rochester Institute of Technology} }
[D-2019-04] Yashar Seyed Vahedein. (2019). “Methods and Algorithms for Cardiovascular Hemodynamics with Applications to Noninvasive Monitoring of Proximal Blood Pressure and Cardiac Output Using Pulse Transit Time.” Rochester Institute of Technology.
Abstract: Advanced health monitoring and diagnostics technology are essential to reduce the unrivaled number of human fatalities due to cardiovascular diseases (CVDs). Traditionally, gold standard CVD diagnosis involves direct measurements of the aortic blood pressure (central BP) and flow by cardiac catheterization, which can lead to certain complications. Understanding the inner-workings of the cardiovascular system through patient-specific cardiovascular modeling can provide new means to CVD diagnosis and relating treatment. BP and flow waves propagate back and forth from heart to the peripheral sites, while carrying information about the properties of the arterial network. Their speed of propagation, magnitude and shape are directly related to the properties of blood and arterial vasculature. Obtaining functional and anatomical information about the arteries through clinical measurements and medical imaging, the digital twin of the arterial network of interest can be generated. The latter enables prediction of BP and flow waveforms along this network. Point of care devices (POCDs) can now conduct in-home measurements of cardiovascular signals, such as electrocardiogram (ECG), photoplethysmogram (PPG), ballistocardiogram (BCG) and even direct measurements of the pulse transit time (PTT). This vital information provides new opportunities for designing accurate patient-specific computational models eliminating, in many cases, the need for invasive measurements. One of the main efforts in this area is the development of noninvasive cuffless BP measurement using patient's PTT. Commonly, BP prediction is carried out with regression models assuming direct or indirect relationships between BP and PTT. However, accounting for the nonlinear FSI mechanics of the arteries and the cardiac output is indispensable. In this work, a monotonicity-preserving quasi-1D FSI modeling platform is developed, capable of capturing the hyper-viscoelastic vessel wall deformation and nonlinear blood flow dynamics in arbitrary arterial networks. Special attention has been dedicated to the correct modeling of discontinuities, such as mechanical properties mismatch associated with the stent insertion, and the intertwining dynamics of multiscale 3D and 1D models when simulating the arterial network with an aneurysm. The developed platform, titled Cardiovascular Flow ANalysis (CardioFAN), is validated against well-known numerical, in vitro and in vivo arterial network measurements showing average prediction errors of 5.2%, 2.8% and 1.6% for blood flow, lumen cross-sectional area, and BP, respectively. CardioFAN evaluates the local PTT, which enables patient-specific calibration and its application to input signal reconstruction. The calibration is performed based on BP, stroke volume and PTT measured by POCDs. The calibrated model is then used in conjunction with noninvasively measured peripheral BP and PTT to inversely restore the cardiac output, proximal BP and aortic deformation in human subjects. The reconstructed results show average RMSEs of 1.4% for systolic and 4.6% for diastolic BPs, as well as 8.4% for cardiac output. This work is the first successful attempt in implementation of deterministic cardiovascular models as add-ons to wearable and smart POCD results, enabling continuous noninvasive monitoring of cardiovascular health to facilitate CVD diagnosis.
Publisher Site: RIT Repository
PDF (Requires Sign-In): PDF
Click for Bibtex
@book{vahedein2019methods, title = {Methods and Algorithms for Cardiovascular Hemodynamics with Applications to Noninvasive Monitoring of Proximal Blood Pressure and Cardiac Output Using Pulse Transit Time}, author = {Vahedein, Yashar Seyed}, year = {2019}, school = {Rochester Institute of Technology} }
[D-2019-03] Shusil Dangi. (2019). “Computational Methods for Segmentation of Multi-Modal Multi-Dimensional Cardiac Images.” Rochester Institute of Technology.
Abstract: Segmentation of the heart structures helps compute the cardiac contractile function quantified via the systolic and diastolic volumes, ejection fraction, and myocardial mass, representing a reliable diagnostic value. Similarly, quantification of the myocardial mechanics throughout the cardiac cycle, analysis of the activation patterns in the heart via electrocardiography (ECG) signals, serve as good cardiac diagnosis indicators. Furthermore, high quality anatomical models of the heart can be used in planning and guidance of minimally invasive interventions under the assistance of image guidance. The most crucial step for the above mentioned applications is to segment the ventricles and myocardium from the acquired cardiac image data. Although the manual delineation of the heart structures is deemed as the gold-standard approach, it requires significant time and effort, and is highly susceptible to inter- and intra-observer variability. These limitations suggest a need for fast, robust, and accurate semi- or fully-automatic segmentation algorithms. However, the complex motion and anatomy of the heart, indistinct borders due to blood flow, the presence of trabeculations, intensity inhomogeneity, and various other imaging artifacts, makes the segmentation task challenging. In this work, we present and evaluate segmentation algorithms for multi-modal, multi-dimensional cardiac image datasets. Firstly, we segment the left ventricle (LV) blood-pool from a tri-plane 2D+time trans-esophageal (TEE) ultrasound acquisition using local phase based filtering and graph-cut technique, propagate the segmentation throughout the cardiac cycle using non-rigid registration-based motion extraction, and reconstruct the 3D LV geometry. Secondly, we segment the LV blood-pool and myocardium from an open-source 4D cardiac cine Magnetic Resonance Imaging (MRI) dataset by incorporating average atlas based shape constraint into the graph-cut framework and iterative segmentation refinement. The developed fast and robust framework is further extended to perform right ventricle (RV) blood-pool segmentation from a different open-source 4D cardiac cine MRI dataset. Next, we employ convolutional neural network based multi-task learning framework to segment the myocardium and regress its area, simultaneously, and show that segmentation based computation of the myocardial area is significantly better than that regressed directly from the network, while also being more interpretable. Finally, we impose a weak shape constraint via multi-task learning framework in a fully convolutional network and show improved segmentation performance for LV, RV and myocardium across healthy and pathological cases, as well as, in the challenging apical and basal slices in two open-source 4D cardiac cine MRI datasets. We demonstrate the accuracy and robustness of the proposed segmentation methods by comparing the obtained results against the provided gold-standard manual segmentations, as well as with other competing segmentation methods.
Publisher Site: RIT Repository
PDF (Requires Sign-In): PDF
Click for Bibtex
@book{dangi2019computational, title = {Computational Methods for Segmentation of Multi-Modal Multi-Dimensional Cardiac Images}, author = {Dangi, Shusil}, year = {2019}, school = {Rochester Institute of Technology} }
[D-2019-02] Utsav B. Gewali. (2019). “Machine Learning for Robust Understanding of Scene Materials in Hyperspectral Images.” Rochester Institute of Technology.
Abstract: The major challenges in hyperspectral (HS) imaging and data analysis are expensive sensors, high dimensionality of the signal, limited ground truth, and spectral variability. This dissertation develops and analyzes machine learning based methods to address these problems. In the first part, we examine one of the most important HS data analysis tasks-vegetation parameter estimation. We present two Gaussian processes based approaches for improving the accuracy of vegetation parameter retrieval when ground truth is limited and/or spectral variability is high. The first is the adoption of covariance functions based on well-established metrics, such as, spectral angle and spectral correlation, which are known to be better measures of similarity for spectral data. The second is the joint modeling of related vegetation parameters by multitask Gaussian processes so that the prediction accuracy of the vegetation parameter of interest can be improved with the aid of related vegetation parameters for which a larger set of ground truth is available. The efficacy of the proposed methods is demonstrated by comparing them against state-of-the art approaches on three real-world HS datasets and one synthetic dataset. In the second part, we demonstrate how Bayesian optimization can be applied to jointly tune the different components of hyperspectral data analysis frameworks for better performance. Experimental validation on the spatial-spectral classification framework consisting of a classifier and a Markov random field is provided. In the third part, we investigate whether high dimensional HS spectra can be reconstructed from low dimensional multispectral (MS) signals, that can be obtained from much cheaper, lower spectral resolution sensors. A novel end-to-end convolutional residual neural network architecture is proposed that can simultaneously optimize both the MS bands and the transformation to reconstruct HS spectra from MS signals by analyzing a large quantity of HS data. The learned band can be implemented in sensor hardware and the learned transformation can be incorporated in the data processing pipeline to build a low-cost hyperspectral data collection system. Using a diverse set of real-world datasets, we show how the proposed approach of optimizing MS bands along with the transformation rather than just optimizing the transformation with fixed bands, as proposed by previous studies, can drastically increase the reconstruction accuracy. Additionally, we also investigate the prospects of using reconstructed HS spectra for land cover classification.
Publisher Site: RIT Repository
PDF (Requires Sign-In): PDF
Click for Bibtex
@book{gewali2019machine, title = {Machine Learning for Robust Understanding of Scene Materials in Hyperspectral Images}, author = {Gewali, Utsav B.}, year = {2019}, school = {Rochester Institute of Technology} }
[D-2019-01] Di Bai. (2019). “A Hyperspectral Image Classification Approach to Pigment Mapping of Historical Artifacts Using Deep Learning Methods.” Rochester Institute of Technology.
Abstract: Hyperspectral image (HSI) classification has been used to identify material diversity in remote sensing images. Recently, hyperspectral imaging has been applied to historical artifact studies. For example, the Gough Map, one of the earliest surviving maps of Britain, was imaged in 2015 using a hyperspectral imaging system while in the collection at the Bodleian Library, Oxford University. The collection of the HSI data was aimed at pigment analysis for the material diversity of its composition and potentially the timeline of its creation. Traditional methods used spectral unmixing and the spectral angle mapper to classify features in HSIs of historical artifact, those approaches are based only on spectral information of the HSIs. To make full use of both the spatial and spectral features, we developed a novel deep learning technique called 3D-SE-ResNet and applied it to five HSI datasets, including three HSI benchmarks, Indian Pines, Kennedy Space Center, University of Pavia and two HSIs of cultural heritage artifacts, the Gough Map and the Selden Map of China. We trained this deep learning framework to classify pigments in large HSIs with a limited amount of reference (labelled) data automatically. Meanwhile, different spatial and spectral input size and various hyper-parameters of the framework were evaluated. With much less effort and much higher efficiency, this is a breakthrough in object identification and classification in cultural heritage studies that leverages the spectral and spatial information contained in this imagery. Historical geographers, cartographic historians and other scholars will benefit from this work to analyze the pigment mapping of cultural heritage artifacts in the future.
Publisher Site: RIT Repository
PDF (Requires Sign-In): PDF
Click for Bibtex
@book{bai2019a, title = {A Hyperspectral Image Classification Approach to Pigment Mapping of Historical Artifacts Using Deep Learning Methods}, author = {Bai, Di}, year = {2019}, school = {Rochester Institute of Technology} }
[D-2018-11] Brody Kutt. (2018). “Using High-Order Prior Belief Predictions in Hierarchical Temporal Memory for Streaming Anomaly Detection.” Rochester Institute of Technology.
Abstract: Autonomous streaming anomaly detection can have a significant impact in any domain where continuous, real-time data is common. Often in these domains, datasets are too large or complex to hand label. Algorithms that require expensive global training procedures and large training datasets impose strict demands on data and are accordingly not fit to scale to real-time applications that are noisy and dynamic. Unsupervised algorithms that learn continuously like humans therefore boast increased applicability to these real-world scenarios. Hierarchical Temporal Memory (HTM) is a biologically constrained theory of machine intelligence inspired by the structure, activity, organization and interaction of pyramidal neurons in the neocortex of the primate brain. At the core of HTM are spatio-temporal learning algorithms that store, learn, recall and predict temporal sequences in an unsupervised and continuous fashion to meet the demands of real-time tasks. Unlike traditional machine learning and deep learning encompassed by the act of complex functional approximation, HTM with the surrounding proposed framework does not require any offline training procedures, any massive stores of training data, any data labels, it does not catastrophically forget previously learned information and it need only make one pass through the temporal data. Proposed in this thesis is an algorithmic framework built upon HTM for intelligent streaming anomaly detection. Unseen in earlier streaming anomaly detection work, the proposed framework uses high-order prior belief predictions in time in the effort to increase the fault tolerance and complex temporal anomaly detection capabilities of the underlying time-series model. Experimental results suggest that the framework when built upon HTM redefines state-of-the-art performance in a popular streaming anomaly benchmark. Comparative results with and without the framework on several third-party datasets collected from real-world scenarios also show a clear performance benefit. In principle, the proposed framework can be applied to any time-series modeling algorithm capable of producing high-order predictions.
Publisher Site: RIT Repository
PDF (Requires Sign-In): PDF
Click for Bibtex
@book{kutt2018using, title = {Using High-Order Prior Belief Predictions in Hierarchical Temporal Memory for Streaming Anomaly Detection}, author = {Kutt, Brody}, year = {2018}, school = {Rochester Institute of Technology} }
[D-2018-10] Ronald Kemker. (2018). “Low-Shot Learning for the Semantic Segmentation of Remote Sensing Imagery.” Rochester Institute of Technology.
Abstract: Deep-learning frameworks have made remarkable progress thanks to the creation of large annotated datasets such as ImageNet, which has over one million training images. Although this works well for color (RGB) imagery, labeled datasets for other sensor modalities (e.g., multispectral and hyperspectral) are minuscule in comparison. This is because annotated datasets are expensive and man-power intensive to complete; and since this would be impractical to accomplish for each type of sensor, current state-of-the-art approaches in computer vision are not ideal for remote sensing problems. The shortage of annotated remote sensing imagery beyond the visual spectrum has forced researchers to embrace unsupervised feature extracting frameworks. These features are learned on a per-image basis, so they tend to not generalize well across other datasets. In this dissertation, we propose three new strategies for learning feature extracting frameworks with only a small quantity of annotated image data; including 1) self-taught feature learning, 2) domain adaptation with synthetic imagery, and 3) semi-supervised classification. ``Self-taught'' feature learning frameworks are trained with large quantities of unlabeled imagery, and then these networks extract spatial-spectral features from annotated data for supervised classification. Synthetic remote sensing imagery can be used to boot-strap a deep convolutional neural network, and then we can fine-tune the network with real imagery. Semi-supervised classifiers prevent overfitting by jointly optimizing the supervised classification task along side one or more unsupervised learning tasks (i.e., reconstruction). Although obtaining large quantities of annotated image data would be ideal, our work shows that we can make due with less cost-prohibitive methods which are more practical to the end-user.
Publisher Site: RIT Repository
PDF (Requires Sign-In): PDF
Click for Bibtex
@book{kemker2018low-shot, title = {Low-Shot Learning for the Semantic Segmentation of Remote Sensing Imagery}, author = {Kemker, Ronald}, year = {2018}, school = {Rochester Institute of Technology} }
[D-2018-09] Isaac Bernabe Perez-Raya. (2018). “Numerical Investigation of Heat and Mass Transfer Phenomena in Boiling.” Rochester Institute of Technology.
Abstract: Applications boiling are found in heat sinks for electronics cooling, nuclear and fossil fuel powered steam generators, distillation columns, concentrated solar power systems, glass melting furnaces, desalination chambers, and heat and mass exchangers. In order to increase the performance and safety margins of these applications, there is a need to develop tools that predict the thermal and fluid behavior during bubble growth. The analysis of boiling has been addressed by computer simulations, which employ methods for approximating mass and heat transfer at the interface. However, most simulations make assumptions that could adversely affect the prediction of the thermal and dynamic fluid behavior near the bubble-edge. These assumptions include: (i) computation of mass transfer with local temperature differences or with temperature gradients at cell-centers rather than with temperature gradients at the interface, (ii) modified discretization schemes at neighboring-cells or a transition region to account for the interface saturation temperature, and (iii) interface smearing or distribution of mass transfer into multiple cells around the interface to prevent interface deformations. The present work proposes methods to perform a simulation of nucleate boiling. The proposed methods compute mass transfer with temperature gradients at the interface, account for the interface saturation temperature, and model a sharp interface (interface within one cell) with mass transfer only at interface-cells. The proposed methods lead to a more realistic representation of the heat and mass transfer at the interface. Results of the simulation are in excellent agreement with theory on planar interface evaporation and growth of spherical bubbles in superheated liquid. In addition, numerical bubble growth rates compare well with experimental data on single bubble nucleation over a heated surface. The simulation of nucleate boiling with water and a 6.2 K wall superheat reveals large heat transfer coefficients over a 200 um distance from the interface. In addition, analyses of the wall shear stress indicate an influence region of two-times the departure bubble diameter.
Publisher Site: RIT Repository
PDF (Requires Sign-In): PDF
Click for Bibtex
@book{perez-raya2018numerical, title = {Numerical Investigation of Heat and Mass Transfer Phenomena in Boiling}, author = {Perez-Raya, Isaac Bernabe}, year = {2018}, school = {Rochester Institute of Technology} }
[D-2018-08] Chao Zhang. (2018). “Functional Imaging Connectome of the Human Brain and its Associations with Biological and Behavioral Characteristics.” Rochester Institute of Technology.
Abstract: Functional connectome of the human brain explores the temporal associations of different brain regions. Functional connectivity (FC) measures derived from resting state functional magnetic resonance imaging (rfMRI) characterize the brain network at rest and studies have shown that rfMRI FC is closely related to individual subject's biological and behavioral measures. In this thesis we investigate a large rfMRI dataset from the Human Connectome Project (HCP) and utilize statistical methods to facilitate the understanding of fundamental FC-behavior associations of the human brain. Our studies include reliability analysis of FC statistics, demonstration of FC spatial patterns, and predictive analysis of individual biological and behavioral measures using FC features. Covering both static and dynamic FC (sFC and dFC) characterizations, the baseline FC patterns in healthy young adults are illustrated. Predictive analyses demonstrate that individual biological and behavioral measures, such as gender, age, fluid intelligence and language scores, can be predicted using FC. While dFC by itself performs worse than sFC in prediction accuracy, if appropriate parameters and models are utilized, adding dFC features to sFC can significantly increase the predictive power. Results of this thesis contribute to the understanding of the neural underpinnings of individual biological and behavioral differences in the human brain.
Publisher Site: RIT Repository
PDF (Requires Sign-In): PDF
Click for Bibtex
@book{zhang2018functional, title = {Functional Imaging Connectome of the Human Brain and its Associations with Biological and Behavioral Characteristics}, author = {Zhang, Chao}, year = {2018}, school = {Rochester Institute of Technology} }
[D-2018-07] Pruthvik A. Raghupathi. (2018). “On Contact Line Region Heat Transfer, Bubble Dynamics and Substrate Effects During Boiling.” Rochester Institute of Technology.
Abstract: Rapid advancement of electronics used in domestic, commercial and military applications has necessitated the development of thermal management solutions capable of dissipating large amounts of heat in a reliable and efficient manner. Traditional methods of cooling, including air and liquid cooling, require large fluid flow rates and temperature differences to remove high heat fluxes and are therefore unsuited for many advanced applications. Phase change heat transfer, specifically boiling, is capable of dissipating large heat fluxes with low temperature gradients and hence is an attractive technique for cooling high heat flux applications. However, due to the complex interactions between the fluid dynamics, heat transfer, and surface chemistry, the fundamental physics associated with boiling is not completely understood. The focus of this work is to get a better understanding of the role played by a nucleating bubble in removing the heat from the substrate. The interfacial forces acting on a bubble, contact line motion, and the thermal interaction with the heater surfaces are some of the important considerations which have not been well understood in literature. The work reported in this dissertation is divided into three parts. In the first part, an analytical study of the effect of evaporation momentum force on bubble growth rate and bubble trajectory was conducted. It was shown that the trajectory of a bubble can be controlled by creating an asymmetric temperature field. This understanding was used to develop a bubble diverter that increased the Critical Heat Flux (CHF) over a horizontal tubular surface by 60% and improved the heat transfer coefficient by 75%. In the second part of the work, additional contact line regions were generated using microgrooves. This enhancement technique increased the CHF with water by 46% over a plain copper surface to 187 W/cm2. Finally, the effect of the heater properties and surface fouling during boiling was evaluated. This included a study on the effect of thermophysical properties of the heater surface on CHF and an investigation of fouling over a heater surface during boiling of seawater.
Publisher Site: RIT Repository
PDF (Requires Sign-In): PDF
Click for Bibtex
@book{raghupathi2018on, title = {On Contact Line Region Heat Transfer, Bubble Dynamics and Substrate Effects During Boiling}, author = {Raghupathi, Pruthvik A.}, year = {2018}, school = {Rochester Institute of Technology} }
[D-2018-06] Brittany L. Smith. (2018). “Development and Life Cycle Assessment of Advanced-concept III-V Multijunction Photovoltaics.” Rochester Institute of Technology.
Abstract: III-V semiconductors make for highly efficient solar cells, but are expensive to manufacture. However, there are many mechanisms for improving III-V photovoltaics in order to make them more competitive with other photovoltaic (PV) technologies. One possible method is to design cells for high efficiency under concentrated sunlight, effectively trading expensive III-V material for cheaper materials such as glass lenses. Another approach is to reduce the amount of III-V material necessary for the same power output, which can be achieved by removing the substrate and installing a reflector on the back of the cell, while also adding quantum structures to the cell to permit absorption of a greater portion of the solar spectrum. Regarding the first approach, this dissertation focused on the development of an InAlAsSb material for a mulitjunction design with the potential of achieving 52.8% efficiency under 500 suns. First, development of a single-junction InAlAs cell lattice-matched to InP was executed as a preliminary step. The InAlAs cell design was optimized via simulation, then grown via metal organic vapor phase epitaxy (MOVPE) and fabricated resulting in 17.9% efficiency under 1-sun AM1.5, which was unprecedented for the InAlAs material. Identical InAlAs cells were grown using alternative MOVPE precursors to study the effects of necessary precursors for InAlAsSb. Fits to experimental device results showed longer lifetimes when grown with the alternative aluminum precursor. InAlAsSb grown using these alternative precursors targeted a 1.8 eV bandgap required for the multijunction design. Ultimately, InAlAsSb material with the desired bandgap was confirmed by photoreflectance spectroscopy. For the second approach, this dissertation studied the integration of InAs quantum dots (QDs) in a GaAs solar cell in conjunction a back surface reflector (BSR). A quantum dot solar cell (QDSC) with a BSR has the potential to increase short-circuit current by 2.5 mA/cm2 and also increase open-circuit voltage due to photon recycling. In this study, multiple textured BSRs were fabricated by growing inverted QDSCs on epitaxial lift-off templates and then texturing the rear surface before removing the device from the substrate. Identical cells with a flat BSR served as controls. Optimization of inverted QDSC growth conditions was also performed via a cell design study. Device results showed increased open-circuit voltage with increasing optical path length, and the greatest improvement in sub-band current over a flat BSR control device was 40%. In the final chapter, a life cycle assessment (LCA) of these technologies was performed to identify the hypothetical optimum at which energy investments in cell performance (such as the two described above) no longer correspond to improvements in the overall life cycle performance of the PV system. Four cell designs with sequentially increasing efficiencies were compared using a functional unit of 1 kWp. The first is a commercially available and has been studied in previous LCAs. The second is the design containing InAlAsSb mentioned above. The third represents the most material-intensive option, which bonds two substrates to create a five-junction cell. The fourth is a six-junction cell that uses a metamorphic grade between subcells and represents the most energy-intensive option. A thorough literature review of existing LCAs of high-concentration photovoltaic (HCPV) systems was performed, which obviated the need for data on the manufacture of MOVPE precursors and substrates. LCAs for the most common III-V substrate (GaAs) and precursors were executed prior to conducting the HCPV system LCA, due to the absence of detailed information on the life cycle impacts of these compounds in literature. Ultimately, both the cumulative energy demand and greenhouse gas emissions of the HCPV system decreased proportionally with increasing cell efficiency, even for the most energy and material-intensive cell designs. It was found that the substrates and precursors corresponded to less than 2% of system impacts. This implies that current mechanisms to increase cell efficiency are environmentally viable in HCPV applications without the need for material reduction, and would make III-V HCPV more environmentally competitive with dominant silicon PV technologies.
Publisher Site: RIT Repository
PDF (Requires Sign-In): PDF
Click for Bibtex
@book{smith2018development, title = {Development and Life Cycle Assessment of Advanced-concept III-V Multijunction Photovoltaics}, author = {Smith, Brittany L.}, year = {2018}, school = {Rochester Institute of Technology} }
[D-2018-05] Greg Badura. (2018). “The Photometric Effect of Macroscopic Surface Roughness on Sediment Surfaces.” Rochester Institute of Technology.
Abstract: The focus of this work was on explaining the effect of macroscopic surface roughness on the reflected light from a soil surface. These questions extend from deciding how to best describe roughness mathematically, to figuring out how to quantify its effect on the spectral reflectance from a soil's surface. In this document, I provide a background of the fundamental literature in the fields of remote sensing and computer vision that have been instrumental in my research. I then outline the software and hardware tools that I have developed to quantify roughness. This includes a detailed outline of a custom "LiDAR" operating mode for the GRIT-T goniometer system that was developed and characterized over the course of this research, as well as proposed methods for using convergent images acquired by our goniometer system's camera to derive useful structure from motion point clouds. These tools and concepts are then used in two experiments that aim to explain the relationship between soil surface roughness and spectral BRF phenomena. In the first experiment, clay sediment samples were gradually pulverized into a smooth powderized state and in steps of reduced surface roughness. Results show that variance in the continuum spectra as a function of viewing angle increased with the roughness of the sediment surface. This result suggests that inter-facet multiple scattering caused a variance in absorption band centering and depth due to an increased path length traveled through the medium. In the second experiment, we examine the performance of the Hapke photometric roughness correction for sand sediment surfaces of controlled sample density. We find that the correction factor potentially underpredicts the effect of shadowing in the forward scattering direction. The percentage difference between forward-modeled BRF measurements and empirically measured BRF measurements is constant across wavelength, suggesting that a factor can be empirically derived. Future results should also investigate the scale at which the photometric correction factor should be applied. Finally, I also outline a structure from motion processing chain aimed at deriving meaningful metrics of vegetation structure. Results show that correlations between these metrics and observed directional reflectance phenomena of chordgrass are strong for peak growing state plants. We observe good agreement between destructive LAI metrics and contact-based LAI metrics.
Publisher Site: RIT Repository
PDF (Requires Sign-In): PDF
Click for Bibtex
@book{badura2018the, title = {The Photometric Effect of Macroscopic Surface Roughness on Sediment Surfaces}, author = {Badura, Greg}, year = {2018}, school = {Rochester Institute of Technology} }
[D-2018-04] Chi Zhang. (2018). “Evolution of A Common Vector Space Approach to Multi-Modal Problems.” Rochester Institute of Technology.
Abstract: A set of methods to address computer vision problems has been developed. Video un- derstanding is an activate area of research in recent years. If one can accurately identify salient objects in a video sequence, these components can be used in information retrieval and scene analysis. This research started with the development of a course-to-fine frame- work to extract salient objects in video sequences. Previous work on image and video frame background modeling involved methods that ranged from simple and efficient to accurate but computationally complex. It will be shown in this research that the novel approach to implement object extraction is efficient and effective that outperforms the existing state-of-the-art methods. However, the drawback to this method is the inability to deal with non-rigid motion. With the rapid development of artificial neural networks, deep learning approaches are explored as a solution to computer vision problems in general. Focusing on image and text, the image (or video frame) understanding can be achieved using CVS. With this concept, modality generation and other relevant applications such as automatic im- age description, text paraphrasing, can be explored. Specifically, video sequences can be modeled by Recurrent Neural Networks (RNN), the greater depth of the RNN leads to smaller error, but that makes the gradient in the network unstable during training.To overcome this problem, a Batch-Normalized Recurrent Highway Network (BNRHN) was developed and tested on the image captioning (image-to-text) task. In BNRHN, the highway layers are incorporated with batch normalization which diminish the gradient vanishing and exploding problem. In addition, a sentence to vector encoding framework that is suitable for advanced natural language processing is developed. This semantic text embedding makes use of the encoder-decoder model which is trained on sentence paraphrase pairs (text-to-text). With this scheme, the latent representation of the text is shown to encode sentences with common semantic information with similar vector rep- resentations. In addition to image-to-text and text-to-text, an image generation model is developed to generate image from text (text-to-image) or another image (image-to- image) based on the semantics of the content. The developed model, which refers to the Multi-Modal Vector Representation (MMVR), builds and encodes different modalities into a common vector space that achieve the goal of keeping semantics and conversion between text and image bidirectional. The concept of CVS is introduced in this research to deal with multi-modal conversion problems. In theory, this method works not only on text and image, but also can be generalized to other modalities, such as video and audio. The characteristics and performance are supported by both theoretical analysis and experimental results. Interestingly, the MMVR model is one of the many possible ways to build CVS. In the final stages of this research, a simple and straightforward framework to build CVS, which is considered as an alternative to the MMVR model, is presented.
Publisher Site: RIT Repository
PDF (Requires Sign-In): PDF
Click for Bibtex
@book{zhang2018evolution, title = {Evolution of A Common Vector Space Approach to Multi-Modal Problems}, author = {Zhang, Chi}, year = {2018}, school = {Rochester Institute of Technology} }
[D-2018-03] Shagan Sah. (2018). “Multi-Modal Deep Learning to Understand Vision and Language.” Rochester Institute of Technology.
Abstract: Developing intelligent agents that can perceive and understand the rich visual world around us has been a long-standing goal in the field of artificial intelligence. In the last few years, significant progress has been made towards this goal and deep learning has been attributed to recent incredible advances in general visual and language understanding. Convolutional neural networks have been used to learn image representations while recurrent neural networks have demonstrated the ability to generate text from visual stimuli. In this thesis, we develop methods and techniques using hybrid convolutional and recurrent neural network architectures that connect visual data and natural language utterances. Towards appreciating these methods, this work is divided into two broad groups. Firstly, we introduce a general purpose attention mechanism modeled using a continuous function for video understanding. The use of an attention based hierarchical approach along with automatic boundary detection advances state-of-the-art video captioning results. We also develop techniques for summarizing and annotating long videos. In the second part, we introduce architectures along with training techniques to produce a common connection space where natural language sentences are efficiently and accurately connected with visual modalities. In this connection space, similar concepts lie close, while dissimilar concepts lie far apart, irrespective` of their modality. We discuss four modality transformations: visual to text, text to visual, visual to visual and text to text. We introduce a novel attention mechanism to align multi-modal embeddings which are learned through a multi-modal metric loss function. The common vector space is shown to enable bidirectional generation of images and text. The learned common vector space is evaluated on multiple image-text datasets for cross-modal retrieval and zero-shot retrieval. The models are shown to advance the state-of-the-art on tasks that require joint processing of images and natural language.
Publisher Site: RIT Repository
PDF (Requires Sign-In): PDF
Click for Bibtex
@book{sah2018multi-modal, title = {Multi-Modal Deep Learning to Understand Vision and Language}, author = {Sah, Shagan}, year = {2018}, school = {Rochester Institute of Technology} }
[D-2018-02] Zichao Han. (2018). “The Design and Realization of a Dual Mode Photoacoustic and Ultrasound Imaging Camera.” Rochester Institute of Technology.
Abstract: Prostate cancer is currently the second leading cause of cancer death in American men. Diagnosis of the disease is based on persisting elevated prostate-specific antigen (PSA) levels and suspicious lesion felt on digital rectal examination (DRE), prompting transrectal ultrasound (TRUS) imaging guided biopsy. This method, however, has long been criticized for its poor sensitivity in detecting cancerous lesions, leading to the fact that these biopsies generally are not targeted but systematic multi-core in nature that try to sample the entire gland. The thesis presents a new modality that, in combination of ultrasound (US) imaging with multi-wavelength photoacoustic (PA) imaging, improves the physician's ability to locate the suspicious cancerous regions during biopsy. Here, building further on the innovation of an acoustic lens based focusing technology for fast PA imaging, a novel concept with the use of a polyvinylidene fluoride (PVDF) film that incorporates US imaging into our existing PA imaging probe is presented. The method takes advantage of the lens based PA signal focusing technology, while simultaneously incorporates US imaging modality without interfering with the current PA imaging system design and structure. Simulation and experimental support on tissue equivalent phantoms is provided in detail. The thesis also elaborates on the signal-to-noise ratio (SNR) improvement of the US imaging component by driving the film with frequency modulated (FM) signals. In addition, a custom-designed US simulation software that is developed to explore and evaluate various system design options is discussed. The dual modality transrectal probe is only intended as a first step. The long term goal of the study is to facilitate locating the cancer region in-vivo with PA imaging, transfer it to co-registered US image, and use the real-time US imaging for needle guidance during biopsy.
Publisher Site: RIT Repository
PDF (Requires Sign-In): PDF
Click for Bibtex
@book{han2018the, title = {The Design and Realization of a Dual Mode Photoacoustic and Ultrasound Imaging Camera}, author = {Han, Zichao}, year = {2018}, school = {Rochester Institute of Technology} }
[D-2018-01] Zhaoyu Cui. (2018). “System Engineering Analyses for the Study of Future Multispectral Land Imaging Satellite Sensors for Vegetation Monitoring.” Rochester Institute of Technology.
Abstract: Vegetation monitoring is one of the key applications of earth observing systems. Landsat data have spatial resolution of 30 meters, moderate temporal coverage, and reasonable spectral sampling to capture key vegetation features. These characteristics of Landsat make it a good candidate for generating vegetation monitoring products. Recently, the next satellite in the Landsat series has been under consideration and different concepts have been proposed. In this research, we studied the impact on vegetation monitoring of two proposed potential design concepts: a wider field-of-view (FOV) instrument and the addition of red-edge spectral band(s). Three aspects were studied in this thesis: First, inspired by the potential wider FOV design, the impacts of a detector relative spectral response (RSR) central wavelength shift effect at high angles of incidence (AOI) on the radiance signal were studied and quantified. Results indicate: 1) the RSR shift effect is band-dependent and more significant in the green, red and SWIR 2 bands; 2) At high AOI, the impact of the RSR shift effect will exceed sensor noise specifications in all bands except the SWIR 1 band; and 3) The RSR shift will cause SWIR2 band more to be sensitive to atmospheric conditions. Second, also inspired by the potential wider FOV design, the impacts of the potential new wider angular observations on vegetation monitoring scientific products were studied. Both crop classification and biophysical quantity retrieval applications were studied using the simulation code DIRSIG and the canopy radiative transfer model PROSAIL. It should be noted that the RSR shift effect was also considered. Results show that for single view observation based analysis, the higher view angular observations have limited influence on both applications. However, for situations where two different angular observations are available potentially from two platforms, up to 4% improvement for crop classification and 2.9% improvement for leaf chlorophyll content retrieval were found. Third, to quantify the benefits of a potential new design with red-edge band(s), the impact of adding red-edge spectral band(s) in future Landsat instruments on agroecosystem leaf area index (LAI) and canopy chlorophyll content (CCC) retrieval were studied using a real dataset. Three major retrieval approaches were tested, results show that a potential new spectral band located between the Landsat-8 Operational Land Imager (OLI) red and NIR bands slightly improved the retrieval accuracy (LAI: R2 of 0.787 vs. 0.810 for empirical vegetation index regression approach, 0.806 vs. 0.828 for look-up-table inversion approach, and 0.925 vs. 0.933 for machine learning approach; CCC: R2 of 0.853 vs. 0.875 for empirical vegetation index regression approach, 0.500 vs. 0.570 for look-up-table inversion approach, and 0.854 vs. 0.887 for machine learning approach). In general, for the potential wider FOV design, the RSR shift effect was found to cause noticable radiance signal difference that is higher than detector noise in all OLI bands except SWIR1 band, which is not observed in the current OLI design with its 15 degree FOV. Also both the new wider angular observations and potential red-edge band(s) were found to slightly improve the vegetation monitoring product accuracy. In the future, the RSR shift effect in other optical designs should be evaluated since this study assumed the angle reaching the filter array is the same as the angle reaching the sensor. In addition to improve the accuracy of the off angle imaging study, a 3D vegetation geometry model should be explored for vegetation monitoring related studies instead of the 2D PROSAIL model used in this thesis.
Publisher Site: RIT Repository
PDF (Requires Sign-In): PDF
Click for Bibtex
@book{cui2018system, title = {System Engineering Analyses for the Study of Future Multispectral Land Imaging Satellite Sensors for Vegetation Monitoring}, author = {Cui, Zhaoyu}, year = {2018}, school = {Rochester Institute of Technology} }
[D-2017-16] Lei Fan. (2017). “Graph-based Data Modeling and Analysis for Data Fusion in Remote Sensing.” Rochester Institute of Technology.
Abstract: Hyperspectral imaging provides the capability of increased sensitivity and discrimination over traditional imaging methods by combining standard digital imaging with spectroscopic methods. For each individual pixel in a hyperspectral image (HSI), a continuous spectrum is sampled as the spectral reflectance/radiance signature to facilitate identification of ground cover and surface material. The abundant spectrum knowledge allows all available information from the data to be mined. The superior qualities within hyperspectral imaging allow wide applications such as mineral exploration, agriculture monitoring, and ecological surveillance, etc. The processing of massive high-dimensional HSI datasets is a challenge since many data processing techniques have a computational complexity that grows exponentially with the dimension. Besides, a HSI dataset may contain a limited number of degrees of freedom due to the high correlations between data points and among the spectra. On the other hand, merely taking advantage of the sampled spectrum of individual HSI data point may produce inaccurate results due to the mixed nature of raw HSI data, such as mixed pixels, optical interferences and etc. Fusion strategies are widely adopted in data processing to achieve better performance, especially in the field of classification and clustering. There are mainly three types of fusion strategies, namely low-level data fusion, intermediate-level feature fusion, and high-level decision fusion. Low-level data fusion combines multi-source data that is expected to be complementary or cooperative. Intermediate-level feature fusion aims at selection and combination of features to remove redundant information. Decision level fusion exploits a set of classifiers to provide more accurate results. The fusion strategies have wide applications including HSI data processing. With the fast development of multiple remote sensing modalities, e.g. Very High Resolution (VHR) optical sensors, LiDAR, etc., fusion of multi-source data can in principal produce more detailed information than each single source. On the other hand, besides the abundant spectral information contained in HSI data, features such as texture and shape may be employed to represent data points from a spatial perspective. Furthermore, feature fusion also includes the strategy of removing redundant and noisy features in the dataset. One of the major problems in machine learning and pattern recognition is to develop appropriate representations for complex nonlinear data. In HSI processing, a particular data point is usually described as a vector with coordinates corresponding to the intensities measured in the spectral bands. This vector representation permits the application of linear and nonlinear transformations with linear algebra to find an alternative representation of the data. More generally, HSI is multi-dimensional in nature and the vector representation may lose the contextual correlations. Tensor representation provides a more sophisticated modeling technique and a higher-order generalization to linear subspace analysis. In graph theory, data points can be generalized as nodes with connectivities measured from the proximity of a local neighborhood. The graph-based framework efficiently characterizes the relationships among the data and allows for convenient mathematical manipulation in many applications, such as data clustering, feature extraction, feature selection and data alignment. In this thesis, graph-based approaches applied in the field of multi-source feature and data fusion in remote sensing area are explored. We will mainly investigate the fusion of spatial, spectral and LiDAR information with linear and multilinear algebra under graph-based framework for data clustering and classification problems.
Publisher Site: RIT Repository
PDF (Requires Sign-In): PDF
Click for Bibtex
@book{fan2017graph-based, title = {Graph-based Data Modeling and Analysis for Data Fusion in Remote Sensing}, author = {Fan, Lei}, year = {2017}, school = {Rochester Institute of Technology} }
[D-2017-15] Md Shahriar Shamim. (2017). “Overcoming the Challenges for Multichip Integration: A Wireless Interconnect Approach.” Rochester Institute of Technology.
Abstract: The physical limitations in the area, power density, and yield restrict the scalability of the single-chip multicore system to a relatively small number of cores. Instead of having a large chip, aggregating multiple smaller chips can overcome these physical limitations. Combining multiple dies can be done either by stacking vertically or by placing side-by-side on the same substrate within a single package. However, in order to be widely accepted, both multichip integration techniques need to overcome significant challenges. In the horizontally integrated multichip system, traditional inter-chip I/O does not scale well with technology scaling due to limitations of the pitch. Moreover, to transfer data between cores or memory components from one chip to another, state-of-the-art inter-chip communication over wireline channels require data signals to travel from internal nets to the peripheral I/O ports and then get routed over the inter-chip channels to the I/O port of the destination chip. Following this, the data is finally routed from the I/O to internal nets of the target chip over a wireline interconnect fabric. This multi-hop communication increases energy consumption while decreasing data bandwidth in a multichip system. On the other hand, in vertically integrated multichip system, the high power density resulting from the placement of computational components on top of each other aggravates the thermal issues of the chip leading to degraded performance and reduced reliability. Liquid cooling through microfluidic channels can provide cooling capabilities required for effective management of chip temperatures in vertical integration. However, to reduce the mechanical stresses and at the same time, to ensure temperature uniformity and adequate cooling competencies, the height and width of the microchannels need to be increased. This limits the area available to route Through-Silicon-Vias (TSVs) across the cooling layers and make the co-existence and co-design of TSVs and microchannels extreamly challenging. Research in recent years has demonstrated that on-chip and off-chip wireless interconnects are capable of establishing radio communications within as well as between multiple chips. The primary goal of this dissertation is to propose design principals targeting both horizontally and vertically integrated multichip system to provide high bandwidth, low latency, and energy efficient data communication by utilizing mm-wave wireless interconnects. The proposed solution has two parts: the first part proposes design methodology of a seamless hybrid wired and wireless interconnection network for the horizontally integrated multichip system to enable direct chip-to-chip communication between internal cores. Whereas the second part proposes a Wireless Network-on-Chip (WiNoC) architecture for the vertically integrated multichip system to realize data communication across interlayer microfluidic coolers eliminating the need to place and route signal TSVs through the cooling layers. The integration of wireless interconnect will significantly reduce the complexity of the co-design of TSV based interconnects and microchannel based interlayer cooling. Finally, this dissertation presents a combined trade-off evaluation of such wireless integration system in both horizontal and vertical sense and provides future directions for the design of the multichip system.
Publisher Site: RIT Repository
PDF (Requires Sign-In): PDF
Click for Bibtex
@book{shamim2017overcoming, title = {Overcoming the Challenges for Multichip Integration: A Wireless Interconnect Approach}, author = {Shamim, Md Shahriar}, year = {2017}, school = {Rochester Institute of Technology} }
[D-2017-14] Kelly Laraby. (2017). “Landsat Surface Temperature Product: Global Validation and Uncertainty Estimation.” Rochester Institute of Technology.
Abstract: Surface temperature is an important Earth system data record that is useful to fields such as change detection, climate research, environmental monitoring, and many smaller scale applications like agriculture. Earth-observing satellites can be used to derive this metric, with the goal that a global product can be established. There are a series of Landsat satellites designed for this purpose, whose data archives provides the longest running source of continuously acquired multispectral imagery. The moderate spatial and temporal resolution, in addition to its well calibrated sensors and data archive make Landsat an unparalleled and attractive choice for many research applications. Through the support of the National Aeronautics and Space Administration (NASA) and the United States Geological Survey (USGS), a Landsat Surface Temperature product (LST) has been developed. Currently, it has been validated for Landsat 5 scenes in North America, and Landsat 7 on a global scale. Transmission and cloud proximity were used to characterize LST error for various conditions, which showed that 30% of the validation data had root mean squared errors (RMSEs) less than 1 K, and 62% had RMSEs less than 2 K. Transmission and cloud proximity were also used to develop a LST uncertainty estimation method, which will allow the user to choose data points that meet their accuracy requirements. For the same dataset, about 20% reported LST uncertainties less than 1 K, and 63% had uncertainties less than 2 K. Enabling global validation and establishing an uncertainty estimation method were crucially important achievements for the LST product, which is now ready to be implemented and scaled so that it is available to the public. This document will describe the LST algorithm in full, and it will also discuss the validation results and uncertainty estimation process.
Publisher Site: RIT Repository
PDF (Requires Sign-In): PDF
Click for Bibtex
@book{laraby2017landsat, title = {Landsat Surface Temperature Product: Global Validation and Uncertainty Estimation}, author = {Laraby, Kelly}, year = {2017}, school = {Rochester Institute of Technology} }
[D-2017-13] Gajendra Jung Katuwal. (2017). “Machine Learning Based Autism Detection Using Brain Imaging.” Rochester Institute of Technology.
Abstract: Autism Spectrum Disorder (ASD) is a group of heterogeneous developmental disabilities that manifest in early childhood. Currently, ASD is primarily diagnosed by assessing the behavioral and intellectual abilities of a child. This behavioral diagnosis can be subjective, time consuming, inconclusive, does not provide insight on the underlying etiology, and is not suitable for early detection. Diagnosis based on brain magnetic resonance imaging (MRI)—a widely used non- invasive tool—can be objective, can help understand the brain alterations in ASD, and can be suitable for early diagnosis. However, the brain morphological findings in ASD from MRI studies have been inconsistent. Moreover, there has been limited success in machine learning based ASD detection using MRI derived brain features. In this thesis, we begin by demonstrating that the low success in ASD detection and the inconsistent findings are likely attributable to the heterogeneity of brain alterations in ASD. We then show that ASD detection can be significantly improved by mitigating the heterogeneity with the help of behavioral and demographics information. Here we demonstrate that finding brain markers in well-defined sub-groups of ASD is easier and more insightful than identifying markers across the whole spectrum. Finally, our study focused on brain MRI of a pediatric cohort (3 to 4 years) and achieved a high classification success (AUC of 95%). Results of this study indicate three main alterations in early ASD brains: 1) abnormally large ventricles, 2) highly folded cortices, and 3) low image intensity in white matter regions suggesting myelination deficits indicative of decreased structural connectivity. Results of this thesis demonstrate that the meaningful brain markers of ASD can be extracted by applying machine learning techniques on brain MRI data. This data-driven technique can be a powerful tool for early detection and understanding brain anatomical underpinnings of ASD.
Publisher Site: RIT Repository
PDF (Requires Sign-In): PDF
Click for Bibtex
@book{katuwal2017machine, title = {Machine Learning Based Autism Detection Using Brain Imaging}, author = {Katuwal, Gajendra Jung}, year = {2017}, school = {Rochester Institute of Technology} }
[D-2017-12] Yehuda K. Ben-Zikri. (2017). “Development, Implementation and Pre-clinical Evaluation of Medical Image Computing Tools in Support of Computer-aided Diagnosis: Respiratory, Orthopedic and Cardiac Applications.” Rochester Institute of Technology.
Abstract: Over the last decade, image processing tools have become crucial components of all clinical and research efforts involving medical imaging and associated applications. The imaging data available to the radiologists continue to increase their workload, raising the need for efficient identification and visualization of the required image data necessary for clinical assessment. Computer-aided diagnosis (CAD) in medical imaging has evolved in response to the need for techniques that can assist the radiologists to increase throughput while reducing human error and bias without compromising the outcome of the screening, diagnosis or disease assessment. More intelligent, but simple, consistent and less time-consuming methods will become more widespread, reducing user variability, while also revealing information in a more clear, visual way. Several routine image processing approaches, including localization, segmentation, registration, and fusion, are critical for enhancing and enabling the development of CAD techniques. However, changes in clinical workflow require significant adjustments and re-training and, despite the efforts of the academic research community to develop state-of-the-art algorithms and high-performance techniques, their footprint often hampers their clinical use. Currently, the main challenge seems to not be the lack of tools and techniques for medical image processing, analysis, and computing, but rather the lack of clinically feasible solutions that leverage the already developed and existing tools and techniques, as well as a demonstration of the potential clinical impact of such tools. Recently, more and more efforts have been dedicated to devising new algorithms for localization, segmentation or registration, while their potential and much intended clinical use and their actual utility is dwarfed by the scientific, algorithmic and developmental novelty that only result in incremental improvements over already algorithms. In this thesis, we propose and demonstrate the implementation and evaluation of several different methodological guidelines that ensure the development of image processing tools --- localization, segmentation and registration --- and illustrate their use across several medical imaging modalities --- X-ray, computed tomography, ultrasound and magnetic resonance imaging --- and several clinical applications: Lung CT image registration in support for assessment of pulmonary nodule growth rate and disease progression from thoracic CT images. Automated reconstruction of standing X-ray panoramas from multi-sector X-ray images for assessment of long limb mechanical axis and knee misalignment. Left and right ventricle localization, segmentation, reconstruction, ejection fraction measurement from cine cardiac MRI or multi-plane trans-esophageal ultrasound images for cardiac function assessment. When devising and evaluating our developed tools, we use clinical patient data to illustrate the inherent clinical challenges associated with highly variable imaging data that need to be addressed before potential pre-clinical validation and implementation. In an effort to provide plausible solutions to the selected applications, the proposed methodological guidelines ensure the development of image processing tools that help achieve sufficiently reliable solutions that not only have the potential to address the clinical needs, but are sufficiently streamlined to be potentially translated into eventual clinical tools provided proper implementation. G1: Reducing the number of degrees of freedom (DOF) of the designed tool, with a plausible example being avoiding the use of inefficient non-rigid image registration methods. This guideline addresses the risk of artificial deformation during registration and it clearly aims at reducing complexity and the number of degrees of freedom. G2: The use of shape-based features to most efficiently represent the image content, either by using edges instead of or in addition to intensities and motion, where useful. Edges capture the most useful information in the image and can be used to identify the most important image features. As a result, this guideline ensures a more robust performance when key image information is missing. G3: Efficient method of implementation. This guideline focuses on efficiency in terms of the minimum number of steps required and avoiding the recalculation of terms that only need to be calculated once in an iterative process. An efficient implementation leads to reduced computational effort and improved performance. G4: Commence the workflow by establishing an optimized initialization and gradually converge toward the final acceptable result. This guideline aims to ensure reasonable outcomes in consistent ways and it avoids convergence to local minima, while gradually ensuring convergence to the global minimum solution. These guidelines lead to the development of interactive, semi-automated or fully-automated approaches that still enable the clinicians to perform final refinements, while they reduce the overall inter- and intra-observer variability, reduce ambiguity, increase accuracy and precision, and have the potential to yield mechanisms that will aid with providing an overall more consistent diagnosis in a timely fashion.
Publisher Site: RIT Repository
PDF (Requires Sign-In): PDF
Click for Bibtex
@book{ben-zikri2017development,, title = {Development, Implementation and Pre-clinical Evaluation of Medical Image Computing Tools in Support of Computer-aided Diagnosis: Respiratory, Orthopedic and Cardiac Applications}, author = {Ben-Zikri, Yehuda K.}, year = {2017}, school = {Rochester Institute of Technology} }
[D-2017-11] Zack Fitzsimmons. (2017). “Election-Attack Complexity for More Natural Models.” Rochester Institute of Technology.
Abstract: Elections are arguably the best way that a group of agents with preferences over a set of choices can reach a decision. This can include political domains, as well as multiagent systems in artificial-intelligence settings. It is well-known that every reasonable election system is manipulable, but determining whether such a manipulation exists may be computationally infeasible. We build on an exciting line of research that considers the complexity of election-attack problems, which include voters misrepresenting their preferences (manipulation) and attacks on the structure of the election itself (control). We must properly model such attacks and the preferences of the electorate to give us insight into the difficulty of election attacks in natural settings. This includes models for how the voters can state their preferences, their structure, and new models for the election attack itself. We study several different natural models on the structure of the voters. In the computational study of election attacks it is generally assumed that voters strictly rank all of the candidates from most to least preferred. We consider the very natural model where voters are able to cast votes with ties, and the model where they additionally have a single-peaked structure. Specifically, we explore how voters with varying amounts of ties and structure in their preferences affect the computational complexity of different election attacks and the complexity of determining whether a given electorate is single-peaked. For the representation of the voters, we consider how representing the voters succinctly affects the complexity of election attacks and discuss how approaches for the nonsuccinct case can be adapted. Control and manipulation are two of the most commonly studied election-attack problems. We introduce a model of electoral control in the setting where some of the voters act strategically (i.e., are manipulators), and consider both the case where the agent controlling the election and the manipulators share a goal, and the case where they have competing goals. The computational study of election-attack problems allows us to better understand how different election systems compare to one another, and it is important to study these problems for natural settings, as this thesis does.
Publisher Site: RIT Repository
PDF (Requires Sign-In): PDF
Click for Bibtex
@book{fitzsimmons2017election-attack, title = {Election-Attack Complexity for More Natural Models}, author = {Fitzsimmons, Zack}, year = {2017}, school = {Rochester Institute of Technology} }
[D-2017-10] Wei Yao. (2017). “Investigating the Impact of Spatially-Explicit Sub-Pixel Structural Variation on the Assessment of Vegetation Structure from Imaging Spectroscopy Data.” Rochester Institute of Technology.
Abstract: Consistent and scalable estimation of vegetation structural parameters from imaging spectroscopy is essential to remote sensing for ecosystem studies, with applications to a wide range of biophysical assessments. NASA has proposed the Hyperspectral Infrared Imager (HyspIRI) imaging spectrometer, which measures the radiance between 380-2500 nm in 10 nm contiguous bands with 60 m ground sample distance (GSD), in support of global vegetation assessment. However, because of the large pixel size on the ground, there is uncertainty as to the effects of sub-pixel vegetation structure on observed radiance. The purpose of this research was to evaluate the link between vegetation structure and imaging spectroscopy spectra. Specifically, the goal was to assess the impact of sub-pixel vegetation density and position, i.e., structural variability, on large-footprint spectral radiances. To achieve this objective, three virtual forest scenes were constructed, corresponding to the actual vegetation structure of the National Ecological Observatory Network (NEON) Pacific Southwest domain (PSW; D17; Fresno, CA). These scenes were used to simulate anticipated HyspIRI data (60 m GSD) using the Digital Imaging and Remote Sensing Image Generation (DIRSIG) model, a physics-driven synthetic image generation model developed by the Rochester Institute of Technology (RIT). Airborne Visible / Infrared Imaging Spectrometer (AVIRIS) and NEON's high-resolution imaging spectrometer (NIS) data were used to verify the geometric parameters and physical models. Multiple simulated HyspIRI data sets were generated by varying within-pixel structural variables, such as forest density, tree position, and distribution of trees, in order to assess the impact of sub-pixel structural variation on the observed HyspIRI data. As part of the effort, a partial least squares (PLS) regression model, along with narrow-band vegetation indices (VIs), were used to characterize the sub-pixel vegetation structure from simulated HyspIRI-like spectroscopy data-like. These simulations were extended to quantitative assessments of within-pixel impact on pixel-level spectral response. The correlation coefficients (R^2) of leaf area index-to-normalized difference vegetation index (LAI-NDVI), canopy cover-to-vegetation index (VI), and PLS models were 0.92, 0.98, and 0.99, respectively. Results of the research have shown that HyspIRI is sensitive to sub-pixel vegetation density variation in the visible to short-wavelength infrared spectrum, due to vegetation structural changes, and associated pigment and water content variation. These findings have implications for improving the system's suitability for consistent global vegetation structural assessments by adapting calibration strategies to account for this sub-pixel variation.
Publisher Site: RIT Repository
PDF (Requires Sign-In): PDF
Click for Bibtex
@book{yao2017investigating, title = {Investigating the Impact of Spatially-Explicit Sub-Pixel Structural Variation on the Assessment of Vegetation Structure from Imaging Spectroscopy Data}, author = {Yao, Wei}, year = {2017}, school = {Rochester Institute of Technology} }
[D-2017-09] McKay D. Williams. (2017). “Generation, Validation, and Application of Abundance Map Reference Data for Spectral Unmixing.” Rochester Institute of Technology.
Abstract: Reference data ("ground truth") maps traditionally have been used to assess the accuracy of imaging spectrometer classification algorithms. However, these reference data can be prohibitively expensive to produce, often do not include sub-pixel abundance estimates necessary to assess spectral unmixing algorithms, and lack published validation reports. Our research proposes methodologies to efficiently generate, validate, and apply abundance map reference data (AMRD) to airborne remote sensing scenes. We generated scene-wide AMRD for three different remote sensing scenes using our remotely sensed reference data (RSRD) technique, which spatially aggregates unmixing results from fine scale imagery (e.g., 1-m Ground Sample Distance (GSD)) to co-located coarse scale imagery (e.g., 10-m GSD or larger). We validated the accuracy of this methodology by estimating AMRD in 51 randomly-selected 10 m x 10 m plots, using seven independent methods and observers, including field surveys by two observers, imagery analysis by two observers, and RSRD using three algorithms. Results indicated statistically-significant differences between all versions of AMRD, suggesting that all forms of reference data need to be validated. Given these significant differences between the independent versions of AMRD, we proposed that the mean of all (MOA) versions of reference data for each plot and class were most likely to represent true abundances. We then compared each version of AMRD to MOA. Best case accuracy was achieved by a version of imagery analysis, which had a mean coverage area error of 2.0%, with a standard deviation of 5.6%. One of the RSRD algorithms was nearly as accurate, achieving a mean error of 3.0%, with a standard deviation of 6.3%, showing the potential of RSRD-based AMRD generation. Application of validated AMRD to specific coarse scale imagery involved three main parts: 1) spatial alignment of coarse and fine scale imagery, 2) aggregation of fine scale abundances to produce coarse scale imagery-specific AMRD, and 3) demonstration of comparisons between coarse scale unmixing abundances and AMRD. Spatial alignment was performed using our scene-wide spectral comparison (SWSC) algorithm, which aligned imagery with accuracy approaching the distance of a single fine scale pixel. We compared simple rectangular aggregation to coarse sensor point spread function (PSF) aggregation, and found that the PSF approach returned lower error, but that rectangular aggregation more accurately estimated true abundances at ground level. We demonstrated various metrics for comparing unmixing results to AMRD, including mean absolute error (MAE) and linear regression (LR). We additionally introduced reference data mean adjusted MAE (MA-MAE), and reference data confidence interval adjusted MAE (CIA-MAE), which account for known error in the reference data itself. MA-MAE analysis indicated that fully constrained linear unmixing of coarse scale imagery across all three scenes returned an error of 10.83% per class and pixel, with regression analysis yielding a slope = 0.85, intercept = 0.04, and R^2 = 0.81. Our reference data research has demonstrated a viable methodology to efficiently generate, validate, and apply AMRD to specific examples of airborne remote sensing imagery, thereby enabling direct quantitative assessment of spectral unmixing performance.
Publisher Site: RIT Repository
PDF (Requires Sign-In): PDF
Click for Bibtex
@book{williams2017generation,, title = {Generation, Validation, and Application of Abundance Map Reference Data for Spectral Unmixing}, author = {Williams, McKay D.}, year = {2017}, school = {Rochester Institute of Technology} }
[D-2017-08] Brittany L. Ambeau. (2017). “Using the Opposition Effect in Remotely Sensed Data to Assist in the Retrieval of Bulk Density.” Rochester Institute of Technology.
Abstract: Bulk density is an important geophysical property that impacts the mobility of military vehicles and personnel. Accurate retrieval of bulk density from remotely sensed data is, therefore, needed to estimate the mobility on "off-road" terrain. For a particulate surface, the functional form of the opposition effect can provide valuable information about composition and structure. In this research, we examine the relationship between bulk density and angular width of the opposition effect for a controlled set of laboratory experiments. Given a sample with a known bulk density, we collect reflectance measurements on a spherical grid for various illumination and view geometries — increasing the amount of reflectance measurements collected at small phase angles near the opposition direction. Bulk densities are varied using a custom-made pluviation device, samples are measured using the Goniometer of the Rochester Institute of Technology-Two (GRIT-T), and observations are fit to the Hapke model using a grid-search method. The method that is selected allows for the direct estimation of five parameters: the single-scattering albedo, the amplitude of the opposition effect, the angular width of the opposition effect, and the two parameters that describe the single-particle phase function. As a test of the Hapke model, the retrieved bulk densities are compared to the known bulk densities. Results show that with an increase in the availability of multi-angular reflectance measurements, the prospects for retrieving the spatial distribution of bulk density from satellite and airborne sensors are imminent.
Publisher Site: RIT Repository
PDF (Requires Sign-In): PDF
Click for Bibtex
@book{ambeau2017using, title = {Using the Opposition Effect in Remotely Sensed Data to Assist in the Retrieval of Bulk Density}, author = {Ambeau, Brittany L.}, year = {2017}, school = {Rochester Institute of Technology} }
[D-2017-07] Fan Wang. (2017). “Understanding High Resolution Aerial Imagery Using Computer Vision Techniques.” Rochester Institute of Technology.
Abstract: Computer vision can make important contributions to the analysis of remote sensing satellite or aerial imagery. However, the resolution of early satellite imagery was not sufficient to provide useful spatial features. The situation is changing with the advent of very-high-spatial-resolution (VHR) imaging sensors. This change makes it possible to use computer vision techniques to perform analysis of man-made structures. Meanwhile, the development of multi-view imaging techniques allows the generation of accurate point clouds as ancillary knowledge. This dissertation aims at developing computer vision and machine learning algorithms for high resolution aerial imagery analysis in the context of application problems including debris detection, building detection and roof condition assessment. High resolution aerial imagery and point clouds were provided by Pictometry International for this study. Debris detection after natural disasters such as tornadoes, hurricanes or tsunamis, is needed for effective debris removal and allocation of limited resources. Significant advances in aerial image acquisition have greatly enabled the possibilities for rapid and automated detection of debris. In this dissertation, a robust debris detection algorithm is proposed. Large scale aerial images are partitioned into homogeneous regions by interactive segmentation. Debris areas are identified based on extracted texture features. Robust building detection is another important part of high resolution aerial imagery understanding. This dissertation develops a 3D scene classification algorithm for building detection using point clouds derived from multi-view imagery. Point clouds are divided into point clusters using Euclidean clustering. Individual point clusters are identified based on extracted spectral and 3D structural features. The inspection of roof condition is an important step in damage claim processing in the insurance industry. Automated roof condition assessment from remotely sensed images is proposed in this dissertation. Initially, texture classification and a bag-of-words model were applied to assess the roof condition using features derived from the whole rooftop. However, considering the complexity of residential rooftop, a more sophisticated method is proposed to divide the task into two stages: 1) roof segmentation, followed by 2) classification of segmented roof regions. Deep learning techniques are investigated for both segmentation and classification. A deep learned feature is proposed and applied in a region merging segmentation algorithm. A fine-tuned deep network is adopted for roof segment classification and found to achieve higher accuracy than traditional methods using hand-crafted features. Contributions of this study include the development of algorithms for debris detection using 2D images and building detection using 3D point clouds. For roof condition assessment, the solutions to this problem are explored in two directions: features derived from the whole rooftop and features extracted from each roof segments. Through our research, roof segmentation followed by segments classification was found to be a more promising method and the workflow processing developed and tested. Deep learning techniques are also investigated for both roof segmentation and segments classification. More unsupervised feature extraction techniques using deep learning can be explored in future work.
Publisher Site: RIT Repository
PDF (Requires Sign-In): PDF
Click for Bibtex
@book{wang2017understanding, title = {Understanding High Resolution Aerial Imagery Using Computer Vision Techniques}, author = {Wang, Fan}, year = {2017}, school = {Rochester Institute of Technology} }
[D-2017-06] Colin Axel. (2017). “Towards Automated Analysis of Urban Infrastructure after Natural Disasters using Remote Sensing.” Rochester Institute of Technology.
Abstract: Natural disasters, such as earthquakes and hurricanes, are an unpreventable component of the complex and changing environment we live in. Continued research and advancement in disaster mitigation through prediction of and preparation for impacts have undoubtedly saved many lives and prevented significant amounts of damage, but it is inevitable that some events will cause destruction and loss of life due to their sheer magnitude and proximity to built-up areas. Consequently, development of effective and efficient disaster response methodologies is a research topic of great interest. A successful emergency response is dependent on a comprehensive understanding of the scenario at hand. It is crucial to assess the state of the infrastructure and transportation network, so that resources can be allocated efficiently. Obstructions to the roadways are one of the biggest inhibitors to effective emergency response. To this end, airborne and satellite remote sensing platforms have been used extensively to collect overhead imagery and other types of data in the event of a natural disaster. The ability of these platforms to rapidly probe large areas is ideal in a situation where a timely response could result in saving lives. Typically, imagery is delivered to emergency management officials who then visually inspect it to determine where roads are obstructed and buildings have collapsed. Manual interpretation of imagery is a slow process and is limited by the quality of the imagery and what the human eye can perceive. In order to overcome the time and resource limitations of manual interpretation, this dissertation inves- tigated the feasibility of performing fully automated post-disaster analysis of roadways and buildings using airborne remote sensing data. First, a novel algorithm for detecting roadway debris piles from airborne light detection and ranging (lidar) point clouds and estimating their volumes is presented. Next, a method for detecting roadway flooding in aerial imagery and estimating the depth of the water using digital elevation models (DEMs) is introduced. Finally, a technique for assessing building damage from airborne lidar point clouds is presented. All three methods are demonstrated using remotely sensed data that were collected in the wake of recent natural disasters. The research presented in this dissertation builds a case for the use of automatic, algorithmic analysis of road networks and buildings after a disaster. By reducing the latency between the disaster and the delivery of damage maps needed to make executive decisions about resource allocation and performing search and rescue missions, significant loss reductions could be achieved.
Publisher Site: RIT Repository
PDF (Requires Sign-In): PDF
Click for Bibtex
@book{axel2017towards, title = {Towards Automated Analysis of Urban Infrastructure after Natural Disasters using Remote Sensing}, author = {Axel, Colin}, year = {2017}, school = {Rochester Institute of Technology} }
[D-2017-05] Yuanhao Zhang. (2017). “Searching for Gravitational Waves from Scorpius X-1 with a Cross-correlation Method: From Mock Data to Advanced LIGO.” Rochester Institute of Technology.
Abstract: Gravitational waves (GWs) are propagating ripples of space-time predicted by general relativity. 100 years after Albert Einstein published his theory of GR, the Laser Interferometer Gravitational-Wave Observatory (LIGO) found the first direct detection of GW in the first Advanced LIGO observing run. The GW signal known as GW150914 (Abbott et al., 2016), was the first of a series of binary black hole mergers observed by LIGO. These detections marked the beginning of gravitational-wave astronomy. The continuous wave (CW) signal emitted by fast spinning neutron stars (NSs) is an another interesting source for a detector like LIGO. The low-mass X-ray binary (LMXB) Scorpius X-1 (Sco X-1) is considered to be one of the most promising CW sources. With improving sensitivity of advanced detectors and improving methods, we are getting closer to being able to detect an astrophysically feasible GW signal from Sco X-1 in the coming few years. Searching for CWs from NSs of unknown phase evolution is computationally intensive. For a target with large uncertainty in its parameters such as Sco X-1, the fully coherent search is computationally impractical, while faster algorithms have limited sensitivity. The cross-correlation method combines all data-pairs in a maximum time offset from same and different detectors coherently based on the signal model. We can adjust the maximum coherence time to trade off computing cost and sensitivity. The cross-correlation method is flexible and so far the most sensitive. In this dissertation I will present the implementation of Cross-correlation method for Sco X-1, its test on a Sco X-1 mock-data challenge (MDC) data set and the Advanced LIGO O1 observations. This search gave the best results in the Sco X-1 mock data challenge and recent LIGO Sco X-1 search. In the O1 run, the Cross-correlation search managed to improve the upper limit on GW strain strength from Sco X-1 closer than ever before to the level estimated from a torque balance argument.
Publisher Site: RIT Repository
PDF (Requires Sign-In): PDF
Click for Bibtex
@book{zhang2017searching, title = {Searching for Gravitational Waves from Scorpius X-1 with a Cross-correlation Method: From Mock Data to Advanced LIGO}, author = {Zhang, Yuanhao}, year = {2017}, school = {Rochester Institute of Technology} }
[D-2017-04] Naseef Mansoor. (2017). “Robust and Traffic Aware Medium Access Control Mechanisms for Energy-Efficient mm-Wave Wireless Network-on-Chip Architectures.” Rochester Institute of Technology.
Abstract: To cater to the performance/watt needs, processors with multiple processing cores on the same chip have become the de-facto design choice. In such multicore systems, Network-on-Chip (NoC) serves as a communication infrastructure for data transfer among the cores on the chip. However, conventional metallic interconnect based NoCs are constrained by their long multi-hop latencies and high power consumption, limiting the performance gain in these systems. Among, different alternatives, due to the CMOS compatibility and energy-efficiency, low-latency wireless interconnect operating in the millimeter wave (mm-wave) band is nearer term solution to this multi-hop communication problem. This has led to the recent exploration of millimeter-wave (mm-wave) wireless technologies in wireless NoC architectures (WiNoC). To realize the mm-wave wireless interconnect in a WiNoC, a wireless interface (WI) equipped with on-chip antenna and transceiver circuit operating at 60GHz frequency range is integrated to the ports of some NoC switches. The WIs are also equipped with a medium access control (MAC) mechanism that ensures a collision free and energy-efficient communication among the WIs located at different parts on the chip. However, due to shrinking feature size and complex integration in CMOS technology, high-density chips like multicore systems are prone to manufacturing defects and dynamic faults during chip operation. Such failures can result in permanently broken wireless links or cause the MAC to malfunction in a WiNoC. Consequently, the energy-efficient communication through the wireless medium will be compromised. Furthermore, the energy efficiency in the wireless channel access is also dependent on the traffic pattern of the applications running on the multicore systems. Due to the bursty and self-similar nature of the NoC traffic patterns, the traffic demand of the WIs can vary both spatially and temporally. Ineffective management of such traffic variation of the WIs, limits the performance and energy benefits of the novel mm-wave interconnect technology. Hence, to utilize the full potential of the novel mm-wave interconnect technology in WiNoCs, design of a simple, fair, robust, and efficient MAC is of paramount importance. The main goal of this dissertation is to propose the design principles for robust and traffic-aware MAC mechanisms to provide high bandwidth, low latency, and energy-efficient data communication in mm-wave WiNoCs. The proposed solution has two parts. In the first part, we propose the cross-layer design methodology of robust WiNoC architecture that can minimize the effect of permanent failure of the wireless links and recover from transient failures caused by single event upsets (SEU). Then, in the second part, we present a traffic-aware MAC mechanism that can adjust the transmission slots of the WIs based on the traffic demand of the WIs. The proposed MAC is also robust against the failure of the wireless access mechanism. Finally, as future research directions, this idea of traffic awareness is extended throughout the whole NoC by enabling adaptiveness in both wired and wireless interconnection fabric.
Publisher Site: RIT Repository
PDF (Requires Sign-In): PDF
Click for Bibtex
@book{mansoor2017robust, title = {Robust and Traffic Aware Medium Access Control Mechanisms for Energy-Efficient mm-Wave Wireless Network-on-Chip Architectures}, author = {Mansoor, Naseef}, year = {2017}, school = {Rochester Institute of Technology} }
[D-2017-03] Timothy Gibbs. (2017). “Physical Property Extraction of Powder Contaminated Surfaces from Longwave Infrared Hyperspectral Imagery.” Rochester Institute of Technology.
Abstract: The detection and characterization of powdered contaminants is a challenging aspect of remote sensing in the longwave infrared spectral region. Powders are small in size (less than 45 microns in diameter) and exhibit weakened spectral features due to increased volume scattering, which is more prevalent as particle size decreases. Meanwhile, atmospheric effects such as wind, clouds, or shadowing cause large fluctuations in temperature of a surface on a microscale. This affects the ability of temperature emissivity separation algorithms to adequately derive a material's spectral emissivity from spectral radiance measurements. Hazardous powdered contaminants that are inaccessible need to be monitored from afar by using instruments of remote sensing. While spectral emissivity signatures alone can be useful, information about the physical properties and phenomenology of the material would be advantageous. Therefore, a method for estimating various physical properties including contaminant mass is presented. The proposed method relies on the principles of the Non-Conventional Exploitation Factors Data System (NEFDS) Contamination Model, which creates spectral reflectance mixtures based on two materials from its own database. Here, a three-step parameter inversion model was utilized that estimates several physical parameters to derive a contaminant mass from three spectral emissivity measurements. This information is then used to inject synthetic target mixtures into real airborne hyperspectral imagery from the Blue Heron LWIR sensor at pixel and sub-pixel levels. Target detection was performed on these images using the adaptive cosine/coherence estimator (ACE) with several types of target spectra. The target spectrum with the largest detection statistic for each target pixel represents the best spectrum to detect its physical properties and informs the detection method. Results indicate that best performance is not always achieved when using the pure contaminant spectrum, but varies with level of contamination and pixel fill fraction. The inversion algorithm method was also applied to real targets in LWIR imagery and demonstrated the ability to extract contaminant mass from the data directly.
Publisher Site: RIT Repository
PDF (Requires Sign-In): PDF
Click for Bibtex
@book{gibbs2017physical, title = {Physical Property Extraction of Powder Contaminated Surfaces from Longwave Infrared Hyperspectral Imagery}, author = {Gibbs, Timothy}, year = {2017}, school = {Rochester Institute of Technology} }
[D-2017-02] Preethi Vaidyanathan. (2017). “Visual-Linguistic Semantic Alignment: Fusing Human Gaze and Spoken Narratives for Image Region Annotation.” Rochester Institute of Technology.
Abstract: Advanced image-based application systems such as image retrieval and visual question answering depend heavily on semantic image region annotation. However, improvements in image region annotation are limited because of our inability to understand how humans, the end users, process these images and image regions. In this work, we expand a framework for capturing image region annotations where interpreting an image is influenced by the end user's visual perception skills, conceptual knowledge, and task-oriented goals. Human image understanding is reflected by individuals' visual and linguistic behaviors, but the meaningful computational integration and interpretation of their multimodal representations (e.g. gaze, text) remain a challenge. Our work explores the hypothesis that eye movements can help us understand experts' perceptual processes and that spoken language descriptions can reveal conceptual elements of image inspection tasks. We propose that there exists a meaningful relation between gaze, spoken narratives, and image content. Using unsupervised bitext alignment, we create meaningful mappings between participants' eye movements (which reveal key areas of images) and spoken descriptions of those images. The resulting alignments are then used to annotate image regions with concept labels. Our alignment accuracy exceeds baseline alignments that are obtained using both simultaneous and a fixed-delay temporal correspondence. Additionally, comparison of alignment accuracy between a method that identifies clusters in the images based on eye movements and a method that identifies clusters using image features shows that the two approaches perform well on different types of images and concept labels. This suggests that an image annotation framework could integrate information from more than one technique to handle heterogeneous images. The resulting alignments can be used to create a database of low-level image features and high-level semantic annotations corresponding to perceptually important image regions. We demonstrate the applicability of the proposed framework with two datasets: one consisting of general-domain images and another with images from the domain of medicine. This work is an important contribution toward the highly challenging problem of fusing human-elicited multimodal data sources, a problem that will become increasingly important as low-resource scenarios become more common.
Publisher Site: RIT Repository
PDF (Requires Sign-In): PDF
Click for Bibtex
@book{vaidyanathan2017visual-linguistic, title = {Visual-Linguistic Semantic Alignment: Fusing Human Gaze and Spoken Narratives for Image Region Annotation}, author = {Vaidyanathan, Preethi}, year = {2017}, school = {Rochester Institute of Technology} }
[D-2017-01] Arvind Jaikumar. (2017). “Multiscale Mechanistic Approach to Enhance Pool Boiling Performance for High Heat Flux Applications.” Rochester Institute of Technology.
Abstract: The advent of cloud computing and the complex packaging architecture of next generation electronic devices drives methods for advanced thermal management solutions. Convection based single-phase cooling systems are inefficient due to their large pressure drops, fluid temperature differences and costs, and are incapable of meeting the cooling requirements in the high power density components and systems. Alternatively, phase-change cooling techniques are attractive due to their ability to remove large amounts of heat while maintaining uniform fluid temperatures. Pool boiling heat transfer mechanism centers on the nucleation, growth and departure of a bubble from the heat transfer surface in a stagnant pool of liquid. The pool boiling performance is quantified by the Critical Heat Flux (CHF) and Heat Transfer Coefficients (HTC) which dictate the operating ranges and efficiency of the heat transfer process. In this work, three novel geometries are introduced to modify the nucleation characteristics, liquid pathways and contact line motion on the prime heater surface for a simultaneous increase in CHF and HTC. First, sintered microchannels and nucleating region with feeder channels (NRFC) were developed through the mechanistic concept of separate liquid-vapor pathways and enhanced macroconvection heat transfer. A maximum CHF of 420 W/cm2 at a wall superheat of 1.7 °C with a HTC of 2900 MW/m2°C was achieved with the sintered-channels configuration, while the NRFC reached a CHF of 394 W/cm2 with a HTC of 713 kW/m2°C. Second, the scale effect of liquid wettability, roughness and microlayer evaporation was exploited to facilitate capillary wicking in graphene through interlaced porous copper particles. A CHF of 220 W/cm2 with a HTC of 155 kW/m2°C was achieved using an electrodeposition coating technique. Third, the chemical heterogeneity on nanoscale coatings was shown to increase the contribution from transient conduction mechanisms. A maximum CHF of 226 W/cm2 with a HTC of 107 kW/m2°C was achieved. The enhancement techniques developed here provide a mechanistic tool at the microscale and nanoscale to increase the boiling CHF and HTC.
Publisher Site: RIT Repository
PDF (Requires Sign-In): PDF
Click for Bibtex
@book{jaikumar2017multiscale, title = {Multiscale Mechanistic Approach to Enhance Pool Boiling Performance for High Heat Flux Applications}, author = {Jaikumar, Arvind}, year = {2017}, school = {Rochester Institute of Technology} }
[D-2016-03] Jeffrey S. Lillie. (2016). “Development, Validation, and Clinical Application of a Numerical Model for Pulse Wave Velocity Propagation in a Cardiovascular System with Application to Noninvasive Blood Pressure Measurements.” Rochester Institute of Technology.
Abstract: High blood pressure blood pressure is an important risk factor for cardiovascular disease and affects almost one-third of the U.S. adult population. Historical cuff-less non-invasive techniques used to monitor blood pressure are not accurate and highlight the need for first principal models. The first model is a one-dimensional model for pulse wave velocity (PWV) propagation in compliant arteries that accounts for nonlinear fluids in a linear elastic thin walled vessel. The results indicate an inverse quadratic relationship (R^2=.99) between ejection time and PWV, with ejection time dominating the PWV shifts (12%). The second model predicts the general relationship between PWV and blood pressure with a rigorous account of nonlinearities in the fluid dynamics, blood vessel elasticity, and finite dynamic deformation of a membrane type thin anisotropic wall. The nonlinear model achieves the best match with the experimental data. To retrieve individual vascular information of a patient, the inverse problem of hemodynamics is presented, calculating local orthotropic hyperelastic properties of the arterial wall. The final model examines the impact of the thick arterial wall with different material properties in the radial direction. For a hypertensive subject the thick wall model provides improved accuracy up to 8.4% in PWV prediction over its thin wall counterpart. This translates to nearly 20% improvement in blood pressure prediction based on a PWV measure. The models highlight flow velocity is additive to the classic pressure wave, suggesting flow velocity correction may be important for cuff-less, non-invasive blood pressure measures. Systolic flow correction of the measured PWV improves the R2 correlation to systolic blood pressure from 0.81 to 0.92 for the mongrel dog study, and 0.34 to 0.88 for the human subjects study. The algorithms and insight resulting from this work can enable the development of an integrated microsystem for cuff-less, non-invasive blood pressure monitoring.
Publisher Site: RIT Repository
PDF (Requires Sign-In): PDF
Click for Bibtex
@book{lillie2016development, title = {Development, Validation, and Clinical Application of a Numerical Model for Pulse Wave Velocity Propagation in a Cardiovascular System with Application to Noninvasive Blood Pressure Measurements}, author = {Lillie, Jeffrey S.}, year = {2016}, publisher = {Rochester Institute of Technology} }
[D-2016-02] Biru Cui. (2016). “From Information Cascade to Knowledge Transfer: Predictive Analyses on Social Networks.” Rochester Institute of Technology.
Abstract: As social media continues to influence our daily life, much research has focused on analyzing characteristics of social networks and tracking how information flows in social media. Information cascade originated from the study of information diffusion which focused on how decision making is affected by others depending on the network structure. An example of such study is the SIR (Susceptible, Infected, Removed) model. The current research on information cascade mainly focuses on three open questions: diffusion model, network inference, and influence maximization. Different from these studies, this dissertation aims at deriving a better understanding to the problem of who will transfer information to whom. Particularly, we want to investigate how knowledge is transferred in social media. The process of transferring knowledge is similar to the information cascade observed in other social networks in the way that both processes transfer particular information from information container to users who do not have the information. The study first works on understanding information cascade in term of detecting information outbreak in Twitter and the factors affecting the cascades. Then we analyze how knowledge is transferred in the sense of adopting research topic among scholars in the DBLP network. However, the knowledge transfer is not able to be well modeled by scholars' publications since a "publication" action is a result of many complicated factors which is not controlled by the knowledge transfer only. So, we turn to Q&A forum, a different type of social media that explicitly contain the process of transferring knowledge, where knowledge transfer is embodied by the question and answering process. This dissertation further investigates Stack-Overflow, a popular Q&A forum, and models how knowledge is transferred among StackOverflow users. The knowledge transfer includes two parts: whether a question will receive answers, and whether an answer will be accepted. By investigating these two problems, it turns out that the knowledge transfer process is affected by the temporal factor and the knowledge level, defined as the combination of the user reputation and posted text. Take these factors into consideration, this work proposes TKTM (Time based Knowledge Transfer Modeling) where the likelihood of a user transfers knowledge to another is modeled as a continuous function of time and the knowledge level being transferred. TKTM is applied to solve several predictive problems: how many user accounts will be involved in the thread to provide answers and comments over time; who will provide the answer; and who will provide the accepted answer. The result is compared to NetRate, QLI, and regression methods such as RandomForest, linear regression. In all experiments, TKTM outperforms other methods significantly.
Publisher Site: RIT Repository
PDF (Requires Sign-In): PDF
Click for Bibtex
@book{cui2016information, title = {From Information Cascade to Knowledge Transfer: Predictive Analyses on Social Networks}, author = {Cui, Biru}, year = {2016}, publisher = {Rochester Institute of Technology} }
[D-2016-01] Ryan M. Bowen. (2016). “Online Novelty Detection System: One-Class Classification of Systemic Operation.” Rochester Institute of Technology.
Abstract: Presented is an Online Novelty Detection System (ONDS) that uses Gaussian Mixture Models (GMMs) and one-class classification techniques to identify novel information from multivariate times-series data. Multiple data preprocessing methods are explored and features vectors formed from frequency components obtained by the Fast Fourier Transform (FFT) and Welch's method of estimating Power Spectral Density (PSD). The number of features are reduced by using bandpower schemes and Principal Component Analysis (PCA). The Expectation Maximization (EM) algorithm is used to learn parameters for GMMs on feature vectors collected from only normal operational conditions. One-class classification is achieved by thresholding likelihood values relative to statistical limits. The ONDS is applied to two different applications from different application domains. The first application uses the ONDS to evaluate systemic health of Radio Frequency (RF) power generators. Four different models of RF power generators and over 400 unique units are tested, and the average robust true positive rate of 94.76% is achieved and the best specificity reported as 86.56%. The second application uses the ONDS to identify novel events from equine motion data and assess equine distress. The ONDS correctly identifies target behaviors as novel events with 97.5% accuracy. Algorithm implementation for both methods is evaluated within embedded systems and demonstrates execution times appropriate for online use.
Publisher Site: RIT Repository
PDF (Requires Sign-In): PDF
Click for Bibtex
@book{bowen2016online, title = {Online Novelty Detection System: One-Class Classification of Systemic Operation}, author = {Bowen, Ryan M.}, year = {2016}, publisher = {Rochester Institute of Technology} }
Master’s Theses
[T-2023-38] Christian Rego. (2023). “The Akastral Voyager.” Rochester Institute of Technology.
Abstract: This project looks to depict a realistic interpretation of a space colony that places a great focus upon pastoralism. This is done in order to provide a more optimistic counterpoint to the prevalence of dystopian pessimistic themes common throughout a majority of science fiction. In essence, rather than being a warning of what the future may hold, it is intended as a possible direction that could be aspired towards. How might we go about portraying a kinder, more optimistic future that we actively aspire towards in the long term rather than seek to avert? Moreover, what sort of design opportunities does technological projection and advancement pose for us as a species? We should envision what an isolated example of a futurist location that showcases technological possibilities could possibly look like, and present it in such a way that it evokes the feeling of wanting to spend time there. The Akastral Voyager presents a look into a future that perhaps isn't quite so bleak as we may often fear. Like its namesake, the Voyager 1 space probe, it seeks to be a symbol of humanity's will to seek out and dwell amongst the stars. We as a species still have countless horizons to seek out and places to find our own dwelling, and the Akastral Voyager represents that quite well. It's a vision of us taking a piece ourselves and our world wherever we go, something that's very innately human.
Publisher Site: RIT Repository
PDF (Requires Sign-In): PDF
Click for Bibtex
@book{rego2023the, title = {The Akastral Voyager}, author = {Rego, Christian}, year = {2023}, school = {Rochester Institute of Technology} }
[T-2023-37] Harrison Barnes. (2023). “A Survey of Qubit Routing Algorithms.” Rochester Institute of Technology.
Abstract: Before applications actually run on quantum hardware, the design of the quantum application and its pre-processing take place in the classical environment. Transforming a quantum circuit into a form that is able to run on quantum hardware, known as transpilation, is a crucial process in performing quantum experiments. Routing is a particularly important and computationally expensive part of the transpilation process. The routing process can alter a circuit's accuracy, execution time, quantum gate count, and depth. Several routing algorithms exist that aim to optimize some portion of the routing process. There are many variables to consider when choosing an optimal routing algorithm, such as routing time, circuit accuracy, gate count, circuit execution time, and more. Identifying what routing algorithm is best for a given quantum experiment based on various properties can allow researchers to make informed routing decisions, as well as optimize target aspects of their work. This thesis draws connections between the properties of circuits, hardware, and routing algorithms to identify when a specific routing algorithm will outperform the others.
Publisher Site: RIT Repository
PDF (Requires Sign-In): PDF
Click for Bibtex
@book{barnes2023a, title = {A Survey of Qubit Routing Algorithms}, author = {Barnes, Harrison}, year = {2023}, school = {Rochester Institute of Technology} }
[T-2023-36] Matthew Klein. (2023). “System Integration of CSTARS: Cryogenic Star Tracking Attitude Regulation System.” Rochester Institute of Technology.
Abstract: The Cryogenic Star Tracking Attitude Regulation System (CSTARS) utilizes a Scientific CMOS (sCMOS) CIS2521F image sensor operating at cryogenic temperatures (77K) to track stars. This is used aboard a sounding rocket for attitude control of the CIBER-2 experiment payload. Standard optical sensors used traditionally for astronomical observations, such as CCD's, have a low responsivity at cryogenic temperatures. In contrast, CMOS sensors do not exhibit this issue. During flight, the CIBER-2 primary imaging sensors and CSTARS are co-boresighted to the same telescope, all of which are cooled down to cryogenic temperatures. The attitude feed- back provided by CSTARS ensures that CIBER-2 remains aligned with the desired starfield. This work has integrated and tested the necessary control and acquisition system and has determined for the first time the responsivity and minimum detectable star magnitude for a sCMOS image sensor operating at cryogenic temperatures. This paper describes the design, verification, and testing methodologies used in the current work, which are also applicable to similar systems and image sensors. CSTARS was flown along with CIBER-2 on April 16th 2023. The results leading up to and during this flight have proven the readiness of sCMOS sensors for cryogenic space flight operation.
Publisher Site: RIT Repository
PDF (Requires Sign-In): PDF
Click for Bibtex
@book{klein2023system, title = {System Integration of CSTARS: Cryogenic Star Tracking Attitude Regulation System}, author = {Klein, Matthew}, year = {2023}, school = {Rochester Institute of Technology} }
[T-2023-35] Anusha Holavanahali. (2023). “An Analysis of Heterogeneous Quantization Schemes for Neural Networks.” Rochester Institute of Technology.
Abstract: Quantization of neural network models is becoming a necessary step in deploying artificial intelligence (AI) at the edge. The quantization process reduces the precision of model parameters, thereby lowering memory and computational costs. However, in doing so, this process also limits the model's representational capacity, which can alter both its performance on nominal inputs (clean accuracy) as well as its robustness to adversarial attacks (adversarial accuracy). Few researchers have explored these two metrics simultaneously in the context of quantized neural networks, leaving several open questions about the security and trustworthiness of AI algorithms implemented on edge devices. This research explores the effects of different weight quantization schemes on both clean and adversarial accuracies of neural network models subjected to memory constraints. Two models—VGG-16 and a 3-layer multilayer perceptron (MLP)—were studied with the MNIST and CIFAR-10 image classification datasets. The weights of the models were quantized during training using the deterministic rounding technique. The models were either quantized homogeneously, with all weights quantized to the same precision, or heterogeneously, with weights quantized to different precisions. Several different bitwidths were used for homogeneous quantization, while several different probability mass function-based distributions of bitwidths were used for heterogeneous quantization. To the best of the author's knowledge, this is the first work to study adversarial robustness under homogeneous quantization based on different probability mass functions. Results show that clean accuracy generally increases when quantized homogeneously at higher bitwidths. For the heterogeneously quantized VGG-16, the distributions that contain a higher quantity of low bitwidth weights have worse performance than those that did not. The heterogeneously quantized MLP performance, however, is generally consistent across distributions. Both models perform far better on the MNIST dataset than the CIFAR-10. For the MNIST dataset, the VGG-16 model displayed higher levels of adversarial robustness when the quantization of the model contained a greater quantity of lower bitwidth weights. However, the adversarial robustness of the MLP decreases with larger attack strength for all bitwidths. Neither model shows convincing levels of adversarial robustness on the CIFAR-10 dataset. Overall, the results of this research show that both clean and adversarial accuracies have complex dependencies on the total capacity of weight memory and the distribution of precisions among individual weights.
Publisher Site: RIT Repository
PDF (Requires Sign-In): PDF
Click for Bibtex
@book{holavanahali2023an, title = {An Analysis of Heterogeneous Quantization Schemes for Neural Networks}, author = {Holavanahali, Anusha}, year = {2023}, school = {Rochester Institute of Technology} }
[T-2023-34] Le Nguyen. (2023). “A Graph-Based Approach to Studying the Spread of Radical Online Sentiment.” Rochester Institute of Technology.
Abstract: The spread of radicalization and extremism through the Internet is a growing problem. We are witnessing a rise in online hate groups, inspiring the impressionable and vulnerable population towards extreme actions in the real world. Though the body of research to address these issues is growing in kind, they lack a key understanding of the structure and behavior of online extremist com- munities. In this thesis, we study the structure and behavior of extremist online communities and the spread of hateful sentiments through them to address this gap in the research. We propose a novel Graph-Based Approach to Studying the Spread of Radical Online Sentiment for studying the dynamics of online com- ment threads by representing them as graphs. Our Graph-Based Approach to Studying the Spread of Radical Online Sentiment allows us to leverage network analysis tools to reveal the most influential members in a social network and investigate sentiment propagation through the network as well. By combining sentiment analysis, social network analysis, and graph theory, we aim to shed light on the propagation of hate speech in online forums and the extent to which such speech can influence individuals. In this thesis, we pose four main research questions; firstly, to what ex- tent do connected members in an online comment thread and connected threads themselves share sentiment? Further, what is the impact of the frequency of interaction, measured by the degree of connection, on the sharing of sentiment?. Secondly, who are the most influential members in a comment thread, and how do they shape the sentiment in that thread? Thirdly, what does the sentiment of the thread look like over time as more members join threads and more comments are made? Finally, can the behavior of online sentiment spread be generalized? Can we develop a model for it? To answer these questions, we apply our Graph- Based Approach to Studying the Spread of Radical Online Sentiment to 1,973 long comment threads (30+ comments), totaling to 137k comments posted on dark-web forums. These threads contain a combination of benign posts and extremist comments on the Islamic religion from an unmoderated source. To answer our first research question, we constructed intra- and inter-thread graphs where we could analyze weighted and unweighted connections between threads and members within threads. Our results show that 73% of connected members within a comment thread shares a similar sentiment, and 64% of connected com- ment threads share a similar sentiment on the inter-thread level when weighted by the degree of connection. Additionally, we found the most influential mem- bers of our graphs using information centrality. We found that the original poster was the most influential member in our comment threads 57% of the time, with the mean sentiment of the thread matching the sentiment of the original poster. For our third research question, we performed a temporal anal- ysis of our threads. This analysis further supported our findings in our second research question. Over time, the majority of our threads had their overall sen- timent regress to the sentiment of the original poster, with the original poster being the member with the highest influence for 40% of the time steps. For our fourth and final research question, we used our understanding of our comment threads to create a model that can classify the sentiment of a thread member based on the members they are connected with. We achieved 87% accuracy with our classification model and further used it as a sentiment contagion model, which predicted the sentiment of a new member to a thread based on existing members with 72% accuracy. We plan to expand our study and further the robustness of our models on larger data sets and incorporate stance detection tools.
Publisher Site: RIT Repository
PDF (Requires Sign-In): PDF
Click for Bibtex
@book{nguyen2023a, title = {A Graph-Based Approach to Studying the Spread of Radical Online Sentiment}, author = {Nguyen, Le}, year = {2023}, school = {Rochester Institute of Technology} }
[T-2023-33] Ajay Shewale. (2023). “Investigating the Impact of Baselines on Integrated Gradients for Explainable AI.” Rochester Institute of Technology.
Abstract: Deep Neural Networks have rapidly developed over the last few years, demonstrating state-of-the-art performances on various machine learning tasks such as image classification, natural language processing, and speech recognition. Despite their remarkable performance, deep neural networks are often criticized for their need for more interpretability, which makes it difficult to comprehend their decision-making process and get insights into their workings. Explainable AI has emerged as an important area of study that aims to overcome this issue by providing understandable explanations for deep neural network predictions. In this thesis, we focus on one of the explainability methods called Integrated Gradients (IG) and propose a contour-based analysis method for assessing the faithfulness of the IG algorithm. Our experiments on the IG algorithm showcase that it is an effective technique for generating attributions for deep neural networks. We found that the IG algorithm effectively generated attributions consistent with human intuition, highlighting relevant regions of the input images. However, there are still significant issues with the performance and interpretability of IG. For example, choosing the correct baselines for computing IG attributions is still important. The baseline in this context refers to the lack of features, which is used as a starting point to get the attributions. To address this issue, we assessed the performance of the IG algorithm by using multiple random baselines and aggregating the resulting attributions using mean and median techniques to obtain the final attribution. To evaluate the aggregated attributions, we propose a contour-based analysis method. This method provides an important continuous patch of aggregated IG attribution's top 10\% values. The continuous patch of important features allows us a more intuitive interpretation of IG's performance. We use the Captum library to implement the IG algorithm and experiment with multiple random baselines to compare the attributions generated by the IG algorithm. Our results demonstrate that the contour-based analysis method can be used to evaluate the performance of the IG algorithm for different baselines and can be applied to other attribution algorithms as well. Our findings suggest that the IG algorithm can identify the most critical elements of an image, and the contour-based approach can extract more localized and detailed information. Our research sheds light on the effectiveness of the multiple random baselines on the Integrated Gradients (IG) algorithm. It provides valuable insights into its performance when generating attributions for deep neural networks with different baselines. We also identify several limitations of our study, such as focusing on a single model architecture and data type and using a perturbation-based method to create random baselines. Future work can address these limitations by evaluating the performance of IG on other types of models and data using different ways to create the baselines.
Publisher Site: RIT Repository
PDF (Requires Sign-In): PDF
Click for Bibtex
@book{shewale2023investigating, title = {Investigating the Impact of Baselines on Integrated Gradients for Explainable AI}, author = {Shewale, Ajay}, year = {2023}, school = {Rochester Institute of Technology} }
[T-2023-32] Andrew Thompson. (2023). “An Examination of the Effects of the Cost Volume on Vision Transformer-Based Optical Flow Models.” Rochester Institute of Technology.
Abstract: The optical flow task is an active research domain in the machine learning community with numerous downstream applications such as autonomous driving and action recognition. Convolutional neural networks have often been the basis of design for these models. However, given the recent popularity of self-attention architectures, many optical flow models now utilize a vision transformer backbone. Despite the differing backbone architectures used, consistencies in model design, especially regarding auxiliary operations, have been observed. Perhaps most apparent is the calculation and use of the cost volume. While prior works have well documented the effects of the cost volume on models with a convolutional neural network backbone, similar research does not exist for optical flow models based on other architectures, such as the vision transformer. Naturally, a research question arises: what are the effects of utilizing a cost volume in vision transformer-based optical flow models? In this thesis, a series of experiments examine the impact of the cost volume on training time, model accuracy, average inference time, and model size. The observed results show that cost volume use increases the model size and training time while improving model accuracy and reducing average inference time. These results differ from those regarding the effects of the cost volume on convolutional neural network-based models. With the results presented, researchers can now adequately consider the potential benefits and drawbacks of cost volume use in vision transformer-based optical flow models.
Publisher Site: RIT Repository
PDF (Requires Sign-In): PDF
Click for Bibtex
@book{thompson2023an, title = {An Examination of the Effects of the Cost Volume on Vision Transformer-Based Optical Flow Models}, author = {Thompson, Andrew}, year = {2023}, school = {Rochester Institute of Technology} }
[T-2023-31] Martin A. Hoffnagle. (2023). “Quantum Acceleration of Linear Regression for Artificial Neural Networks.” Rochester Institute of Technology.
Abstract: Through proofs and small scale implementations, quantum computing has shown potential to provide significant speedups in certain applications such as searches and matrix calculations. Recent library developments have introduced the concept of hybrid quantum-classical compute models where quantum processor units could be used as additional hardware accelerators by classical computers. While these developments have opened the prospect of applying quantum computing to machine learning tasks, there are still many limitations of near and midterm quantum computing. If implemented carefully, the advantages of quantum algorithms could be used to accelerate current machine learning models. In this work, a hybrid quantum-classical model is designed to solve a gradient descent problem. The quantum HHL algorithm is used to solve a system of linear equations. The quantum swap test circuit is then used to extract the Euclidean distance between a test point and the quantum solution. The Euclidean distance is then passed to a classical gradient descent algorithm to reduce the number of iterations required by the gradient descent algorithm to converge on a solution.
Publisher Site: RIT Repository
PDF (Requires Sign-In): PDF
Click for Bibtex
@book{hoffnagle2023quantum, title = {Quantum Acceleration of Linear Regression for Artificial Neural Networks}, author = {Hoffnagle, Martin A.}, year = {2023}, school = {Rochester Institute of Technology} }
[T-2023-30] Dhana Lavanya Balasubramanian. (2023). “A Bit-Exact Hierarchical SystemC Model of pPIM for Verification and Performance Modeling.” Rochester Institute of Technology.
Abstract: The semiconductor industry has seen tremendous advancement lately and is expected to continue to grow since multiple industries like automobile, health care, meteorology, etc are developing dedicated chips with advancements in Artificial Intelligence (AI). These improvements also come with increased complexities. Engineers now require advanced ways to model the designs in addition to existing Hardware Description Languages (HDLs) such as Very High-Speed Integrated Circuit Hardware Description Language (VHDL) and Verilog. One such way to model the designs is by using SystemC, which is a library of C++ classes and macros that can be used similar to an HDL for modeling hardware for functional verification and performance modeling of the design. This paper discusses a bit-exact hierarchical SystemC model written for a multi-core programmable Processor-In-Memory (pPIM) and explores the advantages it has over existing HDLs such as Verilog, SystemVerilog, or VHDL. The pPIM is a Look Up Table (LUT) based Processing In Memory (PIM) architecture that can perform parallel processing with ultra-lowlatency for implementing data-intensive applications like Deep Neural Networks (DNN) and Convolution Neural Networks (CNN).
Publisher Site: RIT Repository
PDF (Requires Sign-In): PDF
Click for Bibtex
@book{balasubramanian2023a, title = {A Bit-Exact Hierarchical SystemC Model of pPIM for Verification and Performance Modeling}, author = {Balasubramanian, Dhana Lavanya}, year = {2023}, school = {Rochester Institute of Technology} }
[T-2023-29] Andy Belle-Isle. (2023). “Memory Protection with Cached Authentication Trees.” Rochester Institute of Technology.
Abstract: The use of embedded systems and the amount of data they process is rapidly growing in the modern information age. Given physical access to a device, an attacker can monitor the signals between the CPU and Memory to intercept, and possibly even inject new data into the system. A variety of attacks are possible including, replay, spoofing, and splicing attacks, each one threatening the safety of the system. Ensuring this data is intact is imperative, and as physical protection is difficult, data protection hardware is a must. Protecting memory was researched in the past, and there are several methods of achieving it, with techniques such as memory encryption, memory hashes, and message authentication codes. While these achieve the desired effect, they do it at the cost of performance, memory usage, and additional hardware. To overcome these concerns, authentication tree designs have been proposed to protect memory with reduced overhead. For example, static tree designs such as TEC-Trees have been proven effective in the past, but have limited performance in certain access patterns (workloads). Most recently proposed dynamically balanced trees provide an additional solution with improved performance in certain workloads, however; with its own additional limitations. This research built on the top of the dynamic tree design by integrating tree node caches and evaluating the improved viability of the dynamic authentication tree (DAT) approach. The design was implemented on a Xilinx Zynq-7000 SoC that used a hard processing system core to communicate with the fabric-based memory protection controller. The addition of caches to the dynamic authentication tree design increased the performance enough to perform similarly to TEC-Trees. As expected, in certain memory access patterns, such as those that repeatedly accessed a group of common memory locations, the cache-added DAT was able to outperform both the original design, and TEC-Tree based designs.
Publisher Site: RIT Repository
PDF (Requires Sign-In): PDF
Click for Bibtex
@book{belle-isle2023memory, title = {Memory Protection with Cached Authentication Trees}, author = {Belle-Isle, Andy}, year = {2023}, school = {Rochester Institute of Technology} }
[T-2023-28] Brody A. Wrighter. (2023). “Improved Grover’s Implementation of Quantum Binary Neural Networks.” Rochester Institute of Technology.
Abstract: Binary Neural Networks (BNNs) are the result of a simplification of network parameters in Artificial Neural Networks (ANNs). The computational complexity of training ANNs increases significantly as the size of the network increases. This complexity can be greatly reduced if the parameters of the network are binarized. Binarization, which is a one bit quantization, can also come with complications including quantization error and information loss. The implementation of BNNs on quantum hardware could potentially provide a computational advantage over its classical counterpart. This is due to the fact that binarized parameters fit nicely to the nature of quantum hardware. Quantum superposition allows the network to be trained more efficiently, without using back propagation techniques, with the application of Grover's Algorithm for the training process. This thesis presents two BNN designs that utilize only quantum hardware, and provides practical implementations for both of them. Looking into their scalability, improvements on the design are proposed to reduce complexity even further.
Publisher Site: RIT Repository
PDF (Requires Sign-In): PDF
Click for Bibtex
@book{wrighter2023improved, title = {Improved Grover's Implementation of Quantum Binary Neural Networks}, author = {Wrighter, Brody A.}, year = {2023}, school = {Rochester Institute of Technology} }
[T-2023-27] Long H. Nguyen. (2023). “Optical Phased Array for lidar systems at blue wavelengths.” Rochester Institute of Technology.
Abstract: Optical Phased Array has become a popular solution for light detection and rang- ing (lidar) due to its compact size, steering ability without physical moving parts, and integration with conventional CMOS manufacturing processes. With the rising demand in advanced driver assistance systems (ADAS) and surveying in agriculture and oceanography industries, there is a need for a durable, high performing, and integrable lidar module. For that, a suitable integrated OPA is required to carry sufficient power and have large steering angle at a particular wavelength range. This thesis is specifically focused on blue wavelengths for use in applications such as aug- mented/virtual reality, displays, oceanography, atmospheric sensing, and quantum information systems based on atoms/ions. The steering angle of a OPA depends heavily on the distribution of emitters. Ide- ally, the OPA can steer 180 degrees when the spacing of the array elements are exactly a half wavelength apart. However, at this spacing, fabrication and crosstalk between waveguides become a major issue. Consequently, the emitters must be spaced farther apart, reducing the steering angle due to the creation of side-lobes. To address this challenge, in this work, we present and demonstrate OPAs that utilize non-periodic element spacings. Specifically, we investigated emitters that are distributed using a Golomb ruler or with random distributions. We were able to achieve a large steering angle at a blue wavelength (450nm) without any emitters at a half-wavelength spac- ing. We also demonstrate that by varying the widths of the waveguides, crosstalk can be significantly reduced. These methods could be applied to other wavelengths to increase the OPA performance and robustness.
Publisher Site: RIT Repository
PDF (Requires Sign-In): PDF
Click for Bibtex
@book{nguyen2023optical, title = {Optical Phased Array for lidar systems at blue wavelengths}, author = {Nguyen, Long H.}, year = {2023}, school = {Rochester Institute of Technology} }
[T-2023-26] Benjamin Beaudry. (2023). “Numerical and Experimental Investigation and Optimization of Particle Size Distribution in a Medical Jet Nebulizer Using A Multiphase Multiscale Approach.” Rochester Institute of Technology.
Abstract: Jet nebulizers are a common form of nebulizer used for aerosol treatment. They are known to function poorly in children under five due to differences in patient attributes. They must reduce their mass median aerodynamic diameter (MMAD) to work more effectively. This allows more medicine to be deposited in the lungs and absorbed. Solving this problem can affect 9 million annual deaths worldwide due to repository disease. While lowering the billions of dollars, the U.S. spends on these deaths. This study focused on the standard Hudson Micro Mist Nebulizer and investigated spray physics using experimental and simulation methods to enhance its design. The present investigation aims to fill a research gap on jet nebulizers. Unlike previous studies, this analysis divides the primary atomization into one simulation and the secondary atomization into a separate simulation. This methodology accelerates simulation speed from months or years to a single week and facilitates parametric optimization. Moreover, it provides more insights into the primary atomization region and particle transport, which no experimental technique can obtain simultaneously. The numerical results optimize multiple metrics, including MMAD, geometric standard deviation (GSD), and air-to-liquid ratio (ALR). The present study introduces modifications to the conventional Hudson Micro Mist nebulizer by manipulating the inlet pressure (10, 20, 30 psi), the baffle geometry (spherical, baffle), and the nozzle orifice area ratio (0.59, 0.70, 1.10). The original design uses a pulsating 12 psi inlet pressure, spherical baffle, and the 0.59 orifice area ratio. The investigation identifies superior designs and elucidates the underlying mechanisms that enhance spray distribution. The numerical simulations exhibited a trend-following behavior and generally agreed with the experimental outcomes, providing valuable insights into the design factors that affect the critical metrics of MMAD, ALR, and GSD. The results indicated that the orifice area ratio had little effect on these metrics, while the inlet pressure and baffle geometry had significant impacts, corroborated by experiments. Both the experiments and simulations suggested that the 30-psi bar baffle design was optimal for achieving the desired MMAD and GSD values, reducing the MMAD by 70% in experiments and 66% in simulation. However, it was found to be suboptimal for ALR. Therefore the 30-psi bar baffle design is suggested for use.
Publisher Site: RIT Repository
PDF (Requires Sign-In): PDF
Click for Bibtex
@book{beaudry2023numerical, title = {Numerical and Experimental Investigation and Optimization of Particle Size Distribution in a Medical Jet Nebulizer Using A Multiphase Multiscale Approach}, author = {Beaudry, Benjamin}, year = {2023}, school = {Rochester Institute of Technology} }
[T-2023-25] Alexander Dacey. (2023). “Passive Reconfigurable Dual Linear and Dual Circular Polarization with CLRH-TL for Microstrip Patch Antennas.” Rochester Institute of Technology.
Abstract: Passively controlled reconfigurable antennas are desirable for their relatively inexpensive cost compared to the intensive implementation of RF integrated circuits. Extensive research continues to go into structures utilizing frequency selective metamaterials and their applications in reconfigurable antennas in order to find alternatives to active components. Composite left/right-handed transmission lines (CLRH-TLs), consisting of series capacitors and shunt inductors, are one such structure that is capable of operating as a left-handed transmission line at select frequencies. In this thesis, we propose and investigate a unique frequency selective open circuit utilizing CLRH-TLs that operates as an electrical open and a transmission line at two different frequencies, as well as a quadrature hybrid that is configured with these structures to create the required power and phase distribution for dual linear and dual circular polarizations at four different frequencies. The frequency selective open circuit is verified through simulation and validated through prototyping. The modified quadrature hybrid is integrated in the feed system of an dual linear polarized aperture coupled square microstrip patch antenna that is set in a stacked arrangement to increase the bandwidth to 17% (10.7-12.7GHz). Equivalent circuits to the modified quadrature hybrid demonstrate the four polarizations at four frequencies.
Publisher Site: RIT Repository
PDF (Requires Sign-In): PDF
Click for Bibtex
@book{dacey2023passive, title = {Passive Reconfigurable Dual Linear and Dual Circular Polarization with CLRH-TL for Microstrip Patch Antennas}, author = {Dacey, Alexander}, year = {2023}, school = {Rochester Institute of Technology} }
[T-2023-24] Gavin Burris. (2023). “Efficiently Annotating Source Code Identifiers Using a Scalable Part of Speech Tagger.” Rochester Institute of Technology.
Abstract: This thesis details the process in which a part-of-speech tagger is developed in order to determine grammar patterns in source code identifiers. These grammar patterns are used to aid in the proper naming of identifiers in order to improve reader comprehension. This tagger is a continuation of an effort of a previous Ensemble Tagger [62], but with a focus on increasing the tagging rate while maintaining the accuracy, in order to make the tagger scalable. The Scalable Tagger will be trained on open source data sets, with a machine learning model and training features that are chosen to best suit the needs for accuracy and tagging rate. The results of the experiment will be contrasted with the results of the Ensemble Tagger to determine the Scalable Tagger's efficacy.
Publisher Site: RIT Repository
PDF (Requires Sign-In): PDF
Click for Bibtex
@book{burris2023efficiently, title = {Efficiently Annotating Source Code Identifiers Using a Scalable Part of Speech Tagger}, author = {Burris, Gavin}, year = {2023}, school = {Rochester Institute of Technology} }
[T-2023-23] Georgi Thomas. (2023). “Continual Domain Adaptation through Knowledge Distillation.” Rochester Institute of Technology.
Abstract: Domain Adaptation (DA) techniques aim to overcome the domain shift between a source domain used for training and the target domain used for testing and deployment. Domain adaptation methods assume the entire target domain is accessible during the adaptation process. We use efficient architectures in the continual data-constrained DA paradigm where the unlabeled data in the target domain is received continually in batches. In recent years, Vision Transformers have emerged as an alternative to traditional Convolutional Neural Networks (CNNs) as the feature extraction backbone for image classification and other computer vision tasks. Within the field of DA, these attention-based architectures have proven to be more powerful than their traditional counterparts. However, they possess a larger computational overhead due to their model size. We design a novel framework, called Continual Domain Adaptation through Knowledge Distillation (CAKE), that uses knowledge distillation (KD) to transfer to a CNN the more complex Vision Transformer's knowledge. By doing so, CNN-based adaptation obtains a similar performance to transformer-based adaptation while reducing the computational overhead. With this framework, we selectively choose samples from these batches to store in a buffer for selective replay. We mix the samples from the buffer with the incoming samples to incrementally update and adapt our model. We show that distilling to a smaller network after adapting a larger model allows the smaller network to achieve better accuracy than if the smaller network adapted to the target domain. We also demonstrate that CAKE outperforms state-of-the-art unsupervised domain adaptation methods without full access to the target domain or any access to the source domain.
Publisher Site: RIT Repository
PDF (Requires Sign-In): PDF
Click for Bibtex
@book{thomas2023continual, title = {Continual Domain Adaptation through Knowledge Distillation}, author = {Thomas, Georgi}, year = {2023}, school = {Rochester Institute of Technology} }
[T-2023-22] Matthew Krebs. (2023). “FSMLock: Sequential Logic Locking through Encryption.” Rochester Institute of Technology.
Abstract: As the technology node size for integrated circuit (IC) designs decreases, the cost of building and maintaining an IC foundry rapidly increases. Companies unable to afford local manufacturing have become reliant on outsourcing the physical manufacturing process. This introduces confidentiality, integrity, and authenticity security vulnerabilities into the IC design lifecycle. Even companies that manufacture in-house and use field-programmable gate array (FPGA) chips may require third-party system integrators to assemble the final product. When said product is sent to a third-party foundry or system integrator, the embodied IC/FPGA circuitry is susceptible to IP theft, Trojan insertion, and reverse engineering (RE) attacks. To address this, we realize a novel approach to sequential logic locking, FSMLock, that conceals a finite state machine's (FSM) output and next-state logic through classical encryption. The FSM is abstracted as the configuration data for a lookup table (LUT), encrypted with a chip-specific (individual) internal key, and stored in the newly mandated non-volatile memory (NVM). The configuration data is then decrypted in blocks and loaded into the in-scope random access memory (RAM) when required. Doing so locks the sequential FSM logic and conceals its functionality from third-party foundries and system integrators, system design engineers with access to the post-locked hardware description language (HDL) files, and end-users with production units. FSMLock has applications in reconfigurable hardware, such as FPGAs, even when no third-party access is initially required. In older and low-cost FPGA devices with externally stored bitstream configurations, the absence of trusted bitstream encryption/authentication means that if the bitstream is recovered from the external memory device, an adversary can reconstruct and modify the original design functionality. FSMLock can improve the security of such FPGA chips by storing targeted FSM logic in encrypted NVM. Therefore, a breach of the bitstream contents and the NVM's individual internal key would be required to compromise the security of the targeted sequential circuitry. Further, if a key preprocessor utilizing a physically unclonable function (PUF) is included to discriminate the boundary level (chip) key from the internal key, the confidentiality of the locked circuit is assured, even considering the disclosure of a chip key with its paired encrypted NVM configuration. For the scope of this thesis, we sought to develop an automated software toolset capable of translating pre-partitioned FSMs into encrypted memory configurations. When the configuration is combined with the provided HDL entity responsible for run-time decryption and scope control, a locked HDL model of the FSM, i.e., the FSMLock primitive, is formed.
Publisher Site: RIT Repository
PDF (Requires Sign-In): PDF
Click for Bibtex
@book{krebs2023fsmlock:, title = {FSMLock: Sequential Logic Locking through Encryption}, author = {Krebs, Matthew}, year = {2023}, school = {Rochester Institute of Technology} }
[T-2023-21] Cheyenne Dailey. (2023). “Applying Homomorphic Encryption to a Cross Domain Problem.” Rochester Institute of Technology.
Abstract: The Cross Domain Problem (CDP) strives to ensure protected data transference across varying security domains. In order to accomplish this, a Cross Domain Solution (CDS) is needed. A common method to protect data is to focus on risk management between trusted parties; however, untrusted parties pose ongoing concern. The problem is determining a method that transfers classified data through various security domains without exposing any information to intermediary parties. Attempts to mitigate this problem have been made utilizing Homomorphic Encryption (HE), a type of encryption that allows for computations to be executed on encrypted data without needing to decrypt it. Research studies have demonstrated the feasibility of applying an HE scheme paired with a cipher to successfully create a CDS for untrusted parties. By researching recent enhancements in the fields of homomorphic encryption, lightweight ciphers, and hybrid homomorphic ciphers a pair was found with the hope of practical main steam use has been achieved. The homomorphic scheme, BFV, has been around for many years with thorough testing and new optimizations applied. The cipher, Pasta, is a hybrid homomorphic cipher specifically catered to the application of homomorphic decryption. Together, a software test case was created that would mimic the required behavior needed to create a CDS. The final implementation offered testing of homomorphic decryption with both 3-Round and 4- Round Pasta with acceptable speeds given the processing power available. Along with the rounds changing, size of key, plaintext, and multiplicative depth influenced overall performance. Verifying the usability post decryption, comparison of values at any index demonstrated the ability to search and compare specific plaintext or metadata values for viable information about transmission through encountered gateways. In both variations, the speed was favorable, proving to be at least 5 times faster than similar implementations.
Publisher Site: RIT Repository
PDF (Requires Sign-In): PDF
Click for Bibtex
@book{dailey2023applying, title = {Applying Homomorphic Encryption to a Cross Domain Problem}, author = {Dailey, Cheyenne}, year = {2023}, school = {Rochester Institute of Technology} }
[T-2023-20] Yuval H. Levental. (2023). “LIDAR Voxel Segmentation Using 3D Convolutional Neural Networks.” Rochester Institute of Technology.
Abstract: Light detection and ranging (lidar) forest models are important for studying forest composition in great detail, and for tracking objects in the understory. In this study we used DIRSIG, a first-principles and physics-based simulation tool, to turn the lidar data into voxels, towards classifying forest voxel types. A voxel is a 3D cube where the dimension represents a certain distance. These voxels are split into categories consisting of background, leaf, bark, ground, and object elements. Voxel content is then predicted from the provided simulated and real National Ecological Observation Network (NEON) data. The inputs are 3D neighborhood cubes which surround each voxel, which contain surrounding lidar signal and content type information. Provided simulated data are from two sources: a VLP-16 drone, which collects discrete lidar data close to the canopy, and the NEON Airborne Observation Platform (AOP), which is attached to an airplane flying 1000 m above ground level and collects both discrete and waveform lidar data. Different machine learning algorithms were implemented, with 3D CNN algorithms shown to be the most effective. The Keras library was used, since creating the layers with the sequential model was regarded as an elegant approach. The simulated VLP-16 waveform data were significantly more accurate than the simulated NEON waveform data, which was attributed to its proximity to the canopy. Leaves and branches exhibited acceptable accuracies, due to their relatively random shapes. However, ground and objects in both cases had very high accuracy due to the high intensities and their rigid shapes, respectively. A sample of real NEON waveform lidar data was used, though the sample primarily focused on the canopy region; however, most of the voxels were correctly predicted as leaves. Additional channels were added to the input voxels in order to improve accuracy. One input parameter which proved to be very useful were the local z-values of each input array. Additionally, the Keras Tuner framework was used to obtain improved hyperparameters. The learning rate was reduced by a factor of 10, which provided slower, but steadier convergence towards accurate predictions. The resulting accuracies from the predictions are promising, but there is room for improvement. Different ML algorithms that use the point cloud should also be considered. Further segmentation of forest classes is another possibility. For example, there are different types of trees and bushes, so each tree or bush could have its own unique classes, which would make predicting the shapes much easier. Overall, discovering a method for accurate object prediction has been the most significant finding. For the ground truth models, the best object precision is approximately 99% and the best recall is 78%.
Publisher Site: RIT Repository
PDF (Requires Sign-In): PDF
Click for Bibtex
@book{levental2023lidar, title = {LIDAR Voxel Segmentation Using 3D Convolutional Neural Networks}, author = {Levental, Yuval H.}, year = {2023}, school = {Rochester Institute of Technology} }
[T-2023-19] Michael W. Anderson. (2023). “Design of an Accelerated Lifetime Tester for Industrial Gas Compressor Poppet Valves.” Rochester Institute of Technology.
Abstract: There is increasing interest within the petrochemical industry in the development of predictive maintenance algorithms for gas compressors and pipelines. To this end, this thesis outlines an analysis of the feasibility and design of a lifetime accelerator for poppet valves used in industrial gas compressors. First, we investigate the most common compressor and valve types. We then compare existing valve accelerators to determine the gap in current research on poppet valve lifetime accelerators. We demonstrate our design for a lifetime accelerator with the ability to actuate poppet valves at 100[Hz], which is more than 15 times higher than the normal operating rate of 6.4[Hz]. Based on preliminary calculations, the system will not operate near the resonant frequency, and the system will be able to safely hold 100[psig]. Further, an orifice-flow system model indicates the design will be able to transfer air through the system quickly enough to support desired valve actuation rate. Finally, our constructed system demonstrates that the system will be able to achieve and maintain pressures of up to 100[psig] safely and with the possibility to function beyond the initially designed parameters.
Publisher Site: RIT Repository
PDF (Requires Sign-In): PDF
Click for Bibtex
@book{anderson2023design, title = {Design of an Accelerated Lifetime Tester for Industrial Gas Compressor Poppet Valves}, author = {Anderson, Michael W.}, year = {2023}, school = {Rochester Institute of Technology} }
[T-2023-18] Shery George Matthews. (2023). “Design of a Current Mode Circuit for Zeroth-Order Optimization of Memristor-Based Neuromorphic Systems.” Rochester Institute of Technology.
Abstract: Artificial neural networks (ANNs) have become widely used over the past decade due to their excellent performance in applications such as object recognition and classification, time series forecasting, machine translation, and more. Key factors contributing to the success of ANNs in these domains include the availability of large datasets and constant improvements in powerful parallel processing architectures such as graphics processing units (GPUs). However, modern ANN models' growing size and complexity preclude their deployment on resource-constrained hardware for edge applications. Neuromorphic computing seeks to address this challenge by using brain-inspired principles to co-design the ANN hardware and algorithms for improved size, weight, and power (SWaP) consumption. Unfortunately, though, standard gradient-based ANN training algorithms like backpropagation incur a large overhead when they are implemented in neuromorphic hardware. In addition, the gradient calculations required for the backpropagation algorithm are especially challenging to implement in neuromorphic hardware, which typically has low precision, functional discontinuities, non-linear weights, and several other non-ideal behaviors. This thesis proposes a novel circuit design for training memristor-based neuromorphic systems using the weighted sum simultaneous perturbation algorithm (WSSPA). WSSPA is a zeroth-order ANN training algorithm that estimates loss gradients using perturbations of neuron inputs, avoiding large-overhead circuitry needed for backpropagation. The training circuit optimizes the ANN by adjusting the conductance of the memristor-based weights using the loss gradient estimates. Current mode design techniques, including the translinear principle, are used to significantly reduce the number of transistors required for existing implementations, thereby improving the SWaP efficiency. The proposed circuit consists of a sample and hold circuit, an error calculation circuit, a memristor threshold voltage selector, and synapse input selector circuits. The design was implemented in LTSpice, using 45 nm predictive technology MOSFET models and a simple linear memristor model fit to published experimental data. Demonstration of the proposed hardware was carried out by training an ANN to perform Boolean logic functions such as XOR. Results show that the current mode circuit design of WSSPA is able to converge on the correct functionality within 16 training iterations. After this, the ANN output current oscillates around the target. Areas for future work include making the feedback adjustment voltage value dependent on the error magnitude, rather than just the sign. This will help the error to converge to 0 after training, eliminating output oscillations. In addition, future studies should confirm the scalability of the proposed design for larger neural networks.
Publisher Site: RIT Repository
PDF (Requires Sign-In): PDF
Click for Bibtex
@book{matthews2023design, title = {Design of a Current Mode Circuit for Zeroth-Order Optimization of Memristor-Based Neuromorphic Systems}, author = {Matthews, Shery George}, year = {2023}, school = {Rochester Institute of Technology} }
[T-2023-17] Namita Bhosle. (2023). “Programmable Processing-in-Memory Core and Cluster Design and Verification.” Rochester Institute of Technology.
Abstract: The surge in demand for semiconductors and AI-driven applications has led to an amplified requirement for swift and efficient semiconductor design production cycles. These shortened cycles, however, pose a challenge as they reduce the time available for complex chip design and verification, consequently increasing the likelihood of producing error-prone chips. Current research concentrates on utilizing a Lookup Table (LUT) based pPIM (Programmable Processor in Memory) technology to deliver efficient, low-latency, and low-power computation specifically tailored to 4-bit data requirements, ideal for repetitive and data-expensive AI algorithms. This thesis presents the user-defined Generation 2 pPIM, a redesigned and enhanced version of the existing static Generation 1 architecture, offering a scalable, configurable, and fully automated framework to expedite the design, verification, and implementation process. The user-defined Gen 2 pPIM Cluster, consisting of nine interconnected user-defined Gen 2 pPIM Cores and an included Accumulator, extends the capability to execute complex operations like Multiply-and-Accumulate (MAC). Both the Gen 2 pPIM Core and Gen 2 pPIM Cluster undergo extensive verification and testing. A Python-based suite has been developed to enable user-specific Gen 2 pPIM Core and Gen 2 pPIM Cluster design and verification efficiently, providing an end-to-end automated toolkit catering to the user's specific needs. All variants of core and cluster design are benchmarked with 28nm, 65nm and 180nm technology libraries and are compared for area, power and timing.
Publisher Site: RIT Repository
PDF (Requires Sign-In): PDF
Click for Bibtex
@book{bhosle2023programmable, title = {Programmable Processing-in-Memory Core and Cluster Design and Verification}, author = {Bhosle, Namita}, year = {2023}, school = {Rochester Institute of Technology} }
[T-2023-16] Benjamin James Vaughan. (2023). “Commissioning the Tomographic Ionized-carbon Mapping Experiment.” Rochester Institute of Technology.
Abstract: Line intensity mapping is a novel technique that traces the evolution of cosmological structure sourcing a particular emission line through measurements of the aggregate emission of all galaxies along a line of sight. The Tomographic Ionized-carbon Mapping Experiment (TIME) is a far infrared waveguide imaging spectrometer that operates from 183-323 GHz, allowing it to trace the emission of the singly ionized carbon fine structure line ([CII], 157.7 µm) from redshift 5 to 9 to probe the infrared emission from star formation during the Epoch of Reionization. Further, TIME will also perform line intensity mapping observations of several carbon monoxide rotational lines during cosmic noon, constraining the column density of molecular hydrogen that is the raw fuel for star formation. As a secondary goal, TIME will be able to measure the line of sight peculiar velocity of galaxies using the kinetic Sunyaev-Zeldovich effect. The instrument design is similar to the now decommissioned Z-spec, a millimeter wave spectrometer that was deployed at the Caltech Sub-millimeter Observatory. TIME is comprised of 32 individual curved grating spectrometers that are coupled to an array of 1920 transition edge sensor bolometers and placed in the optical path of the Arizona Radio Observatory 12M Telescope, located in Kitt Peak, Arizona. In this work I will describe the scientific motivation for this project, its mechanical and optical design, and results from its commissioning in the winter of 2021.
Publisher Site: RIT Repository
PDF (Requires Sign-In): PDF
Click for Bibtex
@book{vaughan2023commissioning, title = {Commissioning the Tomographic Ionized-carbon Mapping Experiment}, author = {Vaughan, Benjamin James}, year = {2023}, school = {Rochester Institute of Technology} }
[T-2023-15] Winston O. James III. (2023). “3D Computational Modeling of Multiphase Phenomena in Boiling Heat Transfer.” Rochester Institute of Technology.
Abstract: Boiling heat transfer is used in industrial processes, such as heat exchangers, nuclear reactors, and high-powered electronics. There is a need to generate effective heat dissipation techniques to increase the operation limits of these technologies. To enhance heat dissipation with boiling flows, it is important to study boiling phenomena from a fundamental perspective to identify the relation between the fluid and heat transfer behavior near the interface during bubble growth. Boiling simulations have been used as a tool to visualize and quantify the heat transfer mechanisms near the interface. However, challenges exist in modeling bubble growth with a sharp interface and with a saturation condition. In addition, 3D modeling of bubble growth leads to simulations with millions of computational cells and requires expensive computer resources with multicore capabilities. The present work proposes methods to perform high-fidelity and time-saving 3D simulations of nucleate boiling. These methods account for 3D sharp interface and thermal conditions of saturation temperature while considering adaptive mesh refinement. The adaptive mesh refinement creates small cells only at critical regions near the interface and coarse cells at regions far from the bubble edge. User defined functions (UDFs) were developed to customize the software Ansys-Fluent to preserve the interface sharpness, maintain saturation temperature conditions, and perform effective adaptive mesh refinement in 3D. UDFs preserve the interface sharpness by declaring mass transfer only at interface-cells located next to vapor-cells. To impose a condition of saturation temperature, a UDF interpolates the temperature of the interface-cell based on the saturation temperature and the interface curvature. Adaptive mesh refinement is accomplished by a UDF that identifies the cells near the contact line and liquid-vapor interface and applies the adaptive mesh refinement algorithms only at the identified cells. Additionally, an external microlayer model was implemented to account for submicron evaporation at the contact line. Validating the approach considered spherical bubble growth in superheated liquid, and the observed agreement between theoretical and simulation bubble growth rates within 10%. Simulations results of bubble growth over a heated surface revealed an influence region of 3 times the bubble departure diameter. The simulation captured temperature distribution near the contact line showing a meniscus with high temperature gradients. Moreover, results revealed a reduction of 75 hours of computational time with adaptive mesh compared with the uniform computational mesh. The present work demonstrates the use of customized Ansys-Fluent in performing 3D numerical simulations of nucleate boiling. The developed simulation approach is a reliable and effective tool to investigate 3D boiling phenomena by accurately capturing the thermal and fluid dynamic interfacial vapor-liquid interaction and reducing the computational time.
Publisher Site: RIT Repository
PDF (Requires Sign-In): PDF
Click for Bibtex
@book{james iii20233d, title = {3D Computational Modeling of Multiphase Phenomena in Boiling Heat Transfer}, author = {James III, Winston O.}, year = {2023}, school = {Rochester Institute of Technology} }
[T-2023-14] Alances Vargas. (2023). “The Nine Dot Problem: an Immersive Experience Blending the Digital and Physical Worlds.” Rochester Institute of Technology.
Abstract: Anamorphic billboards, the incredibly eye-catching social media magnets one sees where a digital shoe seems to be breaking through the walls of a building or a hand is reaching out through the screens of Times Square into the streets of New York, are commonly seen in major urban environments. These displays, often used for marketing purposes selling the next hit video game or popular sneaker, are made by joining 2 angled screens at a 90 degree angle and utilize the concept of forced perspective to create the illusion of space and 3D visuals without the need for special glasses. However, this technique is often fairly limited in terms of its applications as the displays require a viewer to be looking at them from a very precise angle to experience the illusion. Any other view point yields a heavily warped image. As a result anamorphic billboards are often most successful from a distance as it limits the possible viewing angles of any spectator. The Nine Dot Problem, on the contrary, is an installation that uses 3D motion graphics and experience design to offer a solution into how one might bring these awe inspiring displays into a smaller more intimate setting. By utilizing black and white geometric visuals, and the simplicity in the shape of a cube, The Nine Dot Problem is able to maintain the integrity of the illusion even while the viewer is up close to the screens, effectively reducing the scale necessary for this technique to be viable in the field of design.
Publisher Site: RIT Repository
PDF (Requires Sign-In): PDF
Click for Bibtex
@book{vargas2023the, title = {The Nine Dot Problem: an Immersive Experience Blending the Digital and Physical Worlds}, author = {Vargas, Alances}, year = {2023}, school = {Rochester Institute of Technology} }
[T-2023-13] Chamodhi Ravihansi Polgampola Ralalage. (2023). “Analysis of the Aerosol Composition, Its Deposition and Uptake in Electronic Cigarette Users.” Rochester Institute of Technology.
Abstract: Electronic cigarettes (e-cigarettes) generate aerosol (particles in a gas matrix) by vaporizing the e-liquid, consisting of propylene glycol (PG), glycerol (GL), nicotine, and other additives. Aerosol enters the respiratory tract (RT) of the user, where part of it is deposited, while the rest is exhaled. Compounds in the gas and particle phase have different deposition mechanisms in the RT, thus determining the location of deposition. The location of deposition and amount of a compound will influence the biological response in the body. Identifying the composition of each constituent in the gas phase and particle phase would enhance the understanding of their deposition in the RT, thus absorption into the body. Study 1: assess the composition difference in aerosol particle phase (PP) from its e-liquid, and identify the mechanism associated with the composition difference. Gas chromatography analysis was conducted on e-liquid and aerosol PP for PG, GL, and nicotine. Experiments were designed to isolate the mechanism for composition difference by testing evaporation, aging, vaporization, and condensation. Study 2: streamline a methodology to study impact of user-specific parameters on aerosol deposition in e-cigarette users. A pilot study was conducted with participation of e-cigarette consumers to test the usability of exhale breath collection device. Feasibility of quantifying yield, exhale breath, puff and respiratory topographies were evaluated. Salivary cotinine boost was quantified to measure nicotine uptake. PG in aerosol PP showed a significant reduction from the e-liquid. Nicotine composition difference between e-liquid and the PP for non-acidified e-liquid was greater from acidified e-liquids. Study data is in agreement with the condensation model to explain the composition change of aerosol PP vs the initial e-liquid. The pilot study successfully quantified exhale breath composition, deposition efficiencies, salivary cotinine boost, puff and respiratory topographies. Findings confirmed the composition of aerosol PP to be different from its e-liquid and identified the mechanism for the difference. Pilot study successfully demonstrated the proof of concept to study the impact of user-specific parameters in e-cigarette users.
Publisher Site: RIT Repository
PDF (Requires Sign-In): PDF
Click for Bibtex
@book{ralalage2023analysis, title = {Analysis of the Aerosol Composition, Its Deposition and Uptake in Electronic Cigarette Users}, author = {Ralalage, Chamodhi Ravihansi Polgampola}, year = {2023}, school = {Rochester Institute of Technology} }
[T-2023-12] Colin J. Maloney. (2023). “A Study on the Impact of Nonlinear Effects on Hyperspectral Sub-Pixel Target Detection.” Rochester Institute of Technology.
Abstract: In the realm of hyperspectral (HS) sub-pixel target detection, the Linear Mixing Model (LMM) proposes that the macroscopic interaction between incident light and materials in a scene may be modeled as linear. For individual pixels in hyperspectral data which contain multiple materials, this means that they may be accurately represented by a linear combination of the spectra of those pure materials, which are often called endmembers. However, when nonlinear mixing, such as shadowing and adjacent reflections, are present, the foundational assumptions of the LMM are violated and its accuracy in predicting target detection performance is reduced. This thesis aims to investigate the impact of such nonlinear effects on the performance of the LMM with regards to a HS sub-pixel target detection task. To quantify the impact of nonlinear effects, an experimental data collect was completed in September 2022 with a Headwall Nano HSI sensor at RIT's Tait Preserve. The Headwall Nano has 272 bands in the visible to the near-infrared (VNIR) region of the electromagnetic spectrum. This data collect utilized five novel sub-pixel targets designed by Chase Canas with pre-determined fill fractions of 100%, 80%, 60%, 40%, and 20%. In addition to the collection of experimental data, two types of modeling software were leveraged to produce results that were based on the LMM and that utilized a path-tracing technique which is better able to deal with nonlinear effects. The Forecasting and Analysis of Spectroradiometric System Performance (FASSP) model was designed to predict HS sub-pixel target detection performance. The LMM is foundational to FASSP's systems' performance computations and is used by FASSP to model the propagation of spectral radiance from a user-specified scene through a MODTRAN-informed atmosphere to a user-defined sensor. FASSP relies on a vast array of user inputs to specify the sensor parameters and post-processing algorithms used to detect a target spectrum. FASSP also includes parameters that can account for the shadowing of the target class. As FASSP has been validated in previous studies, it is a reliable reference that can be used to simulate the performance of the LMM both when nonlinear effects are and aren't present. The Digital Imaging and Remote Sensing Image Generation (DIRSIG) model was first developed in the 1990s by the Digital Imaging and Remote Sensing (DIRS) lab at the Chester F. Carlson Center for Imaging Science (CIS) at the Rochester Institute of Technology (RIT). DIRSIG utilizes a path-tracing model and the three-dimensional geometry of a scene and the spectral properties of the materials within to generate radiometrically-accurate synthetic data that is able to capture nonlinear effects. As a result of the differences in their modeling paradigms, FASSP and DIRSIG provide two different perspectives on a systems' performance analysis of a HS sub-pixel target detection task. Four setups were designed for the experimental collect to investigate the effects of shadowing and non-linear mixing on the performance of the LMM. These four setups were replicated within FASSP and DIRSIG. Five targets with varying fill fraction percentages were painted a distinct color and served as the target class within the scene. To replicate the effects of shadowing, five panels were constructed and placed to shadow the five targets. To induce multiple reflections and spectral contamination, five treeshine reflector (TR) panels were constructed and painted with a bright red paint. To determine the effectiveness of this contamination and to inform specular material descriptions in DIRSIG, the Bi-Directional Reflectance Factor (BRF) of the TR panels was also measured with RIT's Goniometer at the Rochester Institute of Technology version Two (GRIT-T) instrument. The four setups consisted of a base setup with no shadowing or TR panels, a shadowing panel setup, a TR-S panel setup with both the shadowing and TR panels, and a TR panel setup. Results were produced in the form of predicted mean target radiances, Signal-to-Noise-Ratios (SNRs) and target detection performances in the form of Receiver-Operating Characteristic (ROC) curves, and Area-Under-the-Curves (AUCs). By leveraging the three sources of data (experimental, the LMM-based FASSP, and the path-tracing DIRSIG), a unique parallel analysis was performed to determine the ability of each modeling approach to deal with shadowing and adjacent reflections. It was found that FASSP's ability to accurately predict mean target radiances, SNRs, and target detection performances was degraded dramatically relative to DIRSIG when shadowing was present. Its accuracy was also degraded when adjacent reflections were present, but not to the same degree. Results were also produced to examine the impact of including a description of the TR panel specularity in the DIRSIG simulations; it was found that utilizing a Lambertian TR panel resulted in higher mean target radiances than a specular one, but only when the shadowing was also present. The results from DIRSIG indicated a high level of accuracy, however, more work could be conducted to improve the user inputs to ensure optimal settings.
Publisher Site: RIT Repository
PDF (Requires Sign-In): PDF
Click for Bibtex
@book{maloney2023a, title = {A Study on the Impact of Nonlinear Effects on Hyperspectral Sub-Pixel Target Detection}, author = {Maloney, Colin J.}, year = {2023}, school = {Rochester Institute of Technology} }
[T-2023-11] Dongyu Wu. (2023). “Type- and Control-flow Analysis for System Fw.” Rochester Institute of Technology.
Abstract: Type- and control-flow analysis combines control-flow analysis and type-flow analysis. It improves the approximation given by control-flow analysis by using type-flow information to filter out abstract values with incompatible types. Type-flow analysis and control-flow analysis are mutually beneficial since the control-flow analysis approximates the Λ-expressions in type applications. Previously, a type- and control-flow analysis for System F has been defined. However, System F only has limited support for polymorphism. System Fω, a more sophisticated type system, augments System F with type-level functions, thereby introducing abstract type constructors. It enables the use of generic functions that are parameterized by polymorphic data structures. In this work, a new type- and control-flow analysis for System Fω is defined. This work includes a specification-based formulation of the type- and control-flow analysis for System Fω and a proof of the soundness of the analysis. This work presents a flow-graph-based formulation. The soundness of the flow-graph-based formulation is proved by relating to the specification-based formulation.
Publisher Site: RIT Repository
PDF (Requires Sign-In): PDF
Click for Bibtex
@book{wu2023type-, title = {Type- and Control-flow Analysis for System Fw}, author = {Wu, Dongyu}, year = {2023}, school = {Rochester Institute of Technology} }
[T-2023-10] Andrew O’Brien. (2023). “Self-Assembling Peptide-Based High-Relaxivity Prostate-Cancer-Targeted MRI Contrast Agents.” Rochester Institute of Technology.
Abstract: Magnetic Resonance Imaging (MRI) is a widely used, highly effective imaging method for diagnosing cancer and guiding treatment. MRI contrast agents enhance image quality, with higher relaxivity (r1) contrast agents providing the best utility. Attaching a cancer-specific-targeting group enhances the image further. Therefore, a high-relaxivity cancer-targeted contrast agent is a highly desirable tool for diagnosis and treatment. Our group previously synthesized a peptide-based MRI contrast agent with the Ala(Gd-DO3A) chelator that targeted the prostate cancer biomarker PSMA using the small-molecule ligand DCL. While this agent displayed nominal relaxivity, it was serendipitously found that the relaxivity of a synthetic intermediate containing Fmoc-protected tryptophan (Trp) increased dramatically. This was hypothesized to occur due to intermolecular self-assembly above a critical aggregation concentration (CAC). The first goal was to expand upon this finding by designing peptide-based MRI contrast agents using the simpler Lys(Gd-DOTA) chelator developed earlier in our lab. Di- and tripeptides were synthesized to test the effect of peptide length, net charge, and relative location and stereochemistry of Fmoc, Trp, and Lys(Gd-DOTA) on self-assembly through measuring r1 at 1.0 Tesla. Self-assembly was found to increase r1 from 3-7 to 13-17 mM-1s-1 in H2O and PBS and 11-13 mM-1s-1 in FBS, with remarkably low CACs in FBS. The second goal was to investigate self-assembly using other motifs, including Cbz-protected Trp, two adjacent Trp residues, and other Fmoc-protected aromatic amino acids. The third goal was to apply these findings to synthesize high-relaxivity cancer-targeted MRI contrast agents. Two prostate-cancer-targeted self-assembling MRI contrast agents containing DCL and a breast-cancer-targeted MRI contrast agent containing the peptide 18-4 were synthesized. This work represents the first example of self-assembly in MRI contrast agents induced by Fmoc and aromatic amino acids, and in the broader scope, the synthetic approach is amenable to a wide variety of self-assembling cancer-targeted MRI contrast agents.
Publisher Site: RIT Repository
PDF (Requires Sign-In): PDF
Click for Bibtex
@book{o'brien2023self-assembling, title = {Self-Assembling Peptide-Based High-Relaxivity Prostate-Cancer-Targeted MRI Contrast Agents}, author = {O'Brien, Andrew}, year = {2023}, school = {Rochester Institute of Technology} }
[T-2023-09] Jesdin Raphael. (2023). “Prediction of Unplanned 30-Day Readmission of Heart Failure Patients Using LSTM.” Rochester Institute of Technology.
Abstract: In the context of heart failure, a leading cause of hospitalization in the United States, approximately 15\% of patients discharged within 30 days face readmission, contributing to escalated healthcare costs and compromised clinical outcomes. This study leverages a wealth of personal information extracted from Emergency Health Records (EHRs) spanning two decades. This research aims to enhance the predictive capabilities of re-hospitalization for cardiac patients by creating a two-stage LSTM model while incorporating features into regression and time series datasets. A critical aspect of this study involves ensuring the integration of all relevant features across both datasets. Including constant data elements, such as demographics and cohort-level statistics, alongside time series data in the model is deemed suboptimal. Consequently, the time series model accommodates two distinct feature sets—one dedicated to time series information and the other to constant data. This work demonstrates that the utilization of time series modeling significantly improves predictive outcomes. Leveraging Recurrent Neural Networks (RNNs) in the form of an LSTM model, the research emphasizes the superiority of this approach in enhancing re-hospitalization prediction accuracy for cardiac patients.
Publisher Site: RIT Repository
PDF (Requires Sign-In): PDF
Click for Bibtex
@book{raphael2023prediction, title = {Prediction of Unplanned 30-Day Readmission of Heart Failure Patients Using LSTM}, author = {Raphael, Jesdin}, year = {2023}, school = {Rochester Institute of Technology} }
[T-2023-08] Kesa M. Abbas. (2023). “Application of AI in Financial Sector: Earnings Call Dataset Analysis.” Rochester Institute of Technology.
Abstract: Deep learning has emerged as a cornerstone in diverse scientific domains, demonstrating profound implications both in academic research and conventional applications. The utilization of deep learning has branched to the financial sector for stock prediction, management of assets, credit score analysis, etc. During the end of the financial fiscal year, top executives present their companies' growth and losses in earnings calls. These earnings calls invariably webcasted, furnish stakeholders with insights into a firm's fiscal performance during a given period. These audios along with transcripts are published on the company website for the general public. If these audio and transcripts are collected over time, they are capable of forming an extensive audio and sentiment analysis database, which could be utilized to train a model effectively. The quality and quantity of the dataset are essential for a machine-learning model. The availability of these discussions in both audio and text formats on corporate portals presents an invaluable repository for longitudinal audio and sentiment analysis. The clarity in the statistics of the earnings calls would also predict the bona fide outlook of the companies' financial prospects. This thesis work will focus on adding and extending the earnings call data for the companies for MAEC dataset and create a diverse, updated machine learning dataset. This would be further followed by the proposal of a sentiment analysis tool inspired by the deep neural network to label the data collected and form the baseline method for future work to compare. The established algorithm would provide a pathway for a dataset and data annotation that would further be utilized by the models out there, to perform financial analysis and factual correctness of the data presented, and draw appropriate conclusions. The addition to this dataset and the creation of an algorithm would involve rigorous empirical investigation and the iterative design of a methodological framework conducive to the systematic accrual and annotation of earnings call records.
Publisher Site: RIT Repository
PDF (Requires Sign-In): PDF
Click for Bibtex
@book{abbas2023application, title = {Application of AI in Financial Sector: Earnings Call Dataset Analysis}, author = {Abbas, Kesa M.}, year = {2023}, school = {Rochester Institute of Technology} }
[T-2023-07] Arnab Ghosh. (2023). “Shift Variant Image Deconvolution using Deep Learning.” Rochester Institute of Technology.
Abstract: Image Deconvolution is a well-studied problem that seeks to restore the original sharp image from a blurry image formed in the imaging system. The Point Spread function(PSF) of a particular system can be used to infer the original sharp image given the blurred image. However, such a problem is usually simplified by making the shift-invariant assumption over the Field of View (FOV). Realistic systems are shift-variant; the optical system's point spread function depends on the position of the object point from the principal axis. For example, asymmetrical lenses can cause space variant aberration. In this paper, we first simulate our shift-variant aberrations by generating Point Spread Functions using the Seidel Aberration polynomial and use a shift-variant forward blur model to generate our shift-variant blurred image pairs. We then introduce, ShiVaNet. It is a two-stage architecture that builds upon the Learnable Wiener Deconvolution block as described in Yanny, Monakhova, Shuai, and Waller (Yanny et al.) by introducing Simplified Channel Attention and Transpose Attention to improve the performance of the module. We also devise a novel UNet refinement block by fusing a ConvNext-V2 block with Channel Attention and coupling with Transposed Attention Zamir, Arora, Khan, Hayat, Khan, and Yang (Zamir et al.). Our model performs better than state-of-the-art restoration models by a factor of 0.2 dB Peak Signal to Noise Ratio.
Publisher Site: RIT Repository
PDF (Requires Sign-In): PDF
Click for Bibtex
@book{ghosh2023shift, title = {Shift Variant Image Deconvolution using Deep Learning}, author = {Ghosh, Arnab}, year = {2023}, school = {Rochester Institute of Technology} }
[T-2023-06] Saurabh Bodke. (2023). “Hybrid Test-Smell Based Approach for Prediction of Flaky Tests.” Rochester Institute of Technology.
Abstract: Regression testing is an essential component in software development, aimed at verifying that recent code changes do not negatively impact existing functionalities. A prevalent challenge in this area is the occurrence of flaky tests, which exhibit inconsistent outcomes—passing or failing without any code alterations. Such tests erode the reliability of automated testing frameworks, leading to increased debugging efforts and diminished confidence in software stability. This research addresses the challenge of flaky tests by exploring machine learning and prediction models, specifically focusing on test smells and four principal root causes of flakiness: Async Wait, Concurrency, Test Order Dependency, and Resource Leak. While existing methods that analyze test case vocabulary often encounter issues such as elevated operational costs, tendencies to overfit, and context sensitivity, our study enhances the accuracy of identifying flaky tests by incorporating these four additional determinants into the test smell-based approach. We conducted a thorough evaluation of this augmented model's predictive capabilities in a cross-project context and analyzed the contribution of each newly added flakiness factor in terms of information gain. Our findings indicate that Async Wait and Concurrency, in particular, show the highest information gain, highlighting their significant role in flakiness prediction. Moreover, we compared the performance of our hybrid feature based approach against both the vocabulary-based approach and the traditional test-smell based approach, focusing on the improved accuracy, precision, recall in predicting flaky tests.
Publisher Site: RIT Repository
PDF (Requires Sign-In): PDF
Click for Bibtex
@book{bodke2023hybrid, title = {Hybrid Test-Smell Based Approach for Prediction of Flaky Tests}, author = {Bodke, Saurabh}, year = {2023}, school = {Rochester Institute of Technology} }
[T-2023-05] Rajiv Snape. (2023). “Antibiotics and Secondary Metabolites Analysis SHell (antiSMASH) as a tool to detect putative novel antibiotics.” Rochester Institute of Technology.
Abstract: In the Hudson Lab, which is focused on discovering new antibiotics, bacteria samples are taken from the environment and cultured in large quantities. Then they are tested for antibiotic resistance before they are sequenced and their secondary metabolite compounds are extracted. This is both a lengthy and expensive process that becomes more and more difficult as the number of samples one is working increases. This project assessed a different approach to rejuvenate antibiotic development with antiSMASH. antiSMASH is an online tool created by collaborators from many different institutions that uses profile Hidden Markov Models (pHMMs) to detect gene clusters which produce secondary metabolites in bacteria. The antiSMASH tool has its own repository of these "profiles" which are position specific information about an amino acid from a protein encoding gene derived from multiple sequence alignments. Once a genome is entered into antiSMASH, if these profile modules are detected and they are outputted to the user if a certain metabolite/cluster is present. Many gene clusters are known to produce metabolites with antimicrobial properties which the antiSMASH tool could potentially detect. Using this tool, the goal was to identify a potential pipeline of antibiotic discovery that would be a great improvement in time and reduce costs by using the tool as a screen of a possible viable candidate for antibiotics. In this project 30 genomes were used and fed into antiSMASH. They were broken down into positive and negative controls, known producers and unknown producers. We then looked at the tools ability to screen for antibiotics in each of those data types.
Publisher Site: RIT Repository
PDF (Requires Sign-In): PDF
Click for Bibtex
@book{snape2023antibiotics, title = {Antibiotics and Secondary Metabolites Analysis SHell (antiSMASH) as a tool to detect putative novel antibiotics}, author = {Snape, Rajiv}, year = {2023}, school = {Rochester Institute of Technology} }
[T-2023-04] Griffin Hurt. (2023). “Normalization and Generalization in Deep Learning.” Rochester Institute of Technology.
Abstract: In this thesis, we discuss the importance of data normalization in deep learning and its relationship with generalization. Normalization is a staple of deep learning architectures and has been shown to improve the stability and generalizability of deep learning models, yet the reason why these normalization techniques work is still unknown and is an active area of research. Inspired by this uncertainty, we explore how different normalization techniques perform when employed in different deep learning architectures, while also exploring generalization and metrics associated with generalization in congruence with our investigation into normalization. The goal behind our experiments was to investigate if there exist any identifiable trends for the different normalization methods across an array of different training schemes with respect to the various metrics employed. We found that class similarity was seemingly the strongest predictor for train accuracy, test accuracy, and generalization ratio across all employed metrics. Overall, BatchNorm and EvoNormBO generally performed the best on measures of test and train accuracy, while InstanceNorm and Plain performed the worst.
Publisher Site: RIT Repository
PDF (Requires Sign-In): PDF
Click for Bibtex
@book{hurt2023normalization, title = {Normalization and Generalization in Deep Learning}, author = {Hurt, Griffin}, year = {2023}, school = {Rochester Institute of Technology} }
[T-2023-03] Jason D. Rakowsky. (2023). “Analyzing the Atomic and Electronic Structures of Oxide Heterostructures for Electrolyte Applications in Solid Oxide Fuel Cells.” Rochester Institute of Technology.
Abstract: Perovskite oxide heterostructures have key applications in technologies such as solid oxide fuel cells (SOFCs), batteries, solar cells, etc. because of novel properties at the interface. Although it is well-known that this is caused by lattice mismatch and misfit dislocations, the role defects play in enhancing certain properties is unknown. We have investigated SrTiO$_3$ (STO) and BaZrO$_3$ (BZO) structures because they both experience novel oxygen vacancy formation which is desired for high ionic conductivity applications such as the thin film electrolyte of a SOFC. Through explicit density functional theory calculations of four STO/BZO interfaces, electron migration, oxygen vacancy formation energies, and density of states (DOS) plots have been analyzed and compared. From identifying the most optimal interfaces for ion transport, we have developed a method of predicting which solid oxide heterostructures take the most advantage of strain relaxation and misfit dislocations. Out of the four orientations of the STO/BZO heterostructure, doped ZrO$_2$-TiO$_2$ calculations contained sites of spontaneous vacancy formation while the orientations BaO-SrO and SrO-ZrO$_2$ have been identified with defects that are undesirable for vacancies. Discussion is offered on the extent that ground state electronic structures can guide material analysis for nanoionic devices.
Publisher Site: RIT Repository
PDF (Requires Sign-In): PDF
Click for Bibtex
@book{rakowsky2023analyzing, title = {Analyzing the Atomic and Electronic Structures of Oxide Heterostructures for Electrolyte Applications in Solid Oxide Fuel Cells}, author = {Rakowsky, Jason D.}, year = {2023}, school = {Rochester Institute of Technology} }
[T-2023-02] Isaac Olantunji. (2023). “Multimodal AI for Prediction of Distant Metastasis in Carcinoma Patients.” Rochester Institute of Technology.
Abstract: Metastasis of cancer is directly related to death in almost all cases, however a lot is yet to be understood about this process. Despite advancements in the available radiological investigation techniques, not all cases of Distant Metastasis (DM) are diagnosed at initial clinical presentation. Also, there are currently no standard biomarkers of metastasis. Early, accurate diagnosis of DM is however crucial for clinical decision making, and planning of appropriate management strategies. Previous works have achieved little success in attempts to predict DM from either clinical, genomic, radiology, or histopathology data. In this work we attempt a multimodal approach to predict the presence of DM in cancer patients by combining gene expression data, clinical data and histopathology images. We tested a novel combination of Random Forest (RF) algorithm with an optimization technique for gene selection, and investigated if gene expression pattern in the primary tissues of three cancer types (Bladder Carcinoma, Pancreatic Adenocarcinoma, and Head and Neck Squamous Carcinoma) with DM are similar or different. Gene expression biomarkers of DM identified by our proposed method outperformed Differentially Expressed Genes (DEGs) identified by the DESeq2 software package in the task of predicting presence or absence of DM. Genes involved in DM tend to be more cancer type specific rather than general across all cancers. Our results also indicate that multimodal data is more predictive of metastasis than either of the three unimodal data tested, and genomic data provides the highest contribution by a wide margin. Also, the results re-emphasize the importance for availability of sufficient image data when a weakly supervised training technique is used. Code is made available at: https://github.com/rit-cui-lab/Multimodal-AI-for-Prediction-of-Distant-Metastasis-in-Carcinoma-Patients.
Publisher Site: RIT Repository
PDF (Requires Sign-In): PDF
Click for Bibtex
@book{olantunji2023multimodal, title = {Multimodal AI for Prediction of Distant Metastasis in Carcinoma Patients}, author = {Olantunji, Isaac}, year = {2023}, school = {Rochester Institute of Technology} }
[T-2023-01] Ryan Willis. (2023). “Measurements of the Sunyaev- Zel’dovich effect in a Herschel- SPIRE Survey of Galaxy Clusters.” Rochester Institute of Technology.
Abstract: As the largest gravitationally bound objects in the modern universe, galaxy clusters are excellent environments for studying the large-scale structure and evolution of matter in the cosmos. The environments of galaxy clusters change significantly over time from sites of intense star formation at z ~ 2-4 to mostly quenched objects with very little star formation in the current epoch. During this evolution, galaxy clusters also establish an intra-cluster medium (ICM) of hot plasma, which is typically firmly in place by a redshift of z ~ 1. The properties of the ICM are intimately tied to the formation history of the cluster. Cluster mergers are well known to affect the thermodynamics of the ICM, but collisional interactions between dust and gas particles in the ICM may also affect the ICM thermodynamics in ways that are not yet well understood. One way to study the properties of the ICM is by measuring the distortion in the cosmic microwave background (CMB) caused by the hot ICM, known as the Sunyaev-Zel'dovich (SZ) effect, which can be measured at millimeter and sub-millimeter wavelengths. The research presented in this thesis combines data from the space-based observatories Herschel Space Observatory, Hubble Space Telescope, Chandra X-ray Observatory, and ground based sub-mm telescopes to measure the SZ spectrum. A probabilistic component separation tool is used to disentangle the SZ signal from other positive signals in Herschel maps, HST observations are used to account for the effects of lensing, and ICM temperatures from Chandra are combined with data from ground based sub-mm telescopes to estimate the shape and amplitudes of the SZ effect at sub-millimeter wavelengths. In this work, I present updates to an already established pipeline for measuring the SZ effect and results for a sample of eight galaxy clusters.
Publisher Site: RIT Repository
PDF (Requires Sign-In): PDF
Click for Bibtex
@book{willis2023measurements, title = {Measurements of the Sunyaev- Zel'dovich effect in a Herschel- SPIRE Survey of Galaxy Clusters}, author = {Willis, Ryan}, year = {2023}, school = {Rochester Institute of Technology} }
[T-2022-39] Siddhant Reddy. (2022). “Unsupervised Transfer Learning with Autoencoders.” Rochester Institute of Technology.
Abstract: There have been massive improvements in the field of computer vision in recent years, mostly due to the efficacy of deep learning. Most if not all of these tasks involve use of supervised learning. However, building a labelled dataset of sufficient volume can be prohibitively expensive. This is where transfer learning is applied, where the predictive models are trained on a related pretext task(most commonly using supervised learning) before being fine-tuned on the target task. This reduces the volume of data required for the target task but we are still bound by the volume of labelled data available for the pre-training task. Unsupervised transfer learning by removing the need for labelled data would allow any image to be used as a datapoint for a computer vision task. Autoencoders are a class of unsupervised learning models. Autoencoders trained on image reconstruction can be fine-tuned on a target task such as image classifcation and semantic segmentation to perform unsupervised transfer learning. This thesis seeks to test the efficacy of using autoencoders (image reconstruction) as a pre-training task against other unsupervised tasks. It aims to employ state of the art findings on autoencoders as well as apply modifcations on top of it to maximize unsupervised transfer learning performance. When tested on image classiffication and semantic segmentation it shows 3% to 26% performance improvement depending on the model architecture.
Publisher Site: RIT Repository
PDF (Requires Sign-In): PDF
Click for Bibtex
@book{reddy2022unsupervised, title = {Unsupervised Transfer Learning with Autoencoders}, author = {Reddy, Siddhant}, year = {2022}, school = {Rochester Institute of Technology} }
[T-2022-38] Shogo Honda. (2022). “Speech Feature Analysis and Discrimination in Biological Information.” Rochester Institute of Technology.
Abstract: A silent speech interface is a system that allows people doing speech communication without using their own speech sounds. Today, a variety of speech interfaces have been developed using biological signals such as the eye movement, and the articulatory. These interfaces are mainly for supporting people who have speech disorder to communicate with others, yet there are many speech disorder that have not been addressed by the current technologies. The possible cause of the issue is the limited numbers of the biological signals used for the speech interface. The uncovered issues with speech disorders can be addressed through identifying new biological signals for speech interface development. Therefore, we aim to find new biological signals that can be used for speech interface developments. The biological signals we focused on were the vibration of the vocal folds and brain waves. After measuring the data and extracting the features, we verified whether this data can be used to classify speech sounds through machine learning models: Support Vector Machine for the vocal folds vibration, and Echo State Network for the brain waves. As a result, using the vocal folds vibration signals, Japanese vowels could be classified with 71 % accuracy on average. Using the brain waves, five different consonants were classified with 28.3 % accuracy on average. These findings indicate the possibility that the vocal folds vibration signals and the brain waves can be used as new biological signals for speech interface developments. From this study, we were able to discover some needed improvements that should be considered in the future that may lead to further improvement in the classification accuracy.
Publisher Site: RIT Repository
PDF (Requires Sign-In): PDF
Click for Bibtex
@book{honda2022speech, title = {Speech Feature Analysis and Discrimination in Biological Information}, author = {Honda, Shogo}, year = {2022}, school = {Rochester Institute of Technology} }
[T-2022-37] Akshay Pratap Singh. (2022). “Effects of DC electric field on particle transportation and deposition in evaporating droplets.” Rochester Institute of Technology.
Abstract: Microfluidics(uF) is the science of manipulating and controlling the fluids usually in the range of microliters (10-6L) to picolitres (10-12L). Physical parameters, such as surface tension and contact angle do not play a significant effect on macro scale but play a crucial role at the microscopic level. Microfluidics is viewed as an essential tool for life science research and flexible electronics. A deeper understanding of physical parameters of microfluidics would result in more efficient and lower cost devices (‘Lab-on-a-Chip' devices) and foldable electronics. In short, the concepts of microfluidics can be used to reduce the cost, size, and ease of usage in a wide variety of futuristic products. In our study we are going to explore the effects of electric fields on a particle transport in evaporating droplet and deposition patterns left behind evaporating droplets. We will be studying droplets evaporating on ‘Electrowetting on Dielectric' (EWOD) devices where the droplet is separated from the active electrode by dielectric layers. These types of devices are relevant in a variety of applications such as medical diagnostics and optics. We believe that understanding this phenomenon will impact printing and the development of flexible electronics. This work will further the understanding of transport and deposition of particles in evaporating droplets under applied electric field by understanding the effects of particle concentrations and different dielectric layer. Previous works have demonstrated that particle transport in evaporating droplets and their resultant deposition patterns can be altered under the presence of the electric fields. Applied electric fields have potential to provide real-time control of the particle transport in evaporating droplets by allowing an instantaneous control of the contact line dynamics, electrophoretic manipulation of particles inside the droplet, changes in interface shape, dielectrophoretic manipulation and particle motion inside the droplet due to forces induced by the electric field and evaporation. Our work is going to provide a deeper insight into the effects of DC electric fields on droplets with varying particle concentrations on different dielectric layer. We will better understand the effects of changing the variables (i.e., hydrophobicity ,polarity and particle concentration) under applied DC electric field.
Publisher Site: RIT Repository
PDF (Requires Sign-In): PDF
Click for Bibtex
@book{singh2022effects, title = {Effects of DC electric field on particle transportation and deposition in evaporating droplets}, author = {Singh, Akshay Pratap}, year = {2022}, school = {Rochester Institute of Technology} }
[T-2022-36] Vigneshwar Lakshminarayanan. (2022). “Impact of Noise in Automatic Speech Recognition for Low-Resourced Languages.” Rochester Institute of Technology.
Abstract: The usage of deep learning algorithms has resulted in significant progress in auto- matic speech recognition (ASR). The ASR models may require over a thousand hours of speech data to accurately recognize the speech. There have been case studies that have indicated that there are certain factors like noise, acoustic distorting conditions, and voice quality that has affected the performance of speech recognition. In this research, we investigate the impact of noise on Automatic Speech Recognition and explore novel methods for developing noise-robust ASR models using the Tamil lan- guage dataset with limited resources. We are using the speech dataset provided by SpeechOcean.com and Microsoft for the Indian languages. We add several kinds of noise to the dataset and find out how these noises impact the ASR performance. We also determine whether certain data augmentation methods like raw data augmen- tation and spectrogram augmentation (SpecAugment) are better suited to different types of noises. Our results show that all noises, regardless of the type, had an impact on ASR performance, and upgrading the architecture alone were unable to mitigate the impact of noise. Raw data augmentation enhances ASR performance on both clean data and noise-mixed data, however, this was not the case with SpecAugment on the same test sets. As a result, raw data augmentation performs way better than SpecAugment over the baseline models.
Publisher Site: RIT Repository
PDF (Requires Sign-In): PDF
Click for Bibtex
@book{lakshminarayanan2022impact, title = {Impact of Noise in Automatic Speech Recognition for Low-Resourced Languages}, author = {Lakshminarayanan, Vigneshwar}, year = {2022}, school = {Rochester Institute of Technology} }
[T-2022-35] Duc H. Le. (2022). “Robust L1-norm Singular-Value Decomposition and Estimation.” Rochester Institute of Technology.
Abstract: Singular-Value Decomposition (SVD) is a ubiquitous data analysis method in engineering, science, and statistics. Singular-value estimation, in particular, is of critical importance in an array of engineering applications, such as channel estimation in communication systems, EMG signal analysis, and image compression, to name just a few. Conventional SVD of a data matrix coincides with standard Principal-Component Analysis (PCA). The L2-norm (sum of squared values) formulation of PCA promotes peripheral data points and, thus, makes PCA sensitive against outliers. Naturally, SVD inherits this outlier sensitivity. In this work, we present a novel robust method for SVD based on a L1-norm (sum of absolute values) formulation, namely L1-norm compact Singular-Value Decomposition (L1-cSVD). We then propose a closed-form algorithm to solve this problem and find the robust singular values with cost $\mathcal{O}(N^3K^2)$. Accordingly, the proposed method demonstrates sturdy resistance against outliers, especially for singular values estimation, and can facilitate more reliable data analysis and processing in a wide range of engineering applications.
Publisher Site: RIT Repository
PDF (Requires Sign-In): PDF
Click for Bibtex
@book{le2022robust, title = {Robust L1-norm Singular-Value Decomposition and Estimation}, author = {Le, Duc H.}, year = {2022}, school = {Rochester Institute of Technology} }
[T-2022-34] David Pastuch. (2022). “Single-Input Signature Register-Based Time Delay Reservoir.” Rochester Institute of Technology.
Abstract: Machine learning continues to play a critical role in our society. The ability to automatically identify intricate relationships in large volumes of data has proven incredibly useful for problems such as automatic speech recognition and image processing. In particular, neural networks have become increasingly popular in a wide set of application domains, given their ability to solve complex problems and process high-dimensional data. However, the impressive performance of state-of-the-art neural networks comes at the cost of large area and power consumption for the computation resources used in training and inference. As a result, a growing area of research concerns hardware implementations of neural networks. This work proposes a hardware-friendly design for a time-delay reservoir (TDR), a type of recurrent neural network. TDRs represent one class of reservoir computing neural network topologies, which employ random spatio-temporal feature extraction from time series data in order to produce a linearly separable set of features. Reservoir computing topologies differ from traditional recurrent neural networks because their recurrent weights are fixed, and the only the feedforward output weights need to be trained, usually with linear regression. Previous work on TDRs includes photonic implementation, software implementation, and both digital and analog electronic implementations. This work adds to the body of previous research by exploring the design space of a novel TDR based on single-input signature registers (SISRs), which are common digital circuits used for built-in self-test. The work is motivated by the structural similarity (delayed feedback loop) between TDRs and SISRs, and the possibility of dual-purpose of SISRs for conventional testing as well as machine learning within a single chip. The proposed designs can perform classification on multivariate datasets and perform better than a traditional TDR with quantized reservoir states for parity check, MNIST classification, and temperature prediction tasks. Classification accuracies of up to 100% were observed for some configurations of the SISR for the parity check task and accuracies of up to 85% were observed for MNIST classification. We also observe overfitting on a temperature prediction task with longer data sequences and provide analyses of the results based on the reservoir dynamics, as measured by the rate of divergence between SISR states and the SISR period.
Publisher Site: RIT Repository
PDF (Requires Sign-In): PDF
Click for Bibtex
@book{pastuch2022single-input, title = {Single-Input Signature Register-Based Time Delay Reservoir}, author = {Pastuch, David}, year = {2022}, school = {Rochester Institute of Technology} }
[T-2022-33] Rajiv Mandya Nagaraju. (2022). “Semi-Supervised Video and Image in-painting.” Rochester Institute of Technology.
Abstract: Human brain is inherently good at pattern recognition. AI researchers have always struggled to emulate such levels of performance in machine vision algorithms. Building as an extension of the pattern recognition, humans are also exceptional in selectively learning and bridging the gap when there is missing data. Even when the missing data is in complex images and videos formats. Human brain is able to surmise and comprehend the scene with reasonable certainty given enough contextual information. We believe that selective learning aids in selectively filling the missing data. To this end we experiment with partial convolutions, and the networks can learn selectively. The idea behind partial convolutions is simple. We use the semantic segmentation masks which are obtained from our novel semantic segmentation network and apply convolutions only on the unmasked pixels of the images. When the image embedding is obtained at the end of the encoder, the data from the masked region of the image will be absent in the image embedding. This is encoded onto the latent vector space. When the images are rebuilt again by from the embedding with a decoder network, the object in the masked region is removed. Furthermore, the problem of image/video in-painting are reformulated as a domain transfer problem. This facilitates our network to be trained as semi-supervised learning. Our network uses less computation power while training semi-supervised, end-to-end and while offering performance close to the current state of the art. We test our network extensively on different datasets. The results while experimenting with partial convolutions and selective learning network have been promising. We have used places2 and cityscapes dataset to experiment on images and Davis 2017 Dataset as video dataset.
Publisher Site: RIT Repository
PDF (Requires Sign-In): PDF
Click for Bibtex
@book{nagaraju2022semi-supervised, title = {Semi-Supervised Video and Image in-painting}, author = {Nagaraju, Rajiv Mandya}, year = {2022}, school = {Rochester Institute of Technology} }
[T-2022-32] Andrew C. Allard. (2022). “Machine Learning Based Characterization of preictal EEG.” Rochester Institute of Technology.
Abstract: Convolutional neural networks (CNNs) that incorporate Long Short-term Memory (LSTM) have shown a great deal of success in recognizing preictal activity in electroencephalogram (EEG) analysis. It is postulated that the convolutional portion of the neural network (NN) is using some particular feature or set of features to determine this preictal state. In an attempt to gain a better understanding of these features, Gradient-weighted Class Activation Mapping (Grad-CAM) and augmented Gradient-weighted Class Activation Mapping (augmented Grad-CAM) are applied to the convolutional portion of patient specific neural networks trained to recognize preictal activity. While no particular set of features were consistently highlighted by augmented Grad-CAM, it was possible to discern that some EEG channels strongly influenced an EEG epoch as being correctly labeled as preictal.
Publisher Site: RIT Repository
PDF (Requires Sign-In): PDF
Click for Bibtex
@book{allard2022machine, title = {Machine Learning Based Characterization of preictal EEG}, author = {Allard, Andrew C.}, year = {2022}, school = {Rochester Institute of Technology} }
[T-2022-31] Liam Megraw. (2022). “Invasive Species Identification and Monitoring using Computer Vision, Street View Imagery, and Community Science.” Rochester Institute of Technology.
Abstract: Invasive plants present significant challenges for ecosystem integrity, biodiversity preservation, and agricultural production. Continuous surveillance efforts are necessary to detect and effectively respond to emerging infestations. However, monitoring methods currently available each have their own limits, whether due to cost, time, or sampling bias. Computer vision applied to roadside imagery is a previously undeveloped methodological synergy that can support existing monitoring efforts. Further, because roadsides are a vector of spread, they are an ideal pathway to monitor. In this study, we present research and management applications of a dataset generated by a computer vision model for Phragmites (Common reed) and knotweed complex species across roadsides in New York State. To better understand spread and inform risk assessment, we examined plant presence in relation to road size, site, and culvert characteristics. Results indicated that Phragmites presence decreased with increasing distance from a culvert and was less likely near forested areas and on roadsides with low traffic volume. Results also suggest that the risk of invasion relative to road size is species specific: Phragmites was found to occur more frequently on highway ramps and primary roads, while the knotweed complex was found to occur more often on secondary roads. Additionally, we developed a framework in partnership with stakeholders that enables managers and community scientists to verify, interpret and act upon the very large datasets resulting from computer vision models. Two ArcGIS Dashboards, several GIS layers, and web-based forms were created to this end. Through this work, we identified that culverts and highway ramps should be monitored given their role as Phragmites hotspots. We also generated a new framework that distills a large amount of new data into a form usable by both professionals and the public, expanding upon the capacity of existing monitoring workflows. Supplemental tables S1 and S2 detail data sources, filters, and product dependencies.
Publisher Site: RIT Repository
PDF (Requires Sign-In): PDF
Click for Bibtex
@book{megraw2022invasive, title = {Invasive Species Identification and Monitoring using Computer Vision, Street View Imagery, and Community Science}, author = {Megraw, Liam}, year = {2022}, school = {Rochester Institute of Technology} }
[T-2022-30] Brian Luan. (2022). “Combating Global Warming: Modeling Enhanced Rock Weathering of Wollastonite Using Density Functional Theory.” Rochester Institute of Technology.
Abstract: Negative emissions technologies target the removal of carbon dioxide from the atmosphere as a way of combating global warming. Enhanced rock weathering (ERW) is a vital negative emissions technology that applied globally could remove gigatons of carbon dioxide per year from the atmosphere. In ERW, silicate minerals exposed to the atmosphere trap CO2 via mineral carbonation as thermodynamically stable carbonates. To obtain an atomic scale understanding of the weathering process and to design more reactive silicates for enhanced rock weathering, carbon dioxide adsorption on low Miller index wollastonite (CaSiO3) surfaces was modeled using density functional theory. Atomic scale structure of (100), (010), and (001) surfaces of wollastonite was predicted and the thermodynamics of their interaction with carbon dioxide was modeled. Based on surface energy calculations, (001) and (010) surfaces of wollastonite exhibit similar stabilities, while (100) surface is found to be least stable. Depending on the surface structure and chemistry, different carbon dioxide adsorption geometries are possible. A common trend emerges, wherein carbon dioxide adsorbs molecularly and demonstrates proclivity to bond with surface layer calcium and oxygen binding sites. Mechanisms for electronic charge transfer between the adsorbate and the substrate were studied to shed light on the fundamental aspects of these interactions. The most favorable bent dioxide geometry was bridged between calcium atoms, revealing that the enhancement of the likelihood of this geometry and binding site could pave the way to designing reactive silicates for efficient carbon dioxide sequestration via ERW.
Publisher Site: RIT Repository
PDF (Requires Sign-In): PDF
Click for Bibtex
@book{luan2022combating, title = {Combating Global Warming: Modeling Enhanced Rock Weathering of Wollastonite Using Density Functional Theory}, author = {Luan, Brian}, year = {2022}, school = {Rochester Institute of Technology} }
[T-2022-29] Maheen Riaz Contractor. (2022). “Improving the Flexibility of CLARA’s Automated Matching and Repair Processes.” Rochester Institute of Technology.
Abstract: More computer science researchers focus on automated program repair, with the world steadfastly moving towards automation. CLARA is an example of an automated program repair tool that provides feedback to novice programmers solving introductory programming assignments in Java, C++, and Python. CLARA involves test-based repair, requiring as input a correct program, an incorrect program, and its corresponding test case. Our work only focuses on Python. CLARA has two main limitations. The first involves lacking support for commonly used language constructs such as standard input, standard output, and import statements. We address this issue by extending CLARA's abstract syntax tree processor and interpreter to include these constructs. The second limitation is that CLARA requires both the correct and the incorrect program to have the same control flow. In a real-world setting, it is not easy to find such programs, reducing the true impact CLARA can have on the learning of novice programmers. Therefore, we implement a graph matching technique between the correct and incorrect programs that considers both the semantic and the topological information to help overcome this limitation. Using this matching, we modify the incorrect program to match its control flow with the correct program. To verify that our technique overcomes the control flow limitation, we conduct experiments to run CLARA and compare the number of programs repaired with and without the graph matching technique. We also analyze the percentage of the program modified by CLARA and the number of correct programs needed to repair all valid incorrect programs. Our experiments show that CLARA can parse, process, and repair many more programs after our extensions. Additionally, our experiments indicate that we never enable CLARA to replace all the source code of an incorrect program with all the source code of a correct program.
Publisher Site: RIT Repository
PDF (Requires Sign-In): PDF
Click for Bibtex
@book{contractor2022improving, title = {Improving the Flexibility of CLARA's Automated Matching and Repair Processes}, author = {Contractor, Maheen Riaz}, year = {2022}, school = {Rochester Institute of Technology} }
[T-2022-28] Benjamin Dow. (2022). “Alternative Participation: An exploratory study on participation in open source software beyond code contributions.” Rochester Institute of Technology.
Abstract: While it is commonly accepted that well-engineered commercial software projects rely on a variety of activities, this not the case with open source software development. A poorly understood area of open source software is what types of activities are present and being completed day-to-day. Understanding what activities exist would give developers and project leads additional attributes of the software engineering process to modify and improve. Identifying these activities is challenging as they are often abstract in nature and activities may not be formally defined within projects, but may still be executed; for example, the way in which a project accepts feedback may be defined or simply accepted through the issue tracker with no formal declaration. In this paper I investigate alternative participation activities in a variety of open source software projects. I found that a majority of these projects have alternative participation occurring but many struggle to formally define discrete activities or provide calls to action.
Publisher Site: RIT Repository
PDF (Requires Sign-In): PDF
Click for Bibtex
@book{dow2022alternative, title = {Alternative Participation: An exploratory study on participation in open source software beyond code contributions}, author = {Dow, Benjamin}, year = {2022}, school = {Rochester Institute of Technology} }
[T-2022-27] Stephen J. Cook. (2022). “Taxonomy of Software Readability Changes.” Rochester Institute of Technology.
Abstract: Software readability has emerged as an important software quality metric. Numerous pieces of research have highlighted the importance of readability. Developers generally spend a large amount of their time reading and understanding existing code, rather than writing new code. By creating more readable code, engineers can limit the mental load required to understand specific code segments. With this importance established, research has been done into how to improve software readability. This research looked for ways of measuring readability, how to create more readable software, and how to potentially improve readability. While some research has examined the changes developers make, their use of automatic source code analysis may miss some aspects of these changes. As such, this study conducted a manual review of software readability commits to identify what changes developers tend to make. In this study, we identified 1,782 potential readability commits for 800 open-source Java projects, by mining keyword patterns in commit messages. These commits were then reviewed by human reviewers to identify the changes made by the developers. The observations made by the reviewers were then reviewed for trends, from which several categories would be established. These categories would be further reviewed for additional trends, developing a taxonomy of readability changes. Overall, this research looked at 314 changes from 194 commits across 154 unique projects. This study shows the developers' actions when improving software readability, identifying the common trends of method extraction, identifier renaming, and code formatting, supported by existing research. In addition, this research presents less observed trends, such as code removal or keyword modification, which were changes not seen in other research. Overall, this work provides a taxonomy of the trends seen, identifying high level trends as well as subgroups within those trends.
Publisher Site: RIT Repository
PDF (Requires Sign-In): PDF
Click for Bibtex
@book{cook2022taxonomy, title = {Taxonomy of Software Readability Changes}, author = {Cook, Stephen J.}, year = {2022}, school = {Rochester Institute of Technology} }
[T-2022-26] Luis Angel Gutierrez Galaviz. (2022). “Why did you clone these identifiers? Using Grounded Theory to understand Identifier Clones.” Rochester Institute of Technology.
Abstract: Developers spend most of their time comprehending source code, with some studies estimating this activity takes between 58% to 70% of a developer's time. To improve the readability of source code, and therefore the productivity of developers, it is important to understand what aspects of static code analysis and syntactic code structure hinder the understandability of code. Identifiers are a main source of code comprehension due to their large volume and their role as implicit documentation of a developer's intent when writing code. Despite the critical role that identifiers play during program comprehension, there are no regulated naming standards for developers to follow when picking identifier names. Our research supports previous work aimed at understanding what makes a good identifier name, and practices to follow when picking names by exploring a phenomenon that occurs during identifier naming: identifier clones. Identifier clones are two or more identifiers that are declared using the same name. This is an important yet unexplored phenomenon in identifier naming where developers intentionally give the same name to two or more identifiers in separate parts of a system. We must study identifier clones to understand it's impact on program comprehension and to better understand the nature of identifier naming. To accomplish this, we conducted an empirical study on identifier clones detected in open-source software engineered systems and propose a taxonomy of identifier clones containing categories that can explain why they are introduced into systems and whether they represent naming antipatterns.
Publisher Site: RIT Repository
PDF (Requires Sign-In): PDF
Click for Bibtex
@book{gutierrez galaviz2022why, title = {Why did you clone these identifiers? Using Grounded Theory to understand Identifier Clones}, author = {Gutierrez Galaviz, Luis Angel}, year = {2022}, school = {Rochester Institute of Technology} }
[T-2022-25] James Dugan. (2022). “Leveraging Identifier Naming Structures in Source Code and Bug Reports to Localize Relevant Bugs.” Rochester Institute of Technology.
Abstract: When bugs are found in source code, bug reports are created which contain relevant information for developers to locate and fix the bug. In large source code repositories, it can be difficult and time consuming for developers to manually analyze bug reports to locate a bug. The discovery of patterns between bug reports and source files has led to the creation of automated tools using various techniques. Automated bug localization techniques can reduce the amount of manual effort required by developers by ranking the most probable location of the bug using textual information from bug reports and source code. Although these approaches offer some assistance, the lexical mismatch between the bug reports and the source code makes it difficult to accurately locate the buggy source code file(s) using Information Retrieval (IR) techniques. Our research proposes a technique that takes advantage of the lexical and structural patterns observed in source code identifier names to help offset the mismatch between bug reports and their related source code files. Our observations reveal that there are lexical and structural identifier naming trends for different identifier types in the source code. Using two open-source projects, and collecting frequencies for observed identifier patterns across the project, we applied the observed frequencies to matched word occurrences in bug reports across our evaluation data set to modify the significance of that word. Based on observations discovered in our empirical analysis of open source repositories ElasticSearch and RxJava, we developed a method to modify the significance of a word by altering the weight of the matched word represented in the Term Frequency - Inverse Document Frequency (TF-IDF) vectorization of that particular bug report. The idea behind this approach is that if we come across a word perceived to be significant based on our observed identifier pattern frequency data, we can apply a weight to that word in the bug report vectorization to increase the cosine similarity score between the bug report and source file vectors. This work expands and improves upon previous work by Gharibi et al. [1], who propose a multicomponent approach that uses token matching, stack trace, semantic similarity, and a revised vector space model (rVSM). Specifically, our approach modifies the rVSM component, and our work is evaluated on the same three open-source software projects: AspectJ, SWT, and ZXing. The results of our approach are comparable to the results of Gharibi et al., and we achieve an improvement in some cases. It was observed that our work outperforms many existing bug localization approaches. Top@N, Mean Reciprocal Rank (MRR), and Mean Average Precision (MAP) are metrics used to evaluate and rank our work against other approaches, revealing some improvement in bug localization across three open-source projects.
Publisher Site: RIT Repository
PDF (Requires Sign-In): PDF
Click for Bibtex
@book{dugan2022leveraging, title = {Leveraging Identifier Naming Structures in Source Code and Bug Reports to Localize Relevant Bugs}, author = {Dugan, James}, year = {2022}, school = {Rochester Institute of Technology} }
[T-2022-24] Sabrina Ly. (2022). “Quantum Solutions for Training a Single Layer Binary Neural Network.” Rochester Institute of Technology.
Abstract: Quantum computing is a relatively new field starting in the early 1980s when a physicist named Paul Benioff proposed a quantum mechanical model of the Turing machine, introducing quantum computers. Previously, the focus of most quantum computers was in the study of quantum applications instead of broad applications due to the fact that quantum technology is a newer field with many technology constraints, such as limited qubits and noisy environments. However, quantum computers are still capable of using quantum mechanics to solve specific algorithms with an exponential speed-up in comparison to their classical counterparts. One key algorithm is the HHL algorithm proposed by Harrow, Hassidim and Lloyd in 2009 [1]. This algorithm outlines a quantum approach to solve a linear systems of equations with a best case time complexity of O(poly(log N )), in comparison to the best case time complexity for classical algorithms of O(N^3 ). The HHL algorithm outlines a use for quantum circuits outside of quantum applications. One such application is in machine learning, as many networks use linear regression in their training algorithm. Currently it is not feasible to solve for weight vectors of floating point precision on a quantum computer, but if the weight vector is constrained to binary values 0 or 1 then the problem becomes small enough to implement even on current noisy quantum computers. This work outlines two different circuit designs to solve for 2 x 2 and 4 x 4 systems of equations, so long as the matrices follow the eigenvalue constraint of having eigenvalues be powers of 2. In addition, the problem of reading data from the quantum state to classical data is addressed through the use of a swap test between the solution state |x> and an test state |test>. By using a swap test vector of all 1s, it is shown it is possible to find how many ones lay in the solution vector; thus reducing the number of possible solution states without performing quantum tomography. While it is not possible to beat classical algorithms with the noise on current quantum circuits, this work shows it is possible to implement quantum algorithms for non-quantum applications establishing potential for future hybrid approaches.
Publisher Site: RIT Repository
PDF (Requires Sign-In): PDF
Click for Bibtex
@book{ly2022quantum, title = {Quantum Solutions for Training a Single Layer Binary Neural Network}, author = {Ly, Sabrina}, year = {2022}, school = {Rochester Institute of Technology} }
[T-2022-23] Devan Lad. (2022). “Testing of Neural Networks.” Rochester Institute of Technology.
Abstract: Research in Neural Networks is becoming more popular each year. Re- search has introduced different ways to utilize Neural Networks, but an important aspect is missing: Testing. There are only 16 papers that strictly address Testing Neural Networks with a majority of them focusing on Deep Neural Networks and a small part on Recurrent Neural Networks. Testing Re- current neural networks is just as important as testing Deep Neural Networks as they are used in products like Autonomous Vehicles. So there is a need to ensure that the recurrent neural networks are of high quality, reliable, and have the correct behavior. For the few existing research papers on the testing of recurrent neural networks, they only focused on LSTM or GRU recurrent neural network architectures, but more recurrent neural network architectures exist such as MGU, UGRNN, and Delta-RNN. This means we need to see if ex- isting test metrics works for these architectures or do we need to introduce new testing metrics. For this paper we have two objectives. First, we will do a comparative analysis of the 16 papers with research in Testing Neural Networks. We define the testing metrics and analyze the features such as code availability, programming languages, related testing software concepts, etc. We then perform a case study with the Neuron Coverage Test Metric. We will conduct an experiment using unoptimized RNN models trained by a tool within EXAMM, a RNN Framework and optimized RNN Models trained and optimized using ANTS. We compared the Neuron Coverage Outputs with the assumption that the Optimized Models will perform better.
Publisher Site: RIT Repository
PDF (Requires Sign-In): PDF
Click for Bibtex
@book{lad2022testing, title = {Testing of Neural Networks}, author = {Lad, Devan}, year = {2022}, school = {Rochester Institute of Technology} }
[T-2022-22] Matt Krol. (2022). “Low-Rank Clustering via LP1-PCA.” Rochester Institute of Technology.
Abstract: In recent years, subspace clustering has found many practical use cases which include, for example, image segmentation, motion segmentation, and facial clustering. The image and video data that is common to these types of applications often has high dimensionality. Rather than viewing high dimensionality as a drawback, we propose a novel algorithm for subspace clustering that takes advantage of the high dimensional nature of such data. We call this algorithm LP1-PCA Spectral Clustering. Specifically, we introduce a concept that we call cluster-ID sparsity, and we propose an algorithm called LP1-PCA to attain this in low data dimensions. Our novel LP1-PCA algorithm is simple to implement and typically converges after only a few iterations. Conditions for which our algorithm performs well are discussed both theoretically and empirically, and we show that our method often attains superior clustering performance when compared to other common clustering algorithms on synthetic and real world datasets.
Publisher Site: RIT Repository
PDF (Requires Sign-In): PDF
Click for Bibtex
@book{krol2022low-rank, title = {Low-Rank Clustering via LP1-PCA}, author = {Krol, Matt}, year = {2022}, school = {Rochester Institute of Technology} }
[T-2022-21] Sean Bonaventure. (2022). “High Error Rate Qubit Routing.” Rochester Institute of Technology.
Abstract: The current state of quantum computers is characterized by its limited resources and high noise levels. These are known as Noisy Intermediate Scale Quantum Computing (NISQ). Reduction of noise is addressed at the technology level, while noise mitigation strategies have been proposed through the compilation process of quantum circuits. The compilation process entails a number of steps that are computationally intensive and scale poorly as the problems grow in size and resource usage. This paper addresses the problems associated with noise by proposing a noise aware qubit routing algorithm. This algorithm attempts to improve accuracy of circuits by using SWAP gates attempt to avoid links with high error rates. This differs from existing algorithms which try to minimize the amount of SWAP gates used. In addition, multiple metrics are evaluated for Qiskit's routing algorithms. The proposed algorithm improves the accuracy against circuits compiled using Qiskit's basic routing algorithm, and against other more sophisticated routing algorithms, depending on the application circuit. Other metrics being considered, the routing algorithm also demonstrates to have minimal computational cost when compared to other approaches. Lastly, it is shown that different circuits benefit from different routing algorithms.
Publisher Site: RIT Repository
PDF (Requires Sign-In): PDF
Click for Bibtex
@book{bonaventure2022high, title = {High Error Rate Qubit Routing}, author = {Bonaventure, Sean}, year = {2022}, school = {Rochester Institute of Technology} }
[T-2022-20] Das Prangon. (2022). “Implementation and Evaluation of Deep Neural Networks in Commercially Available Processing in Memory Hardware.” Rochester Institute of Technology.
Abstract: Deep Neural Networks (DNN), specifically Convolutional Neural Networks (CNNs) are often associated with a large number of data-parallel computations. Therefore, data-centric computing paradigms, such as Processing in Memory (PIM), are being widely explored for CNN acceleration applications. A recent PIM architecture, developed and commercialized by the UPMEM company, has demonstrated impressive performance boost over traditional CPU-based systems for a wide range of data parallel applications. However, the application domain of CNN acceleration is yet to be explored on this PIM platform. In this work, successful implementations of CNNs on the UPMEM PIM system are presented. Furthermore, multiple operation mapping schemes with different optimization goals are explored. Based on the data achieved from the physical implementation of the CNNs on the UPMEM system, key-takeaways for future implementations and further UPMEM improvements are presented. Finally, to compare UPMEM's performance with other PIMs, a model is proposed that is capable of producing estimated performance results of PIMs given architectural parameters. The creation and usage of the model is covered in this work.
Publisher Site: RIT Repository
PDF (Requires Sign-In): PDF
Click for Bibtex
@book{prangon2022implementation, title = {Implementation and Evaluation of Deep Neural Networks in Commercially Available Processing in Memory Hardware}, author = {Prangon, Das}, year = {2022}, school = {Rochester Institute of Technology} }
[T-2022-19] Isabella G. Cox. (2022). “Using GMOS Spectroscopy to Study Star Formation and AGN in the CANDELS COSMOS and UDS Fields.” Rochester Institute of Technology.
Abstract: Spectroscopic measurements of galaxies can help us to better understand their properties. Spectroscopy allows for the measurement of spectroscopic redshifts, which have better precision than redshifts derived from photometry. Precise redshifts are needed to eliminate distance un- certainties when deriving distance-dependent properties of galaxies. Additionally, spectroscopy provides insight into the physical state and processes within a galaxy, such as its rate of on- going star formation, its chemical abundance, and the properties of the interstellar medium within the galaxy. Spectroscopy is particularly powerful when coupled with broad, multiwavelength photometric data. The five extragalactic fields from the Cosmic Assembly Near-infrared Deep Extragalactic Legacy Survey (CANDELS) have excellent multiwavelength photometric coverage. Two of the fields however, were lacking in spectroscopic coverage. To rectify this, spectroscopic observations were taken for sources in those particular fields in 2014, 2016, and 2019 with the Gemini Multi-Object Spectrometer on the twin Gemini Telescopes. We reduced the spectroscopic observations, which were taken in a configuration not compatible with existing reduction pipelines. We were able to extract spectra for 196 sources and measured their spectroscopic redshifts. We also measured emission fluxes from the spectra. We used the Halpha and [OII]3727 emission lines as star formation rate indicators to measure the star formation rates of our galaxies, and compared these results to star formation rates obtained from other techniques. We also used Hbeta, [OIII]5007, Halpha, and [NII]6583 to construct diagnostics to detect AGN in our sample, and compared those classifications to sources in our sample detected as an AGN with other methods (e.g., mid-infrared colors, X-ray flux). Using the spectroscopic data, we identified seven possible AGN sources not identified with X-ray or infrared data that could be followed up.
Publisher Site: RIT Repository
PDF (Requires Sign-In): PDF
Click for Bibtex
@book{cox2022using, title = {Using GMOS Spectroscopy to Study Star Formation and AGN in the CANDELS COSMOS and UDS Fields}, author = {Cox, Isabella G.}, year = {2022}, school = {Rochester Institute of Technology} }
[T-2022-18] Joseph Zonghi. (2022). “Towards Real-Time Classification of Human Imagined Language.” Rochester Institute of Technology.
Abstract: The primary goal of this research was to develop a system capable of predicting a given user's imagined language in real time using their brainwave data. This research analyzed both FPGA-based and software based approaches to real-time classification of imagined language. The classification was binary between English and Japanese, and a dataset containing imagined speech from both languages was also created. Another goal of this research was to consider the effects of quantization of the network weights in order to examine the resulting utilization of the FPGA to allow for other applications to run in conjunction with our proposed system. With test accuracies over 95% but real-time accuracies only barely approaching 60%, it can be considered partly successful. Real-time approaches to predicting imagined EEG words are rare, and attempts at predicting imagined language are even rarer. Such a system could be beneficial in helping multi-lingual environments that standard natural language processing systems have difficulty in noticing changes in language, especially those that occur in real time. Further iterations on this proposed system could also assist those who have difficulty articulating speech and would benefit from having a brainwave-based system that is portable and works in real-time. It is hopeful that this work can lead to future iterations and advancements in the realm of real-time imagined speech classification both through their own attempts or perhaps with the help of the English/Japanese imagined speech dataset created through this research.
Publisher Site: RIT Repository
PDF (Requires Sign-In): PDF
Click for Bibtex
@book{zonghi2022towards, title = {Towards Real-Time Classification of Human Imagined Language}, author = {Zonghi, Joseph}, year = {2022}, school = {Rochester Institute of Technology} }
[T-2022-17] Christie Agbalessi. (2022). “Design of an 8-bit Configurable CNN Hardware Accelerator for Audio and Image Classification.” Rochester Institute of Technology.
Abstract: Picture yourself resting or attending a meeting in the back seat while your car is driving you home with all the privacy a driverless vehicle offers. Designing autonomous systems involves decoding environmental cues and making safe decisions. Convolution Neural Networks (CNNs) are the leading choices for computer vision tasks due to their high performance and scalability to pieces of hardware. They have long been run on Graphical Processing Units (GPUs) and Central Processing Units (CPUs). Yet, today, there is an urgent need to accelerate CNNs in low-power consumption hardware for real-time inference. This research aims to design a configurable hardware accelerator for 8-bit fixed point audio and image CNN models. An audio network is developed to classify environmental sounds from children playing in the streets, car horns, and sirens; an image network is designed to classify cars, lanes, road signs, traffic lights, and pedestrians. The two CNNs are quantized from a 32-bit floating-point to an 8-bit fixed-point format while maintaining high accuracy. The hardware accelerator is verified in SystemVerilog and compared to similar works.
Publisher Site: RIT Repository
PDF (Requires Sign-In): PDF
Click for Bibtex
@book{agbalessi2022design, title = {Design of an 8-bit Configurable CNN Hardware Accelerator for Audio and Image Classification}, author = {Agbalessi, Christie}, year = {2022}, school = {Rochester Institute of Technology} }
[T-2022-16] Matthew Gould. (2022). “Advanced Lease Caching.” Rochester Institute of Technology.
Abstract: Since the dawn of computing, CPU performance has continually grown, buoyed by Moore's Law. Execution speed for parallelizable programs in particular has massively increased with the now widespread employment of GPUs, TPUs, and FPGAs, capable of preforming hundreds of computations simultaneously, for data processing. A major bottleneck for further performance increases, which has impeded speedup of sequential programming in particular, is the processor memory performance gap. One of the approaches to address this block is improving cache management algorithms. Caching is transparent to software, but traditional caching algorithms forgo hardware-software collaboration. Previous work introduced the idea of assigning leases to cache blocks as a form of collaborative cache eviction policy and introduced two lease-caching algorithms, Compiler Lease of cAche Memory (CLAM) and Phased Reference Leasing (PRL), evaluating them over 7 benchmarks from the Polybench benchmark suite. This work evaluates CLAM and PRL over all thirty benchmarks of the Polybench suite for multiple dataset sizes. Additionally, to address the flaws CLAM and PRL, two new lease-caching algorithms have been developed: Scoped Hooked Eviction Lease (SHEL) and Cross-Scope Eviction Lease (C-SHEL). These algorithms are evaluated not just for a single-level cache, typically found in embedded systems, but also for a multi-level cache as exists in more high-performance systems including multi-core CPUs. The test system uses a RISCV architecture to run benchmarks. All four lease caching algorithms outperform the baseline Pseudo Least Recently Used (PLRU) policy at both levels of the cache hierarchy. Further, SHEL and C-SHEL display significant performance increases over PRL for certain benchmarks, demonstrating the value of scoped leasing in addressing complex reuse interval (RI) behavior.
Publisher Site: RIT Repository
PDF (Requires Sign-In): PDF
Click for Bibtex
@book{gould2022advanced, title = {Advanced Lease Caching}, author = {Gould, Matthew}, year = {2022}, school = {Rochester Institute of Technology} }
[T-2022-15] Vedgopal Balasubramanian Govindhram. (2022). “Density functional theory study of oxygen vacancy formation and doping on wollastonite surfaces.” Rochester Institute of Technology.
Abstract: In recent times, the rising amount of carbon dioxide (CO2) in the atmosphere have caused a concerning increase in global warming. To combat global warming, several negative emissions technologies have been employed. One such technology is Enhanced Rock Weathering (ERW), wherein CO2 is trapped as thermodynamically stable carbonates in silicate minerals that are exposed to the atmosphere. This process happens via mineral carbonation. Wollastonite (CaSiO3) is one such silicate that has applications in ERW. Our study is focused on utilizing density functional theory-based calculations to investigate the thermodynamic stability of oxygen vacancies on the surfaces of wollastonite mineral. Formation of oxygen vacancy naturally requires large amounts of energy. Oxygen vacancy formation is more favorable on the surface rather than in the bulk of the wollastonite mineral. We further studied if adding dopants (aluminum dopants) on the surface of the mineral is thermodynamically favorable and evaluated their overall impact on oxygen vacancy formation. Formation of oxygen vacancies on the wollastonite surfaces is found to be an endothermic process, whereas addition of aluminum dopants makes it an exothermic process. This fundamental knowledge will be instrumental in enhancing the reactivity of wollastonite minerals for effective sequestration of carbon dioxide using ERW.
Publisher Site: RIT Repository
PDF (Requires Sign-In): PDF
Click for Bibtex
@book{govindhram2022density, title = {Density functional theory study of oxygen vacancy formation and doping on wollastonite surfaces}, author = {Govindhram, Vedgopal Balasubramanian}, year = {2022}, school = {Rochester Institute of Technology} }
[T-2022-14] Andrew H. Meyer. (2022). “InfoMixup : An Intuitive and Information-Driven Approach to Robust Generalization.” Rochester Institute of Technology.
Abstract: The discovery of Adversarial Examples — data points which are easily recognized by humans, but which fool artificial classifiers with ease, is relatively new in the world of machine learning. Corruptions imperceptible to the human eye are often sufficient to fool state of the art classifiers. The resolution of this problem has been the subject of a great deal of research in recent years as the prevalence of Deep Neural Networks grows in everyday systems. To this end, we propose InfoMixup , a novel method to improve the robustness of Deep Neural Networks without significantly affecting performance on clean samples. Our work is focused in the domain of image classification, a popular target in contemporary literature due to the proliferation of Deep Neural Networks in modern products. We show that our method achieves state of the art improvements in robustness against a variety of attacks under several measures.
Publisher Site: RIT Repository
PDF (Requires Sign-In): PDF
Click for Bibtex
@book{meyer2022infomixup, title = {InfoMixup : An Intuitive and Information-Driven Approach to Robust Generalization}, author = {Meyer, Andrew H.}, year = {2022}, school = {Rochester Institute of Technology} }
[T-2022-13] Lucy Zimmerman. (2022). “Comparison of Methane Plume Detection Using Shortwave and Longwave Infrared Hyperspectral Sensors Under Varying Environmental Conditions.” Rochester Institute of Technology.
Abstract: Methane is a prevalent greenhouse gas with potent heat trapping capabilities, but methane emissions can be difficult to detect and track. Hyperspectral imagery is an effective method of detection which can locate methane emission sources in order to mitigate leaks, as well as provide accountability for reaching emissions reduction goals. Because of methane's absorption features in the infrared, both shortwave infrared (SWIR) and longwave infrared (LWIR) hyperspectral sensors have been used to accurately detect methane plumes. However, surface, environmental and atmospheric background conditions can cause methane detectability to vary. This study compared methane detectability under varying environmental conditions for two airborne hyperspectral sensors: AVIRIS-NG in the SWIR and HyTES in the LWIR. For this trade study, we modeled methane plume detection under a wide variety of precisely known conditions by making use of synthetic images which were comprised of MODTRAN-generated radiance curves. We applied a matched filter to these images to assess detection accuracy, and used these results to identify the conditions which have the greatest impact on detectability in the SWIR and LWIR: surface reflectance, surface temperature, and water vapor concentration. We then computed the specific boundaries on these conditions which make methane most detectable for each instrument. The results of this trade study can help inform decision making about which sensors are most useful for various types of methane emission analysis, such as leak detection, plume mapping, and emissions rate quantification.
Publisher Site: RIT Repository
PDF (Requires Sign-In): PDF
Click for Bibtex
@book{zimmerman2022comparison, title = {Comparison of Methane Plume Detection Using Shortwave and Longwave Infrared Hyperspectral Sensors Under Varying Environmental Conditions}, author = {Zimmerman, Lucy}, year = {2022}, school = {Rochester Institute of Technology} }
[T-2022-12] Thomas Mountford. (2022). “Address Obfuscation to Protect Against Hardware Trojans in Network-on-Chips.” Rochester Institute of Technology.
Abstract: Interconnection networks for multi/many-core processors or server systems are the backbone of the system as they enable data communication among the processing cores, caches, memory, and other peripherals. Given the criticality of the interconnects, the system can be severely subverted if the interconnection is compromised. The threat of Hardware Trojans (HTs) penetrating complex hardware systems such as multi/many-core processors are increasing due to the increasing presence of third-party players in a System-on-chip (SoC) design. Even by deploying native HTs, an adversary can exploit the Network-on-Chip (NoC) backbone of the processor and get access to communication patterns in the system. This information, if leaked to an attacker, can reveal important insights regarding the application suites running on the system; thereby compromising the user privacy and paving the way for more severe attacks on the entire system. In this paper, we demonstrate that one or more HTs embedded in the NoC of a multi/many-core processor is capable of leaking sensitive information regarding traffic patterns to an external malicious attacker, who, in turn, can analyze the HT payload data with machine learning techniques to infer the applications running on the processor. Furthermore, to protect against such attacks, we propose a LUT based obfuscation method. The proposed defense can obfuscate the attacker's data processing capabilities to infer the user profiles successfully. Our experimental results demonstrate that the proposed obfuscation could reduce the accuracy of identifying user profiles by the attacker from >99% to
Publisher Site: RIT Repository
PDF (Requires Sign-In): PDF
Click for Bibtex
@book{mountford2022address, title = {Address Obfuscation to Protect Against Hardware Trojans in Network-on-Chips}, author = {Mountford, Thomas}, year = {2022}, school = {Rochester Institute of Technology} }
[T-2022-11] Benjamin J. Haag. (2022). “Device-Free Sensing and Modeling of Wireless Channels in Dynamic Indoor Environments.” Rochester Institute of Technology.
Abstract: Sensing human activity and/or location using wireless channel received signal strength, aka channel response, has been extensively investigated for a variety of applications. To this end, modeling of wireless channels in dynamic indoor environments typically relies on stochastic channel distributions with constant distribution parameters coupled with site-specific calibration. The Rician distribution with a constant Rician factor is a prominent example. In this paper, we propose and investigate a calibration-less stochastic channel model based on the Rician distribution with a time varying Rician factor, where the variation in time is set to reflect specific dynamic scenarios. We apply this model to experimental data captured from several typical indoor scenarios such as walking, jumping, and entering/exiting a room. We show the proposed model provides a high level of predictability when simulating the channel response compared to experimental data. We also demonstrate how the model can be used to explain captured channel response data in such dynamic indoor environments. The proposed model and modeling approach can be used to test existing methods of location and activity sensing as well as support the development of new methods.
Publisher Site: RIT Repository
PDF (Requires Sign-In): PDF
Click for Bibtex
@book{haag2022device-free, title = {Device-Free Sensing and Modeling of Wireless Channels in Dynamic Indoor Environments}, author = {Haag, Benjamin J.}, year = {2022}, school = {Rochester Institute of Technology} }
[T-2022-10] Peter Fabinski. (2022). “Side-Channel Attacks and Countermeasures for the MK-3 Authenticated Encryption Scheme.” Rochester Institute of Technology.
Abstract: In the field of cryptography, the focus is often placed on security in a mathematical or information-theoretic sense; for example, cipher security is typically evaluated by the difficulty of deducing the plaintext from the ciphertext without knowledge of the key. However, once these cryptographic schemes are implemented in electronic devices, another class of attack presents itself. Side-channel attacks take advantage of the side effects of performing a computation, such as power consumption or electromagnetic emissions, to extract information outside of normal means. In particular, these side-channels can reveal parts of the internal state of a computation. This is important because intermediate values occurring during computation are typically considered implementation details, invisible to a potential attacker. If this information is revealed, then the assumptions of a non-side-channel-aware security analysis based only on inputs and outputs will no longer hold, potentially enabling an attack. This work tests the effectiveness of power-based side-channel attacks against MK-3, a customizable authenticated encryption scheme developed in a collaboration between RIT and L3Harris Technologies. Using an FPGA platform, Correlation Power Analysis (CPA) is performed on several different implementations of the algorithm to evaluate their resistance to power side-channel attacks. This method does not allow the key to be recovered directly; instead, an equivalent 512-bit intermediate state value is targeted. By applying two sequential stages of analysis, a total of between 216 and 322 bits are recovered, dependent on customization parameters. If a 128-bit key is used, then this technique has no benefit to an attacker over brute-forcing the key itself; however, in the case of a 256-bit key, CPA may provide up to a 66-bit advantage. In order to completely defend MK-3 against this type of attack, several potential countermeasures are discussed at the implementation, design, and overall system levels.
Publisher Site: RIT Repository
PDF (Requires Sign-In): PDF
Click for Bibtex
@book{fabinski2022side-channel, title = {Side-Channel Attacks and Countermeasures for the MK-3 Authenticated Encryption Scheme}, author = {Fabinski, Peter}, year = {2022}, school = {Rochester Institute of Technology} }
[T-2022-09] Jacob Kiggins. (2022). “Development of a Bio-Inspired Computational Astrocyte Model for Spiking Neural Networks.” Rochester Institute of Technology.
Abstract: The mammalian brain is the most capable and complex computing entity known today. For many years there has been research focused on reproducing the brain's processing capabilities. An early example of this endeavor was the perceptron which has become the core building block of neural network models in the deep learning era. Deep learning has had tremendous success in well-defined tasks like object detection, games like go and chess, and automatic speech recognition. In fact, some deep learning models can match and even outperform humans in specific situations. However, in general, they require much more training, have higher power consumption, are more susceptible to noise and adversarial perturbations, and have very different behavior than their biological counterparts. In contrast, spiking neural network models take a step closer to biology, and in some cases behave identically to measurements of real neurons. Though there has been advancement, spiking neural networks are far from reaching their full potential, in part because the full picture of their biological underpinnings is unclear. This work attempts to reduce that gap further by exploring a bio-inspired configuration of spiking neurons coupled with a computational astrocyte model. Astrocytes, initially thought to be passive support cells in the brain are now known to actively participate in neural processing. They are believed to be critical for some processes, such as neural synchronization, self-repair, and learning. The developed astrocyte model is geared towards synaptic plasticity and is shown to improve upon existing local learning rules, as well as create a generalized approach to local spike-timing-dependent plasticity. Beyond generalizing existing learning approaches, the astrocyte is able to leverage temporal and spatial integration to improve convergence, and tolerance to noise. The astrocyte model is expanded to influence multiple synapses and configured for a specific learning task. A single astrocyte paired with a single leaky integrate and fire neuron is shown to converge on a solution in 2, 3, and 4 synapse configurations. Beyond the more concrete improvements in plasticity, this work provides a foundation for exploring supervisory astrocyte-like elements in spiking neural networks, and a framework to implement and extend many three-factor learning rules. Overall, this work brings the field a bit closer to leveraging some of the distinct advantages of biological neural networks.
Publisher Site: RIT Repository
PDF (Requires Sign-In): PDF
Click for Bibtex
@book{kiggins2022development, title = {Development of a Bio-Inspired Computational Astrocyte Model for Spiking Neural Networks}, author = {Kiggins, Jacob}, year = {2022}, school = {Rochester Institute of Technology} }
[T-2022-08] Madhusudan Rajendran. (2022). “Functional evolutionary adaptation of binding dynamics in the SARS-CoV-2/ACE2 interface from bats to humans.” Rochester Institute of Technology.
Abstract: The COVID-19 pandemic highlights the substantial public health, economic, and societal consequences of virus spillover from a wildlife reservoir. Widespread human transmission of severe acute respiratory syndrome coronavirus 2 (SARS-CoV-2) also presents a new set of challenges when considering viral spillover from people to naïve wildlife and other animal populations. Here, we used molecular dynamic (MD) simulations to understand the transmission of the SARS-CoV-2 virus from bats to humans and investigate the evolution of the various human variants. More specifically, we used MD simulations to understand the atomic fluctuation dampening at the receptor-binding domain (RBD)/ angiotensin-converting enzyme 2 (ACE2) interface when the RBD of the different SARS-CoV-2 variants is simulated with ACE2 of bat origin (bACE2) or human origin (hACE2). Towards that end, we found that the RaTG13 RBD is more stabilized with strong atomic fluctuation dampening when bound to bACE2 than hACE2. Interestingly, in terms of the variants being monitored (VBM - beta, kappa, and epsilon variants), we saw very similar binding dynamics and MD profiles between bACE2 and hACE2. Of note, when either bACE2 or hACE2 was simulated with variants of concern (VOC – alpha, delta, and omicron variants) RBD, we saw slightly stabilized atomic fluctuation dampening when the RBD was simulated with hACE2. Our results indicated that the RBD of the newer human SARS-CoV-2 variants does not differ significantly in the atomic fluctuation dampening when interacting with ACE2 of either bat or human origin. As a result, reverse zoonosis events that cause the virus to jump back to bats are highly possible, including the emergence of new and different variants. Therefore, in addition to the ongoing genomic surveillance, we also advise the inclusion of MD simulation surveillance that investigates the binding dynamics of the new variants with the receptors of the different host species.
Publisher Site: RIT Repository
PDF (Requires Sign-In): PDF
Click for Bibtex
@book{rajendran2022functional, title = {Functional evolutionary adaptation of binding dynamics in the SARS-CoV-2/ACE2 interface from bats to humans}, author = {Rajendran, Madhusudan}, year = {2022}, school = {Rochester Institute of Technology} }
[T-2022-07] Eleni Gogos. (2022). “Problematic Social Media Use in the Context of Romantic Relationships: Relation to Attachment, Emotion Regulation, and Motivations for Use.” Rochester Institute of Technology.
Abstract: Social media is an increasingly popular form of connecting with others, especially among young adults, but problematic social media use (PSMU) has become a growing concern. Research has shown that people with anxious attachment styles and poor emotion regulation have a greater likelihood of having PSMU (Liu & Ma, 2019), but how social media usage might play a role in these relationships has not been well-studied. This research asked if the association between anxious attachment and PSMU will be affected by both emotion regulation and online social surveillance in romantic relationships as mediating influences. We utilized advanced mobile phone features to gather screen time data to measure as a covariate. Young adult participants who were in a romantic relationship and were users of social media (N=158) completed online questionnaires regarding relationship behavior (attachment style, online social surveillance), emotion regulation, and social media use. A subset of the sample also provided detailed screen time data (n=76). Results demonstrated that both emotion regulation difficulties and social surveillance were significantly, positively associated with PSMU, and also were significantly, positively associated with anxious attachment. In contrast to previous work, however, anxious attachment was not directly associated with PSMU. Screen time measures revealed that Facebook has been replaced by newer platforms like Snapchat, Instagram, and TikTok in young adults' media preferences. Future research should examine the differences among social platforms and their uses.
Publisher Site: RIT Repository
PDF (Requires Sign-In): PDF
Click for Bibtex
@book{gogos2022problematic, title = {Problematic Social Media Use in the Context of Romantic Relationships: Relation to Attachment, Emotion Regulation, and Motivations for Use}, author = {Gogos, Eleni}, year = {2022}, school = {Rochester Institute of Technology} }
[T-2022-06] Alexander Avery. (2022). “DeepRM: Deep Recurrent Matching for 6D Pose Refinement.” Rochester Institute of Technology.
Abstract: Precise 6D pose estimation of rigid objects from RGB images is a critical but challenging task in robotics and augmented reality. To address this problem, we propose DeepRM, a novel recurrent network architecture for 6D pose refinement. DeepRM leverages initial coarse pose estimates to render synthetic images of target objects. The rendered images are then matched with the observed images to predict a rigid transform for updating the previous pose estimate. This process is repeated to incrementally refine the estimate at each iteration. LSTM units are used to propagate information through each refinement step, significantly improving overall performance. In contrast to many 2-stage Perspective-n-Point based solutions, DeepRM is trained end-to-end, and uses a scalable backbone that can be tuned via a single parameter for accuracy and efficiency. During training, a multi-scale optical flow head is added to predict the optical flow between the observed and synthetic images. Optical flow prediction stabilizes the training process, and enforces the learning of features that are relevant to the task of pose estimation. Our results demonstrate that DeepRM achieves state-of-the-art performance on two widely accepted challenging datasets.
Publisher Site: RIT Repository
PDF (Requires Sign-In): PDF
Click for Bibtex
@book{avery2022deeprm:, title = {DeepRM: Deep Recurrent Matching for 6D Pose Refinement}, author = {Avery, Alexander}, year = {2022}, school = {Rochester Institute of Technology} }
[T-2022-05] Mariel V. Pridmore. (2022). “Identification of Essential Gene Relations For Lung Adenocarcinoma Therapy Targets by Cross-Referencing Literature-Mined and RNASeq Data.” Rochester Institute of Technology.
Abstract: Lung adenocarcinoma is the most frequently diagnosed subtype of lung cancer, and while the disease itself can be strenuous, the typical treatments such as chemotherapy are also known to cause whole body harm to the patient due to its systemic nature. Target therapies are increasingly gaining popularity for their ability to selectively apply the therapy to a specific cell. In terms of cancer, this method would lessen some, if not all the painful side-effects of chemotherapy and enhance a patient's quality of life. Narrowing down what genes are deemed to be essential to the operation of the cell can help determine which gene-gene interactions would make prime candidates for further target therapy research. This project aimed to develop a cross referencing method using literature-minded and RNASeq data in order to identify essential gene-gene relations unique to lung adenocarcinoma cell lines. In doing so, two gene-gene relations with significant changes in gene expression values across all cell lines under chemotherapeutic conditions were successfully discovered. These relations can be used in future research to study as potential treatment targets to include alongside chemotherapy with the possibility to alleviate the severe side effects that the patients currently undergo with standard treatment.
Publisher Site: RIT Repository
PDF (Requires Sign-In): PDF
Click for Bibtex
@book{pridmore2022identification, title = {Identification of Essential Gene Relations For Lung Adenocarcinoma Therapy Targets by Cross-Referencing Literature-Mined and RNASeq Data}, author = {Pridmore, Mariel V.}, year = {2022}, school = {Rochester Institute of Technology} }
[T-2022-04] Aaron Pennington. (2022). “Bioinformatics Analyses of New Genes of Focus in the Research of Autism with Dogs, Rats, and a Variety of Other Model Organisms.” Rochester Institute of Technology.
Abstract: Autism is a mental disorder in which multiple genes are involved in the development of its various symptoms. However, as a result of the challenges inherent to identifying the responsible genes, many studies are ongoing and inconclusive. 1 To date, studies have shown that two genes, BAG3 and PALLD, were upregulated in autistic individuals. 2–6 BAG3 encodes BAG cochaperone 3 32; while PALLD encodes Palladin, Cytoskeletal Associated Protein 33. This project analyzed the similarities and differences between the human BAG3 and PALLD genes and those in various model organisms. While it is likely that epigenetic modifications affect the expression and activity of these genes, they were not a focus of this work due to the paucity of prior research. 7–11 It is hypothesized that there is a model organism best suited for studying BAG3 and/or PALLD for a better understanding of its role in human autism. Comparisons were performed using a variety of bioinformatics tools in order to identify mRNA variants in model organisms. The most similar variants of BAG3 and PALLD were then assessed for the significance of these variations on the predicted structure and expression of the encoded protein. Finally, the same variants' regulatory regions were predicted and compared in order to identify similarities and differences found upstream of the BAG3 and PALLD genes. The findings of this study suggest that the BAG3 and PALLD protein structures from the model organisms dog and rat are sufficiently similar compared to those in humans so they can be used to better understand the genes in humans diagnosed with autism. Furthermore, there are similarities in the regulatory regions predicted for select model organisms. However, these regulatory regions are in areas where there is very little known and therefore where future research into these genes' effect on autism should be focused. The results from this work provide guidance as well as evidence justifying the need for future research and experimental manipulation of BAG3 and PALLD in these model organisms.
Publisher Site: RIT Repository
PDF (Requires Sign-In): PDF
Click for Bibtex
@book{pennington2022bioinformatics, title = {Bioinformatics Analyses of New Genes of Focus in the Research of Autism with Dogs, Rats, and a Variety of Other Model Organisms}, author = {Pennington, Aaron}, year = {2022}, school = {Rochester Institute of Technology} }
[T-2022-03] Karl Daningburg. (2022). “Acquisition Strategies for Gravitational Wave Surrogate Modeling.” Rochester Institute of Technology.
Abstract: Gravitational wave science is dependent upon expensive numerical simulations, which provide the foundational understanding of binary merger radiation needed to interpret observations of massive binary black holes. The high cost of these simulations limits large-scale campaigns to explore the binary black hole parameter space. Surrogate models have been developed to efficiently interpolate between simulation results, but these models require a sufficiently comprehensive sample to train on. Acquisition functions can be used to identify points in the domain for simulation. We develop a new acquisition function which accounts for the cost of simulating new points. We show that when applied to a 3D domain of binary mass ratio and dimensionless spins, the accumulated cost of simulation is reduced by a factor of about 10.
Publisher Site: RIT Repository
PDF (Requires Sign-In): PDF
Click for Bibtex
@book{daningburg2022acquisition, title = {Acquisition Strategies for Gravitational Wave Surrogate Modeling}, author = {Daningburg, Karl}, year = {2022}, school = {Rochester Institute of Technology} }
[T-2022-02] Kyle G. Tezanos. (2022). “Optical Properties of MacEtch-Fabricated Porous Silicon Nanowire Arrays.” Rochester Institute of Technology.
Abstract: The increasing demand for complex devices that utilize unique, three-dimensional nanostructures has spurred the development of controllable and versatile semiconductor fabrication techniques. However, there exists a need to refine such methodologies to overcome existing processing constraints that compromise device performance and evolution. Conventional wet etching techniques (e.g., crystallographic KOH etching of Si) successfully generate textured Si structures with smooth sidewalls but lack the capabilities of controllably producing high aspectratio structures. Alternatively, dry etching techniques (e.g., reactive-ion etching), while highly controllable and capable of generating vertically aligned, high aspect-ratio structures for IC technologies, introduce considerable sidewall and lattice damage as a result of high-energy ion bombardment that may compromise device performance. Metal-assisted chemical etching (MacEtch) provides an alternative process that is capable of anisotropically generating high aspect-ratio micro and nanostructures using a room temperature, solution-based technique. This fabrication process employs an appropriate metal catalyst (e.g., Au, Ag, Pt, Pd) to induce etching in several semiconducting materials (e.g., Si, GaAs) submerged in a solution containing an oxidant and an etchant. The MacEtch process resembles a galvanic cell such that cathodic and anodic half reactions take place at the catalyst/solution interface and catalyst/substrate interface, respectively. At the cathode, the metal catalyzes the reduction of the oxidant resulting in the generation and accumulation of charge carriers (e.g., holes, h+) that are subsequently injected into the underlying substrate at the anode. This results in the formation of oxide species that are preferentially dissolved by the etchant. Thus, MacEtch provides a tunable, top-down, catalytic fabrication technique enabling greater process control and versatility for generating high aspect-ratio semiconductor structures. In this thesis, Au and Au/Pd catalyzed MacEtch is used to generate ultradeep Si micropillar structures, and porous SiNW (p-SiNW) arrays with enhanced optical properties. Using a combination of Au-MacEtch and a crystallographic KOH etch, Si micropillars with ~100 μm height were fabricated with up to 70 μm clearance between pillars to allow efficient fluid flow for optical detection of viral particles. Alternatively, porous SiNW arrays fabricated via AuPd- MacEtch demonstrated broadband absorption ≥ 90% from 200 – 900 nm and were shown to outperform RCWA-simulated SiNW arrays with similar morphologies. Additionally, photoluminescence (PL) spectra collected from as prepared p-SiNW showed significant enhancement in intensity centered near 650 nm as etch depth increased from 30 μm to 100 μm, attributed to an increase in the porous volume. Using atomic layer deposition (ALD) the p-SiNW were passivated using alumina (Al2O3) and hafnia (HfO2) thin films in addition to ITO thin films deposited via sputtering. PL intensity also increased after ALD passivation, attributed to a quenching effect on non-radiative SRH recombination sites on the NW surfaces, with a red shift in the peak wavelength as ALD film thickness increased from 10 nm to 50 nm, resulting from strain effects acting on the NW themselves. These results show promise in such micropillar and coated and uncoated p-SiNW structures towards applications in microfluidic devices, and indoor light-harvesting and outdoor solar-based technologies.
Publisher Site: RIT Repository
PDF (Requires Sign-In): PDF
Click for Bibtex
@book{tezanos2022optical, title = {Optical Properties of MacEtch-Fabricated Porous Silicon Nanowire Arrays}, author = {Tezanos, Kyle G.}, year = {2022}, school = {Rochester Institute of Technology} }
[T-2022-01] Jason Ziskind. (2022). “Measuring Entanglement using Programmable Holograms.” Rochester Institute of Technology.
Abstract: The Einstein–Podolsky–Rosen (EPR) paradox proposes an entangled quantum state in high dimensional non-commuting observables, position and momentum. We experimentally demonstrate a novel method for measuring spatial correlations in joint position and joint momentum space for entangled photons in an EPR-like state. Research in the field of quantum optics can provide insight into quantum information processing, communication, quantum key distribution, and further investigation into the EPR paradox and locality. Unlike existing techniques, we take measurements of non-commuting observables using a static configuration. A 405nm pump laser incident on a Bismuth Borate nonlinear crystal produces an EPR state as a pair of 810nm photons through the process of spontaneous parametric downconversion. To measure spatial correlations, we take advantage of holograms displayed on digital micromirror devices (DMDs). This method allows for control over the basis that is measured only by changing what hologram is displayed on the DMD, without having to add lenses or other bulk optic components. The field interaction that generates a hologram can be computationally simulated and displayed on the DMD allowing for a momentum mode projection onto the incident state. Collection of joint position and joint momentum correlations provide an entanglement witness. Verification of entanglement using this technique provides the framework to investigate projections onto arbitrary states and explore further quantum communication advances.
Publisher Site: RIT Repository
PDF (Requires Sign-In): PDF
Click for Bibtex
@book{ziskind2022measuring, title = {Measuring Entanglement using Programmable Holograms}, author = {Ziskind, Jason}, year = {2022}, school = {Rochester Institute of Technology} }
[T-2021-58] Tommy Li. (2021). “Model Extraction and Adversarial Attacks on Neural Networks Using Side-Channel Information.” Rochester Institute of Technology.
Abstract: Artificial neural networks (ANNs) have gained significant popularity in the last decade for solving narrow AI problems in domains such as healthcare, transportation, and defense. As ANNs become more ubiquitous, it is imperative to understand their associated safety, security, and privacy vulnerabilities. Recently, it has been shown that ANNs are susceptible to a number of adversarial evasion attacks - inputs that cause the ANN to make high-confidence misclassifications despite being almost indistinguishable from the data used to train and test the network. This thesis explores to what degree finding these examples may be aided by using side-channel information, specifically power consumption, of hardware implementations of ANNs. A blackbox threat scenario is assumed, where an attacker has access to the ANN hardware's input, outputs, and topology, but the trained model parameters are unknown. The extraction of the ANN parameters is performed by training a surrogate model using a dataset derived from querying the blackbox (oracle) model. The effect of the surrogate's training set size on the accuracy of the extracted parameters was examined. It was found that the distance between the surrogate and oracle parameters increased with larger training set sizes, while the angle between the two parameter vectors held approximately constant at 90 degrees. However, it was found that the transferability of attacks from the surrogate to the oracle improved linearly with increased training set size with lower attack strength. Next, a novel method was developed to incorporate power consumption side-channel information from the oracle model into the surrogate training based on a Siamese neural network structure and a simplified power model. Comparison between surrogate models trained with and without power consumption data indicated that incorporation of the side channel information increases the fidelity of the model extraction by up to 30%. However, no improvement of transferability of adversarial examples was found, indicating behavior dissimilarity of the models despite them being closer in weight space.
Publisher Site: RIT Repository
PDF (Requires Sign-In): PDF
Click for Bibtex
@book{li2021model, title = {Model Extraction and Adversarial Attacks on Neural Networks Using Side-Channel Information}, author = {Li, Tommy}, year = {2021}, school = {Rochester Institute of Technology} }
[T-2021-57] Sheethal Umesh Nagalakshmi. (2021). “Analysis of nucleosomal DNA patterns around transcription factor binding sites.” Rochester Institute of Technology.
Abstract: Nucleosomes are ~147bp DNA wrapped around the histone octamer which are involved in regulating gene transcription. They have the ability to disassemble depending on the process they are involved in and the nucleosome positioning controls the output of the genome. Therefore, it is important to understand the nucleosome positioning and how its positioning affects the binding of transcription factors (TFs) and gene expression thereby regulating the transcription outcome of the genome. Many studies suggest that TFs and nucleosomes compete with each other for genome accessibility. However, the majority of the studies focus on the nucleosome organization rather than underlying DNA sequences and its patterns which might actually be playing an important role in understanding the regulatory role of nucleosomes in gene transcription. This research study focuses on identifying the specific sequence patterns at or around TF binding sites. The study specifically focuses on identifying the fraction of nucleosomes with WW/SS and anti - WW/SS sequence patterns as they might be responsible for maintaining the stability of the nucleosomes. This will provide a new molecular mechanism underlying NDR formation around TF binding sites and pioneer TF-induced chromatin opening.
Publisher Site: RIT Repository
PDF (Requires Sign-In): PDF
Click for Bibtex
@book{nagalakshmi2021analysis, title = {Analysis of nucleosomal DNA patterns around transcription factor binding sites}, author = {Nagalakshmi, Sheethal Umesh}, year = {2021}, school = {Rochester Institute of Technology} }
[T-2021-56] Geeta Madhav Gali. (2021). “Analyzing emotion regulation using multimodal data.” Rochester Institute of Technology.
Abstract: An emotion is a state of one's mind that derives from situations, mood, etc. Understanding people's emotions is a complex problem. People express their emotions using facial expressions or via voice modulations; also, they use emotion regulation strategies such as cognitive reappraisal and expressive suppression in certain instances. Emotion regulation is a method by which we alter our emotions by changing how we express and perceive different situations. Understanding these emotional regulations helps to interact better, communicate and provide care. In this research, we analyze, visualize the differences and distinguish the emotions of disgust and humor with and without emotion regulation strategies. This is done by analyzing electrocardiography data (EKG), electroencephalogram data (EEG), galvanic skin response (GSR), and facial action units (FAU) data with machine learning algorithms such as convolutional neural networks (CNN), gated recurrent units (GRU), long short term memory (LSTM) and support vector machines (SVM). The data is collected by exposing participants (N = 21) to an inductive emotion video in a controlled environment.
Publisher Site: RIT Repository
PDF (Requires Sign-In): PDF
Click for Bibtex
@book{gali2021analyzing, title = {Analyzing emotion regulation using multimodal data}, author = {Gali, Geeta Madhav}, year = {2021}, school = {Rochester Institute of Technology} }
[T-2021-55] Sultan Fahad Almassari. (2021). “Can feature requests reveal the refactoring types?.” Rochester Institute of Technology.
Abstract: Software refactoring is the process of improving the design of a software system while preserving its external behavior. In recent years, refactoring research has been growing as a response to the degradation of software quality. Recent studies performed an in-depth investigation in (1) how refactoring practices are taking place during the software evolution, (2) how to recommend refactoring to improve the design of software, and (3) what type of refactoring operations can be implemented. However, there is a lack of support when it comes to developers' typical programming tasks, including feature updates and bug fixes. The goal of this thesis is to investigate whether it is possible to support the developer through recommending appropriate refactoring types to be performed when the developer is assigned a given issue to handle. Our proposed solution will take as input the text of the issue along with the source code and tries to protect the appropriate refactoring type that would help in adapting efficiently the existing source code to the given feature request. To do so, we rely on the use of supervised learning. We start with collecting various issues that were handled using refactoring. This data will be used to train a model that will be able to predict the appropriate refactoring, given as input an Open issue description. We design a classification model that inputs a feature request and suggests a method-level refactoring. The classification model was trained with a total of 4008 feature request examples of four refactoring types. Our initial results show that this solution suffers from several challenges including the class imbalance: not all refactoring types are equally used to handle issues. Another challenge we detected is related to the description of the issue itself which typically does not explicitly mention any potential refactoring. Therefore, there will be a need for a large set of issues to be able to appropriately learn any patterns among them that would discriminate towards a given refactoring type.
Publisher Site: RIT Repository
PDF (Requires Sign-In): PDF
Click for Bibtex
@book{almassari2021can, title = {Can feature requests reveal the refactoring types?}, author = {Almassari, Sultan Fahad}, year = {2021}, school = {Rochester Institute of Technology} }
[T-2021-54] Ethan Morris. (2021). “Automatic Speech Recognition for Low-Resource and Morphologically Complex Languages.” Rochester Institute of Technology.
Abstract: The application of deep neural networks to the task of acoustic modeling for automatic speech recognition (ASR) has resulted in dramatic decreases of word error rates, allowing for the use of this technology in smart phones and personal home assistants in high-resource languages. Developing ASR models of this caliber, however, requires hundreds or thousands of hours of transcribed speech recordings, which presents challenges for most of the world's languages. In this work, we investigate the applicability of three distinct architectures that have previously been used for ASR in languages with limited training resources. We tested these architectures using publicly available ASR datasets for several typologically and orthographically diverse languages, whose data was produced under a variety of conditions using different speech collection strategies, practices, and equipment. Additionally, we performed data augmentation on this audio, such that the amount of data could increase nearly tenfold, synthetically creating higher resource training. The architectures and their individual components were modified, and parameters explored such that we might find a best-fit combination of features and modeling schemas to fit a specific language morphology. Our results point to the importance of considering language-specific and corpus-specific factors and experimenting with multiple approaches when developing ASR systems for resource-constrained languages.
Publisher Site: RIT Repository
PDF (Requires Sign-In): PDF
Click for Bibtex
@book{morris2021automatic, title = {Automatic Speech Recognition for Low-Resource and Morphologically Complex Languages}, author = {Morris, Ethan}, year = {2021}, school = {Rochester Institute of Technology} }
[T-2021-53] Saad Khan. (2021). “Pedagogical Evaluation of Cognitive Accessibility Learning Lab in the Classroom.” Rochester Institute of Technology.
Abstract: In a study conducted by Webaim, 98.1% of sites had a detectable accessibility issue. This poses a profound challenge to the 1 billion users across the world who have a disability. This indicates that developers either are not aware of how to make the sites accessible or aware of how critical it is to make the sites usable by all users. This problem is further compounded by the lack of available resources that can educate students and future developers in making their software accessible. To address current limitations/challenges, we have developed an all-in-one immersive learning experience known as the Accessibility Learning Labs (ALL). These modules are carefully crafted to provide students a better understanding of various accessibility topics and increase awareness. They incorporate the best of all learning methods, from case studies to hands-on activities and quizzes. In this paper, we focus specifically on the cognitive module developed under the Accessibility Learning Labs. This module strives to educate students on the importance of building accessible software for users with cognitive disabilities. We discuss the pedagogical approach used to craft the components of the cognitive module and the design rationale behind the experiential activity. We investigate how the order of the reading and experiential activity affect the students' understanding of the material. To do this, we perform a study involving 28 students in 2 computer science-related courses. Our findings include: (I) The accessibility improvements made in the lab have a positive impact on the students' performance when compared to the inaccessible version (II) When the reading material is presented after the experiential activity, students have a better understanding of the cognitive accessibility principles.
Publisher Site: RIT Repository
PDF (Requires Sign-In): PDF
Click for Bibtex
@book{khan2021pedagogical, title = {Pedagogical Evaluation of Cognitive Accessibility Learning Lab in the Classroom}, author = {Khan, Saad}, year = {2021}, school = {Rochester Institute of Technology} }
[T-2021-52] Joe Klesczewski. (2021). “Combinatorial and Stochastic Approach to Parallelization of the Kangaroo Method of Solving the Discrete Logarithm Problem.” Rochester Institute of Technology.
Abstract: The kangaroo method for the Pollard's rho algorithm provides a powerful way to solve discrete log problems. There exist parameters for it that allow it to be optimized in such a way as to prevent what are known as "useless collisions" in exchange for the limitation that the number of parallel resources used must be both finite and known ahead of time. This thesis puts forward an analysis of the situation and examines the potential acceleration that can be gained through the use of parallel resources beyond those initially utilized by an algorithm so configured. In brief, the goal in doing this is to reconcile the rapid rate of increase in parallel processing capabilities present in consumer level hardware with the still largely sequential nature of a large portion of the algorithms used in the software that is run on that hardware. The core concept, then, would be to allow "spare" parallel resources to be utilized in an advanced sort of guess-and-check to potentially produce occasional speedups whenever, for lack of a better way to put it, those guesses are correct. The methods presented in this thesis are done so with an eye towards expanding and reapplying them to this broadly expressed problem, however herein the discrete log problem has been chosen to be utilized as a suitable example of how such an application can proceed. This is primarily due to the observation that Pollard's parameters for the avoidance of so-called "useless collisions" generated from the kangaroo method of solving said problem are restrictive in the number of kangaroos used at any given time. The more relevant of these restrictions to this point is the fact that they require the total number of kangaroos to be odd. Most consumer-level hardware which provides more than a single computational core provides an even number of such cores, so as a result it is likely the utilization of such hardware for this purpose will leave one or more cores idle. While these idle compute cores could also potentially be utilized for other tasks given that we are expressly operating in the context of consumer-level hardware, such considerations are largely outside the scope of this thesis. Besides, with the rate of change consumer computational hardware and software environments have historically changed it seems to be more useful to address the topic on a more purely algorithmic level; at the very least, it is more efficient as less effort needs to be expended future-proofing this thesis against future changes to its context than might have otherwise been necessary.
Publisher Site: RIT Repository
PDF (Requires Sign-In): PDF
Click for Bibtex
@book{klesczewski2021combinatorial, title = {Combinatorial and Stochastic Approach to Parallelization of the Kangaroo Method of Solving the Discrete Logarithm Problem}, author = {Klesczewski, Joe}, year = {2021}, school = {Rochester Institute of Technology} }
[T-2021-51] Hannah Saxena. (2021). “Evaluating Electronic Pulse Generators for Cooling Tower Sanitation.” Rochester Institute of Technology.
Abstract: Cooling towers are possible sources of contamination to the environment, with implications to human health. Cooling towers are known sources for disease outbreaks. The cooling process releases aerosols, which if cooling towers are not adequately sanitized, pathogenic bacteria may be released and contaminate the environment. Compounding the risk of pathogenic bacteria release, cooling towers provide ideal conditions for biofilms to grow which encourage the exchange of antibiotic resistance genes. Current sanitation methods are unable to prevent or effectively remove biofilms in cooling towers. Therefore, a new sanitation method is necessary. This research explores the feasibility of using electrical pulse generators (EPG) manufactured by Environmental Energy Technologies Inc. (EET) to disinfect cooling tower water. This sanitation method constantly lyses bacterial cells by sending pulsed electrical fields (PEF) through the water. EET has developed a standard EPG (STD EPG) that is currently in use and an experimental EPG (EXP EPG) that is a purportedly improved version of the STD EPG. The purpose of this study was to determine if the EXP EPG was more effective than the STD EPG to sanitize cooling towers through evaluating the microbial CFUs/ ml and diversity. Water samples from each EPG treatment were collected from several building installation on the Rochester Institute of Technology (RIT) campus. These were examined for microbial richness, diversity, antibiotic resistance, and identity using the 16S rRNA gene. The results of the study suggest that there is antibiotic resistant bacteria present in cooling towers. In addition to this seasonality impacted species diversity where the fall had a lower diversity than the summer. Finally, it was determined the two EPG treatments both able to effectively sanitized cooling towers, but it was indistinguishable which treatment was more effective.
Publisher Site: RIT Repository
PDF (Requires Sign-In): PDF
Click for Bibtex
@book{saxena2021evaluating, title = {Evaluating Electronic Pulse Generators for Cooling Tower Sanitation}, author = {Saxena, Hannah}, year = {2021}, school = {Rochester Institute of Technology} }
[T-2021-50] Daniel W. Adams. (2021). “The Application of Echo State Networks to Atypical Speech Recognition.” Rochester Institute of Technology.
Abstract: Automatic speech recognition (ASR) techniques have improved extensively over the past few years with the rise of new deep learning architectures. Recent sequence-to-sequence models have been shown to have high accuracy by utilizing the attention mechanism, which evaluates and learns the magnitude of element relationships in sequences. Despite being highly accurate, commercial ASR models have a weakness when it comes to accessibility. Current commercial deep learning ASR models find difficulty evaluating and transcribing speech for individuals with unique vocal features, such as those with dysarthria, heavy accents, as well as deaf and hard-of-hearing individuals. Current methodologies for processing vocal data revolve around convolutional feature extraction layers, dulling the sequential nature of the data. Alternatively, reservoir computing has gained popularity for the ability to translate input data to changing network states, which preserves the overall feature complexity of the input. Echo state networks (ESN), a type of reservoir computing mechanism employing a random recurrent neural network, have shown promise in a number of time series classification tasks. This work explores the integration of ESNs into deep learning ASR models. The Listen, Attend and Spell, and Transformer models were utilized as a baseline. A novel approach that used the echo state network as a feature extractor was explored and evaluated using the two models as baseline architectures. The models were trained on 960 hours of LibriSpeech audio data and tuned on various atypical speech data, including the Torgo dysarthric speech dataset and University of Memphis SPAL dataset. The ESN-based Echo, Listen, Attend, and Spell model produced more accurate transcriptions when evaluating on the LibriSpeech test set compared to the ESN-based Transformer. The baseline transformer model achieved a 43.4% word error rate on the Torgo test set after full network tuning. A prototype ASR system was developed to utilize both the developed model as well as commercial smart assistant language models. The system operates on a Raspberry Pi 4 using the Assistant Relay framework.
Publisher Site: RIT Repository
PDF (Requires Sign-In): PDF
Click for Bibtex
@book{adams2021the, title = {The Application of Echo State Networks to Atypical Speech Recognition}, author = {Adams, Daniel W.}, year = {2021}, school = {Rochester Institute of Technology} }
[T-2021-49] Daniel F. Stafford. (2021). “Evaluating Performance and Efficiency of a 16-bit Substitution Box on an FPGA.” Rochester Institute of Technology.
Abstract: A Substitution Box (S-Box) is an integral component of modern block ciphers that provides confusion. The term "confusion" was introduced by Shannon in 1949 and it refers to the complexity of the relationship between the key and the ciphertext. Most S-Boxes are non-linear in order to promote confusion. Due to this, the S-Box is usually the most complex component of a block cipher. The Advanced Encryption Standard (AES) features an 8-bit S-Box where the output depends on the Galois field multiplicative inverse of the input. MK-3 is a sponge based Authenticated Encryption (AE) algorithm which provides both authenticity and confidentiality. It was developed through a joint effort between the Rochester Institute of Technology and the former Harris Corporation, now L3Harris. The MK-3 algorithm has a state that is 512 bits wide and it uses 32 instances of a 16-bit S-Box to cover the entire state. These 16-bit S-Boxes are similar to what is seen in the AES, however, they are notably larger and more complex. Binary Galois field arithmetic is well suited to hardware implementations where addition and multiplication are mapped to a combination of basic XOR and AND operations. A simple method to calculate Galois field multiplicative inversion is through the extended Euclidean algorithm. This is, however, very expensive to implement in hardware. A possible solution is to use a composite field representation, where the original operation is broken down to a series of simpler operations in the base field. This lends itself very well to implementations that consume less area and power with better performance. Given the size and number of the S-Boxes in MK-3, these units contribute to the majority of the implementation resources. Several composite field structures are explored in this work which provide different area utilization and clock frequency characteristics. This thesis evaluates the composite field structures and recommends several candidates for high performing MK-3 Field Programmable Gate Array (FPGA) applications.
Publisher Site: RIT Repository
PDF (Requires Sign-In): PDF
Click for Bibtex
@book{stafford2021evaluating, title = {Evaluating Performance and Efficiency of a 16-bit Substitution Box on an FPGA}, author = {Stafford, Daniel F.}, year = {2021}, school = {Rochester Institute of Technology} }
[T-2021-48] Andrew R. Haverly. (2021). “A Comparison of Quantum Algorithms for the Maximum Clique Problem.” Rochester Institute of Technology.
Abstract: Two of the most promising computational models for quantum computing are the qubit-based model and the continuous variable model, which result in two different computational approaches, namely the qubit gate model and boson sampling. The qubit gate model is a universal form of quantum computation that relies heavily on the principles of superposition and entanglement to solve problems using qubits based on technologies ranging from magnetic fields created from superconducting materials to the spins of valence electrons in atoms. Boson sampling is a non-universal form of quantum computation that uses bosons as continuous-variable values for its computation. Both models show promising prospects for useful quantum advantages over classical computers, but these models are fundamentally different, not only on their technologies but on their applications. Each model excels in different sets of applications. A direct comparison for solving a problem using qubit gate models and boson sampling allows one to better understand not only the individual technologies, but how to decide which model is better suited to solving a given problem and how to start development on solving the given problem. This thesis uses the maximum clique problem to examine the application development process in the qubit gate model and boson sampling as well as a comparison of other known algorithms to the maximum clique problem. The maximum clique problem is an NP-Hard problem concerned with finding the largest fully-connected subgraph. The qubit gate model algorithm to the maximum clique problem is a novel algorithm.
Publisher Site: RIT Repository
PDF (Requires Sign-In): PDF
Click for Bibtex
@book{haverly2021a, title = {A Comparison of Quantum Algorithms for the Maximum Clique Problem}, author = {Haverly, Andrew R.}, year = {2021}, school = {Rochester Institute of Technology} }
[T-2021-47] Matthew T. Millar. (2021). “Flexible Memory Protection with Dynamic Authentication Trees.” Rochester Institute of Technology.
Abstract: As computing appliances increase in use and handle more critical information and functionalities, the importance of security grows even greater. In cases where the device processes sensitive data or performs important functionality, an attacker may be able to read or manipulate it by accessing the data bus between the processor and memory itself. As it is impossible to provide physical protection to the piece of hardware in use, it is important to provide protection against revealing confidential information and securing the device's intended operation. Defense against bus attacks such as spoofing, splicing, and replay attacks are of particular concern. Traditional memory authentication techniques, such as hashes and message authentication codes, are costly when protecting off-chip memory during run-time. Balanced authentication trees such as the well-known Merkle tree or TEC-Tree are widely used to reduce this cost. While authentication trees are less costly than conventional techniques it still remains expensive. This work proposes a new method of dynamically updating an authentication tree structure based on a processor's memory access pattern. Memory addresses that are more frequently accessed are dynamically shifted to a higher tree level to reduce the number of memory accesses required to authenticate that address. The block-level AREA technique is applied to allow for data confidentiality with no additional cost. An HDL design for use in an FPGA is provided as a transparent and highly customizable AXI-4 memory controller. The memory controller allows for data confidentiality and authentication for random-access memory with different speed or memory size constraints. The design was implemented on a Zynq 7000 system-on-chip using the processor to communicate with the hardware design. The performance of the dynamic tree design is comparable to the TEC-Tree in several memory access patterns. The TEC-Tree performs better than a dynamic design in particular applications; however, speedup over the TEC-Tree is possible to achieve when applied in scenarios that frequently accessed previously processed data.
Publisher Site: RIT Repository
PDF (Requires Sign-In): PDF
Click for Bibtex
@book{millar2021flexible, title = {Flexible Memory Protection with Dynamic Authentication Trees}, author = {Millar, Matthew T.}, year = {2021}, school = {Rochester Institute of Technology} }
[T-2021-46] Diptanu Sarkar. (2021). “An Empirical Study of Offensive Language in Online Interactions.” Rochester Institute of Technology.
Abstract: In the past decade, usage of social media platforms has increased significantly. People use these platforms to connect with friends and family, share information, news and opinions. Platforms such as Facebook, Twitter are often used to propagate offensive and hateful content online. The open nature and anonymity of the internet fuels aggressive and inflamed conversations. The companies and federal institutions are striving to make social media cleaner, welcoming and unbiased. In this study, we first explore the underlying topics in popular offensive language datasets using statistical and neural topic modeling. The current state-of-the-art models for aggression detection only present a toxicity score based on the entire post. Content moderators often have to deal with lengthy texts without any word-level indicators. We propose a neural transformer approach for detecting the tokens that make a particular post aggressive. The pre-trained BERT model has achieved state-of-the-art results in various natural language processing tasks. However, the model is trained on general-purpose corpora and lacks aggressive social media linguistic features. We propose fBERT, a retrained BERT model with over $1.4$ million offensive tweets from the SOLID dataset. We demonstrate the effectiveness and portability of fBERT over BERT in various shared offensive language detection tasks. We further propose a new multi-task aggression detection (MAD) framework for post and token-level aggression detection using neural transformers. The experiments confirm the effectiveness of the multi-task learning model over individual models; particularly when the number of training data is limited.
Publisher Site: RIT Repository
PDF (Requires Sign-In): PDF
Click for Bibtex
@book{sarkar2021an, title = {An Empirical Study of Offensive Language in Online Interactions}, author = {Sarkar, Diptanu}, year = {2021}, school = {Rochester Institute of Technology} }
[T-2021-45] Elijah Cantella. (2021). “Architectural Style: Distortions for Deploying and Managing Deception Technologies in Software Systems.” Rochester Institute of Technology.
Abstract: Deception technologies are software tools that simulate/dissimulate information as security measures in software systems. Such tools can help prevent, detect, and correct security threats in the systems they are integrated with. Despite the continued existence and use of these technologies (~20+ years) the process for integrating them into software systems remains undocumented. This is due to deception technologies varying greatly from one another in a number of different ways. To begin the process of documentation, I have proposed an architectural style that describes one possible way deception technologies may be integrated into software systems. To develop this architectural style, I performed a literature review on deception technologies and the art of deception as a discipline. I break down how deception technologies work according to the art of deception through the simulation and dissimulation of software components. I then examined existing deception technologies and categorize them according to their simulations/dissimulations. The documented and proposed architectural style describes how software systems deploy and manage deceptions. Afterwards, I propose a number of future research opportunities surrounding this subject.
Publisher Site: RIT Repository
PDF (Requires Sign-In): PDF
Click for Bibtex
@book{cantella2021architectural, title = {Architectural Style: Distortions for Deploying and Managing Deception Technologies in Software Systems}, author = {Cantella, Elijah}, year = {2021}, school = {Rochester Institute of Technology} }
[T-2021-44] Mark Connolly. (2021). “A Programmable Processing-in-Memory Architecture for Memory Intensive Applications.” Rochester Institute of Technology.
Abstract: While both processing and memory architectures are rapidly improving in performance, memory architecture is lagging behind. As performance of processing architecture continues to eclipse that of memory, the memory architecture continues to become an increasingly unavoidable bottleneck in computer architecture. There are two drawbacks that are commonly associated with memory accesses: i) large delays causing the processor to remain idle waiting on data to become available and ii) the power consumption required to transfer the data. These performance issues are especially notable in research and enterprise computing applications such as deep learning models. Even when data for an application such as this is transferred to a cache before processing to avoid the delay, the large power cost of the transfer is still incurred. Processing-in-memory (PIM) architectures offer a solution to the issues in modern memory architecture. The inclusion of processing elements within the memory architecture reduces data transfers between the host processor and memory, thus reducing penalties incurred by memory accesses. The programmable-PIM (pPIM) architecture is a novel PIM architecture that delivers the performance enhancements of PIM while delivering a high degree of reprogrammability through the use of look-up tables (LUTs). A novel instruction set architecture (ISA) for the pPIM architecture facilitates the architecture's reprogrammability without large impacts on performance. The ISA takes a microcoded approach to handling the control signals of the pPIM control signals. This approach allows variable-stage instructions at a low additional cost to the overall power and area of the architecture. The versatility of the pPIM architecture enables MAC operations and several common activation functions to be modeled for execution on the architecture. As a measure of performance, post-synthesis models of both the pPIM architecture and the ISA are generated for CNN inference. As a proof-of-concept an FPGA model of the pPIM architecture is developed for representations of a single layer neural network (NN) model for classification of MNIST images.
Publisher Site: RIT Repository
PDF (Requires Sign-In): PDF
Click for Bibtex
@book{connolly2021a, title = {A Programmable Processing-in-Memory Architecture for Memory Intensive Applications}, author = {Connolly, Mark}, year = {2021}, school = {Rochester Institute of Technology} }
[T-2021-43] Andrew deVries. (2021). “Automation and Advancement of Non-Invasive Continuous Blood Glucose Measurements.” Rochester Institute of Technology.
Abstract: Researching the RF application of noninvasive continuous, real time, blood glucose monitoring has been a persistent topic of interest for the research group at the electromagnetic theory and application (ETA) lab at RIT. The technique is based on the effects of glucose on the dielectric properties of blood. When the dielectric permittivity of the tissue changes due to glucose, the resonant frequency and input impedance of an antenna sensor can be related to the change in glucose level, as shown in [1] and [2]. The feasibility of this technique has been demonstrated successfully with one diabetic and one non-diabetic patient in [3]. The goal of this work is to utilize the existing developments to create a robust automated system to be used in clinical trial in a hospital setting. The system has been designed to make real time measurements of blood glucose and remotely monitor the data through a cell phone app. The clinical trial will consist of on-body antenna sensor measurements, and dielectric permittivity measurements of in-vitro blood samples contaminated by glucose. Four different phases are mapped out focusing on consistency, correlation, data collection and accuracy verification. The clinical trial will consist of on-body antenna sensor measurements, dialectic permittivity measurements of blood and traditionally measured blood glucose values. Developing a relationship between these measurements will lead to an algorithm for determining the blood glucose level of a patient. Utilizing existing designs, the antenna sensor is a planar monopole antenna with an artificial magnetic conductor design from [5]. The sensor assembly is a more robust antenna than that which was previously constructed with an AMC layer. The sensor is designed to be insensitive to patient movement because the stability of the sensor is critical for performance in the clinical trial. Simulations have also been performed with a human body model in HFSS to ensure that the Specific Absorption Rate (SAR) levels conform to FDA regulations for biomedical applications. Antenna sensors of each design were fabricated and tested to show an agreement with simulation results for both the resonant frequencies and radiation patterns. An automated measurement system has been developed using MATLAB App Designer to be compatible with bench top Agilent network analyzers as well as the small and portable R140 network analyzer from Copper Mountain Technologies. The application is capable of recording S-parameter measurements and doing real time analysis, as well as uploading the data to an RIT server. Once the processed data is on the server, it can be accessed immediately over the internet by a different computer or a smartphone application. A MATLAB app was designed for monitoring the data remotely and a smart phone application was also developed for the same purpose. The clinical trial will also include an in-vitro study where permittivity measurements will be collected of blood samples containing glucose. In order to support the antenna sensor measurements, a correlation will be obtained from the in-vitro samples between the dielectric permittivity and the concentration of glucose. The dielectric probe has been automated in preparation for the trial. In order to prepare the system for use in clinical trials, extensive testing using an experimental test setup was carried out. The test setup incorporated both the antenna sensor and the dielectric probe to make measurements of water and glucose. With the antenna close enough to the dish and the dielectric probe in the water, both the antenna sensor response and the relative permittivity of the water are observed to change when the concentration of glucose in the dish changes. This combined testing will be imitated in the clinical trial when the antenna sensor is used on a patient's body and permittivity measurements are made of in-vitro blood samples.
Publisher Site: RIT Repository
PDF (Requires Sign-In): PDF
Click for Bibtex
@book{devries2021automation, title = {Automation and Advancement of Non-Invasive Continuous Blood Glucose Measurements}, author = {deVries, Andrew}, year = {2021}, school = {Rochester Institute of Technology} }
[T-2021-42] Dawei Liu. (2021). “Volume-based Estimates of Left Ventricular Blood Pool Volume and Ejection Fraction from Multi-plane 2D Ultrasound Images.” Rochester Institute of Technology.
Abstract: Accurate estimations of left ventricular (LV) blood pool volume and left ventricular ejection fraction (LVEF) are crucial for the clinical diagnosis of cardiac disease, patient management, or other therapeutic treatment decisions, especially given a patient's LVEF often affects his or her candidacy for cardiovascular intervention. Ultrasound (US) imaging is the most common and least expensive imaging modalities used to non-invasively image the heart to estimate the LV blood pool volume and assess LVEF. Despite advances in 3D US imaging, 2D US images are routinely used by cardiologists to image the heart and their interpretation is inherently based on the 2D LV blood pool area information immediately available in the US images, rather than 3D LV blood pool volume information. This work proposes a method to reconstruct the 3D geometry of the LV blood pool from three tri-plane 2D US images to estimate the LV blood pool volume and subsequently the LVEF. This technique uses a statistical shape model (SSM) of the LV blood pool characterized by several anchor points – the mitral valve hinges, apex, and apex-to-mitral valve midpoints – identified from the three multi-plane 2D US images. Given a new patient image dataset, the diastolic and systolic LV blood pool volumes are estimated using the SSM either as a linear combination of the n-closest LV geometries according to the Mahalanobis distance or based on the n-most dominant principal components identified after projecting the new patient into the principal component space defined by the training dataset. The performance of the proposed method was assessed by comparing the estimated LV blood pool volume and LVEF to those measured using the EchoPac PC clinical software on a dataset consisting of 66 patients, and several combinations of 50-16 used for training and validation, respectively. The studies show the proposed method achieves LV volume and LVEF estimates within 5% of those computed using the clinical software. Lastly, this work proposes an approach that requires minimal user interaction to obtain accurate 3D estimates of LV blood pool volume and LVEF using multi-plane 2D US images and confirms its performance similar to the ground truth clinical measurements.
Publisher Site: RIT Repository
PDF (Requires Sign-In): PDF
Click for Bibtex
@book{liu2021volume-based, title = {Volume-based Estimates of Left Ventricular Blood Pool Volume and Ejection Fraction from Multi-plane 2D Ultrasound Images}, author = {Liu, Dawei}, year = {2021}, school = {Rochester Institute of Technology} }
[T-2021-41] Patrick Rynkiewicz. (2021). “SecretePEPPr: Computational Prediction and Characterization of Effector-like Proteins Secreted from Vitis vinifera.” Rochester Institute of Technology.
Abstract: Plant pathology research has long placed a focus on pathogen-derived effector proteins: small, secreted proteins translocated into host cells where they subvert host basal immunity and promote infection. Recent studies suggest that some plant species secrete similar effector-like proteins during mutualistic plant-fungal interactions and affect fungal growth. In this project, a computational tool named SecretePEPPr (Secreted Plant Effector-like Protein Predictor) was written, evaluated, and tested for the purpose of predicting candidate plant effector-like proteins from a set of whole genome annotations. Among other factors, this prediction tool considered classical and non-classical secretion, protein size, and the prevalence and presence of clathrin-mediated endocytic motifs. Analysis on testing data revealed a subcellular localization prediction specificity of 90% on a set of over 500 intracellular plant proteins and sensitivity of 55% on a set of experimentally validated secreted plant proteins. Across four analyzed grape proteomes, several germin-like proteins were identified as potentially haustorially localized through clathrin-mediated endocytic means. Protein length distributions revealed that effector-like candidates containing clathrin-mediated endocytic motifs were mostly in the 150-300 amino acid length range. Follow up in vivo validation was conducted in Erysiphe necator-infected Chardonnay grape leaves. Through this process, the first Erysiphe necator haustorial extraction method was devised using a Percoll Density Gradient followed by fluorescent labeling and fluorescence-activated cell sorting (FACS), resulting in 14 and 18 million purified fungal haustorial cells from 20 grams of heavily infected leaves. Computational streamlining of plant effector-like protein prediction established in this project provides a foundation for the top-down discovery and characterization of this recently discovered class of plant proteins, which have important implications in plant-microbe interactions and may act as targets for breeding and gene editing in plants.
Publisher Site: RIT Repository
PDF (Requires Sign-In): PDF
Click for Bibtex
@book{rynkiewicz2021secretepeppr:, title = {SecretePEPPr: Computational Prediction and Characterization of Effector-like Proteins Secreted from Vitis vinifera}, author = {Rynkiewicz, Patrick}, year = {2021}, school = {Rochester Institute of Technology} }
[T-2021-40] Vijay Gopal Thirupakuzi Vangipuram. (2021). “Development of a Monolithically Integrated GaN Nanowire Memory Device.” Rochester Institute of Technology.
Abstract: Gallium nitride (GaN) devices are of particular interest for a variety of fields and application spaces. The materials properties of GaN make it an ideal semiconductor for electronics and optoelectronics. Within electronics, GaN is of great interest for high-power and high-frequency electronics. Within optoelectronics, GaN has enabled efficient lighting and continues to be scaled for augmented reality and virtual reality (AR/VR) application spaces through the realization of micro-LEDs. In scaling such devices for incorporation into larger systems that span multiple fields, monolithically integrating other devices and components would provide greater flexibility and improve system-level performance. One such avenue for explored here is the development and integration of a GaN-based memory device with a light-emitting diode (LED). Specifically, a nanowire memory device integrated vertically with an LED. Initial work involved developing and verifying a high-κ dielectric memory stack on Si for memory characteristics. This was followed by fabrication of the integrated memory device and LED on a green LED GaN-on-sapphire substrate through a top-down approach. Initial electrical testing showed a functional, blue-shifted LED and the existence of a 2.5V memory window for a +/-10V program/erase window. Finally, a new self-limiting etch technique was examined to address possibilities for further scaling nanowires.
Publisher Site: RIT Repository
PDF (Requires Sign-In): PDF
Click for Bibtex
@book{thirupakuzi vangipuram2021development, title = {Development of a Monolithically Integrated GaN Nanowire Memory Device}, author = {Thirupakuzi Vangipuram, Vijay Gopal}, year = {2021}, school = {Rochester Institute of Technology} }
[T-2021-39] Christian Lusardi. (2021). “Robust Multiple Object Tracking Using ReID features and Graph Convolutional Networks.” Rochester Institute of Technology.
Abstract: Deep Learning allows for great advancements in computer vision research and development. An area that is garnering attention is single object tracking and multi-object tracking. Object tracking continues to progress vastly in terms of detection and building re-identification features, but more effort needs to be dedicated to data association. In this thesis, the goal is to use a graph neural network to combine the information from both the bounding box interaction as well as the appearance feature information in a single association chain. This work is designed to explore the usage of graph neural networks and their message passing abilities during tracking to come up with stronger data associations. This thesis combines all steps from detection through association using state of the art methods along with novel re-identification applications. The metrics used to determine success are Multi-Object Tracking Accuracy (MOTA), Multi-Object Tracking Precision (MOTP), ID Switching (IDs), Mostly Tracked, and Mostly Lost. Within this work, the combination of multiple appearance feature vectors to create a stronger single feature vector is explored to improve accuracy. Different types of data augmentations such as random erase and random noise are explored and their results are examined for effectiveness during tracking. A unique application of triplet loss is also implemented to improve overall network performance as well. Throughout testing, baseline models have been improved upon and each successive improvement is added to the final model output. Each of the improvements results in the sacrifice of some performance metrics but the overall benefits outweigh the costs. The datasets used during this thesis are the UAVDT Benchmark and the MOT Challenge Dataset. These datasets cover aerial-based vehicle tracking and pedestrian tracking. The UAVDT Benchmark and MOT Challenge dataset feature crowded scenery as well as substantial object overlap. This thesis demonstrates the increased matching capabilities of a graph network when paired with a robust and accurate object detector as well as an improved set of appearance feature vectors.
Publisher Site: RIT Repository
PDF (Requires Sign-In): PDF
Click for Bibtex
@book{lusardi2021robust, title = {Robust Multiple Object Tracking Using ReID features and Graph Convolutional Networks}, author = {Lusardi, Christian}, year = {2021}, school = {Rochester Institute of Technology} }
[T-2021-38] Hee Tae An. (2021). “Demonstration of Automated DNA Assembly on a Digital Microfluidic Device.” Rochester Institute of Technology.
Abstract: The rapid manufacturing of highly accurate synthetic DNA is crucial for its use as a molecular tool, the understanding and engineering of regulatory elements, protein engineering, genetic refactoring, engineered genetic networks and metabolic pathways, and whole-genome syntheses [1,2]. Recent efforts in the development of enzyme mediated oligonucleotide synthesis have shown much potential to benefit conventional DNA synthesis [3–5]. With its success in applications as chemical microreactors [6–10], biological assays [8–14], and clinical diagnostic tools [10,15–19], digital microfluidic (DMF) devices are an attractive platform to apply the promising benefits of enzymatic oligonucleotide synthesis to the manufacturing of synthetic DNA. This thesis work aims to demonstrate automated DNA assembly using oligonucleotides on a DMF device through the demonstration and validation of an automated DNA assembly protocol. The prototyping process performed through this work revealed various important design considerations for the reliability of fluid handling performance and the mitigation of failure modes. To prevent dielectric breakdown or electrolysis, a relatively thick SU-8 3005 dielectric is used to remove the sensitivity of the device to variances in dielectric thickness and quality. To enable droplet creation, the gap distance between the DMF chip and top-plate is created and minimized using a thick SU-8 2100 layer. Reliable droplet creation is achieved through the use of electrode geometry that targets predictable fluid delivery and cutting. Reliable droplet transport is achieved through the use of a electrode interdigitation geometry that targets lower total electrode surface area and higher interdigitation contact area. The testing of DNA laden fluids revealed that biofouling can be a large concern for the demonstration of DNA assembly on a DMF device if droplets are moved through an air medium. To mitigate its effects, the final DMF device design featured the use of a permanently bonded top-plate with bored inlet/outlet ports as well as a silicone oil medium. The final DMF device design was used to demonstrate automated DNA assembly. This demonstration involved the creation, transport, and mixing of DNA brick samples. These samples are subsequently incubated on a chemical bench or on the DMF chip to create recombinant DNA containing genetic information. DNA gel imaging of DNA assembly products from on-chip protocols compared to protocols performed on a chemical benchtop revealed comparable results. Through the course of this work, the applicability of automated DNA assembly on a DMF device was validated to provide preliminary results in the ultimate goal of DNA synthesis using enzymatic oligonucleotide synthesis.
Publisher Site: RIT Repository
PDF (Requires Sign-In): PDF
Click for Bibtex
@book{an2021demonstration, title = {Demonstration of Automated DNA Assembly on a Digital Microfluidic Device}, author = {An, Hee Tae}, year = {2021}, school = {Rochester Institute of Technology} }
[T-2021-37] Shravan Bhoopasamudram Krishna. (2021). “Experimental analysis of using radar as an extrinsic sensor for human-robot collaboration.” Rochester Institute of Technology.
Abstract: Collaborative robots are expected to be an integral part of driving the fourth industrial revolution that is soon expected to happen. In human-robot collaboration, the robot and a human share a common workspace and work on a common task. Here the safety of a human working with the robot is of utmost importance. A collaborative robot usually consists of various sensors to ensure the safety of a human working with the robot. This research mainly focuses on establishing a safe environment for a human working alongside a robot by mounting an FMCW radar as an extrinsic sensor, through which the workspace of the robot is monitored. A customized tracking algorithm is developed for the sensor used in this study by including a dynamically varying gating threshold, and information about consecutive missed detections to track and localize the human around the workspace of the robot. The performance of the proposed system in successfully establishing a safe human-robot collaboration is examined across a few scenarios that arise when a single human operator is working alongside a robot, with the radar operating in different modes. An OptiTrack Motion Capture System is used as ground truth to validate the efficacy of the proposed system.
Publisher Site: RIT Repository
PDF (Requires Sign-In): PDF
Click for Bibtex
@book{krishna2021experimental, title = {Experimental analysis of using radar as an extrinsic sensor for human-robot collaboration}, author = {Krishna, Shravan Bhoopasamudram}, year = {2021}, school = {Rochester Institute of Technology} }
[T-2021-36] Sahil K. Gogna. (2021). “Securing in-memory processors against Row Hammering Attacks.” Rochester Institute of Technology.
Abstract: Modern applications on general purpose processors require both rapid and power-efficient computing and memory components. As applications continue to improve, the demand for high speed computation, fast-access memory, and a secure platform increases. Traditional Von Neumann Architectures split the computing and memory units, causing both latency and high power-consumption issues; henceforth, a hybrid memory processing system is proposed, known as in-memory processing. In-memory processing alleviates the delay of computation and minimizes power-consumption; such improvements saw a 14x speedup improvement, 87\% fewer power consumption, and appropriate linear scalability versus performance. Several applications of in-memory processing include data-driven applications such as Artificial Intelligence (AI), Convolutional and Deep Neural Networks (CNNs/DNNs). However, processing-in-memory can also suffer from a security and reliability issue known as the Row Hammer Security Bug; this security exploit flips bits within memory without access, leading to error injection, system crashes, privilege separation, and total hijack of a system; the novel Row Hammer security bug can negatively impact the accuracies of CNNs and DNNs via flipping the bits of stored weight values without direct access. Weights of neural networks are stored in a variety of data patterns, resulting in either a solid (all 1s or all 0s), checkered (alternating 1s and 0s in both rows and columns), row-stripe (alternating 1s and 0s in rows), or column-striped (alternating 1s and 0s in columns) manner; the row-stripe data pattern exhibits the largest likelihood of a Row Hammer attack, resulting in the accuracies of neural networks dropping over 30\%. A row-stripe avoidance coding scheme is proposed to reduce the probability of the Row Hammer Attack occurring within neural networks. The coding scheme encodes the binary portion of a weight in a CNN or DNN to reduce the chance of row-stripe data patterns, overall reducing the likelihood of a Row Hammer attack occurring while improving the overall security of the in-memory processing system.
Publisher Site: RIT Repository
PDF (Requires Sign-In): PDF
Click for Bibtex
@book{gogna2021securing, title = {Securing in-memory processors against Row Hammering Attacks}, author = {Gogna, Sahil K.}, year = {2021}, school = {Rochester Institute of Technology} }
[T-2021-35] Aishwarya Rao. (2021). “Calibrating Knowledge Graphs.” Rochester Institute of Technology.
Abstract: A knowledge graph model represents a given knowledge graph as a number of vectors. These models are evaluated for several tasks, and one of them is link prediction, which consists of predicting whether new edges are plausible when the model is provided with a partial edge. Calibration is a postprocessing technique that aims to align the predictions of a model with respect to a ground truth. The idea is to make a model more reliable by reducing its confidence for incorrect predictions (overconfidence), and increasing the confidence for correct predictions that are closer to the negative threshold (underconfidence). Calibration for knowledge graph models have been previously studied for the task of triple classification, which is different than link prediction, and assuming closed-world, that is, knowledge that is missing from the graph at hand is incorrect. However, knowledge graphs operate under the open-world assumption such that it is unknown whether missing knowledge is correct or incorrect. In this thesis, we propose open-world calibration of knowledge graph models for link prediction. We rely on strategies to synthetically generate negatives that are expected to have different levels of semantic plausibility. Calibration thus consists of aligning the predictions of the model with these different semantic levels. Nonsensical negatives should be farther away from a positive than semantically plausible negatives. We analyze several scenarios in which calibration based on the sigmoid function can lead to incorrect results when considering distance-based models. We also propose the Jensen-Shannon distance to measure the divergence of the predictions before and after calibration. Our experiments exploit several pre-trained models of nine algorithms over seven datasets. Our results show that many of these pre-trained models are properly calibrated without intervention under the closed-world assumption, but it is not the case for the open-world assumption. Furthermore, Brier scores (the mean squared error before and after calibration) using the closed-world assumption are generally lower and the divergence is higher when using open-world calibration. From these results, we gather that open-world calibration is a harder task than closed-world calibration. Finally, analyzing different measurements related to link prediction accuracy, we propose a combined loss function for calibration that maintains the accuracy of the model.
Publisher Site: RIT Repository
PDF (Requires Sign-In): PDF
Click for Bibtex
@book{rao2021calibrating, title = {Calibrating Knowledge Graphs}, author = {Rao, Aishwarya}, year = {2021}, school = {Rochester Institute of Technology} }
[T-2021-34] Saurabh Mahendra Sonje. (2021). “3D Object Detection Via 2D LiDAR Corrected Pseudo LiDAR Point Clouds.” Rochester Institute of Technology.
Abstract: The age of automation has led to significant research in the field of Machine Learning and Computer Vision. Computer Vision tasks fundamentally rely on information from digital images, videos, texts and sensors to build intelligent systems. In recent times, deep neural networks combined with computer vision algorithms have been successful in developing 2D object detection methods with a potential to be applied in real-time systems. However, performing fast and accurate 3D object detection is still a challenging problem. The automotive industry is shifting gears towards building electric vehicles, connected cars, sustainable vehicles and is expected to have a high growth potential in the coming years. 3D object detection is a critical task for autonomous driving vehicles and robots as it helps moving objects in the scene to effectively plan their motion around other objects. 3D object detection tasks leverage image data from camera and/or 3D point clouds obtained from expensive 3D LiDAR sensors to achieve high detection accuracy. The 3D LiDAR sensor provides accurate depth information that is required to estimate the third dimension of the objects in the scene. Typically, a 64 beam LiDAR sensor mounted on a self-driving car cost around $75000. In this thesis, we propose a cost-effective approach for 3D object detection using a low-cost 2D LiDAR sensor. We collectively use the single beam point cloud data from 2D LiDAR for depth correction in pseudo-LiDAR. The proposed methods are tested on the KITTI 3D object detection dataset.
Publisher Site: RIT Repository
PDF (Requires Sign-In): PDF
Click for Bibtex
@book{sonje20213d, title = {3D Object Detection Via 2D LiDAR Corrected Pseudo LiDAR Point Clouds}, author = {Sonje, Saurabh Mahendra}, year = {2021}, school = {Rochester Institute of Technology} }
[T-2021-33] Ethan Tola. (2021). “Real-Time UAV Pose Estimation and Tracking Using FPGA Accelerated April Tag.” Rochester Institute of Technology.
Abstract: April Tags and other passive fiducial markers are widely used to determine localization using a monocular camera. It utilizes specialized algorithms that detect markers to calculate their orientation and distance in three dimensional (3-D) space. The video and image processing steps performed to use these fiducial systems dominate the computation time of the algorithms. Low latency is a key component for the real-time application of these fiducial markers. The drawbacks of performing the video and image processing in software is the difficulty in performing the same operation in parallel effectively. Specialized hardware instantiations with the same algorithm scan efficiently parallelize them as well as operate on the image in a streaming fashion. Compared to graphics processing units (GPUs) that also perform well in the field, field programmable gate arrays (FPGAs) operate with less power, making them optimal with tight power constraints. This research describes such an optimization for the April Tag algorithm on an unmanned aerial vehicle with an embedded platform to perform real-time pose estimation, tracking, and localization in GPS-denied (global positioning system) environments at 30 frames per second (FPS) by converting the initial embedded C/C++ solution to a heterogeneous one through hardware acceleration. It compares the size, accuracy, and speed of the April Tag algorithm's various implementations. The initial solution operated at around 2 FPS while the final solution, a novel heterogeneous algorithm on the Fusion 2 Zynq 7020 system on chip (SoC), operated at around 43 FPS using hardware acceleration. The research proposes a pipeline that breaks the algorithm into distinct steps where portions of it can be improved by utilizing algorithms optimized to run on a FPGA. Additional steps were made to further reduce the hardware algorithm's resource utilization. Each step in the software was compared against its hardware counterpart using its utilization and timing as benchmarks.
Publisher Site: RIT Repository
PDF (Requires Sign-In): PDF
Click for Bibtex
@book{tola2021real-time, title = {Real-Time UAV Pose Estimation and Tracking Using FPGA Accelerated April Tag}, author = {Tola, Ethan}, year = {2021}, school = {Rochester Institute of Technology} }
[T-2021-32] Zachary Diermyer. (2021). “A Spectral Component Analysis of Repeating FRB121102.” Rochester Institute of Technology.
Abstract: Fast Radio Bursts (FRBs) are extremely luminous, millisecond duration radio pulses of unknown origin. While many FRBs are transient events, a few sources are confirmed to be the progenitor of multiple bursts. These "repeaters" offer an extraordinary chance to study the properties of mysterious FRB signals. The FRB121102 source was the first repeater to have ever been discovered and it has since provided us with a wealth of data to be analyzed. A unique feature of repeaters, including FRB121102, is the presence of a multicomponent burst structure. This is a phenomenon where individual portions of the burst are separated in time. Understanding the true fraction of FRBs which contain a multicomponent structure will place limitations on the physical mechanisms which can potentially emit the bursts. We investigate this feature within the Breakthrough Listen data of FRB121102. Breakthrough Listen recorded an observation that detected 21 FRBs in one hour, making it a great source of uniform data. Our analysis compares the shapes of multicomponent and single-component bursts within the Breakthrough Listen data set. We measure fluence distribution shape using statistical moments. This process is original work which provides a new method of analysis for FRB research. Through artificial reduction of the signal-to-noise ratio of multicomponent bursts, we observe how their shape changes compared to single-component bursts. This demonstrates the potential for multicomponent burst structure to be "washed out" by noise, making some FRBs appear as single-component to the observer. We assert that the true fraction of multicomponent bursts could potentially be larger than observed.
Publisher Site: RIT Repository
PDF (Requires Sign-In): PDF
Click for Bibtex
@book{diermyer2021a, title = {A Spectral Component Analysis of Repeating FRB121102}, author = {Diermyer, Zachary}, year = {2021}, school = {Rochester Institute of Technology} }
[T-2021-31] Atharva Arun Tembe. (2021). “Monocular 3D Object Detection via Ego View-to-Bird’s Eye View Translation.” Rochester Institute of Technology.
Abstract: The advanced development in autonomous agents like self-driving cars can be attributed to computer vision, a branch of artificial intelligence that enables software to understand the content of image and video. These autonomous agents require a three-dimensional modelling of its surrounding in order to operate reliably in the real-world. Despite the significant progress of 2D object detectors, they have a critical limitation in location sensitive applications as they do not provide accurate physical information of objects in 3D space. 3D object detection is a promising topic that can provide relevant solutions which could improve existing 2D based applications. Due to the advancements in deep learning methods and relevant datasets, the task of 3D scene understanding has evolved greatly in the past few years. 3D object detection and localization are crucial in autonomous driving tasks such as obstacle avoidance, path planning and motion control. Traditionally, there have been successful methods towards 3D object detection but they rely on highly expensive 3D LiDAR sensors for accurate depth information. On the other hand, 3D object detection from single monocular images is inexpensive but lacks in accuracy. The primary reason for such a disparity in performance is that the monocular image-based methods attempt at inferring 3D information from 2D images. In this work, we try to bridge the performance gap observed in single image input by introducing different mapping strategies between the 2D image data and its corresponding 3D representation and use it to perform object detection in 3D. The performance of the proposed method is evaluated on the popular KITTI 3D object detection benchmark dataset.
Publisher Site: RIT Repository
PDF (Requires Sign-In): PDF
Click for Bibtex
@book{tembe2021monocular, title = {Monocular 3D Object Detection via Ego View-to-Bird's Eye View Translation}, author = {Tembe, Atharva Arun}, year = {2021}, school = {Rochester Institute of Technology} }
[T-2021-30] Leander I. Held. (2021). “Designing FeNiCr(CoCu) High Entropy Alloys Using Molecular Dynamics: A Study of the Enhanced Mechanical Properties of a Novel Group of Composites.” Rochester Institute of Technology.
Abstract: High-Entropy Alloys (HEAs) are an emergent class of crystalline materials have exhibited unique mechanical properties and high-temperature functionality. Their unusual composition, having multiple principal elements in contrast to common alloys that use one principal element, results in a variety of useful, and in many cases unexpected characteristics. This project, in partnership with experimentalists at the Idaho National Laboratory and machine learning scientists at the University of Utah, explores these characteristics and composition-property relationships in HEAs. Utilizing Molecular Dynamics (MD) simulations, three HEAs, namely FeNiCr, FeNiCrCo, and FeNiCrCoCu, are studied in detail. The radial distribution function (RDF) and tensile strength (TS) are calculated for each alloy, and their values compared as a function of temperature, chemical content, and slip orientation. We used RDF analysis as a basis to examine the stability of HEAs; Fe- and Ni-dominant alloys consistently show five narrow RDF peaks, indicating a strong fit to the desired FCC lattice. We used this strategy to ensure the accuracy of further calculations as well as to demonstrate that MD offers a cost-effective tool for the qualitative analysis of materials. Additionally, we show that RDF analysis can be used as a basic predictive model for more complicated mechanical properties of a crystal, an important asset in material design and machine learning. Finally, we tested these predictions via study of stress-strain curves to evaluate TS of various alloys. We observe that Fe- and Ni-dominant alloys are strongest with peak values ranging from 23 to 26 GPa, while Co- and Cu-dominant alloys are weakest with peak values of 17 and 13 GPa respectively. We uncover fundamental relationships between TS and chemical composition in HEAs – especially the identity of the dominant element – as well as temperature and slip orientation, all of which are critical variables to consider in material design and functionality. Overall, the results in this work offer basic guidelines to design stable high temperature alloys and further highlight the importance of understanding composition-property relationships in HEAs. This work also underlines the power of MD, as it serves as a first step in establishing a high-throughput computational framework to study diverse alloys, with the ultimate goal of understanding the behavior of HEAs in its entirety.
Publisher Site: RIT Repository
PDF (Requires Sign-In): PDF
Click for Bibtex
@book{held2021designing, title = {Designing FeNiCr(CoCu) High Entropy Alloys Using Molecular Dynamics: A Study of the Enhanced Mechanical Properties of a Novel Group of Composites}, author = {Held, Leander I.}, year = {2021}, school = {Rochester Institute of Technology} }
[T-2021-29] Rachel Golding. (2021). “Hyperspectral Polarization Data for Particulate Materials.” Rochester Institute of Technology.
Abstract: Polarization is a useful quantity in understanding optical data. However, it is also one of the least understood quantities in remote sensing of granular media, and knowledge is particularly sparse in the area of hyperspectral data. There particularly have been few studies of the negative branch of polarization and these models have had limited success in describing observation. This thesis reviews previous studies of both positive and negative polarization by Hapke, Shkuratov, and others, presents experimental results taken in the Rochester Institute of Technology's GRIT laboratory, and describes plans for future research to understand and model polarization. Experimentation described in this thesis shows a relationship between polarization and grain size, as well as a clear wavelength dependence.
Publisher Site: RIT Repository
PDF (Requires Sign-In): PDF
Click for Bibtex
@book{golding2021hyperspectral, title = {Hyperspectral Polarization Data for Particulate Materials}, author = {Golding, Rachel}, year = {2021}, school = {Rochester Institute of Technology} }
[T-2021-28] Narayanan Asuri Krishnan. (2021). “Reproducing and Explaining Entity and Relation Embeddings for Link Prediction in Knowledge Graphs.” Rochester Institute of Technology.
Abstract: Embedding knowledge graphs is a common method used to encode information from the graph at hand projected in a low dimensional space. There are two shortcomings in the field of knowledge graph embeddings for link prediction. The first shortcoming is that, as far as we know, current software libraries to compute knowledge graph embeddings differ from the original papers proposing these embeddings. Certain implementations are faithful to the original papers, while others range from minute differences to significant variations. Due to these implementation variations, it is difficult to compare the same algorithm from multiple libraries and also affects our ability to reproduce results. In this report, we describe a new framework, AugmentedKGE (aKGE), to embed knowledge graphs. The library features multiple knowledge graph embedding algorithms, a rank-based evaluator, and is developed completely using Python and PyTorch. The second shortcoming is that, during the evaluation process of link prediction, the goal is to rank based on scores a positive triple over a (typically large) number of negative triples. Accuracy metrics used in the evaluation of link prediction are aggregations of the ranks of the positive triples under evaluation and do not typically provide enough details as to why a number of negative triples are ranked higher than their positive counterparts. Providing explanations to these triples aids in understanding the results of the link predictions based on knowledge graph embeddings. Current approaches mainly focus on explaining embeddings rather than predictions and single predictions rather than all the link predictions made by the embeddings of a certain knowledge graph. In this report, we present an approach to explain all these predictions by providing two metrics that serve to quantify and compare the explainability of different embeddings. From the results of evaluating aKGE, we observe that the accuracy metrics are better than the accuracy metrics obtained from the standard implementation of OpenKE. From the results of explainability, we observe that the horn rules obtained explain more than 50% of all the negative triples generated.
Publisher Site: RIT Repository
PDF (Requires Sign-In): PDF
Click for Bibtex
@book{krishnan2021reproducing, title = {Reproducing and Explaining Entity and Relation Embeddings for Link Prediction in Knowledge Graphs}, author = {Krishnan, Narayanan Asuri}, year = {2021}, school = {Rochester Institute of Technology} }
[T-2021-27] Geoff Twardokus. (2021). “Intelligent Lower-Layer Denial-of-Service Attacks Against Cellular Vehicle-to-Everything.” Rochester Institute of Technology.
Abstract: Vehicle-to-everything (V2X) communication promises a wide range of benefits for society. Within future V2X-enabled intelligent transportation systems, vehicle-to-vehicle (V2V) communication will allow vehicles to directly exchange messages, improving their situational awareness and allowing drivers or (semi-)autonomous vehicles to avoid collisions, particularly in non-line-of-sight scenarios. Thus, V2V has the potential to reduce annual vehicular crashes and fatalities by hundreds of thousands. Cellular Vehicle-to-Everything (C-V2X) is rapidly supplanting older V2V protocols and will play a critical role in achieving these outcomes. As extremely low latency is required to facilitate split-second collision avoidance maneuvers, ensuring the availability of C-V2X is imperative for safe and secure intelligent transportation systems. However, little work has analyzed the physical- (PHY) and MAC-layer resilience of C-V2X against intelligent, protocol-aware denial-of-service (DoS) attacks by stealthy adversaries. In this thesis, we expose fundamental security vulnerabilities in the PHY- and MAC-layer designs of C-V2X and demonstrate how they can be exploited to devastating effect by devising two novel, intelligent DoS attacks against C-V2X: targeted sidelink jamming and sidelink resource exhaustion. Our attacks demonstrate different ways an intelligent adversary can dramatically degrade the availability of C-V2X for one or many vehicles, increasing the likelihood of fatal vehicle collisions. Through hardware experiments with software-defined radios (SDRs) and state-of-the-art C-V2X devices in combination with extensive MATLAB simulation, we demonstrate the viability and effectiveness of our attacks. We show that targeted sidelink jamming can reduce a targeted vehicle's packet delivery ratio by 90% in a matter of seconds, while sidelink resource exhaustion can reduce C-V2X channel throughput by up to 50% in similarly short order. We further provide and validate detection techniques for each attack based on cluster and regression analysis techniques and propose promising, preliminary approaches to mitigate the underlying vulnerabilities that we expose in the PHY/MAC layers of C-V2X.
Publisher Site: RIT Repository
PDF (Requires Sign-In): PDF
Click for Bibtex
@book{twardokus2021intelligent, title = {Intelligent Lower-Layer Denial-of-Service Attacks Against Cellular Vehicle-to-Everything}, author = {Twardokus, Geoff}, year = {2021}, school = {Rochester Institute of Technology} }
[T-2021-26] Vijay Soorya Shunmuga Sundaram. (2021). “Integration of a Visible-Telecom Photon Pair Source with Silicon Photonics for Quantum Communication.” Rochester Institute of Technology.
Abstract: Reliable transfer of quantum information between nodes of quantum processors and memories is crucial for the realization of many groundbreaking technologies. These include distributed sensing, distributed computation, entanglement swapping, quantum metrology, quantum key distribution etc... which would vastly expand the potential of today's quantum computers and form the framework for a secure quantum internet. However, most quantum nodes are accessed using visible photons which are incompatible with the telecom-band optical fiber network. In this thesis, we propose a method to bridge this spectral mismatch between the quantum nodes and the communication channel using a highly non-degenerate (810nm+1550nm) photon pair source using a Periodically Poled Potassium Titanyl Phosphate (PPKTP) crystal. Previous challenges with such frequency conversion systems like spectral filtering losses, out-coupling losses and feasibility of scalable fabrication are addressed by demonstrating the successful integration of the PPKTP crystal with a Silicon Photonics Integrated Circuit (Si-PIC). Higher pair detection rates than previous studies and efficient coupling between the crystal and the PIC chip are shown. Filter-free operation of the telecom band is demonstrated by using the Silicon waveguides on the PIC for pump photon absorption for the very first time.
Publisher Site: RIT Repository
PDF (Requires Sign-In): PDF
Click for Bibtex
@book{shunmuga sundaram2021integration, title = {Integration of a Visible-Telecom Photon Pair Source with Silicon Photonics for Quantum Communication}, author = {Shunmuga Sundaram, Vijay Soorya}, year = {2021}, school = {Rochester Institute of Technology} }
[T-2021-25] Bahar Canga. (2021). “Design of Reversible Quantum Logic Structures in CMOS Technology.” Rochester Institute of Technology.
Abstract: Reversible logic gates have an equal number of inputs and outputs, which also makes it possible to reverse calculate and reconstruct the inputs from the outputs. Quantum logic elements are inherently reversible and requires very little energy to operate. Some of the most common uses of Quantum Computers are in the design of Convolutional Neural Networks (CNN), Deep Neural Networks (DNN) and for machine learning (ML) purposes. In this research, the reversible logic gates were designed with 45ηm CMOS technology modeled after reversible quantum logic gates. As a proof of concept, hardware that provided Sigmoid Neuron Functionality was carried out by processing the MNIST Dataset, a handwritten digit database for number recognition.
Publisher Site: RIT Repository
PDF (Requires Sign-In): PDF
Click for Bibtex
@book{canga2021design, title = {Design of Reversible Quantum Logic Structures in CMOS Technology}, author = {Canga, Bahar}, year = {2021}, school = {Rochester Institute of Technology} }
[T-2021-24] Suhasa Prabhu Kandikere. (2021). “System Integration of a Tour Guide Robot.” Rochester Institute of Technology.
Abstract: In today's world, people visit many attractive places. On such an occasion, It is of utmost importance to be accompanied by a tour guide, who is known to explain about the cultural and historical importance of places. Due to the advancements in technology, smartphones today have the capability to help a person navigate to any place in the world and can itself act as a tour guide by explaining a significance of a place. However, the person while looking into his phone might not watch his/her step and might collide with other moving person or objects. With a phone tour guide, the person is alone and is missing a sense of contact with other travelers. therefore a human guide is necessary to provide tours for a group of visitors. However, Human tour guides might face tiredness, distraction, and the effects of repetitive tasks while providing tour service to visitors. Robots eliminate these problems and can provide tour consistently until it drains its battery. This experiment introduces a tour-guide robot that can be used on such an occasion. Tour guide robots can navigate autonomously in a known map of a given place and at the same time interact with people. The environment is equipped with artificial landmarks. Each landmark provides information about that specific region. An Animated avatar is simulated on the screen. IBM Watson provides voice recognition and text-to-speech services for human-robot interaction.
Publisher Site: RIT Repository
PDF (Requires Sign-In): PDF
Click for Bibtex
@book{kandikere2021system, title = {System Integration of a Tour Guide Robot}, author = {Kandikere, Suhasa Prabhu}, year = {2021}, school = {Rochester Institute of Technology} }
[T-2021-23] Rohan Pattnaik. (2021). “Automating the measurements of galaxy redshifts and ISM properties using CNN.” Rochester Institute of Technology.
Abstract: Studying the effects that the local environments of galaxies have on their interstellar medium (ISM) properties is crucial for understanding galaxy evolution and large scale structure of the universe. In order to do that we need precise measurements of ISM properties like Star Formation Rate (SFR), metallicity (Z), ionization parameter (U), gas pressure, and extinction. Accurate estimation of redshift and emission line fluxes from a galaxy's spectrum is the first step in measuring these ISM properties. Current techniques for these measurements still rely on time-consuming manual efforts or error-prone cross-correlation codes that are already struggling to process the vast quantities of spectroscopic data that currently exist. With future NASA missions like JWST, Euclid, Roman, and SPHEREx expected to produce even larger amounts of spectroscopic data, a fast and reliable alternative to the current techniques of spectroscopic measurements is the need of the hour. To that end, we train a Convolutional Neural Network (CNN) to estimate redshift directly from an input spectrum. We generate a library of synthetic spectra spanning a wide range of parameter values and use it to train the CNN and later evaluate its performance. We obtain a normalized mean absolute deviation (NMAD) value of 0.0086 and an outlier fraction of 5.36% for our test set. This accuracy and precision is comparable to the current best photometric redshifts estimated using SED fitting codes and is lower than the subset of high quality spectroscopic data estimated using time and labour-intensive techniques. In comparison, our CNN is able to process ~30,000 spectra in around five seconds giving it an important advantage over the current methods of redshift estimation. We plan to extend this technique to estimating other ISM properties from galaxy spectra in the future.
Publisher Site: RIT Repository
PDF (Requires Sign-In): PDF
Click for Bibtex
@book{pattnaik2021automating, title = {Automating the measurements of galaxy redshifts and ISM properties using CNN}, author = {Pattnaik, Rohan}, year = {2021}, school = {Rochester Institute of Technology} }
[T-2021-22] Brandon Bogner. (2021). “Improving sub-bandgap carrier collection in GaAs solar cells by optimizing InGaAs quantum wells using strain-balancing and distributed Bragg reflectors.” Rochester Institute of Technology.
Abstract: Bulk carrier collection in GaAs solar cells is limited by the GaAs bandgap of 1.42eV, leaving low-energy photons below 1.42eV uncollected. The addition of low bandgap materials allows for the collection of these ‘unreachable' photons in a GaAs solar cell.In_(x)Ga_(1-x)As can have bandgaps between 0.354-1.42eV based on indium composition, allowing for tunable collection at longer wavelengths. Including thin 9.2nm In_(x)Ga_(1-x)As quantum wells in the i-region of a GaAs n-i-p structure enhances photon collection past the 870nm GaAs band edge, increasing current production and potentially leading to higher efficiencies. Increased current production in GaAs solar cells is especially valuable since GaAs is used as a mid-junction cell in triple junction InGaP/GaAs/Ge structures, where current-matching between the three structures is vital for reaching efficiencies above 40\% under solar concentration. To prevent accumulation of strain due to the differences in the GaAs and InGaAs lattice constants, tensile GaAs_(1-y)P_(y) barriers are added on either side of the InGaAs wells. The effects of utilizing InGaAs-GaAsP superlattices in the i-region on device performance and material quality are investigated in this work. It was found that strain-balancing quickly became necessary in devices with as few as three wells. Distributed Bragg reflectors were incorporated in the structural design to allow a second pass of photons through the wells and are shown to double the well current production without adversely affecting Voc. Decreasing the local strain between the InGaAs wells and GaAsP barriers by dropping the phosphorus composition from 32% to 10%, along with optimizing the growth conditions of the superlattice, dramatically improved the interface quality of devices grown on 2 deg. and 6 deg. offcut substrates in addition to recovering Voc above 1.00V with a 12xSBQW superlattice. Current production of the superlattice was also shown to increase from 13 microamps/well using 32%P barriers to over 30 microamps/well using 10%P barriers.
Publisher Site: RIT Repository
PDF (Requires Sign-In): PDF
Click for Bibtex
@book{bogner2021improving, title = {Improving sub-bandgap carrier collection in GaAs solar cells by optimizing InGaAs quantum wells using strain-balancing and distributed Bragg reflectors}, author = {Bogner, Brandon}, year = {2021}, school = {Rochester Institute of Technology} }
[T-2021-21] Raaga Madappa. (2021). “Semantic Segmentation and Change Detection in Satellite Imagery.” Rochester Institute of Technology.
Abstract: Processing of satellite images using deep learning and computer vision methods is needed for urban planning, crop assessments, disaster management, and rescue and recovery operations. Deep learning methods which are trained on ground-based imagery do not translate well to satellite imagery. In this thesis, we focus on the tasks of semantic segmentation and change detection in satellite imagery. A segmentation framework is presented based on existing waterfall-based modules. The proposed framework, called PyramidWASP, or PyWASP for short, can be used with two modules. PyWASP with the Waterfall Atrous Spatial Pooling (WASP) module investigates the effects of adding a feature pyramid network (FPN) to WASP. PyWASP with the improved WASP module (WASPv2) determines the effects of adding pyramid features to WASPv2. The pyramid features incorporate multi-scale feature representation into the network. This is useful for high-resolution satellite images, as they are known for having objects of varying scales. The two networks are tested on two datasets containing satellite images and one dataset containing ground-based images. The change detection method identifies building differences in registered satellite images of areas that have gone through drastic changes due to natural disasters. The proposed method is called Siamese Vision Transformers for Change Detection or SiamViT-CD for short. Vision transformers have been gaining popularity recently as they learn features well by using positional embedding information and a self-attention module. In this method, the Siamese branches, containing vision transformers with shared weights and parameters, accept a pair of satellite images and generate embedded patch-wise transformer features. These features are then processed by a classifier for patch-level change detection. The classifier predictions are further processed to generate change maps and the final predicted mask contains damage levels for all the buildings in the image. The robustness of the method is also tested by adding weather-related disturbances to satellite images.
Publisher Site: RIT Repository
PDF (Requires Sign-In): PDF
Click for Bibtex
@book{madappa2021semantic, title = {Semantic Segmentation and Change Detection in Satellite Imagery}, author = {Madappa, Raaga}, year = {2021}, school = {Rochester Institute of Technology} }
[T-2021-20] Jonathan Pereira. (2021). “Anchor Self-Calibrating Schemes for UWB based Indoor Localization.” Rochester Institute of Technology.
Abstract: Traditional indoor localization techniques that use Received Signal Strength or Inertial Measurement Units for dead-reckoning suffer from signal attenuation and sensor drift, resulting in inaccurate position estimates. Newly available Ultra-Wideband radio modules can measure distances at a centimeter-level accuracy while mitigating the effects of multipath propagation due to their very fine time resolution. Known locations of fixed anchor nodes are required to determine the position of tag nodes within an indoor environment. For a large system consisting of several anchor nodes spanning a wide area, physically mapping out the locations of each anchor node is a tedious task and thus makes the scalability of such systems difficult. Hence it is important to develop indoor localization systems wherein the anchors can self-calibrate by determining their relative positions in Euclidean 3D space with respect to each other. In this thesis, we propose two novel anchor self-calibrating algorithms - Triangle Reconstruction Algorithm (TRA) and Channel Impulse Response Positioning (CIRPos) that improve upon existing range-based implementations and solve existing problems such as flip ambiguity and node localization success rate. The localization accuracy and scalability of the self-calibrating anchor schemes are tested in a simulated environment based on the ranging accuracy of the Ultra-Wideband modules.
Publisher Site: RIT Repository
PDF (Requires Sign-In): PDF
Click for Bibtex
@book{pereira2021anchor, title = {Anchor Self-Calibrating Schemes for UWB based Indoor Localization}, author = {Pereira, Jonathan}, year = {2021}, school = {Rochester Institute of Technology} }
[T-2021-19] Ronit Damania. (2021). “Data augmentation for automatic speech recognition for low resource languages.” Rochester Institute of Technology.
Abstract: In this thesis, we explore several novel data augmentation methods for improving the performance of automatic speech recognition (ASR) on low-resource languages. Using a 100-hour subset of English LibriSpeech to simulate a low-resource setting, we compare the well-known SpecAugment augmentation approach to these new methods, along with several other competitive baselines. We then apply the most promising combinations of models and augmentation methods to three genuinely under-resourced languages using the 40-hour Gujarati, Tamil, Telugu datasets from the 2021 Interspeech Low Resource Automatic Speech Recognition Challenge for Indian Languages. Our data augmentation approaches, coupled with state-of-the-art acoustic model architectures and language models, yield reductions in word error rate over SpecAugment and other competitive baselines for the LibriSpeech-100 dataset, showing a particular advantage over prior models for the ``other'', more challenging, dev and test sets. Extending this work to the low-resource Indian languages, we see large improvements over the baseline models and results comparable to large multilingual models.
Publisher Site: RIT Repository
PDF (Requires Sign-In): PDF
Click for Bibtex
@book{damania2021data, title = {Data augmentation for automatic speech recognition for low resource languages}, author = {Damania, Ronit}, year = {2021}, school = {Rochester Institute of Technology} }
[T-2021-18] Erika Bliss. (2021). “Analyzing Temporal Patterns in Phishing Email Topics.” Rochester Institute of Technology.
Abstract: In 2020, the Federal Bureau of Investigation (FBI) found phishing to be the most common cybercrime, with a record number of complaints from Americans reporting losses exceeding $4.1 billion. Various phishing prevention methods exist; however, these methods are usually reactionary in nature as they activate only after a phishing campaign has been launched. Priming people ahead of time with the knowledge of which phishing topic is more likely to occur could be an effective proactive phishing prevention strategy. It has been noted that the volume of phishing emails tended to increase around key calendar dates and during times of uncertainty. This thesis aimed to create a classifier to predict which phishing topics have an increased likelihood of occurring in reference to an external event. After distilling around 1.2 million phishes until only meaningful words remained, a Latent Dirichlet allocation (LDA) topic model uncovered 90 latent phishing topics. On average, human evaluators agreed with the composition of a topic 74% of the time in one of the phishing topic evaluation tasks, showing an accordance of human judgment to the topics produced by the LDA model. Each topic was turned into a timeseries by creating a frequency count over the dataset's two-year timespan. This time-series was changed into an intensity count to highlight the days of increased phishing activity. All phishing topics were analyzed and reviewed for influencing events. After the review, ten topics were identified to have external events that could have possibly influenced their respective intensities. After performing the intervention analysis, none of the selected topics were found to correlate with the identified external event. The analysis stopped here, and no predictive classifiers were pursued. With this dataset, temporal patterns coupled with external events were not able to predict the likelihood of a phishing attack.
Publisher Site: RIT Repository
PDF (Requires Sign-In): PDF
Click for Bibtex
@book{bliss2021analyzing, title = {Analyzing Temporal Patterns in Phishing Email Topics}, author = {Bliss, Erika}, year = {2021}, school = {Rochester Institute of Technology} }
[T-2021-17] Sharan Vidash Vidya Shanmugham. (2021). “Impact of Image Complexity on Early Exit Neural Networks for Edge Applications.” Rochester Institute of Technology.
Abstract: The advancement of deep learning methods has ushered in novel research in the field of computer vision as the success of deep learning methods are irrefutable when it comes to images and video data. However deep learning methods such as convolutional neural networks are computationally heavy and need specialized hardware to give results within a reasonable time. Early-exit neural networks offer a solution to reducing computational complexity by placing exits in traditional networks bypassing the need to compute the output of all convolutional layers. In this thesis, a reinforcement learning-based exit selection algorithm for early-exit neural networks is analyzed. The exit selection algorithm receives information about the previous state of the early-exit network to make decisions during runtime. The state of the early-exit network is determined by the previously achieved accuracy and inference time. A novel feature is proposed to improve the performance of the reinforcement learning network to make better decisions. The feature is based on the input image and attempts to quantify the complexity of every single input. The impacts of adding the new feature and potential performance improvements are documented. The testing is performed on two image classification datasets to record any variance in performance with the dataset. A scenario with the computation of the exit selection algorithm offloaded to a local edge server is also investigated by creating a simple analytical edge computing model. The decision-making rate is varied in this scenario and the potential differences in performance are documented.
Publisher Site: RIT Repository
PDF (Requires Sign-In): PDF
Click for Bibtex
@book{vidya shanmugham2021impact, title = {Impact of Image Complexity on Early Exit Neural Networks for Edge Applications}, author = {Vidya Shanmugham, Sharan Vidash}, year = {2021}, school = {Rochester Institute of Technology} }
[T-2021-16] Divyansh Gupta. (2021). “HandyPose and VehiPose: Pose Estimation of Flexible and Rigid Objects.” Rochester Institute of Technology.
Abstract: Pose estimation is an important and challenging task in computer vision. Hand pose estimation has drawn increasing attention during the past decade and has been utilized in a wide range of applications including augmented reality, virtual reality, human-computer interaction, and action recognition. Hand pose is more challenging than general human body pose estimation due to the large number of degrees of freedom and the frequent occlusions of joints. To address these challenges, we propose HandyPose, a single-pass, end-to-end trainable architecture for hand pose estimation. Adopting an encoder-decoder framework with multi-level features, our method achieves high accuracy in hand pose while maintaining manageable size complexity and modularity of the network. HandyPose takes a multi-scale approach to representing context by incorporating spatial information at various levels of the network to mitigate the loss of resolution due to pooling. Our advanced multi-level waterfall architecture leverages the efficiency of progressive cascade filtering while maintaining larger fields-of-view through the concatenation of multi-level features from different levels of the network in the waterfall module. The decoder incorporates both the waterfall and multi-scale features for the generation of accurate joint heatmaps in a single stage. Recent developments in computer vision and deep learning have achieved significant progress in human pose estimation, but little of this work has been applied to vehicle pose. We also propose VehiPose, an efficient architecture for vehicle pose estimation, based on a multi-scale deep learning approach that achieves high accuracy vehicle pose estimation while maintaining manageable network complexity and modularity. The VehiPose architecture combines an encoder-decoder architecture with a waterfall atrous convolution module for multi-scale feature representation. It incorporates contextual information across scales and performs the localization of vehicle keypoints in an end-to-end trainable network. Our HandyPose architecture has a baseline of vehipose with an improvement in performance by incorporating multi-level features from different levels of the backbone and introducing novel multi-level modules. HandyPose and VehiPose more thoroughly leverage the image contextual information and deal with the issue of spatial loss of resolution due to successive pooling while maintaining the size complexity, modularity of the network, and preserve the spatial information at various levels of the network. Our results demonstrate state-of-the-art performance on popular datasets and show that HandyPose and VehiPose are robust and efficient architectures for hand and vehicle pose estimation.
Publisher Site: RIT Repository
PDF (Requires Sign-In): PDF
Click for Bibtex
@book{gupta2021handypose, title = {HandyPose and VehiPose: Pose Estimation of Flexible and Rigid Objects}, author = {Gupta, Divyansh}, year = {2021}, school = {Rochester Institute of Technology} }
[T-2021-15] Pradumna Suryawanshi. (2021). “Predicting risk of readmission in heart failure patients using electronic health records.” Rochester Institute of Technology.
Abstract: This thesis research investigates the prediction of readmission risk in heart failure patients using their electronic health record (EHR) data from previous hospitalizations. We examine three primary questions. First, we study the use of attention mechanism in readmission prediction model based on long short-term memory(LSTM) networks and investigate the interpretability it offers regarding the importance of critical time during the visit in readmission prediction. Second given that, generally dataset is curated by combining data from multiple hospitals we investigate model generalization across multiple sites. Finally since in real life scenario model will be trained on past data and used to predict future readmission events, we further investigate model generalization across time. Along with those things, model performance across different endpoints will be studied.
Publisher Site: RIT Repository
PDF (Requires Sign-In): PDF
Click for Bibtex
@book{suryawanshi2021predicting, title = {Predicting risk of readmission in heart failure patients using electronic health records}, author = {Suryawanshi, Pradumna}, year = {2021}, school = {Rochester Institute of Technology} }
[T-2021-14] Zachariah Wigent. (2021). “Test Naming Failures. An Exploratory Study of Bad Naming Practices in Test Code.” Rochester Institute of Technology.
Abstract: Unit tests are a key component during the software development process, helping ensure that a developer's code is functioning as expected. Developers interact with unit tests when trying to understand, maintain, and when updating code. Good test names are essential for making these various processes easier, which is important considering the substantial costs and effort of software maintenance. Despite this, it has been found that the quality of test code is often lacking, specifically when it comes to test names. When a test fails, its name is often the first thing developers will see when trying to fix the failure, therefore it is important that names are of high quality in order to help with the debugging process. The objective of this work was to find anti-patterns having to do with test method names that may have a negative impact on developer comprehension. In order to do this, a grounded theory study was conducted on 12 open-source Java and C# GitHub projects. From this dataset, many patterns were discovered to be common throughout the test code. Some of these patterns fit the necessary criteria of anti-patterns that would probably hinder developer comprehension. With the avoidance of these anti-patterns it is believed that developers will be able to write better test names that can help speed the time to debug errors as test names will be more comprehensive.
Publisher Site: RIT Repository
PDF (Requires Sign-In): PDF
Click for Bibtex
@book{wigent2021test, title = {Test Naming Failures. An Exploratory Study of Bad Naming Practices in Test Code}, author = {Wigent, Zachariah}, year = {2021}, school = {Rochester Institute of Technology} }
[T-2021-13] Irfan Rafi Punekar. (2021). “Measuring Shadows: FPGA-based image sensor control systems for next-generation NASA missions.” Rochester Institute of Technology.
Abstract: Astronomy and astrophysics are fields of constant growth and exploration, and discoveries are being made every day. Behind each discovery, however, is the equipment and engineering that makes that science possible. The science and engineering go hand in hand in two ways: advances in engineering make new scientific discoveries possible, and new scientific questions create the need for more advanced engineering. The work that led to this thesis is an example of the latter statement. The big-picture goal is to support the development of next-generation detectors such as the Quanta Image Sensor (QIS), a gigapixel-scaleble Complementary Metal-Oxide Semiconductor (CMOS) photon-number resolving image sensor. This thesis focuses on one crucial part of the development process: the characterisation of the QIS. In order to advance the NASA Technology Readiness Level (TRL) from three to four, the detector needs to undergo extensive laboratory and telescope environment testing. The testing framework is being run by an FPGA hardware design that includes a processor, and this set of hardware and software is responsible for operating the detector, managing experiment parameters, running experiments, and collecting resultant data and passing it to a host PC. The majority of the work of this portion of the project revolved around creating, improving, and testing the framework to allow for fully functional and automated detector characterisation. Test systems already exist for the QIS in a room temperature environment, as well as for current-generation image sensors in cryogenic vacuum environments. However, there is no existing test system that allows the QIS to be tested in a cryo-vac environment. This thesis details a functional system that fills that niche. The system is built to be modular and extensible so that it can be expanded upon to characterise other types of detectors in the future as well. For now, however, the system shines as the only one that allows the QIS to be tested in an environment that simulates its behaviour in outer space.
Publisher Site: RIT Repository
PDF (Requires Sign-In): PDF
Click for Bibtex
@book{punekar2021measuring, title = {Measuring Shadows: FPGA-based image sensor control systems for next-generation NASA missions}, author = {Punekar, Irfan Rafi}, year = {2021}, school = {Rochester Institute of Technology} }
[T-2021-12] Sahil Anande. (2021). “Neural Network Models for TCP - SYN Flood Denial of Service Attack Detection With Source and Destination Anonymization.” Rochester Institute of Technology.
Abstract: The Internet has become a necessity in today's digital world, and making it secure is a pressing concern. Hackers are investing ever-increasing efforts to compromise Internet nodes with novel techniques. According to Forbes, every minute, $ 2,900,000 is lost to cybercrime. A common cyber-attack is Denial of Service (DoS) or Distributed Denial of Service (DDoS) attacks, which may bring a network to a standstill, and unless mitigated, network services could be halted for an extended period. The attack can occur at any layer of the OSI model. This thesis focuses on SYN Flood DoS/DDoS attacks, also known as TCP Flood attacks, and studies the use of artificial neural networks to detect the attacks. Specific neural network models used in this thesis are the Gated Recurrent Units (GRU), the Long Short-Term Memory (LSTM), and a semi-supervised model on label propagation. All neural network models detect attacks by analyzing the individual hexadecimal values in the packet header. A novelty of the approach followed in this thesis is that the neural networks do not consider the lexical values of the network packet (MAC addresses, IP addresses, and port numbers) as input features in their traffic analysis. Instead, the neural network models are designed and trained to detect malicious traffic based on the time pattern of TCP flags. The neural networks base their analysis of traffic on time-sequenced patterns. An important hyperparameter discussed in this paper is the size of the lookup window, that is, the number of past packets the model can access to predict the next packet. Evaluation results based on datasets presented in this thesis show that the accuracies of the GRU, CNN/LSTM, and label propagation models are 81%, 93%, and 96%, respectively.
Publisher Site: RIT Repository
PDF (Requires Sign-In): PDF
Click for Bibtex
@book{anande2021neural, title = {Neural Network Models for TCP - SYN Flood Denial of Service Attack Detection With Source and Destination Anonymization}, author = {Anande, Sahil}, year = {2021}, school = {Rochester Institute of Technology} }
[T-2021-11] Robery Ormal Chancia. (2021). “Toward improved crop management using spectral sensing with unmanned aerial systems.” Rochester Institute of Technology.
Abstract: Remote sensing applications in agriculture are established for large-scale monitoring with satellite and airborne imagery, but unmanned aerial systems (UAS) are poised to bring in-field mapping capabilities to the hands of individual farmers. UAS imaging holds several advantages over traditional methods, including centimeter-scale resolution, reduced atmospheric absorption, flexible timing of data acquisitions, and ease of use. In this work, we present two studies using UAS imaging of specialty crops in upstate New York to work towards improved crop management applications. The first study is an investigation of multispectral imagery obtained over table beet fields in Batavia, NY during the 2018 and 2019 seasons to be used in root yield modeling. We determined optimal growth stages for future observations and establish the importance of quantifying early growth via determination of canopy area, a feature unattainable with lower resolution imaging. We developed models for root mass and count based on area-augmented imagery of our raw study plots and their corresponding ground truth data for practical testing with independent data sets. The second study was designed to determine an optimal subset of wavelengths derived from hyperspectral imagery that are related to grapevine nutrients for improved vineyard nutrient monitoring. Our ensemble wavelength selection and regression algorithm chose wavelengths consistent with known absorption features related to nitrogen content in vegetation. Our model achieved a leave-one-out cross-validation root-mean-squared error of 0.17% nitrogen in our dried vine-leaf samples with 2.4-3.6% nitrogen. This is an improvement upon published studies of typical UAS multispectral sensors used to assess grapevine nitrogen status. With further testing on new data, we can determine consistently selected wavelengths and guide the design of specialty multispectral sensors for improved grapevine nutrient management.
Publisher Site: RIT Repository
PDF (Requires Sign-In): PDF
Click for Bibtex
@book{chancia2021toward, title = {Toward improved crop management using spectral sensing with unmanned aerial systems}, author = {Chancia, Robery Ormal}, year = {2021}, school = {Rochester Institute of Technology} }
[T-2021-10] Federico Rueda. (2021). “Continuous Variable Quantum Compilation Analysis.” Rochester Institute of Technology.
Abstract: Over the last few years quantum computers have rose to prominence as a solution for increasing computing power and tackling problems intractable by classical computers. Classical computers have struggled to meet the ever-increasing demand for data processing and modeling. Thus, quantum computers would meet the demand for heavy computing tasks that classical computers could never achieve. Today there exist two promising quantum technologies that have the potential to prove quantum supremacy in the near future: super-conducting qubits and Continuous Variable (CV) model. Out of the two, superconducting qubits have been at the forefront of quantum computing research. NISQ (Noise intermediate-scale quantum) are the existing superconducting qubit computers, and are defined by having a small number of qubits with high inaccuracies due to quantum noise. The CV approach utilize photons in Gaussian states, qumodes, as the main processing unit. CV-based quantum computers present similar computation benefits to superconducting qubit devices. The ease of manufacturing and operation -- due to being able to operate at room temperature -- of CV-based quantum computers make it a likely candidate for wide adoption and accessibility compared to superconducting qubit systems. But because of the underlying differences in the two technologies, research and development of their software stacks have differed greatly, with a lack-thereof for CV devices. The goal of this thesis is to explain and analyze the compilers implemented by Strawberry Fields -- cross platform python library to simulate and execute programs on photonic hardware -- and propose an additional compilation step that will enable future, more flexible hardware implementations.
Publisher Site: RIT Repository
PDF (Requires Sign-In): PDF
Click for Bibtex
@book{rueda2021continuous, title = {Continuous Variable Quantum Compilation Analysis}, author = {Rueda, Federico}, year = {2021}, school = {Rochester Institute of Technology} }
[T-2021-09] Joseph A. Carrock. (2021). “An Experimental Campaign to Collect Multiresolution Hyperspectral Data with Ground Truth.” Rochester Institute of Technology.
Abstract: Advancements in hyperspectral imaging systems, data processing, and collection methods, have allowed for comprehensive spectral assessment of terrestrial objects to assist in a vast array of imaging problems previously deemed too complex. As the field evolves and more research is completed, the boundaries of what is capable are constantly pushed to new thresholds. At this junction, there exists an opportunity for the capabilities of these new creations to exceed our ability to prove their efficiency and efficacy. One area where this is true, is in the assessment of new hyperspectral panchromatic sharpening (HPS) algorithms. This is due to the lack of a true multi-resolution hyperspectral data focused on the same scene, which is needed to properly test these algorithms. Typically, the assessment process awaiting a HPS algorithm starts by taking a high-resolution Hyperspectral Image (HSI), which is then spatially degraded so that after the sharpening process, the result can be compared to the original and analyzed for accuracy. Presentations featuring the results of such algorithms often lead to immediate questions about quantitative assessments based solely on simulated low-resolution data. To address this problem, a collection experiment seeking to create a multi-resolution hyperspectral data set was designed and completed in Henrietta, NY on July 24th, 2020. Imagery of 48 felt targets, ranging in size from 5 cm to 30 cm and in six different colors, was collected in three resolutions by the Rochester Institute of Technology (RIT) MX-1 sUAS imaging system. In addition to a high resolution 5-band frame camera, the MX-1 utilizes a 272-band hyperspectral line imager that features a silicon detector, 1.88cm GSD at 30m flight altitude, and is responsive from approximately 400nm to 1000nm. To create the desired multi-resolution imagery, three flights were performed, and images of the target scene were collected at flight heights of 30m, 60m, and 120m. The resulting imagery possesses ratios of 2:1 and 4:1 spatial resolution relative to the lowest altitude flight. Use of this data in the evaluation process of HPS algorithms ensures that the radiometric accuracy of the result and the effectiveness of the sharpening method employed can be better ascertained through quantitative analysis of the inputs and output after being applied to this non-simulated multiresolution hyperspectral data set. During planning and after collection, care was taken to rigorously document the data set, its structure, and features, as the intention exists for the data to be used for various purposes long exceeding the extent of its immediate usefulness to researchers at RIT. Additional documentation provided as ancillary metadata describes the spectral signatures of all non-environmental materials present in the HSI target scene, the process of creating both the targets and their layout, as well as the collection plan, the data itself, post processing steps, and preliminary experiments undertaken to improve end-user understanding and ease of use. This documentation also includes an assessment surmised through understanding the flaws present within the data, and portions of the collection plan which did not lead to the intended outcome. Because of the efforts described in this document, there now exists a multi-resolution hyperspectral data set of a known target scene with highly documented ground truth. This data and report will prove useful in the assessment of HPS algorithms currently under development, but also has the potential to assist as useful test data for various applications within the fields of hyperspectral imaging and remote sensing.
Publisher Site: RIT Repository
PDF (Requires Sign-In): PDF
Click for Bibtex
@book{carrock2021an, title = {An Experimental Campaign to Collect Multiresolution Hyperspectral Data with Ground Truth}, author = {Carrock, Joseph A.}, year = {2021}, school = {Rochester Institute of Technology} }
[T-2021-08] Yuan Zheng. (2021). “Underwater.” Rochester Institute of Technology.
Abstract: This thesis seeks to highlight the importance of work-life balance to our mental health. By experiencing an imbalanced life from the first-person view, this thesis focuses on using an abstract visual style to present the character's inner world and the desperation for relaxation.
Publisher Site: RIT Repository
PDF (Requires Sign-In): PDF
Click for Bibtex
@book{zheng2021underwater, title = {Underwater}, author = {Zheng, Yuan}, year = {2021}, school = {Rochester Institute of Technology} }
[T-2021-07] Christopher Thomas Enoch. (2021). “Calculating Common Vulnerability Scoring System’s Environmental Metrics Using Context-Aware Network Graphs.” Rochester Institute of Technology.
Abstract: Software vulnerabilities have significant costs associated with them. To aid in the prioritization of vulnerabilities, analysts often utilize Common Vulnerability Scoring System's Base severity scores. However, the Base scores provided from the National Vulnerability Database are subjective and may incorrectly convey the severity of the vulnerability in an organization's network. This thesis proposes a method to statically analyze context-aware network graphs to increase accuracy of CVSS severity scores. Through experimentation of the proposed methodology, it is determined that context-aware network graphs can capture the required metrics to generate modified severity scores. The proposed approach has some accuracy to it, but leaves room for additional network context to further refine Environmental severity scores.
Publisher Site: RIT Repository
PDF (Requires Sign-In): PDF
Click for Bibtex
@book{enoch2021calculating, title = {Calculating Common Vulnerability Scoring System's Environmental Metrics Using Context-Aware Network Graphs}, author = {Enoch, Christopher Thomas}, year = {2021}, school = {Rochester Institute of Technology} }
[T-2021-06] Gina Kersey. (2021). “Modeling the mechanistic behavioral logic supporting adherence to hormone therapy in breast cancer patients.” Rochester Institute of Technology.
Abstract: This project proposed that given a basic model of the decisional logic driving stable adherence it might be possible to reliably anticipate increased vulnerability to discontinuation and use such a mode to design behavioral interventions that are specifically tailored to deliver course corrections that are temporally and contextually optimal. A logistic regression was coded and run using the core model predicted by the experts as a base for how the variables interact to make predictions. Then a logistic regression with stepwise selection was coded and run using the core model as a base for how the variables interact and to allow for variables to be added to make the prediction more accurate. The logistic regression with stepwise selection produced both an augmented core model and a de novo model. There were two nodes that had a variable added over 75% of the time to the augmented core. These nodes were health literacy and treatment fatigue. The accuracy for the core model, augmented core model, and de novo model were all accurate with respective overall accuracies being 63.5%, 66.3%, and 67.4%. However, it is important to note that we were able to outperform the expert model by doing the logistic regression with stepwise selection. The results show that the causal interaction diagram predicted by the experts was fairly accurate. These results then can be used to further the research needed to make a program that will help predict the chances of adherence and to be able help doctors work with the patients to stay adherent.
Publisher Site: RIT Repository
PDF (Requires Sign-In): PDF
Click for Bibtex
@book{kersey2021modeling, title = {Modeling the mechanistic behavioral logic supporting adherence to hormone therapy in breast cancer patients}, author = {Kersey, Gina}, year = {2021}, school = {Rochester Institute of Technology} }
[T-2021-05] David Smith. (2021). “Effect of Nanoparticle and Acoustic Assisted Spalling on III-V Thin Film Photovoltaic Device Characteristics.” Rochester Institute of Technology.
Abstract: Thin film III-V photovoltaics (PV) are a high efficiency, low weight alternative to silicon. However, high costs make practical use cases limited to weight specific applications. These high costs are largely contained in material costs and especially thick substrates used as seed and handle layers for devices grown atop them. Removal and reuse of the substrates post device growth leads to a significant reduction in the material cost to make these devices. Acoustic assisted spalling (Sonic Wafering) is a low-cost substrate removal method that has the potential to significantly reduce costs related to the fabrication of III-V PVs. This thesis outlines design considerations and material characterization comparison of III-V devices made conventionally without substrate removal with those made using acoustic assisted spalling. The viability of SiOx nanoparticles as a release assist layer is also investigated. This investigation was in collaboration with researchers at Arizona State University (ASU), researchers at the National Renewable Energy Laboratory (NREL), and in conjunction with Crystal Sonic, all sponsored by the DOE under grant number DE-FOA-0002064. 3-4% surface coverage of nanoparticles prior to test structure overgrowth leads to small decreases in photoluminescence (80% of control) whereas 30% surface coverage drastically decreases photoluminescence (
Publisher Site: RIT Repository
PDF (Requires Sign-In): PDF
Click for Bibtex
@book{smith2021effect, title = {Effect of Nanoparticle and Acoustic Assisted Spalling on III-V Thin Film Photovoltaic Device Characteristics}, author = {Smith, David}, year = {2021}, school = {Rochester Institute of Technology} }
[T-2021-04] Sridevi Kayyur Subramanya. (2021). “Deep Learning Models to Characterize Smooth Muscle Fibers in Hematoxylin and Eosin Stained Histopathological Images of the Urinary Bladder.” Rochester Institute of Technology.
Abstract: Muscularis propria (MP) and muscularis mucosa (MM), two types of smooth muscle fibers in the urinary bladder, are major benchmarks in staging bladder cancer to distinguish between muscle-invasive (MP invasion) and non-muscle-invasive (MM invasion) diseases. While patients with non-muscle-invasive tumor can be treated conservatively involving transurethral resection (TUR) only, more aggressive treatment options, such as removal of the entire bladder, known as radical cystectomy (RC) which may severely degrade the quality of patient's life, are often required in those with muscle-invasive tumor. Hence, given two types of image datasets, hematoxylin & eosin-stained histopathological images from RC and TUR specimens, we propose the first deep learning-based method for efficient characterization of MP. The proposed method is intended to aid the pathologists as a decision support system by facilitating accurate staging of bladder cancer. In this work, we aim to semantically segment the TUR images into MP and non-MP regions using two different approaches, patch-to-label and pixel-to-label. We evaluate four different state-of-the-art CNN-based models (VGG16, ResNet18, SqueezeNet, and MobileNetV2) and semantic segmentation-based models (U-Net, MA-Net, DeepLabv3+, and FPN) and compare their performance metrics at the pixel-level. The SqueezeNet model (mean Jaccard Index: 95.44%, mean dice coefficient: 97.66%) in patch-to-label approach and the MA-Net model (mean Jaccard Index: 96.64%, mean dice coefficient: 98.29%) in pixel-to-label approach are the best among tested models. Although pixel-to-label approach is marginally better than the patch-to-label approach based on evaluation metrics, the latter is computationally efficient using least trainable parameters.
Publisher Site: RIT Repository
PDF (Requires Sign-In): PDF
Click for Bibtex
@book{subramanya2021deep, title = {Deep Learning Models to Characterize Smooth Muscle Fibers in Hematoxylin and Eosin Stained Histopathological Images of the Urinary Bladder}, author = {Subramanya, Sridevi Kayyur}, year = {2021}, school = {Rochester Institute of Technology} }
[T-2021-03] Aditya Gupta. (2021). “Literature-Assisted Validation of a Novel Causal Inference Graph in a Sparsely Sampled Multi-Regimen Exercise Data.” Rochester Institute of Technology.
Abstract: Background. Causal mechanisms supporting the cardio-metabolic benefits of exercise can be identified for individuals who cannot exercise. With the use of appropriate causal discovery algorithms, the causal pathways can be found for even sparsely sampled data which will help direct drug discovery and pharmaceutical industries to create the appropriate drug to maintain muscles. Objective. The purpose of this study was to infer novel causal source-target interactions active in sparsely sampled data and embed these in a broader causal network extracted from the literature to test their alignment with community-wide prior knowledge and their mechanistic validity in the context of regulatory feedback dynamics. Methods. To this goal, emphasis was placed on the female STRRIDE1/PD dataset to see how the observed data predicts a Causal Directed Acyclic Graph (C-DAG). The analytes in the dataset with greater than 5 missing values were dropped from further analysis to retain a higher confidence among the graphs. The PC, named after its authors Peter and Clark, algorithm was executed for ten thousand iterations on randomly sampled columns of the modified dataset keeping intensity and amount constant as the first two columns to see their effect on the resultant DAG. Out of the 10,000 iterations, interactions that appeared more than 45%, 50%, 65%, 75% and 100% were observed. The interactions that appeared more than 50% of the times were then compared to the literature mined dataset using MedScan Natural Language Processing (NLP) techniques as a part of Pathway Studio. Results. Full consensus across all sub-sampled networks produced 136 interactions that were fully conserved. Of these 136 interactions, 64 were resolved as direct causal interactions, 5 were not direct causal interactions and 67 could only be described as associative. It was found that about 17% of the interactions were recovered from the text mining of the 285 peer-reviewed journals from a total of 64 that were predicted at a 50% consensus. Out of these 11, 4 were completely recovered whereas 7 were only partially recovered. A completely recovered interaction was LDL → ApoB and a partially recovered interaction was HDL → insulin sensitivity. Conclusion. Only 17% of the predicted interactions were found through literature mining, remaining 83% were a mix of novel interactions and self-interactions that need to be worked on further. Of the remaining interactions, 53 remain novel and give insight into how different clinical parameters interact with the cholesterol molecules, biological markers and how they interact with each other.
Publisher Site: RIT Repository
PDF (Requires Sign-In): PDF
Click for Bibtex
@book{gupta2021literature-assisted, title = {Literature-Assisted Validation of a Novel Causal Inference Graph in a Sparsely Sampled Multi-Regimen Exercise Data}, author = {Gupta, Aditya}, year = {2021}, school = {Rochester Institute of Technology} }
[T-2021-02] Udit Sharma. (2021). “GourmetNet: Food Segmentation Using Multi-Scale Waterfall Features With Spatial and Channel Attention.” Rochester Institute of Technology.
Abstract: Deep learning and Computer vision are extensively used to solve problems in wide range of domains from automotive and manufacturing to healthcare and surveillance. Research in deep learning for food images is mainly limited to food identification and detection. Food segmentation is an important problem as the first step for nutrition monitoring, food volume and calorie estimation. This research is intended to expand the horizons of deep learning and semantic segmentation by proposing a novel single-pass, end-to-end trainable network for food segmentation. Our novel architecture incorporates both channel attention and spatial attention information in an expanded multi-scale feature representation using the WASPv2 module. The refined features will be processed with the advanced multi-scale waterfall module that combines the benefits of cascade filtering and pyramid representations without requiring a separate decoder or postprocessing.
Publisher Site: RIT Repository
PDF (Requires Sign-In): PDF
Click for Bibtex
@book{sharma2021gourmetnet:, title = {GourmetNet: Food Segmentation Using Multi-Scale Waterfall Features With Spatial and Channel Attention}, author = {Sharma, Udit}, year = {2021}, school = {Rochester Institute of Technology} }
[T-2021-01] Hitesh Ulhas Mangala Vaidya. (2021). “Reducing Catastrophic Forgetting in Self-Organizing Maps.” Rochester Institute of Technology.
Abstract: An agent that is capable of continual or lifelong learning is able to continuously learn from potentially infinite streams of pattern sensory data. One major historic difficulty in building agents capable of such learning is that neural systems struggle to retain previously-acquired knowledge when learning from new data samples. This problem is known as catastrophic forgetting and remains an unsolved problem in the domain of machine learning to this day. To overcome catastrophic forgetting, different approaches have been proposed. One major line of thought advocates the use of memory buffers to store data where the stored data is then used to randomly retrain the model to improve memory retention. However, storing and giving access to previous physical data points results in a variety of practical difficulties particularly with respect to growing memory storage costs. In this work, we propose an alternative way to tackle the problem of catastrophic forgetting, inspired by and building on top of a classical neural model, the self-organizing map (SOM) which is a form of unsupervised clustering. Although the SOM has the potential to combat forgetting through the use of pattern-specializing units, we uncover that it too suffers from the same problem and this forgetting becomes worse when the SOM is trained in a task incremental fashion. To mitigate this, we propose a generalization of the SOM, the continual SOM (c-SOM), which introduces several novel mechanisms to improve its memory retention -- new decay functions and generative resampling schemes to facilitate generative replay in the model. We perform extensive experiments using split-MNIST with these approaches, demonstrating that the c-SOM significantly improves over the classical SOM. Additionally, we come up with a new performance metric alpha_mem to measure the efficacy of SOMs trained in a task incremental fashion, providing a benchmark for other competitive learning models.
Publisher Site: RIT Repository
PDF (Requires Sign-In): PDF
Click for Bibtex
@book{mangala vaidya2021reducing, title = {Reducing Catastrophic Forgetting in Self-Organizing Maps}, author = {Mangala Vaidya, Hitesh Ulhas}, year = {2021}, school = {Rochester Institute of Technology} }
[T-2020-50] Brendan Wells. (2020). “Using Classification for Analysis of Multi-Modal Video Summarization.” Rochester Institute of Technology.
Abstract: Video Summarization refers to taking the important contents of a video and condensing it down to an easily consumable piece of data without having to watch the entire video. Currently, Millions of Videos are being recorded and shared every day. These videos range from the consumer level, such as a birthday party or wedding video, all the way up to industry such as film and television. We have constructed a model that seeks to address the problem of not being able to consume all the media that is being presented to you because of time constraints. To do this, we conduct two separate experiments. The first experiment examines the role of different parts of the summarization model, namely modality, sampling rate, and data scaling so that we better understand how summaries are generated. The second experiment utilizes these findings to create a model based in classification. We use classification as a means of interpreting a wide variety of types of video for summarization. By using classification to generate the video and audio features used by the summarizer, the classifier granularity is leveraged, and the maturity of classification problems is leveraged to accomplish a summarization task. We found that while scaling and sampling of the data have little effect on the overall summary, in each experiment the modality played a large role in the results. While many models exclude audio, we found that there are benefits to including this data when generating a video summary. We also found that the use of classification resulted in a separation of impacts for each modality, with video serving to construct the shape of the summary and audio determining importance score.
Publisher Site: RIT Repository
PDF (Requires Sign-In): PDF
Click for Bibtex
@book{wells2020using, title = {Using Classification for Analysis of Multi-Modal Video Summarization}, author = {Wells, Brendan}, year = {2020}, publisher = {Rochester Institute of Technology} }
[T-2020-49] Douglas Bean. (2020). “Gain Enhancement of On-Chip Wireless interconnects at 60 GHz Using an Artificial Magnetic Conductor.” Rochester Institute of Technology.
Abstract: The motivation for this work comes from the increased demand for short range high frequency data communication within and between integrated circuit (IC) chips. The use of wireless interconnects introduces flexibility to the circuit design, reduces power consumption and production costs, since the antennas can be integrated into a standard CMOS process. These findings have been well noted in literature. In addition, wireless interconnects operating in the mm-wave frequency range, at 60GHz, allow for a high data rate of over 1Gb/s for short range of transmissions. The drawback of wireless interconnects operating at high frequencies is the distortion in the radiation pattern caused by the silicon substrate inherent in a standard CMOS process. The high permittivity and a low resistivity of silicon in a CMOS process introduce radiation losses. These losses distort the radiating signal, reducing the directive gain and the antenna efficiency. The objective of the work is to enhance antenna gain and improve the radiation efficiency with the use of a Jerusalem-Cross Artificial Magnetic Conductor (AMC). The Jerusalem Cross AMC can mitigate the effects of the silicon and improve data transmission for inter-Chip data communications. A Yagi antenna was optimized for end-fire radiation in the plane of the chip. It's performance was studied when it was placed in the center and along the front edge of a standard 10mm by 10mm chip, with the AMC layer extending only below the feed system, Partial AMC, and then compared when it extends under the entire antenna, Full AMC. To examine the transmission characteristics two chips were placed facing one another, on an FR4 slab, with the antennas first placed at the front edges of both chips then in the center of their respective chips. For direct comparison a third configuration was made with one antenna in the center of a chip and the other at the edge of the second chip. The performance of this inter-chip transmission was examined with the three AMC layer configurations: No AMC, Partial AMC, and Full AMC. All simulations were performed using ANSYS HFSS. The results show that the partial AMC improves the performance of the Yagi antenna when it was placed at the front edge of the chip facing out. The directive gain (Endfire direction) with the partial AMC was increased by 0.79 dB or 46% when compared to the antenna without an AMC. The radiation efficiency increased from 39% to 45%. When examining the antenna in the center of the larger substrate the full AMC layer improved performance. The directive gain increased by 0.93 dB or 5%. The full AMC layer improved the directional gain of the antenna in the center of the chip because it is more susceptible to the effects of the silicon substrate. Whereas when placed at the edge of the chip the antenna is mainly radiating in free space and not as influenced by the losses due to the silicon. Which is why the partial AMC improves radiation performance for the antenna placed at the edge of the chip. This is more clearly shown by the transmission results. When both antennas were placed at the front edges of their respective chips with full AMC layers the gain increased by 11% and the radiation efficiency increases by 12%; while when both antennas are placed in the center the directive gain increases by 26% and the radiation efficiency increases by only 2%. In the model with one antenna at the front edge of the first chip and the other antenna in the center of the second chip the full AMC improved the directive gain by 12% and 29% respectively. Both results show that the full AMC has a positive effect on the directive gain of the antenna, especially when placed in the center of the substrate.
Publisher Site: RIT Repository
PDF (Requires Sign-In): PDF
Click for Bibtex
@book{bean2020gain, title = {Gain Enhancement of On-Chip Wireless interconnects at 60 GHz Using an Artificial Magnetic Conductor}, author = {Bean, Douglas}, year = {2020}, school = {Rochester Institute of Technology} }
[T-2020-48] Nathan Albert Schuler. (2020). “Physical Activity in Aging: Biological Outcomes, Functional Autonomy, and Policy Implications.” Rochester Institute of Technology.
Abstract: A 2016 study concluded that the elderly spend 2.5 times the national average on health services (De Nardi 2016). Despite consisting of only 14 percent of the United States population, the elderly accounted for 34 percent of all medical spending, and accounted for 73 percent of all deaths (De Nardi 2016). In addition, current projections estimate that with the aging of the baby boomer generation, the percentage of the population that is older than 65 will swell to approximately 20 percent of the population by 2030 (Ortman 2014). If the medical spending holds, this will coincide with massive increases in health service utilization and costs. Costs of health services are currently a contentious topic at both the state and federal levels, with a seemingly insurmountable divide existing between the two main US political parties. This research attempted to gain better understanding of the health effects that physically active lifestyles elicit in older populations. Previous research connected biological outcomes across several systems to improvements in systemic health, but our efforts attempted to apply similar analysis to generalized activity levels in the form of various reported activities standardized using metabolic equivalent task metrics. For this study, the examined age group expanded beyond individuals that are older than 65, and instead included all ages over 50. Analysis used Metabolic Equivalent Task Minutes (METS) as a representative variable of general activity, using ordinary least squares regression to analyze effects on biological systems. It was found that with the selected model, there was minimal to no impact biologically that correlated with increasing general activity levels among the 50+ community. Secondary analyses were performed that lacked sufficient statistical power, however their results may be useful in determining further course of study. In the first of the secondary analyses, we used the exercise model to assess changes in individual perception of health. In this there were very few correlations found between biological health and perceptions of health. Finally, biological health and perception of health were regressed against several variables that were indicative of medical service utilization Analysis found that for the selected cohort, there were minimal measurable biological effects relating to exercise, but that the more active respondents correlated with higher belief in control over their health, as well as reductions in health-based restrictions on ability to perform intermediate level activities of daily living.
Publisher Site: RIT Repository
PDF (Requires Sign-In): PDF
Click for Bibtex
@book{schuler2020physical, title = {Physical Activity in Aging: Biological Outcomes, Functional Autonomy, and Policy Implications}, author = {Schuler, Nathan Albert}, year = {2020}, school = {Rochester Institute of Technology} }
[T-2020-47] Shashank Rudroju. (2020). “Root Failure Analysis in Meshed Tree Networks.” Rochester Institute of Technology.
Abstract: Mesh topologies play a vital role in switched networks. Broadcast storms due to the loops in Mesh Networks are a major concern in switched networks. Logical spanning trees are constructed using algorithms like spanning tree algorithm to avoid loops and hence address the broadcast storm problem. However, in the event of a topology change or a link failure in the network, it takes time to converge and construct new spanning tree to forward frames. Link State routing and other protocols like Rapid Spanning Tree protocol[2][19] were introduced to address the problems of high convergence times in the basic spanning tree protocol(STP) in the event of network component failures. A much efficient and advanced approach was offered with Mesh Tree Protocol based on the Mesh Tree Algorithm. Mesh Tree Protocol constructs multiple tree branches from a single root and quickly falls back to an alternate path or switch in case of link or switch failures. This cuts down the convergence delays considerably. The Mesh Tree Protocol based on the Mesh Tree Algorithm is currently under development as an IEEE standard. Other major changes in the MTP compared to the already existing protocols is that the root is manually assigned instead of using the root election procedure. This will cut down the delays during instantiation of the protocol but also has risk concerning the action of the protocol if the manually assigned root fails. To address this concern, an enhancement to the Mesh Tree protocol is being researched in this thesis. The idea is to implement a Multiple Meshed Tree algorithm where meshed trees will be constructed from multiple roots. This thesis introduces root redundancy in the Mesh Tree Protocol and will be assessed for performance improvements on root failures in comparison with Rapid Spanning Tree Protocol (RSTP) which re-elects a root switch on the current root switch failure.
Publisher Site: RIT Repository
PDF (Requires Sign-In): PDF
Click for Bibtex
@book{rudroju2020root, title = {Root Failure Analysis in Meshed Tree Networks}, author = {Rudroju, Shashank}, year = {2020}, school = {Rochester Institute of Technology} }
[T-2020-46] Avdhesh Bansal. (2020). “Characterization of friction based on specific film thickness and wettability.” Rochester Institute of Technology.
Abstract: Friction and wear in tribological systems lead to monetary loss and environmental damage. A better understanding of factors that affect tribological behavior of a system will reduce losses and damages in a tribological system. Wetting and surface properties are important in solid-fluid interactions and hence should be considered in lubricant-surface tribological systems. Their effects on tribological behavior are not properly understood. This study analyzed the effect of wettability on tribological systems and checked whether inclusion of wettability in the analysis of friction can lead to a more unified approach. Wettability informs how a liquid will behave on a solid surface. Liquids with high wettability spread over the surface, and those with low wettability do not. Wettability depends on properties of not only the liquid but also the surface. The significance of wetting in tribological systems varies from system to system. The relative importance of wetting in tribology varies with the lubrication regime under which a system operates. The lubrication regime is characterized by lambda (λ) parameter. Based on the value of λ there are three lubrication regimes: boundary, mixed, and hydrodynamic. Researchers claim that wetting and surface properties are important in boundary lubrication regime and insignificant in mixed and hydrodynamic lubrication. This research analyzed the effects of wetting in all the regimes to test this claim. There is disagreement among scholars on how to characterize wettability in tribological systems. Some claim that a formulation of spreading parameter that comprises polar and disperse components of surface energy and surface tension provides relevant insight. Others claim that the contact angle formed between liquid and surface is a better measure of wettability. Another group of scholars claim that a non-dimensionalized spreading parameter should be used to characterize wetting. This parameter can be calculated by contact angle. In this study, the non-dimensionalized parameter was used as it is easy to calculate. It considers non-linear relation between contact angle and wetting behavior. The results based on this parameter converge with those based on polar and disperse components to surface tension and energy. This parameter was used along with λ to check whether different friction-coefficient versus λ curves for various lubricant surface systems can collapse into one friction coefficient versus λ*|S^* | curve. If various Stribeck curves for different systems can collapse into one curve, then the process of predicting friction and wear behavior would simplify, and thus the research in the field of tribology would accelerate.
Publisher Site: RIT Repository
PDF (Requires Sign-In): PDF
Click for Bibtex
@book{bansal2020characterization, title = {Characterization of friction based on specific film thickness and wettability}, author = {Bansal, Avdhesh}, year = {2020}, school = {Rochester Institute of Technology} }
[T-2020-45] Premkumar Udaiyar. (2020). “Cross-modal data retrieval and generation using deep neural networks.” Rochester Institute of Technology.
Abstract: The exponential growth of deep learning has helped solve problems across different fields of study. Convolutional neural networks have become a go-to tool for extracting features from images. Similarly, variations of recurrent neural networks such as Long-Short Term Memory and Gated Recurrent Unit architectures do a good job extracting useful information from temporal data such as text and time series data. Although, these networks are good at extracting features for a particular modality, learning features across multiple modalities is still a challenging task. In this work, we develop a generative common vector space model in which similar concepts from different modalities are brought closer in a common latent space representation while dissimilar concepts are pushed far apart in this same space. The developed model not only aims at solving the cross-modal retrieval problem but also uses the vector generated by the common vector space model to generate real looking data. This work mainly focuses on image and text modalities. However, it can be extended to other modalities as well. We train and evaluate the performance of the model on Caltech CUB and Oxford-102 datasets.
Publisher Site: RIT Repository
PDF (Requires Sign-In): PDF
Click for Bibtex
@book{udaiyar2020cross-modal, title = {Cross-modal data retrieval and generation using deep neural networks}, author = {Udaiyar, Premkumar}, year = {2020}, school = {Rochester Institute of Technology} }
[T-2020-44] M. Grady Saunders. (2020). “Randomizable phenology-dependent corn canopy for simulated remote sensing of agricultural scenes.” Rochester Institute of Technology.
Abstract: Crop health assessment and yield prediction from multi-spectral remote sensing imagery are ongoing areas of interest in precision agriculture. It is in these contexts that simulation-based techniques are useful to investigate system parameters, perform preliminary experiments, etc., because remote sensing systems can be prohibitively expensive to design, deploy, and operate. However, such techniques require realistic and reliable models of the real world. We thus present a randomizable time-dependent model of corn (Zea mays L.) canopy, which is suitable for procedural generation of high-fidelity virtual corn fields at any time in the vegetative growth phase, with application to simulated remote sensing of agricultural scenes. This model unifies a physiological description of corn growth subject to environmental factors with a parametric description of corn canopy geometry, and prioritizes computational efficiency in the context of ray tracing for light transport simulation. We provide a reference implementation in C++, which includes a software plug-in for the 5th edition of the Digital Imaging and Remote Sensing Image Generation tool (DIRSIG5), in order to make simulation of agricultural scenes more readily accessible. For validation, we use our DIRSIG5 plug-in to simulate multi-spectral images of virtual corn plots that correspond to those of a United States Department of Agriculture (USDA) site at the Beltsville Agricultural Research Center (BARC), where reference data were collected in the summer of 2018. We show in particular that 1) the canopy geometry as a function of time is in agreement with field measurements, and 2) the radiance predicted by a DIRSIG5 simulation of the virtual corn plots is in agreement with radiance-calibrated imagery collected by a drone-mounted MicaSense RedEdge imaging system. We lastly remark that DIRSIG5 is able to simulate imagery directly as digital counts provided detailed knowledge of the detector array, e.g., quantum efficiency, read noise, and well capacity. That being the case, it is feasible to investigate the parameter space of a remote sensing system via "end-to-end" simulation.
Publisher Site: RIT Repository
PDF (Requires Sign-In): PDF
Click for Bibtex
@book{saunders2020randomizable, title = {Randomizable phenology-dependent corn canopy for simulated remote sensing of agricultural scenes}, author = {Saunders, M. Grady}, year = {2020}, school = {Rochester Institute of Technology} }
[T-2020-43] Brady Collea. (2020). “Efficient Algorithms for Unrelated Parallel Machine Scheduling Considering Time of Use Pricing and Demand Charges.” Rochester Institute of Technology.
Abstract: There is an ever-increasing focus on sustainability and energy consumption worldwide. Manufacturing is one of the major areas where energy reduction is not only environmentally beneficial, but also incredibly financially beneficial. These industrial consumers pay for their electricity according to prices that fluctuate throughout the day. These price fluctuations are in place to shift consumption away from "peak" times, when electricity is in the highest demand. In addition to this consumption cost, industrial consumers are charged according to their highest level of demand in a given window of time in the form of demand charges. This paper presents multiple solution methods to solve a parallel machine shop scheduling problem to minimize the total energy cost of the production schedule under Time of Use (TOU) and demand charge pricing. The greedy heuristic and genetic algorithm developed are designed to provide efficient solutions to this problem. The results of these methods are compared to a previously developed integer program (IP) solved using CPLEX with respect to the quality of the solution and the computational time required to solve it. Findings of these tests show that the greedy heuristic handles the test problems with only a small optimality gap to the genetic algorithm and optimal IP solution. The largest test problems could not be solved by the genetic algorithm in the provided time period due to difficulty generating an initial solution population. However, when successful the genetic algorithm performed comparably to the CPLEX solver in terms solution quality yet provided faster solve times.
Publisher Site: RIT Repository
PDF (Requires Sign-In): PDF
Click for Bibtex
@book{collea2020efficient, title = {Efficient Algorithms for Unrelated Parallel Machine Scheduling Considering Time of Use Pricing and Demand Charges}, author = {Collea, Brady}, year = {2020}, school = {Rochester Institute of Technology} }
[T-2020-42] Robert W. Mason. (2020). “A Study on DNA Memory Encoding Architecture.” Rochester Institute of Technology.
Abstract: The amount of raw generated data is growing at an exponential rate due to the greatly increasing number of sensors in electronic systems. While the majority of this data is never used, it is often kept for cases such as failure analysis. As such, archival memory storage, where data can be stored at an extremely high density at the cost of read latency, is becoming more popular than ever for long term storage. In biological organisms, Deoxyribonucleic Acid (DNA) is used as a method of storing information in terms of simple building blocks, as to allow for larger and more complicated struc- tures in a density much higher than can currently be realized on modern memory devices. Given the ability for organisms to store this information in a set of four bases for an extremely long amounts of time with limited degradation, DNA presents itself as a possible way to store data in a manner similar to binary data. This work investigates the use of DNA strands as a storage regime, where system-level data is translated into an efficient encoding to minimize base pair errors both at a local level and at the chain level. An encoding method using a Bose-Chaudhuri-Hocquenghem (BCH) pre-coded Raptor scheme is implemented in conjunction with an 8 to 6 bi- nary to base translation, yielding an informational density of 1.18 bits/base pair. A Field-Programmable Gate Array (FPGA) is then used in conjunction with a soft-core processor to verify address and key translation abilities, providing strong support that a strand-pool DNA model is reasonable for archival storage.
Publisher Site: RIT Repository
PDF (Requires Sign-In): PDF
Click for Bibtex
@book{mason2020a, title = {A Study on DNA Memory Encoding Architecture}, author = {Mason, Robert W.}, year = {2020}, school = {Rochester Institute of Technology} }
[T-2020-41] Prashant Sanakaran. (2020). “Design and Simulation Analysis of Deep Learning Based Approaches and Multi-Attribute Algorithms for Warehouse Task Selection.” Rochester Institute of Technology.
Abstract: With the growth and adoption of global supply chains and internet technologies, warehouse operations have become more demanding. Particularly, the number of orders being processed over a given time frame is drastically increasing, leading to more work content. This makes operational tasks, such as material retrieval and storage, done manually more inefficient. To improve system-level warehouse efficiency, collaborating Autonomous Vehicles (AVs) are needed. Several design challenges encompass an AV, some critical aspects are navigation, path planning, obstacle avoidance, task selection decisions, communication, and control systems. The current study addresses the warehouse task selection problem given a dynamic pending task list and considering multiple attributes: distance, traffic, collaboration, and due date, using situational decision-making approaches. The study includes the design and analysis of two situational decision-making approaches for multi-attribute dynamic warehouse task selection: Deep Learning Approach for Multi-Attribute Task Selection (DLT) and Situation based Greedy (SGY) algorithm that uses a traditional algorithmic approach. The two approaches are designed and analyzed in the current work. Further, they are evaluated using a simulation-based experiment. The results show that both the DLT and SGY have potential and are effective in comparison to the earliest due date first and shortest travel distance-based rules in addressing the multi-attribute task selection needs of a warehouse operation under the given experimental conditions and trade-offs.
Publisher Site: RIT Repository
PDF (Requires Sign-In): PDF
Click for Bibtex
@book{sanakaran2020design, title = {Design and Simulation Analysis of Deep Learning Based Approaches and Multi-Attribute Algorithms for Warehouse Task Selection}, author = {Sanakaran, Prashant}, year = {2020}, school = {Rochester Institute of Technology} }
[T-2020-40] Zhongchao Qian. (2020). “Deep Convolutional Networks without Learning the Classifier Layer.” Rochester Institute of Technology.
Abstract: Deep convolutional neural networks (CNNs) are effective and popularly used in a wide variety of computer vision tasks, especially in image classification. Conventionally, they consist of a series of convolutional and pooling layers followed by one or more fully connected (FC) layers to produce the final output in image classification tasks. This design descends from traditional image classification machine learning models which use engineered feature extractors followed by a classifier, before the widespread application of deep CNNs. While this has been successful, in models trained for classifying datasets with a large number of categories, the fully connected layers often account for a large percentage of the network's parameters. For applications with memory constraints, such as mobile devices and embedded platforms, this is not ideal. Recently, a family of architectures that involve replacing the learned fully connected output layer with a fixed layer has been proposed as a way to achieve better efficiency. This research examines this idea, extends it further and demonstrates that fixed classifiers offer no additional benefit compared to simply removing the output layer along with its parameters. It also reveals that the typical approach of having a fully connected final output layer is inefficient in terms of parameter count. This work shows that it is possible to remove the entire fully connected layers thus reducing the model size up to 75% in some scenarios, while only making a small sacrifice in terms of model classification accuracy. In most cases, this method can achieve comparable performance to a traditionally learned fully connected classification output layer on the ImageNet-1K, CIFAR-100, Stanford Cars-196, and Oxford Flowers-102 datasets, while not having a fully connected output layer at all. In addition to comparable performance, the method featured in this research also provides feature visualization of deep CNNs at no additional cost.
Publisher Site: RIT Repository
PDF (Requires Sign-In): PDF
Click for Bibtex
@book{qian2020deep, title = {Deep Convolutional Networks without Learning the Classifier Layer}, author = {Qian, Zhongchao}, year = {2020}, school = {Rochester Institute of Technology} }
[T-2020-39] Max Mendelson. (2020). “Identifying Performance Regression From The Commit Phase Utilizing Machine Learning Techniques.” Rochester Institute of Technology.
Abstract: Over the lifespan of a software application, it is inevitable that changes to the source code will be made that causes unintended slowdowns in functionality. These slowdowns are referred to as performance regression. Typically projects who are particularly concerned about performance have performance testing that are run to identify if a performance regression is introduced into the code. This is difficult however due to how time consuming and resource intensive running performance tests are when trying to simulate a realistic scenario. The study of De Oliveira et. al. (Perphecy) [4] suggests a technique to predict the likelihood that a commit will introduce performance regression. This is done through the use of static and dynamic metrics across several projects. The study of Alshoaibi et. al. (PRICE) [2] replicates and expands the work but focuses on a single project, that being "Git". PRICE generated a large dataset consisting of benchmarks for each commit introduced into the code compared to the commit that was its predecessor. This thesis seeks to further expand PRICE and use their collected data to create a machine learning model that can accurately predict performance regression within commits. We have been able to successfully create these models and were able to outperform previous models at identifying performance regression introducing commits.
Publisher Site: RIT Repository
PDF (Requires Sign-In): PDF
Click for Bibtex
@book{mendelson2020identifying, title = {Identifying Performance Regression From The Commit Phase Utilizing Machine Learning Techniques}, author = {Mendelson, Max}, year = {2020}, school = {Rochester Institute of Technology} }
[T-2020-38] Justin Kon. (2020). “Gait Generation for Damaged Hexapods using Genetic Algorithm.” Rochester Institute of Technology.
Abstract: This paper discusses the design and implementation of a Genetic Algorithm for the generation of gaits compensating for system damage on the joint level of a hexapod system. The hexapod base used for this algorithm consists of six three degree of freedom legs on a rectangular body. The purpose of this algorithm is to generate a gait such that when N motors become inoperable, as detected by the robot's internal software, the system is able to continue moving about its environment. While algorithms like this have been implemented before, the generated gaits are a sequence of discrete foot positions. This work aims to generate continuous motions profiles for each joint of the leg rather than discrete foot positions. Previous works commonly disable an entire leg when damage occurs, instead this work aims to disable only individual joint motors.
Publisher Site: RIT Repository
PDF (Requires Sign-In): PDF
Click for Bibtex
@book{kon2020gait, title = {Gait Generation for Damaged Hexapods using Genetic Algorithm}, author = {Kon, Justin}, year = {2020}, school = {Rochester Institute of Technology} }
[T-2020-37] Rohini Jayachandre Gillela. (2020). “Design of Hardware CNN Accelerators for Audio and Image Classification.” Rochester Institute of Technology.
Abstract: Ever wondered how the world was before the internet was invented? You might soon wonder how the world would survive without self-driving cars and Advanced Driver Assistance Systems (ADAS). The extensive research taking place in this rapidly evolving field is making self-driving cars futuristic and more reliable. The goal of this research is to design and develop hardware Convolutional Neural Network (CNN) accelerators for self-driving cars, that can process audio and visual sensory information. The idea is to imitate a human brain that takes audio and visual data as input while driving. To achieve a single die that can process both audio and visual sensory information, it takes two different kinds of accelerators where one processes visual data from images captured by a camera and the other processes audio information from audio recordings. The Convolutional Neural Network AI algorithm is chosen to classify images and audio data. Image CNN (ICNN) is used to classify images and Audio CNN (ACNN) to classify any sound of significance while driving. The two networks are designed from scratch and implemented in software and hardware. The software implementation of the two AI networks utilizes the Numpy library of Python, while the hardware implementation is done in Verilog®. CNN is trained to classify between three classes of objects, Cars, Lights, and Lanes, while the other CNN is trained to classify sirens from an emergency vehicle, vehicle horn, and speech.
Publisher Site: RIT Repository
PDF (Requires Sign-In): PDF
Click for Bibtex
@book{gillela2020design, title = {Design of Hardware CNN Accelerators for Audio and Image Classification}, author = {Gillela, Rohini Jayachandre}, year = {2020}, school = {Rochester Institute of Technology} }
[T-2020-36] Rashmi Ballamajalu. (2020). “Turn and Orientation Sensitive A* for Autonomous Vehicles in Intelligent Material Handling Systems.” Rochester Institute of Technology.
Abstract: Autonomous mobile robots are taking on more tasks in warehouses, speeding up operations and reducing accidents that claim many lives each year. This work proposes a dynamic path planning algorithm, based on A* search method for large autonomous mobile robots such as forklifts, and generates an optimized, time-efficient path. Simulation results of the proposed turn and orientation sensitive A* algorithm show that it has a 94% success rate of computing a better or similar path compared to that of default A*. The generated paths are smoother, have fewer turns, resulting in faster execution of tasks. The method also robustly handles unexpected obstacles in the path.
Publisher Site: RIT Repository
PDF (Requires Sign-In): PDF
Click for Bibtex
@book{ballamajalu2020turn, title = {Turn and Orientation Sensitive A* for Autonomous Vehicles in Intelligent Material Handling Systems}, author = {Ballamajalu, Rashmi}, year = {2020}, school = {Rochester Institute of Technology} }
[T-2020-35] Dennis P. Bleier. (2020). “Radar-Aware Transmission and Scheduling for Cognitive Radio Dynamic Spectrum Access in the CBRS Radio Band.” Rochester Institute of Technology.
Abstract: Use of the wireless spectrum is increasing. In order to meet the throughput requirements, Dynamic Spectrum Access is a popular technique to maximize spectrum usage. This can be applied to the Citizen Broadband Radio Service (3550-3700MHz), a band recently opened by the Federal Communications Commission for opportunistic access. This radio band can be accessed as long as no higher priority users are interfered with. The top priority users are called incumbents, which are commonly naval radar. Naval radars transmit a focused beam that can be modelled as a periodic function. Lower tier users are prohibited from transmitting when their transmissions coincide and interfere with the radar beam. The second and third tier users are called Priority Access Licensees and General Authorized Access, respectively. Lower tier users must account for the transmission outage due to the presence of the radar in their scheduling algorithms. In addition, the scheduling algorithms should take Quality of Service constraints, more specifically delay constraints into account. The contribution of this thesis is the design of a scheduling algorithm for CBRS opportunistic access in the presence of radar that provides Quality of Service for users, consider different traffic needs. This was implemented using the ns-3 discrete-event network simulator to simulate an environment with a radar and randomly placed radios using LTE-U to opportunistically transmit data. The proposed algorithm was compared against the Proportional Fair algorithm and a Proportional Fair algorithm with delay awareness. Performance was measured with and without fading models present. The proposed algorithm better balanced Quality of Service requirements and minimized the effect of transmission outage due to presence of the radar.
Publisher Site: RIT Repository
PDF (Requires Sign-In): PDF
Click for Bibtex
@book{bleier2020radar-aware, title = {Radar-Aware Transmission and Scheduling for Cognitive Radio Dynamic Spectrum Access in the CBRS Radio Band}, author = {Bleier, Dennis P.}, year = {2020}, school = {Rochester Institute of Technology} }
[T-2020-34] Srivallabha Karnam. (2020). “Self-Supervised Learning for Segmentation using Image Reconstruction.” Rochester Institute of Technology.
Abstract: Deep learning is the engine that is piloting tremendous growth in various segments of the industry by consuming valuable fuel called data. We are witnessing many businesses adopting this technology be it healthcare, transportation, defense, semiconductor, or retail. But most of the accomplishments that we see now rely on supervised learning. Supervised learning needs a substantial volume of labeled data which are usually annotated by humans- an arduous and expensive task often leading to datasets that are insufficient in size or human labeling errors. The performance of deep learning models is only as good as the data. Self-supervised learning minimizes the need for labeled data as it extracts the pertinent context and inherited data content. We are inspired by image interpolation where we resize an image from a one-pixel grid to another. We introduce a novel self-supervised learning method specialized for semantic segmentation tasks. We use Image reconstruction as a pre-text task where pixels and or pixel channel (R or G or B pixel channel) in the input images are dropped in a defined or random manner and the original image serves as ground truth. We use the ImageNet dataset for a pretext learning task, and PASCAL V0C to evaluate efficacy of proposed methods. In segmentation tasks decoder is equally important as the encoder, since our proposed method learns both the encoder and decoder as a part of a pretext task, our method outperforms existing self-supervised segmentation methods.
Publisher Site: RIT Repository
PDF (Requires Sign-In): PDF
Click for Bibtex
@book{karnam2020self-supervised, title = {Self-Supervised Learning for Segmentation using Image Reconstruction}, author = {Karnam, Srivallabha}, year = {2020}, school = {Rochester Institute of Technology} }
[T-2020-33] Robert Relyea. (2020). “Improving Omnidirectional Camera-Based Robot Localization Through Self-Supervised Learning.” Rochester Institute of Technology.
Abstract: Autonomous agents in any environment require accurate and reliable position and motion estimation to complete their required tasks. Many different sensor modalities have been utilized for this task such as GPS, ultra-wide band, visual simultaneous localization and mapping (SLAM), and light detection and ranging (LiDAR) SLAM. Many of the traditional positioning systems do not take advantage of the recent advances in the machine learning field. In this work, an omnidirectional camera position estimation system relying primarily on a learned model is presented. The positioning system benefits from the wide field of view provided by an omnidirectional camera. Recent developments in the self-supervised learning field for generating useful features from unlabeled data are also assessed. A novel radial patch pretext task for omnidirectional images is presented in this work. The resulting implementation will be a robot localization and tracking algorithm that can be adapted to a variety of environments such as warehouses and college campuses. Further experiments with additional types of sensors including 3D LiDAR, 60 GHz wireless, and Ultra-Wideband localization systems utilizing machine learning are also explored. A fused learned localization model utilizing multiple sensor modalities is evaluated in comparison to individual sensor models.
Publisher Site: RIT Repository
PDF (Requires Sign-In): PDF
Click for Bibtex
@book{relyea2020improving, title = {Improving Omnidirectional Camera-Based Robot Localization Through Self-Supervised Learning}, author = {Relyea, Robert}, year = {2020}, school = {Rochester Institute of Technology} }
[T-2020-32] Jason Slover. (2020). “Synthetic Aperture Radar simulation by Electro Optical to SAR Transformation using Generative Adversarial Network.” Rochester Institute of Technology.
Abstract: The CycleGAN generative adversarial network is applied to simulated electo-optical (EO) images in order to transition them into a Synthetic Aperture Radar (SAR)-like domain. If possible this would allow the user to simulate radar images without computing the phase history of the scene. Though visual inspection leaves the output images appearing SAR-like, examination by t-distributed Stochastic Neighbor Embedding (t-SNE) shows that CycleGAN was insufficient at generalizing an EO-to-SAR conversion. Further, using the transitioned images as training data for a neural network shows that SAR features used for classification are not present in the simulated images.
Publisher Site: RIT Repository
PDF (Requires Sign-In): PDF
Click for Bibtex
@book{slover2020synthetic, title = {Synthetic Aperture Radar simulation by Electro Optical to SAR Transformation using Generative Adversarial Network}, author = {Slover, Jason}, year = {2020}, school = {Rochester Institute of Technology} }
[T-2020-31] Nitinraj Nair. (2020). “RIT-Eyes: Realistic Eye Image and Video Generation for Eye Tracking Applications.” Rochester Institute of Technology.
Abstract: Deep neural networks for video-based eye tracking have demonstrated resilience to noisy environments, stray reflections, and low resolution. However, to train these networks, a large number of manually annotated images are required. To alleviate the cumbersome process of manual labeling, computer graphics rendering is employed to automatically generate a large corpus of annotated eye images under various conditions. In this work, we introduce a synthetic eye image and video generation platform called RIT-Eyes that improves upon previous work by adding features such as an active deformable iris, an aspherical cornea, retinal retro-reflection, and gaze-coordinated eye-lid deformations. To demonstrate the utility of our platform, we render images reflecting the represented gaze distributions inherent in two publicly available eye image datasets, NVGaze and OpenEDS. Additionally, we also render two datasets which mimic the characteristics of Pupil Labs Core mobile eye tracker. Our platform enables users to render realistic eye images by providing parameters for camera position, illuminator position, and head and eye pose. The pipeline can also be used to render temporal sequences of realistic eye movements captured in datasets such as Gaze-in-Wild.
Publisher Site: RIT Repository
PDF (Requires Sign-In): PDF
Click for Bibtex
@book{nair2020rit-eyes:, title = {RIT-Eyes: Realistic Eye Image and Video Generation for Eye Tracking Applications}, author = {Nair, Nitinraj}, year = {2020}, school = {Rochester Institute of Technology} }
[T-2020-30] Darshan Ramesh Bhanushali. (2020). “Multi-Sensor Fusion for 3D Object Detection.” Rochester Institute of Technology.
Abstract: Sensing and modelling of the surrounding environment is crucial for solving many of the problems in intelligent machines like self-driving cars, autonomous robots, and augmented reality displays. Performance, reliability and safety of the autonomous agents rely heavily on the way the environment is modelled. Two-dimensional models are inadequate to capture the three-dimensional nature of real-world scenes. Three-dimensional models are necessary to achieve the standards required by the autonomy stack for intelligent agents to work alongside humans. Data driven deep learning methodologies for three-dimensional scene modelling has evolved greatly in the past few years because of the availability of huge amounts of data from variety of sensors in the form of well-designed datasets. 3D object detection and localization are two of the key requirements for tasks such as obstacle avoidance, agent-to-agent interaction, and path planning. Most methodologies for object detection work on a single sensor data like camera or LiDAR. Camera sensors provide feature rich scene data and LiDAR provides us 3D geometrical information. Advanced object detection and localization can be achieved by leveraging the information from both camera and LiDAR sensors. In order to effectively quantify the uncertainty of each sensor channel, an appropriate fusion strategy is needed to fuse the independently encoded point clouds from LiDAR with the RGB images from standard vision cameras. In this work, we introduce a fusion strategy and develop a multimodal pipeline which utilizes existing state-of-the-art deep learning based data encoders to produce robust 3D object detection and localization in real-time. The performance of the proposed fusion model is evaluated on the popular KITTI 3D benchmark dataset.
Publisher Site: RIT Repository
PDF (Requires Sign-In): PDF
Click for Bibtex
@book{bhanushali2020multi-sensor, title = {Multi-Sensor Fusion for 3D Object Detection}, author = {Bhanushali, Darshan Ramesh}, year = {2020}, school = {Rochester Institute of Technology} }
[T-2020-29] Saurav Singh. (2020). “Push Recovery for Humanoid Robots using Linearized Double Inverted Pendulum.” Rochester Institute of Technology.
Abstract: Biped robots have come a long way in imitating a human being's anatomy and posture. Standing balance and push recovery are some of the biggest challenges for such robots. This work presents a novel simplified model for a humanoid robot to recover from external disturbances. The proposed Linearized Double Inverted Pendulum, models the dynamics of a complex humanoid robot that can use ankle and hip recovery strategies while taking full advantage of the advances in controls theory research. To support this, an LQR based control architecture is also presented in this work. The joint torque signals are generated along with ankle torque constraints to ensure the Center of Pressure stays within the support polygon. Simulation results show that the presented model can successfully recover from external disturbances while using minimal effort when compared to other widely used simplified models. It optimally uses the the torso weight to generate angular momentum about the pelvis of the robot to counter-balance the effects of external disturbances. The proposed method was validated on simulated `TigerBot-VII', a humanoid robot.
Publisher Site: RIT Repository
PDF (Requires Sign-In): PDF
Click for Bibtex
@book{singh2020push, title = {Push Recovery for Humanoid Robots using Linearized Double Inverted Pendulum}, author = {Singh, Saurav}, year = {2020}, school = {Rochester Institute of Technology} }
[T-2020-28] Sai Shashidhar Nagabandi. (2020). “Self-Supervised Video Representation Learning by Recurrent Networks and Frame Order Prediction.” Rochester Institute of Technology.
Abstract: The success of deep learning models in challenging tasks of computer vision and natural language processing depend on good vector representations of data. For example, learning efficient and salient video representations is one of the fundamental steps for many tasks like action recognition and next frame prediction. Most methods in deep learning rely on large datasets like ImageNet or MSCOCO for training, which is expensive and time consuming to collect. Some of the earlier works in video representation learning relied on encoder-decoder style networks in an unsupervised fashion, which would take in a few frames at a time. Research in the field of self-supervised learning is growing, and has shown promising results on image-related tasks to both learn data representations as well as pre-learn weights for networks using unlabeled data. However, many of these techniques use static architectures like AlexNet, which fail to take into account the temporal aspect of videos. Learning frame-to-frame temporal relationships is essential to learning latent representations of video. In our work, we propose to learn this temporality by pairing static encodings with a recurrent long short term memory network. This research will also investigate applying different methods of encoding architecture along with the recurrent network, to take in a range of different number of frames. We also introduce a novel self-supervised task in which the neural network has two tasks; predicting if a tuple of input frames is temporally consistent, and if not, predict the positioning of incorrect tuple. The efficacy is finally measured by using these trained networks on downstream tasks like action recognition on standard datasets UCF101 and HMDB51.
Publisher Site: RIT Repository
PDF (Requires Sign-In): PDF
Click for Bibtex
@book{nagabandi2020self-supervised, title = {Self-Supervised Video Representation Learning by Recurrent Networks and Frame Order Prediction}, author = {Nagabandi, Sai Shashidhar}, year = {2020}, school = {Rochester Institute of Technology} }
[T-2020-27] Akhil Santha. (2020). “Deepfakes Generation using LSTM based Generative Adversarial Networks.” Rochester Institute of Technology.
Abstract: Deep learning has been achieving promising results across a wide range of complex task domains. However, recent advancements in deep learning have also been employed to create software which causes threats to the privacy of people and national security. One among them is deepfakes, which creates fake images as well as videos that cannot be detected as forgeries by humans. Fake speeches of world leaders can even cause threat to world stability and peace. Apart from the malicious usage, deepfakes can also be used for positive purposes such as in films for post dubbing or performing language translation. This latter case was recently used in the latest Indian election such that politician speeches can be converted to many Indian dialects across the country. This work was traditionally done using computer graphic technology and 3D models. But with advances in deep learning and computer vision, in particular GANs, the earlier methods are being replaced by deep learning methods. This research will focus on using deep neural networks for generating manipulated faces in images and videos. This master's thesis develops a novel architecture which can generate a full sequence of video frames given a source image and a target video. We were inspired by the works done by NVIDIA in vid2vid and few-shot vid2vid where they learn to map source video domains to target domains. In our work, we propose a unified model using LSTM based GANs along with a motion module which uses a keypoint detector to generate the dense motion. The generator network employs warping to combine the appearance extracted from the source image and the motion from the target video to generate realistic videos and also to decouple the occlusions. The training is done end-to-end and the keypoints are learnt in a self-supervised way. Evaluation is demonstrated on the recently introduced FaceForensics++ and VoxCeleb datasets.
Publisher Site: RIT Repository
PDF (Requires Sign-In): PDF
Click for Bibtex
@book{santha2020deepfakes, title = {Deepfakes Generation using LSTM based Generative Adversarial Networks}, author = {Santha, Akhil}, year = {2020}, school = {Rochester Institute of Technology} }
[T-2020-26] Sidharth Makhija. (2020). “Graph Networks for Multi-Label Image Recognition.” Rochester Institute of Technology.
Abstract: Providing machines with a robust visualization of multiple objects in a scene has a myriad of applications in the physical world. This research solves the task of multi-label image recognition using a deep learning approach. For most multi-label image recognition datasets, there are multiple objects within a single image and a single label can be seen many times throughout the dataset. Therefore, it is not efficient to classify each object in isolation, rather it is important to infer the inter-dependencies between the labels. To extract a latent representation of the pixels from an image, this work uses a convolutional network approach evaluating three different image feature extraction networks. In order to learn the label inter-dependencies, this work proposes a graph convolution network approach as compared to previous approaches such as probabilistic graph or recurrent neural networks. In the graph neural network approach, the image labels are first encoded into word embeddings. These serve as nodes on a graph. The correlations between these nodes are learned using graph neural networks. We investigate how to create the adjacency matrix without manual calculation of the label correlations in the respective datasets. This proposed approach is evaluated on the widely-used PASCAL VOC, MSCOCO, and NUS-WIDE multi-label image recognition datasets. The main evaluation metrics used will be mean average precision and overall F1 score, to show that the learned adjacency matrix method for labels along with the addition of visual attention for image features is able to achieve similar performance to manually calculating the label adjacency matrix.
Publisher Site: RIT Repository
PDF (Requires Sign-In): PDF
Click for Bibtex
@book{makhija2020graph, title = {Graph Networks for Multi-Label Image Recognition}, author = {Makhija, Sidharth}, year = {2020}, school = {Rochester Institute of Technology} }
[T-2020-25] Kenneth Alexopoulos. (2020). “Self-Supervision Initialization for Semantic Segmentation Networks.” Rochester Institute of Technology.
Abstract: Convolutional neural networks excel at extracting features from signals. These features are able to be utilized for many downstream tasks. These tasks include object recognition, object detection, depth estimation, pixel level semantic segmentation, and more. These tasks can be used for applications such as autonomous driving where images captured by a camera can be used to give a detailed understanding of the scene. While these models are impressive, they can fail to generalize to new environments. This forces the cumbersome process of collecting images from multifarious environments and annotating them by hand. Annotating thousands or millions of images is both expensive and time consuming. One can use transfer learning to transfer knowledge from a different dataset as an initial starting place for the weights of the same model training on the target dataset. This method requires that another dataset has already been annotated and salient information can be learned from the dataset that can aid the model on the second dataset. Another method that does notrely on human generated annotations is self-supervised learning in which annotation scan be computer generated using tasks that force learning image representations such as predicting the rotation of an image. In this thesis, self-supervised methods are evaluated specifically to improve semantic segmentation as the primary downstream task. Data augmentation's affect during pretraining is observed in context of its effect on downstream performance. Knowledge from multiple self-supervised tasks are combined to create a starting point for training on a target dataset that outperforms either method individually.
Publisher Site: RIT Repository
PDF (Requires Sign-In): PDF
Click for Bibtex
@book{alexopoulos2020self-supervision, title = {Self-Supervision Initialization for Semantic Segmentation Networks}, author = {Alexopoulos, Kenneth}, year = {2020}, school = {Rochester Institute of Technology} }
[T-2020-24] Jason Blocklove. (2020). “Hardware Intellectual Property Protection Through Obfuscation Methods.” Rochester Institute of Technology.
Abstract: Security is a growing concern in the hardware design world. At all stages of the Integrated Circuit (IC) lifecycle there are attacks which threaten to compromise the integrity of the design through piracy, reverse engineering, hardware Trojan insertion, physical attacks, and other side channel attacks — among other threats. Some of the most notable challenges in this field deal specifically with Intellectual Property (IP) theft and reverse engineering attacks. The IP being attacked can be ICs themselves, circuit designs making up those larger ICs, or configuration information for the devices like Field Programmable Gate Arrays (FPGAs). Custom or proprietary cryptographic components may require specific protections, as successfully attacking those could compromise the security of other aspects of the system. One method by which these concerns can be addressed is by introducing hardware obfuscation to the design in various forms. These methods of obfuscation must be evaluated for effectiveness and continually improved upon in order to match the growing concerns in this area. Several different forms of netlist-level hardware obfuscation were analyzed, on standard benchmarking circuits as well as on two substitution boxes from block ciphers. These obfuscation methods were attacked using a satisfiability (SAT) attack, which is able to iteratively rule out classes of keys at once and has been shown to be very effective against many forms of hardware obfuscation. It was ultimately shown that substitution boxes were naturally harder to break than the standard benchmarks using this attack, but some obfuscation methods still have substantially more security than others. The method which increased the difficulty of the attack the most was one which introduced a modified SIMON block cipher as a One-way Random Function (ORF) to be used for key generation. For a substitution box obfuscated in this way, the attack was found to be completely unsuccessful within a five-day window with a severely round-reduced implementation of SIMON and only a 32-bit obfuscation key.
Publisher Site: RIT Repository
PDF (Requires Sign-In): PDF
Click for Bibtex
@book{blocklove2020hardware, title = {Hardware Intellectual Property Protection Through Obfuscation Methods}, author = {Blocklove, Jason}, year = {2020}, school = {Rochester Institute of Technology} }
[T-2020-23] Karthik Subramanian. (2020). “Using Photoplethysmography for Simple Hand Gesture Recognition.” Rochester Institute of Technology.
Abstract: A new wearable band is developed which uses three Photoplethysmography (PPG) sensors for the purpose of hand gesture recognition (HGR). These sensors are typically used for heart rate estimation and detection of cardiovascular diseases. Heart rate estimates obtained from these sensors are disregarded when the arm is in motion on account of artifacts. This research suggests and demonstrates that these artifacts are repeatable in nature based on the gestures performed. A comparative study is made between the developed band and the Myo Armband which uses surface-Electromyography (s-EMG) for gesture recognition. Based on the results of this paper which employs supervised machine learning techniques, it can be seen that PPG can be utilized as a viable alternative modality for gesture recognition applications.
Publisher Site: RIT Repository
PDF (Requires Sign-In): PDF
Click for Bibtex
@book{subramanian2020using, title = {Using Photoplethysmography for Simple Hand Gesture Recognition}, author = {Subramanian, Karthik}, year = {2020}, school = {Rochester Institute of Technology} }
[T-2020-22] Devarth Parikh. (2020). “Gaze Estimation Based on Multi-view Geometric Neural Networks.” Rochester Institute of Technology.
Abstract: Gaze and head pose estimation can play essential roles in various applications, such as human attention recognition and behavior analysis. Most of the deep neural network-based gaze estimation techniques use supervised regression techniques where features are extracted from eye images by neural networks and regress 3D gaze vectors. I plan to apply the geometric features of the eyes to determine the gaze vectors of observers relying on the concepts of 3D multiple view geometry. We develop an end to-end CNN framework for gaze estimation using 3D geometric constraints under semi-supervised and unsupervised settings and compare the results. We explore the mathematics behind the concepts of Homography and Structure-from- Motion and extend it to the gaze estimation problem using the eye region landmarks. We demonstrate the necessity of the application of 3D eye region landmarks for implementing the 3D geometry-based algorithms and address the problem when lacking the depth parameters in the gaze estimation datasets. We further explore the use of Convolutional Neural Networks (CNNs) to develop an end-to-end learning-based framework, which takes in sequential eye images to estimate the relative gaze changes of observers. We use a depth network for performing monocular image depth estimation of the eye region landmarks, which are further utilized by the pose network to estimate the relative gaze change using view synthesis constraints of the iris regions. We further explore CNN frameworks to estimate the relative changes in homography matrices between sequential eye images based on the eye region landmarks to estimate the pose of the iris and hence determine the relative change in the gaze of the observer. We compare and analyze the results obtained from mathematical calculations and deep neural network-based methods. We further compare the performance of the proposed CNN scheme with the state-of-the-art regression-based methods for gaze estimation. Future work involves extending the end-to-end pipeline as an unsupervised framework for gaze estimation in the wild.
Publisher Site: RIT Repository
PDF (Requires Sign-In): PDF
Click for Bibtex
@book{parikh2020gaze, title = {Gaze Estimation Based on Multi-view Geometric Neural Networks}, author = {Parikh, Devarth}, year = {2020}, school = {Rochester Institute of Technology} }
[T-2020-21] Matthew W. Helvey. (2020). “Application of Thermal and Ultraviolet Sensors in Remote Sensing of Upland Ducks.” Rochester Institute of Technology.
Abstract: Detection, mapping, and monitoring of wildlife populations can provide significant insight into the health and trajectory of the ecosystems they rely on. In fact, it was not until recently that the benefits of wetland ecosystems were fully understood. Unfortunately, by that point, the United States had removed more than 50% of its native wetlands. The Prairie Pothole Region in North America is the premier breeding location for ducks; responsible for producing more than 50% of the North American ducks annually. The current survey methods for obtaining duck population counts are accomplished primarily using manned flights with observers manually identifying and counting the ducks below with coordinated ground surveys at a subset of these areas to obtain breeding pair estimates. The current industry standard for in situ assessment of nest locations for reproductive effort estimates is known as the "chain drag method", a manually intensive ground survey technique. However, recent improvements to small unmanned aerial systems (sUAS), coupled with the increased performance of lightweight sensors provide the potential for an alternative survey method. Our objective for this study was to assess the feasibility of utilizing sUAS based thermal longwave infrared (LWIR) imagery for detecting duck nests and ultraviolet (UV) imagery to classify breeding pairs in the Prairie Pothole Region. Our team deployed a DRS Tamarisk 640 LWIR sensor aboard a DJI Matrice 600 hexa-copter at Ducks Unlimited's Coteau Ranch in Sheridan County, North Dakota, to obtain the thermal imagery. At the ranch, 24 nests were imaged at two altitudes (40m and 80m) during the early morning (04h00-06h00), morning (06h00-08h00), and midday (11h00-13h00). Three main parameters, namely altitude, time of day, and terrain, were varied between flights and the impact that each had on detection accuracy was examined. Each nest image was min-max normalized and contrast enhanced using a high-pass filter, prior to input into the detection algorithm. We determined that the variable with the highest impact on detection accuracies was altitude. We were able to achieve detection accuracies of 58% and 69% for the 80m and 40m flights, respectively. We also determined that flights in the early morning yielded the highest detection accuracies, which was attributed to the increased contrast between the landscape and the nests after the prairie cooled overnight. Additionally, the detection accuracies were lowest during morning flights when the hens might be off the nests on a recess break from incubation. Therefore, we determined that with increases in spatial resolution, the use of sUAS based thermal imagery is feasible for detecting nests across the prairie and that flights should occur early in the morning while the hens are on the nest, in order to maximize detection potential. To assess the feasibility of classifying breeding duck pairs using UV imagery, our team took a preliminary step in simulating UAS reflectance imagery by collecting 260 scans across nine species of upland ducks with a fixed measurement geometry using an OceanOptic's spectroradiometer. We established baseline accuracies of 83%, 83%, and 76% for classifying age, sex, and species, respectively, by using a random forest (RF) classifier with simulated panchromatic (250-850nm) image sets. When using imagery at narrow UV bands with the same RF classifier, we were able to increase classification accuracies for age and species by 7%. Therefore, we demonstrated the potential for the use of sUAS based imagery as an alternate method for surveying nesting ducks, as well as potential improvements in age and species classification using UV imagery during breeding pair aerial surveys. Next steps should include efforts to extend these findings to airborne sensing systems, toward eventual operational implementation. Such an approach could alleviate environmental impacts associated with in situ surveys, while increasing the scale (scope and exhaustiveness) of surveys.
Publisher Site: RIT Repository
PDF (Requires Sign-In): PDF
Click for Bibtex
@book{helvey2020application, title = {Application of Thermal and Ultraviolet Sensors in Remote Sensing of Upland Ducks}, author = {Helvey, Matthew W.}, year = {2020}, school = {Rochester Institute of Technology} }
[T-2020-20] Ankush Kaul. (2020). “Implementing and Testing VLANs in Meshed Tree Protocol.” Rochester Institute of Technology.
Abstract: Meshed Tree Protocol (MTP)[1][2] is being developed to overcome the performance challenges faced with older loop avoidance layer 2 protocols like Spanning Tree Protocol (STP)[3] and Rapid STP (RSTP)[5] which have high convergence time, causing a significant delay during initial convergence and subsequent re-convergence in the event of topology change or link failure. This slow convergence is not suitable for the modern high speed and dynamic networks and is being addressed with better performing protocols like MTP which uses Meshed Tree Algorithm (MTA) to form multiple overlapping trees[1]. In this thesis we will implement Virtual Local Area Network(VLAN)[4] for MTP networks using the Global Environment for Network Innovation (GENI)[19] testbed. With the growing size and complexity of modern day networks it is essential to segment a larger network into isolated smaller sections which improve the security, reliability, and efficiency of the network. This is achieved by using VLANs which act as separate smaller networks within a larger network. In this thesis we will discuss the working and benefits of VLANs in the current implementations of STP and how can VLANs be introduced in MTP along with a basic implementation of VLANs in the code developed for MTP by extending it to support Multi Meshed trees, where each meshed tree would cover a VLAN.
Publisher Site: RIT Repository
PDF (Requires Sign-In): PDF
Click for Bibtex
@book{kaul2020implementing, title = {Implementing and Testing VLANs in Meshed Tree Protocol}, author = {Kaul, Ankush}, year = {2020}, school = {Rochester Institute of Technology} }
[T-2020-19] Hector Alejandro Rubio Rivera. (2020). “A Semi-Empirical Compact Model for IGZO TFT to Assess the Impact of Parasitic Elements in Active Matrix Pixel Designs.” Rochester Institute of Technology.
Abstract: In this work, an empirical off-state model was developed for amorphous IGZO TFTs with the purpose of creating a compact model in conjunction with an existing on-state model. The implementation of the compact model was done in Verilog-A to assess the impact of parasitc elements such as source/drain series resistance, and source/drain-to-gate overlap capacitances in a 2T1C pixel circuit. A novel region of operation was presented defined as a bridge between the subthreshold and the on-state regions. Two approaches were followed to solve for the fitting parameters inside this bridge region; an analytical and an empirical approach. The analytical solution provided the insight that there is a point where the derivatives of the on-state and the bridge region are equal. However, this solution showed non-physical behavior at some V_DS bias. Therefore, an empirical approach was followed where experimental data was used to find the V_DS dependence and eliminate the non-physical behavior. Ultimately, the compact model provided a remarkable R^2 in relation to experimental data and allowed for convergence during circuit simulation. The parasitic element assessment was carried out and two different phenomenon were described as they relate to these elements. Charge sharing and rise and fall time were the characteristics that were present with the introduction of parasitic elements. A capacitance ratio of C_ST/C_ov =10.6pF/265.07fF≈40 was used to diminish the former. However, the large capacitances associated in the input of the driver transistor caused the falling transient to be unable to provide full voltage swing. Therefore, proper circuit functionality was not achieved based on the presented design rules. Further work is being done to diminish overlap capacitances such as self-aligned devices.
Publisher Site: RIT Repository
PDF (Requires Sign-In): PDF
Click for Bibtex
@book{rubio rivera2020a, title = {A Semi-Empirical Compact Model for IGZO TFT to Assess the Impact of Parasitic Elements in Active Matrix Pixel Designs}, author = {Rubio Rivera, Hector Alejandro}, year = {2020}, school = {Rochester Institute of Technology} }
[T-2020-18] Andrew Fountain. (2020). “Exploring the Influence of Energy Constraints on Liquid State Machines.” Rochester Institute of Technology.
Abstract: Biological organisms operate under severe energy constraints but are still the most powerful computational systems that we know of. In contrast, modern AI algorithms are generally implemented on power-hungry hardware resources such as GPUs, limiting their use at the edge. This work explores the application of biologically-inspired energy constraints to spiking neural networks to better understand their effects on network dynamics and learning and to gain insight into the creation of more energy-efficient AI. Energy constraints are modeled by abstracting the role of astrocytes in metabolizing glucose and regulating the activity-driven distribution of ATP molecules to "pools" of neurons and synapses. First, energy constraints are applied to the fixed recurrent part (a.k.a. reservoir) of liquid state machines (LSM)—a type of recurrent spiking neural network—in order to analyze their effects on both the network's computational performance and ability to learn. Energy constraints were observed to have a significant influence on the dynamics of the network based on metrics such as Lyapunov exponent and separation ratio. In several cases the energy constraints also led to an increase in the LSM's classification accuracy (up to 6.17\% improvement over baseline) when applied to two time series classification tasks: epileptic seizure detection and gait recognition. This improvement in classification accuracy was also typically correlated with the LSM separation metric (Pearson correlation coefficient of 0.9 for seizure detection task). However, the increased classification accuracy was generally not observed in LSMs with sparse connectivity, highlighting the role of energy constrains in sparsifying the LSM's spike activity, which could lead to real-world energy savings in hardware implementations. In addition to the fixed LSM reservoir, the impact of energy constraints was also explored in the context of unsupervised learning with spike-timing dependent plasticity (STDP). It was observed that energy constraints can have the effect of decreasing the magnitude of the update of synaptic weights by up to 72.4\%, on average, depending on factors such as the energy cost of neuron spikes and energy pool regeneration rate. Energy constraints under certain conditions were also seen to modify which input frequencies the synapses respond to, tending to attenuate or eliminate weight updates from high frequency inputs. The effects of neuronal energy constraints on STDP learning were also studied at the network level to determine their effects on classification task performance. The final part of this work attempts to co-optimize an LSM's energy consumption and performance through reinforcement learning. A proximal policy optimization (PPO) agent is introduced into the LSM reservoir to control the level of neuronal spiking. This was done by allowing it to modify individual energy constraint parameters. The agent is rewarded based on the separation of the reservoir and additionally rewarded for the reduction of reservoir energy consumption.
Publisher Site: RIT Repository
PDF (Requires Sign-In): PDF
Click for Bibtex
@book{fountain2020exploring, title = {Exploring the Influence of Energy Constraints on Liquid State Machines}, author = {Fountain, Andrew}, year = {2020}, school = {Rochester Institute of Technology} }
[T-2020-17] Ian Prechtl. (2020). “CLAM: Compiler Lease of Cache Memory.” Rochester Institute of Technology.
Abstract: Caching is a common solution to the data movement performance bottleneck of today's computational systems and networks. Traditional caching examines program behavior and cache optimization separately, limiting performance. Recently, a new cache policy called Compiler Lease of cAche Memory (CLAM), has been suggested for program-based cache management. CLAM manages cache memory by allowing the compiler to assign leases, or lifespans, to cached items over a hardware-software interface, known as lease cache. Lease cache affords new performance potential, by way of program-driven cache optimization. It is applicable to existing cache architecture optimizations, and can be used to emulate other cache policies. This paper presents the first functional hardware implementation of lease cache for CLAM support. Lease cache hardware architecture is first presented, along with CLAM hardware support systems. The cache is emulated on an FPGA, and benchmarked using a collection of scientific kernels from the PolyBench/C suite, for three CLAM lease assignment policies: Compiler Assigned Reference Leasing (CARL), Phased Reference Leasing (PRL), and Fixed Uniform Leasing (FUL). CARL and PRL are able to achieve superior performance to Least Recently Used (LRU) replacement, while FUL is shown to serve as a safety mechanism for CLAM. Novel spectrum-based cache tenancy analysis verifies PRL's effectiveness in limiting cache utilization, and can identify changes in the working-set that cause the policy to perform adversely. This suggests that CLAM is extendable to more complex workloads if working-set transitions can elicit a similar change in lease policy. Being able to do so could yield appreciable performance improvements for large and highly iterative workloads like tensors.
Publisher Site: RIT Repository
PDF (Requires Sign-In): PDF
Click for Bibtex
@book{prechtl2020clam:, title = {CLAM: Compiler Lease of Cache Memory}, author = {Prechtl, Ian}, year = {2020}, school = {Rochester Institute of Technology} }
[T-2020-16] Brendan Drachler. (2020). “Mixed Signals in Pulsar Timing Arrays - Discerning Red Spin Noise from Gravitational Waves.” Rochester Institute of Technology.
Abstract: The detection of gravitational waves (GWs) with ground-based interferometers like the Laser Interferometer Gravitational Wave Observatory (LIGO) revolutionized our view of the Universe. Pulsar timing arrays (PTAs) like observed by the North American Nanohertz Observatory for Gravitational Waves (NANOGrav) will provide the community with an avenue for exploring the GW spectrum beyond what is capable with ground-based interferometers. Unlike LIGO, pulsars do not exist in an ideal environment where clever engineering can mitigate sources of noise. With that being said, many of the dominant sources of noise in PTAs have been well modeled within NANOGrav. The impact of red spin noise (RSN), which may result from rotational instabilities in the neutron star itself, is particularly problematic because its effect on a PTA's timing residuals could resemble the effects of a gravitational wave background (GWB). In this work, we simulate a PTA where each pulsar suffers from varying amplitudes of RSN and also has an underlying GWB. We recover the parameters describing the RSN to better characterize how a GWB of varying amplitudes biases our recovery efforts. We find regions of parameter space where the GWB and the RSN heavily bias each other. In these regions, RSN could masquerade as a GWB, or vice versa. However, we also find regions of parameter space that do not result in a biased recovery, even in the case of a large GWB amplitude. Finally, we define the problematic regions of parameter space and draw conclusions about recovery efforts in today's operational PTAs.
Publisher Site: RIT Repository
PDF (Requires Sign-In): PDF
Click for Bibtex
@book{drachler2020mixed, title = {Mixed Signals in Pulsar Timing Arrays - Discerning Red Spin Noise from Gravitational Waves}, author = {Drachler, Brendan}, year = {2020}, school = {Rochester Institute of Technology} }
[T-2020-15] Naga Durga Harish Kanamarlapudi. (2020). “Point Completion Networks and Segmentation of 3D Mesh.” Rochester Institute of Technology.
Abstract: Deep learning has made many advancements in fields such as computer vision, natural language processing and speech processing. In autonomous driving, deep learning has made great improvements pertaining to the tasks of lane detection, steering estimation, throttle control, depth estimation, 2D and 3D object detection, object segmentation and object tracking. Understanding the 3D world is necessary for safe end-to-end self-driving. 3D point clouds provide rich 3D information, but processing point clouds is difficult since point clouds are irregular and unordered. Neural point processing methods like GraphCNN and PointNet operate on individual points for accurate classification and segmentation results. Occlusion of these 3D point clouds remains a major problem for autonomous driving. To process occluded point clouds, this research explores deep learning models to fill in missing points from partial point clouds. Specifically, we introduce improvements to methods called deep multistage point completion networks. We propose novel encoder and decoder architectures for efficiently processing partial point clouds as input and outputting complete point clouds. Results will be demonstrated on ShapeNet dataset. Deep learning has made significant advancements in the field of robotics. For a robot gripper such as a suction cup to hold an object firmly, the robot needs to determine which portions of an object, or specifically which surfaces of the object should be used to mount the suction cup. Since 3D objects can be represented in many forms for computational purposes, a proper representation of 3D objects is necessary to tackle this problem. Formulating this problem using deep learning problem provides dataset challenges. In this work we will show representing 3D objects in the form of 3D mesh is effective for the problem of a robot gripper. We will perform research on the proper way for dataset creation and performance evaluation.
Publisher Site: RIT Repository
PDF (Requires Sign-In): PDF
Click for Bibtex
@book{harish kanamarlapudi2020point, title = {Point Completion Networks and Segmentation of 3D Mesh}, author = {Harish Kanamarlapudi, Naga Durga}, year = {2020}, school = {Rochester Institute of Technology} }
[T-2020-14] Abhisek Dey. (2020). “Convex Object Detection.” Rochester Institute of Technology.
Abstract: Scene understanding has become a fundamental research area in computer vision. To achieve this task, localizing and recognizing different objects in space is paramount. From the seminal work of face detection by Viola-Jones using cascaded Haar features to the state-of-the-art deep neural networks, object detection has evolved from just being used in limited cases to be being used extensively for detecting common and custom objects. Algorithm and hardware improvements currently allow for real time object detection on a smartphone. Typically, for each detected object, the object top-left co-ordinate along with bottom-right coordinate or width and height are returned. While this works for objects whose boundaries are orthogonal to the image boundaries, it struggles to accurately localize rotated or non-rectangular objects. By regressing for eight corner points instead of the traditional of the top-left and bottom-right of a rectangular box, we can mitigate these problems. Building up from anchor-free one-stage object detection methods, it is shown that object detection can also be used for arbitrary shaped bounding boxes.
Publisher Site: RIT Repository
PDF (Requires Sign-In): PDF
Click for Bibtex
@book{dey2020convex, title = {Convex Object Detection}, author = {Dey, Abhisek}, year = {2020}, school = {Rochester Institute of Technology} }
[T-2020-13] Rahul Guila. (2020). “Path Loss Model for 2.4GHZ Indoor Wireless Networks with Application to Drones.” Rochester Institute of Technology.
Abstract: Indoor wireless communication using Wireless Fidelity (Wi-Fi) is becoming a major need for the success of Unmanned Aerial Vehicles (UAVs), Internet of Things (IoT) and cloud robotics in both developed and developing countries. With different operating conditions, interference, obstacles and type of building materials used, it is difficult to predict the path loss components in an indoor environment, which are crucial for the network design. It has been observed that the proposed indoor path loss models cannot be used for UAV operations due to variations in building materials utilized, floor plans, scattering on both ends, etc. In this work, we propose a non-deterministic statistical indoor path loss model, namely, the UAV Low Altitude Air to Ground (U-LAAG) model, adapted from ITU-R model, which can be used for the 2.4 - 2.5 GHz, Industrial Scientific and Medical (ISM) band. To test and validate the proposed model, we conduct several experiments with different conditions such as University premise with obstacles, typical dwelling and basement locations. We have also compared U-LAAG with popular path loss models such as ITU-R, Two-ray and Log-distance; U-LAAG matches closely with the drive test results as compared to other models. We believe that the proposed ULAAG model can be used as basis to design accurate indoor communication networks required for regular Wi-Fi communications and deployment and operations of UAV, IoT and cloud robotics.
Publisher Site: RIT Repository
PDF (Requires Sign-In): PDF
Click for Bibtex
@book{guila2020path, title = {Path Loss Model for 2.4GHZ Indoor Wireless Networks with Application to Drones}, author = {Guila, Rahul}, year = {2020}, school = {Rochester Institute of Technology} }
[T-2020-12] Salman Khan. (2020). “The Use of Deep Learning Methods to Assist in the Classification of Seismically Vulnerable Dwellings.” Rochester Institute of Technology.
Abstract: Exciting research is being conducted using Google's Street View imagery. Researchers can have access to training data that allows CNN training for topics ranging from assessing neighborhood environments to estimating the age of a building. However, due to the uncontrolled nature of imagery available via Google's Street View API, data collection can be lengthy and tedious. In an effort to help researchers gather address specific dwelling images efficiently, we developed an innovative and novel way of automatically performing this task. It was accomplished by exploiting Google's publicly available platform with a combination of 3 separate network types and post-processing techniques. Our uniquely developed non-maximum suppression (NMS) strategy helped achieve 99.4%, valid, address specific, dwelling images. We explored the efficacy of utilizing our newly developed mechanism to train a CNN on Unreinforced Masonry (URM) buildings. We made this selection because building collapse during an earthquake account for majority of the deaths during a disaster of this kind. An automated approach for identifying seismically vulnerable buildings using street level imagery has been met with limited success to this point with no promising results presented in the literature. We have been able to achieve the best accuracy reported to date, at 83.63%, in identifying URM, finished URM, and non-URM buildings using manually curated images. We performed an ablation study to establish synergistic parameters on ResNeXt-101-FixRes. We also present a visualization the first layer of the network to ascertain and demonstrate how a deep learning network can distinguish between various types of URM buildings. Lastly, we establish the value of our automatically generated data set for these building types by achieving an accuracy of 84.91%. This is higher than the accuracy achieved using our hand curated data set of 83.63%.
Publisher Site: RIT Repository
PDF (Requires Sign-In): PDF
Click for Bibtex
@book{khan2020the, title = {The Use of Deep Learning Methods to Assist in the Classification of Seismically Vulnerable Dwellings}, author = {Khan, Salman}, year = {2020}, school = {Rochester Institute of Technology} }
[T-2020-11] Ben Christians. (2020). “Self-Admitted Technical Debt - An Investigation from Farm to Table to Refactoring.” Rochester Institute of Technology.
Abstract: Self-Admitted Technical Debt (SATD) is a metaphorical concept which describes the self-documented contribution of technical debt to a software project in the manner of source-code comments. SATD can linger in projects and degrade source-code quality, but its palpable visibility draws a peculiar sort of attention from developers. There is a need to understand the significance of engineering SATD within a software project, as these debts may have lurking repercussions. While the oft-performed action of refactoring may work against a generalized volume of source code degradation, there exists only slight evidence suggesting that the act of refactoring has a distinct impact on SATD. In fact, refactoring is better understood to convalesce the measurable quality of source code which may very well remain unimpressed by the preponderance of SATD instances. In observation of the cross-section of these two concepts, it would seem logical to presume some magnitude of correlation between refactorings and SATD removals. In this thesis, we will address the extent of such concurrence, while also seeking to develop a dependable tool to promote the empirical studies of SATD. Using this tool, we mined data from 5 open source Java projects, from which associations between SATD removals and refactoring actions were drawn to show that developers tend to refactor SATD-containing code differently than they do code elsewhere in their projects. We also concluded that design-related SATD is more likely to entail a refactoring than non-design SATD.
Publisher Site: RIT Repository
PDF (Requires Sign-In): PDF
Click for Bibtex
@book{christians2020self-admitted, title = {Self-Admitted Technical Debt - An Investigation from Farm to Table to Refactoring}, author = {Christians, Ben}, year = {2020}, school = {Rochester Institute of Technology} }
[T-2020-10] Braeden Morrison. (2020). “Tree-Based Hardware Recursion for Divide-and-Conquer Algorithms.” Rochester Institute of Technology.
Abstract: It is well-known that custom hardware accelerators implemented as application-specific integrated circuits (ASICs) or on field-programmable gate arrays (FPGAs) can solve many problems much faster than software running on a central processing unit (CPU). This is because FPGAs and ASICs can have handcrafted data and control paths which exploit parallelism in ways that CPUs cannot. However, designing custom hardware is complicated and implementing algorithms in a way that takes advantage of the desired parallelism can be difficult. One class of algorithms that exemplifies this is divide-and-conquer algorithms. A divide-and-conquer algorithm is a type of recursive algorithm that solves a problem by repeatedly dividing it into smaller sub-problems. These algorithms have a lot of parallelism because they generate a large number of sub-problems that can be computed independently of each other. Unfortunately, traditional stack-based approaches to handling recursion in hardware make exploiting this parallelism difficult. This work proposes a new general-purpose approach to implementing recursive functions in hardware, which we call TreeRecur. TreeRecur uses trees to represent the branching recursive function calls of divide-and-conquer algorithms, which makes it possible to take advantage of their procedure-level parallelism. To allow for design flexibility, TreeRecur executes algorithms using a configurable number of independent function processors. These processors are generated using high-level synthesis, making it easy to implement a variety of different algorithms. Our solution was tested on three different algorithms and compared against software implementations of the same algorithms. Performance results were collected in terms of execution speed and energy consumption. TreeRecur was found to have execution speeds comparable to software when differences in clock speed were accounted for and was found to consume up to 11.2 times less energy.
Publisher Site: RIT Repository
PDF (Requires Sign-In): PDF
Click for Bibtex
@book{morrison2020tree-based, title = {Tree-Based Hardware Recursion for Divide-and-Conquer Algorithms}, author = {Morrison, Braeden}, year = {2020}, school = {Rochester Institute of Technology} }
[T-2020-09] Iti Bansal. (2020). “Analyzing the Impact of Negative Sampling on Fact-Prediction Algorithms.” Rochester Institute of Technology.
Abstract: Knowledge graphs are useful for many applications like product recommendations and web search query engines. However, knowledge graphs are marked by incompleteness. Fact-prediction algorithms aim to expand knowledge graphs by predicting missing facts. Fact-prediction algorithms train models using positive facts present and creating negative facts not present in the knowledge graph at hand. Negative facts are obtained by corrupting information in the positive facts present in the knowledge graph at hand. Although it is generally assumed that negative facts drive the accuracy of fact-prediction algorithms, this concept has not been thoroughly examined yet. In this work, we investigate whether negative facts indeed drive fact-prediction accuracy by employing different negative fact generation strategies in translation-based algorithms, a popular branch of fact-prediction algorithms. We propose a new negative fact generation strategy that utilizes knowledge from immediate neighbors to corrupt a fact. Our extensive experiments using well-known benchmarking datasets show that negative facts indeed drive the accuracy of fact-prediction models, and that this accuracy dramatically changes depending on the negative fact generation strategy used for training and testing models. Assuming that the strategies generate negative facts with different levels of semantic plausibility, we observe that models trained using certain strategies are not able to distinguish missing facts from nonsensical or semantically-related facts. Additionally, our results show that the accuracy of models trained using the local-closed world assumption, the most common negative fact generation strategy, can be achieved with a combination of neighborhood-based and nonsensical strategies. This implies that fact-prediction algorithms can be trained using individual subgraphs instead of the whole knowledge graph, opening new research avenues.
Publisher Site: RIT Repository
PDF (Requires Sign-In): PDF
Click for Bibtex
@book{bansal2020analyzing, title = {Analyzing the Impact of Negative Sampling on Fact-Prediction Algorithms}, author = {Bansal, Iti}, year = {2020}, school = {Rochester Institute of Technology} }
[T-2020-08] Kush Benara. (2020). “Backplane System Design Considerations for Micro LED Displays.” Rochester Institute of Technology.
Abstract: Display technologies have evolved from the bulky Cathode Ray Tube based displays to the latest lightweight and low power micro-Led (uLED) based flat panel displays. A display system consists of a device technology that either manipulates the incoming light or emits its own light and a controller circuit to control the behavior of these devices. This system makes up the backplane of a display technology. uLEDs due to their small size provide higher resolution and better contrast than all the previous display technologies like the LCDs and the OLEDs. Backplane system design considerations for a uLED flat panel display is the primary focus of this work. The uLEDs are arranged in a 2-D matrix on a glass substrate with each uLED driven by an arrangement of 2 transistor and 1 capacitor that make up a pixel circuit. Indium Gallium Zinc Oxide TFTs are used as the choice of transistors for this project. The backplane design considerations are done to support an active matrix of 10x10, 50x50 and 380x380 pixel count in both monochrome and color versions. The behavior of the pixel circuit is evaluated using existing TFT and uLED electrical device compact models to determine the optimal value of the storage capacitor needed for the pixel circuit operation at 30 & 60Hz refresh rates. A model board with shift registers, transistors and LEDs to mimic the operation of a 10x10 uLED array is made and a FPGA is used to control the operation of this board. A timing relationship between the row and column data latch is deduced and the impact of the row-line, column-line RC delay and the pixel transient response time is evaluated. The impact of IR losses due to the power and ground line resistances are evaluated with the help monochrome pixel circuit physical layout. A new pixel circuit to accommodate the RGB pixels is made and care is taken to minimize both the RC delay and IR losses. Finally, a low contact resistance (0.05Ω-mm2) modular packaging scheme to electrically bond the two-dimensional array of pixel circuits on glass with the electronics on the PCB and to reduce RC delay is given.
Publisher Site: RIT Repository
PDF (Requires Sign-In): PDF
Click for Bibtex
@book{benara2020backplane, title = {Backplane System Design Considerations for Micro LED Displays}, author = {Benara, Kush}, year = {2020}, school = {Rochester Institute of Technology} }
[T-2020-07] Anmol S. Modur. (2020). “An Integrated Camera and Radar on-Robot System for Human Robot Collaboration.” Rochester Institute of Technology.
Abstract: The increased demand for collaborative tasks between humans and robots has caused an upsurge in newer sensor technologies to detect, locate, track, and monitor workers in a robot workspace. The challenge is to balance the accuracy, cost, and responsiveness of the system to maximize the safety of the worker. This work presents a sensor system that combines six 60GHz radar modules and six cameras to accurately track the location and speed of the workers in all 360 degrees around the robot. While the radar is tuned to identify moving targets, the cameras perform pose detection to evaluate the humans in the workspace and when fused, provide 4D pose estimates: 3D location and velocity. A custom PCB and enclosure is designed for it and it is mounted to the end-effector of a UR-10 robot. This system performs all of its computation on an Nvidia AGX Xavier for offline processing which allows it to be mounted to a mobile robot for outdoor use. Lastly, this system was evaluated for accuracy in human detection as well as accuracy in velocity measurements through numerous static and dynamic scenarios for the robot, the human, and both combined.
Publisher Site: RIT Repository
PDF (Requires Sign-In): PDF
Click for Bibtex
@book{modur2020an, title = {An Integrated Camera and Radar on-Robot System for Human Robot Collaboration}, author = {Modur, Anmol S.}, year = {2020}, school = {Rochester Institute of Technology} }
[T-2020-06] Maharshi Y. Shukla. (2020). “Influence of Liquid Height on Pool Boiling Heat Transfer.” Rochester Institute of Technology.
Abstract: As technology advances due to continuous research, devices are becoming more compact, efficient, and powerful. Therefore, heat rejection from such devices becomes ever so critical to maximizing their potential. Compared to other heat extraction methods, boiling provides one of the highest heat transfer coefficients. The heat extraction due to the boiling process is limited to the Critical Heat Flux (CHF). At CHF, an insulating layer of escaping bubbles forms upon the surface to prevent boiling continuity. Subsequently, the surface temperature increases uncontrollably, leading to a system failure. Hence, the elevation of CHF is critical to boiling enhancement. Improvements to the heat transfer process can be made with either surface manipulation or liquid manipulation. Based on previous studies, it is found that the removal of bubbles from the heater surface is critical to enhancing performance. Therefore, it is hypothesized that if a bubble can be encouraged to reach liquid-gas the interface quickly, gains in the boiling performance can be achieved. Due to the vapor bubble's movement in liquid bulk, it becomes critical to understand the influence of liquid height on pool boiling for enhancement. While pool boiling enhancement using heating surface modification is extensively studied and documented, there is a research gap between understanding the effect of liquid height at high heat fluxes. Thus, this study tries to evaluate the influence of liquid height on pool boiling performance at higher heat fluxes and identify the underlying bubble movement mechanism. It is observed that as CHF increases with liquid height. Moreover, it is observed that bubble movement is more effortless at low liquid height resulting in higher HTC. On the other hand, larger liquid height provides improved rewetting of the surface resulting in higher CHF. Upon analysis of high-speed recording of the escaping bubbles, it was observed that the maximum heat transfer coefficient is observed when the liquid height is about four times the height of the departing bubble diameter.
Publisher Site: RIT Repository
PDF (Requires Sign-In): PDF
Click for Bibtex
@book{shukla2020influence, title = {Influence of Liquid Height on Pool Boiling Heat Transfer}, author = {Shukla, Maharshi Y.}, year = {2020}, school = {Rochester Institute of Technology} }
[T-2020-05] John Jenco. (2020). “Network Slicing for Wireless Networks Operating in a Shared Spectrum Environment.” Rochester Institute of Technology.
Abstract: Network slicing is a very common practice in modern computer networks. It can serve as an efficient way to distribute network resources to physical groups of users, and allows the network to provide performance guarantees in terms of the Quality of Service. Physical links are divided logically and are assigned on a per-service basis to accomplish this. Traditionally, network slicing has been done mostly in wired networks, and bringing these practices to wireless networks has only been done recently. The main contribution of this thesis is network slicing applied to wireless environments where multiple adjacent networks are forced to share the same spectrum, namely in LTE and 5G. Spectrum in the sub-6GHz range is crowded by a wide range of services, and managing interference between networks is often challenging. A modified graph coloring technique is used both as a means to identify areas of interference and overlap between two networks, as well as assign spectrum resources to each node in an efficient manner. A central entity, known as the "Overseer", was developed as a bridge to pass interference-related information between the two coexisting networks. Performance baselines were first gathered for network slicing in a single-network scenario, followed by the introduction of a second network and the evaluation of the efficacy of the graph coloring approach. In the cases of highest interference from the secondary network, the modified graph coloring approach provided more than 22.3% reduction in median user delay, and more than 36.0% increase in median single-user and slice-aggregate throughput across all three network slices compared to the non-graph coloring scenario.
Publisher Site: RIT Repository
PDF (Requires Sign-In): PDF
Click for Bibtex
@book{jenco2020network, title = {Network Slicing for Wireless Networks Operating in a Shared Spectrum Environment}, author = {Jenco, John}, year = {2020}, school = {Rochester Institute of Technology} }
[T-2020-04] Joseph Panaro. (2020). “Fine-Tuning Sign Language Translation Systems Through Deep Reinforcement Learning.” Rochester Institute of Technology.
Abstract: Sign language is an important communication tool for a vast majority of deaf and hard-of-hearing (DHH) people. Data collected by the World Health organization states that there are 466 million people currently with hearing loss and that number could rise to 630 million by 2030 and over 930 million by 2050 \cite{DHH1}. Currently there are millions of sign language speakers around the world who utilize this skill on a daily basis. Bridging the gap between those who communicate solely with a spoken language and the DHH community is an ever-growing and omnipresent need. Unfortunately, in the field of natural language processing, sign language recognition and translation lags far behind its spoken language counterparts. The following research will seek to successfully leverage the field of Deep Reinforcement Learning (DRL) to make a significant improvement in the task of Sign Language Translation (SLT) with German Sign Language videos to German text sentences. To do this three major experiments are conducted. The first experiment examines the effects of Self-critical Sequence Training (SCST) when fine-tuning a simple Recurrent Neural Network (RNN) Long Short-Term Memory (LSTM) based sequence-to-sequence model. The second experiment takes the same SCST algorithm and applies it to a more powerful transformer based model. And the final experiment utilizes the Proximal Policy Optimization (PPO) algorithm alongside a novel fine-tuning process on the same transformer model. By using this approach of estimating the reward signal and normalization while optimizing for the model's test-time greedy inference procedure we aim to establish a new or comparable SOTA result on the RWTH-PHOENIX-Weather-2014 T German sign language dataset.
Publisher Site: RIT Repository
PDF (Requires Sign-In): PDF
Click for Bibtex
@book{panaro2020fine-tuning, title = {Fine-Tuning Sign Language Translation Systems Through Deep Reinforcement Learning}, author = {Panaro, Joseph}, year = {2020}, school = {Rochester Institute of Technology} }
[T-2020-03] Anisia Jabin. (2020). “Video-based iris feature extraction and matching using Deep Learning.” Rochester Institute of Technology.
Abstract: This research is initiated to enhance the video-based eye tracker's performance to detect small eye movements.[1] Chaudhary and Pelz, 2019, created an excellent foundation on their motion tracking of iris features to detect small eye movements[1], where they successfully used the classical handcrafted feature extraction methods like Scale InvariantFeature Transform (SIFT) to match the features on iris image frames. They extracted features from the eye-tracking videos and then used patent [2] an approach of tracking the geometric median of the distribution. This patent [2] excludes outliers, and the velocity is approximated by scaling by the sampling rate. To detect the microsaccades (small, rapid eye movements that occur in only one eye at a time) thresholding was used to estimate the velocity in the following paper[1]. Our goal is to create a robust mathematical model to create a 2D feature distribution in the given patent [2]. In this regard, we worked in two steps. First, we studied a large number of multiple recent deep learning approaches along with the classical hand-crafted feature extractor like SIFT, to extract the features from the collected eye tracker videos from Multidisciplinary Vision Research Lab(MVRL) and then showed the best matching process for our given RIT-Eyes dataset[3]. The goal is to make the feature extraction as robust as possible. Secondly, we clearly showed that deep learning methods can detect more feature points from the iris images and that matching of the extracted features frame by frame is more accurate than the classical approach.
Publisher Site: RIT Repository
PDF (Requires Sign-In): PDF
Click for Bibtex
@book{jabin2020video-based, title = {Video-based iris feature extraction and matching using Deep Learning}, author = {Jabin, Anisia}, year = {2020}, school = {Rochester Institute of Technology} }
[T-2020-02] Alexis M. Irwin. (2020). “Sub-pixel Sensitivity Variations and their Effects on Precision Aperture Photometry.” Rochester Institute of Technology.
Abstract: The response of pixels in CCDs and CMOS arrays is not uniform; physical aspects of pixel structure can create non-uniform electric fields within a pixel and the diffusion of carriers tends to blur pixel boundaries. It has previously been shown that the sub-pixel response can vary by up to 50% across a pixel, with more light being detected near the center and less around the edges. This intra-pixel response function (IPRF) can have significant effects on the aperture photometry of under-sampled PSFs, like those found in the Kepler and TESS exoplanet hunting missions. Knowledge of the IPRF can be used to correct for systematic variations in photometric measurements introduced by the sub-pixel variations in response. Additionally, in systems for which the optical PSF is not well defined, knowledge of the IPRF can allow for extraction of the optical PSF. Presented here are the results of the direct measurement of the IPRF of a thinned, back-illuminated CCD of the same model used on the NASA Kepler mission. The experimental setup used to measure the IPRF is discussed in detail. Measurements of the IPRF were made at various wavelengths, then combined to create an "effective IPRF" for the broad spectral bandpass filter used for collecting the scientific data of interest. This effective IPRF was then utilized in a model to study the effects of the IPRF on time series aperture photometry. The effects of spacecraft jitter and drift on aperture photometry were also investigated. Such results are relevant for exoplanet searches using the photometric detection technique as implemented in the Kepler and TESS missions.
Publisher Site: RIT Repository
PDF (Requires Sign-In): PDF
Click for Bibtex
@book{irwin2020sub-pixel, title = {Sub-pixel Sensitivity Variations and their Effects on Precision Aperture Photometry}, author = {Irwin, Alexis M.}, year = {2020}, school = {Rochester Institute of Technology} }
[T-2020-01] Andrew J. Rosato. (2020). “Regulation of Transposable Elements by Tumor Suppressor Protein 53.” Rochester Institute of Technology.
Abstract: Multiple transposable elements have been identified by colocalization analysis that display a strong predicted regulatory relationship with p53 associated peaks. RNA-Seq was used to identify differentially expressed transposable elements. ChIP-Seq was used to identify peaks representing transcription factor binding sites in p53 activated cells. The results of both experiments were then combined in a colocalization analysis identifying transposable element locations that were both differentially regulated and located near p53 associated peaks. The colocalization of ChIP-Seq and RNA-Seq analyses allows for the verification of p53's regulatory role in the expression of transposable elements across the genome. A Monte Carlo simulation was performed verifying that the frequency of the colocalizations observed occurred more frequently than due to random chance.
Publisher Site: RIT Repository
PDF (Requires Sign-In): PDF
Click for Bibtex
@book{rosato2020regulation, title = {Regulation of Transposable Elements by Tumor Suppressor Protein 53}, author = {Rosato, Andrew J.}, year = {2020}, school = {Rochester Institute of Technology} }
[T-2019-51] Aneesh Bhat. (2019). “Aerial Object Detection using Learnable Bounding Boxes.” Rochester Institute of Technology.
Abstract: Current methods in computer vision and object detection rely heavily on neural networks and deep learning. This active area of research is used in applications such as autonomous driving, aerial imaging, defense and surveillance. State-of-the-art object detection methods rely on rectangular shaped, horizontal/vertical bounding boxes drawn over an object to accurately localize its position. Such orthogonal bounding boxes ignore object pose, resulting in reduced object localization, and limiting downstream tasks such as object understanding and tracking. To overcome these limitations, this research presents object detection improvements that aid tighter and more precise detections. In particular, we modify the object detection anchor box definition to firstly include rotations along with height and width and secondly to allow arbitrary four corner point shapes. Further, the introduction of new anchor boxes gives the model additional freedom to model objects which are centered about a 45-degree axis of rotation. The resulting network allows minimum compromises in speed and reliability while providing more accurate localization. We present results on the DOTA dataset, showing the value of the flexible object boundaries, especially with rotated and non-rectangular objects.
Publisher Site: RIT Repository
PDF (Requires Sign-In): PDF
Click for Bibtex
@book{bhat2019aerial, title = {Aerial Object Detection using Learnable Bounding Boxes}, author = {Bhat, Aneesh}, year = {2019}, school = {Rochester Institute of Technology} }
[T-2019-50] Tej Pandit. (2019). “Relational Neurogenesis for Lifelong Learning Agents.” Rochester Institute of Technology.
Abstract: Reinforcement learning systems have shown tremendous potential in being able to model meritorious behavior in virtual agents and robots. The ability to learn through continuous reinforcement and interaction with an environment negates the requirement of painstakingly curated datasets and hand crafted features. However, the ability to learn multiple tasks in a sequential manner, referred to as lifelong or continual learning, remains unresolved. The search for lifelong learning algorithms creates the foundation for this work. While there has been much research conducted in supervised learning domains under lifelong learning, the reinforced lifelong learning domain remains open for much exploration. Furthermore, current implementations either concentrate on preserving information in fixed capacity networks, or propose incrementally growing networks which randomly search through an unconstrained solution space. In order to develop a comprehensive lifelong learning algorithm, it seems essential to amalgamate these approaches into a condensed algorithm which can perform both neuroevolution and constrict network growth automatically. This thesis proposes a novel algorithm for continual learning using neurogenesis in reinforcement learning agents. It builds upon existing neuroevolutionary techniques, and incorporates several new mechanisms for limiting the memory resources while expanding neural network learning capacity. The algorithm is tested on a custom set of sequential virtual environments which emulate several meaningful scenarios for intellectually down-scaled species and autonomous robots. Additionally, a library for connecting an unconstrained range of machine learning tools, in a variety of programming languages to the Unity3D simulation engine for the development of future learning algorithms and environments, is also proposed.
Publisher Site: RIT Repository
PDF (Requires Sign-In): PDF
Click for Bibtex
@book{pandit2019relational, title = {Relational Neurogenesis for Lifelong Learning Agents}, author = {Pandit, Tej}, year = {2019}, school = {Rochester Institute of Technology} }
[T-2019-49] Kimberly Bernetski. (2019). “Contact Line Pinning Under AC Electrowetting.” Rochester Institute of Technology.
Abstract: Sepsis is an autoimmune disease where a bacterial infection causes organ failure. Early diagnosis is a challenge when using traditional methods. Improvement in early detection is vital in treating patients with sepsis. One method for early detection is through the use of particle deposition patterns from patient urine. Deposition patterns are the remaining particles from an evaporating droplet and can vary based on a variety of factors. To optimize sepsis detection, a digital microfluidic device can be used to manipulate the deposition pattern of a sample to detect target proteins. These devices use microarrays for improved biomarker detection. Microarrays consist of arrays of hundreds or even thousands of sessile droplets on a substrate and can be used in several biological applications. These microarrays rely on the application of an electric field to control particle deposition. Recent efforts have examined the effects of applying electric fields to evaporating droplets to actively control colloidal transport and deposition in evaporating droplets. To improve target protein detection, electrowetting on dielectric (EWOD) is performed to manipulate particle deposition patterns from evaporating droplets. A further understanding of the affects an electric field has on an evaporating droplet would improve device sensitivity. The ability to manipulate the contact line of a droplet is a critical factor in determining fluid dynamics in a droplet. The dynamics of an evaporating droplet ultimately determine the transport and deposition of particles. This thesis focuses on accurately quantifying the forces that affect droplets with and without particles when EWOD. Understanding the forces acting on a droplet will assist in improving manipulating particle deposition patterns. With this goal in mind, the following has been accomplished within this thesis: proposed a new experimentally validated model for hysteresis under AC electrowetting, verified the proposed model on a variety of different surfaces suitable to EWOD, and performed a preliminary investigation to the effects of particles on hysteresis. Manufacturing costs limit the availability of many LOC devices as a medical diagnostic tool. Typically, a cleanroom facility with expensive equipment and processing is required to manufacture LOC devices. A potential alternative would be to use an inkjet printer and conductive ink to print electrodes at a much lower cost. These devices could then be coated with a dielectric and hydrophobic layer outside a cleanroom. This thesis verifies if inkjet-printed (IJP) devices are a feasible substitute for cleanroom-fabricated (CRF) devices as EWOD devices.
Publisher Site: RIT Repository
PDF (Requires Sign-In): PDF
Click for Bibtex
@book{bernetski2019contact, title = {Contact Line Pinning Under AC Electrowetting}, author = {Bernetski, Kimberly}, year = {2019}, school = {Rochester Institute of Technology} }
[T-2019-48] Christopher R. Sweet. (2019). “Synthesizing Cyber Intrusion Alerts using Generative Adversarial Networks.” Rochester Institute of Technology.
Abstract: Cyber attacks infiltrating enterprise computer networks continue to grow in number, severity, and complexity as our reliance on such networks grows. Despite this, proactive cyber security remains an open challenge as cyber alert data is often not available for study. Furthermore, the data that is available is stochastically distributed, imbalanced, lacks homogeneity, and relies on complex interactions with latent aspects of the network structure. Currently, there is no commonly accepted way to model and generate synthetic alert data for further study; there are also no metrics to quantify the fidelity of synthetically generated alerts or identify critical attributes within the data. This work proposes solutions to both the modeling of cyber alerts and how to score the fidelity of such models. Generative Adversarial Networks are employed to generate cyber alert data taken from two collegiate penetration testing competitions. A list of criteria defining desirable attributes for cyber alert data metrics is provided. Several statistical and information-theoretic metrics, such as histogram intersection and conditional entropy, meet these criteria and are used for analysis. Using these metrics, critical relationships of synthetically generated alerts may be identified and compared to data from the ground truth distribution. Finally, through these metrics, we show that adding a mutual information constraint to the model's generation increases the quality of outputs and successfully captures alerts that occur with low probability.
Publisher Site: RIT Repository
PDF (Requires Sign-In): PDF
Click for Bibtex
@book{sweet2019synthesizing, title = {Synthesizing Cyber Intrusion Alerts using Generative Adversarial Networks}, author = {Sweet, Christopher R.}, year = {2019}, school = {Rochester Institute of Technology} }
[T-2019-47] Andrew Robert Bear. (2019). “A Novel Processing-In-Memory Architecture for Dense and Sparse Matrix Multiplications.” Rochester Institute of Technology.
Abstract: Modern processing speeds in conventional Von Neumann architectures are severely limited by memory access speeds. Read and write speeds of main memory have not scaled at the same rate as logic circuits. In addition, the large physical distance spanned by the interconnect between the processor and the memory incurs a large RC delay and power penalty, often a hundred times more than on chip interconnects. As a result, accessing data from memory becomes a bottleneck in the overall performance of the processor. Operations such as matrix multiplication, which are used extensively in many modern applications such as solving systems of equations, Convolutional Neural Networks, and image recognition, require large volumes of data to be processed. These operations are impacted the most by this bottleneck and their performance is limited as a result. Processing-in-Memory (PIM) is designed to overcome this bottleneck by performing repeated data intensive operations on the same die as the memory. In doing so, the large delay and power penalties caused by data transfers between the processor and the memory can be avoided. PIM architectures are often designed as small, simple, and efficient processing blocks such that they can be integrated into each block of the memory. This allows for extreme parallelism to be achieved, which makes it ideal for big data processes. An issue with this design paradigm, however, is the lack of flexibility in operations that can be performed. Most PIM architectures are designed to perform application specific functions, limiting their widespread use. A novel PIM architecture is proposed which allows for arbitrary functions to be implemented with a high degree of parallelism. The architecture is based on PIM cores which are capable of performing any arbitrary function on two 4-bit inputs. Nine PIM cores are connected together to allow more advanced functions such as an 8-bit Multiply-Accumulate function to be implemented. Wireless interconnects are utilized in the design to aid in communication between clusters. The architecture will be applied to perform matrix multiplication on dense and sparse matrices of 8-bit values, which are prevalent in image and video formats. An analytical model is proposed to evaluate the area, power, and timing of the PIM architecture for both dense and sparse matrices. A real-world performance evaluation will also be conducted by applying the models to image/video data in a standard resolution to examine the timing and power consumption of the system. The results are compared against CPU and GPU results to evaluate the architecture against traditional implementations. The proposed architecture was found to have an execution time similar to a GPU implementation while requiring significantly less power.
Publisher Site: RIT Repository
PDF (Requires Sign-In): PDF
Click for Bibtex
@book{bear2019a, title = {A Novel Processing-In-Memory Architecture for Dense and Sparse Matrix Multiplications}, author = {Bear, Andrew Robert}, year = {2019}, school = {Rochester Institute of Technology} }
[T-2019-46] Yogesh Karnam. (2019). “Multiscale Fluid-Structure Interaction Models Development and Applications to the 3D Elements of a Human Cardiovascular System.” Rochester Institute of Technology.
Abstract: Cardiovascular diseases (CVD) are the number one cause of death of humans in the United States and worldwide. Accurate, non-invasive, and cheaper diagnosis methods have always been on demand as cardiovascular monitoring increase in prevalence. The primary causes of the various forms of these CVDs are atherosclerosis and aneurysms in the blood vessels. Current noninvasive methods (i.e., statistical/medical) permit fairly accurate detection of the disease once clinical symptoms are suggestive of the existence of hemodynamic disorders. Therefore, the recent surge of hemodynamics models facilitated the prediction of cardiovascular conditions. The hemodynamic modeling of a human circulatory system involves varying levels of complexity which must be accounted for and resolved. Pulse-wave propagation effects and high aspect-ratio segments of the vasculature are represented using a quasi-one-dimensional (1D), non-steady, averaged over the cross-section models. However, these reduced 1D models do not account for the blood flow patterns (recirculation zones), vessel wall shear stresses and quantification of repetitive mechanical stresses which helps to predict a vessel life. Even a whole three-dimensional (3D) modeling of the vasculature is computationally intensive and do not fit the timeline of practical use. Thus the intertwining of a quasi 1D global vasculature model with a specific/risk-prone 3D local vessel ones is imperative. This research forms part of a multiphysics project that aims to improve the detailed understanding of the hemodynamics by investigating a computational model of fluid-structure interaction (FSI) of in vivo blood flow. First idealized computational a 3D FSI artery model is configured and executed in ANSYS Workbench, forming an implicit coupling of the blood flow and vessel walls. Then the thesis focuses on an approach developed to employ commercial tools rather than in-house mathematical models in achieving multiscale simulations. A robust algorithm is constructed to combine stabilization techniques to simultaneously overcome the added-mass effect in 3D FSI simulation and mathematical difficulties such as the assignment of boundary conditions at the interface between the 3D-1D coupling. Applications can be of numerical examples evaluating the change of hemodynamic parameters and diagnosis of an abdominal aneurysm, deep vein thrombosis, and bifurcation areas.
Publisher Site: RIT Repository
PDF (Requires Sign-In): PDF
Click for Bibtex
@book{karnam2019multiscale, title = {Multiscale Fluid-Structure Interaction Models Development and Applications to the 3D Elements of a Human Cardiovascular System}, author = {Karnam, Yogesh}, year = {2019}, school = {Rochester Institute of Technology} }
[T-2019-45] Anurag Daram. (2019). “Exploring Neuromodulatory Systems for Dynamic Learning.” Rochester Institute of Technology.
Abstract: In a continual learning system, the network has to dynamically learn new tasks from few samples throughout its lifetime. It is observed that neuromodulation acts as a key factor in continual and dynamic learning in the central nervous system. In this work, the neuromodulatory plasticity is embedded with dynamic learning architectures. The network has an inbuilt modulatory unit that regulates learning depending on the context and the internal state of the system, thus rendering the networks with the ability to self modify their weights. In one of the proposed architectures, ModNet, a modulatory layer is introduced in a random projection framework. This layer modulates the weights of the output layer neurons in tandem with hebbian learning. Moreover, to explore modulatory mechanisms in conjunction with backpropagation in deeper networks, a modulatory trace learning rule is introduced. The proposed learning rule, uses a time dependent trace to automatically modify the synaptic connections as a function of ongoing states and activations. The trace itself is updated via simple plasticity rules thus reducing the demand on resources. A digital architecture is proposed for ModNet, with on-device learning and resource sharing, to facilitate the efficacy of dynamic learning on the edge. The proposed modulatory learning architecture and learning rules demonstrate the ability to learn from few samples, train quickly, and perform one shot image classification in a computationally efficient manner. The ModNet architecture achieves an accuracy of ∼91% for image classification on the MNIST dataset while training for just 2 epochs. The deeper network with modulatory trace achieves an average accuracy of 98.8%±1.16 on the omniglot dataset for five-way one-shot image classification task. In general, incorporating neuromodulation in deep neural networks shows promise for energy and resource efficient lifelong learning systems.
Publisher Site: RIT Repository
PDF (Requires Sign-In): PDF
Click for Bibtex
@book{daram2019exploring, title = {Exploring Neuromodulatory Systems for Dynamic Learning}, author = {Daram, Anurag}, year = {2019}, school = {Rochester Institute of Technology} }
[T-2019-44] Kevin Millar. (2019). “Design of a Flexible Schoenhage-Strassen FFT Polynomial Multiplier with High-Level Synthesis.” Rochester Institute of Technology.
Abstract: Homomorphic Encryption (HE) is a promising field because it allows for encrypted data to be sent to and operated on by untrusted parties without the risk of privacy compromise. The benefits and applications of HE are far reaching, especially in regard to cloud computing. However, current HE solutions require resource intensive arithmetic operations such as high precision, high degree polynomial multiplication resulting in a minimum computational complexity of O(n log(n)) on standard CPUs though application of the Fast Fourier Transform (FFT). These operations result in poor overall performance for HE schemes in software and would benefit greatly from hardware acceleration. This work aims to accelerate the multi-precision arithmetic operations used in HE with specific focus on an implementation of the Schönhage-Strassen FFT based multiplication algorithm. It is to be incorporated into a larger HE library of arithmetic functions tuned for High Level Synthesis (HLS) that enables flexible solutions for hardware/software systems on reconfigurable cloud resources. Although this project was inspired by HE, it could be incorporated within a generic mathematical library and support other domains. The developed FFT based polynomial multiplier exhibits flexibility in the selection of security parameters facilitating its use in a wide range of HE schemes and applications. The design also displayed substantial speedup over the polynomial multiplication functions implemented in the Number Theory Library (NTL) utilized by software based HE solutions.
Publisher Site: RIT Repository
PDF (Requires Sign-In): PDF
Click for Bibtex
@book{millar2019design, title = {Design of a Flexible Schoenhage-Strassen FFT Polynomial Multiplier with High-Level Synthesis}, author = {Millar, Kevin}, year = {2019}, school = {Rochester Institute of Technology} }
[T-2019-43] Sajeed Mohammad Shahriat. (2019). “Global Congestion and Fault Aware Wireless Interconnection Framework for Multicore Systems.” Rochester Institute of Technology.
Abstract: Multicore processors are getting more common in the implementation of all type of computing demands, starting from personal computers to the large server farms for high computational demanding applications. The network-on-chip provides a better alternative to the traditional bus based communication infrastructure for this multicore system. Conventional wire-based NoC interconnect faces constraints due to their long multi-hop latency and high power consumption. Furthermore high traffic generating applications sometimes creates congestion in such system further degrading the systems performance. In this thesis work, a novel two-state congestion aware wireless interconnection framework for network chip is presented. This WiNoC system was designed to able to dynamically redirect traffic to avoid congestion based on network condition information shared among all the core tiles in the system. Hence a novel routing scheme and a two-state MAC protocol is proposed based on a proposed two layer hybrid mesh-based NoC architecture. The underlying mesh network is connected via wired-based interconnect and on top of that a shared wireless interconnect framework is added for single-hop communication. The routing scheme is non-deterministic in nature and utilizes the principles from existing dynamic routing algorithms. The MAC protocol for the wireless interface works in two modes. The first is data mode where a token-based protocol is utilized to transfer core data. And the second mode is the control mode where a broadcast-based communication protocol is used to share the network congestion information. The work details the switching methodology between these two modes and also explain, how the routing scheme utilizes the congestion information (gathered during the control mode) to route data packets during normal operation mode. The proposed work was modeled in a cycle accurate network simulator and its performance were evaluated against traditional NoC and WiNoC designs.
Publisher Site: RIT Repository
PDF (Requires Sign-In): PDF
Click for Bibtex
@book{shahriat2019global, title = {Global Congestion and Fault Aware Wireless Interconnection Framework for Multicore Systems}, author = {Shahriat, Sajeed Mohammad}, year = {2019}, school = {Rochester Institute of Technology} }
[T-2019-42] Jonathan McClure. (2019). “A Low-Cost Search-and-Rescue Drone Platform.” Rochester Institute of Technology.
Abstract: In this work, an unmanned aerial system is implemented to search an outdoor area for an injured or missing person (subject) without requiring a connection to a ground operator or control station. The system detects subjects using exclusively on-board hardware as it traverses a predefined search path, with each implementation envisioned as a single element of a larger swarm of identical search drones. To increase the affordability of such a swarm, the system cost per drone serves as a primary constraint. Imagery is streamed from a camera to an Odroid single-board computer, which prepares the data for inference by a Neural Compute Stick vision accelerator. A single-class TinyYolo network, trained on the Okutama-Action dataset and an original Albatross dataset, is utilized to detect subjects in the prepared frames. The final network achieves 7.6 FPS in the field (8.64 FPS on the bench) with an 800x480 input resolution. The detection apparatus is mounted on a drone and field tests validate the system feasibility and efficacy.
Publisher Site: RIT Repository
PDF (Requires Sign-In): PDF
Click for Bibtex
@book{mcclure2019a, title = {A Low-Cost Search-and-Rescue Drone Platform}, author = {McClure, Jonathan}, year = {2019}, school = {Rochester Institute of Technology} }
[T-2019-41] Odysseus A. Adamides. (2019). “A Time of Flight on-Robot Proximity Sensing System for Collaborative Robotics.” Rochester Institute of Technology.
Abstract: The sensor system presented in this work demonstrates the results of designing an industrial grade exteroceptive sensing device for proximity sensing for collaborative robots. The intention of this design's application is to develop an on-robot small footprint proximity sensing device to prevent safety protected stops from halting a robot during a manufacturing process. Additionally, this system was design to be modular and fit on an size or shape robotic link expanding the sensor system's use cases vastly. The design was assembled and put through a number of benchmark tests to validate the performance of the time of flight (ToF) sensor system when used in proximity sensing: Single Sensor Characterization, Sensor Overlap Characterization, and Sensor Ranging Under Motion. Through these tests, the ToF sensor ring achieves real time data throughput while minimizing blind spots. Lastly, the sensor system was tested at a maximum throughput load of 32 ToF sensors and maintained a stable throughput of data from all sensors in real time.
Publisher Site: RIT Repository
PDF (Requires Sign-In): PDF
Click for Bibtex
@book{adamides2019a, title = {A Time of Flight on-Robot Proximity Sensing System for Collaborative Robotics}, author = {Adamides, Odysseus A.}, year = {2019}, school = {Rochester Institute of Technology} }
[T-2019-40] Chandini Ramesh. (2019). “A Comparative Assessment of the Impact of Various Norms on Wasserstein Generative Adversarial Networks.” Rochester Institute of Technology.
Abstract: Generative Adversarial Networks (GANs) provide a fascinating new paradigm in machine learning and artificial intelligence, especially in the context of unsupervised learning. GANs are quickly becoming a state of the art tool, used in various applications such as image generation, image super resolutions, text generation, text to image synthesis to name a few. However, GANs potential is restricted due to the various training difficulties. To overcome the training difficulties of GANs, the use of a more powerful measure of dissimilarity via the use of the Wasserstein distance was proposed. Thereby giving birth to the GAN extension known as Wasserstein Generative Adversarial Networks (WGANs). Recognizing the crucial and central role played by both the cost function and the order of the Wasserstein distance used in WGAN, this thesis seeks to provide a comparative assessment of the effect of a various common used norms on WGANs. Inspired by the impact of norms like the L1-norm in LASSO Regression, the L2-norm Ridge Regression and the great success of the combination of the L1 and L2 norms in elastic net and its extensions in statistical machine learning, we consider exploring and investigating to a relatively large extent, the effect of these very same norms in the WGAN context. In this thesis, the primary goal of our research is to study the impact of these norms on WGANs from a pure computational and empirical standpoint, with an emphasis on how each norm impacts the space of the weights/parameters of the machines contributing to the WGAN. We also explore the effect of different clipping values which are used to enforce the k-Lipschitz constraint on the functions making up the specific WGAN under consideration. Another crucial component of the research carried out in this thesis focuses on the impact of the number of training iterations on the WGAN loss function (objective function) which somehow gives us an empirical rough estimate of the computational complexity of WGANs. Finally, and quite importantly, in keeping WGANs' application to recovery of scenes and reconstruction of complex images, we dedicate a relative important part of our research to the comparison of the quality of recovery across various choices of the norms considered. Like previous researchers before us, we perform a substantial empirical exploration on both synthetic data and real life data. We specifically explore a simulated data set made up of a mixture of eight bivariate Gaussian random variables with large gaps, the likes of which would be hard task for traditional GANs but can be readily handled quite well be WGANs thanks to the inherent strength/power of the underlying Wasserstein distance. We also explore various real data sets, namely the ubiquitous MNIST datasets made up of handwritten digits and the now very popular CIFAR-10 dataset used an de facto accepted benchmark data set for GANs and WGANs. For all the datasets, synthetic and real, we provide a thorough comparative assessment of the effect and impact of the norms mentioned earlier, and it can be readily observed that there are indeed qualitative and quantitative difference from one norm to another, with differences established via measures such as (a) shape, value and pattern of the generator loss, (b) shape, value and pattern of the discriminator loss (c) shape, value and pattern of the inception score, and (d) human inspection of quality of recovery or reconstruction of images and scenes.
Publisher Site: RIT Repository
PDF (Requires Sign-In): PDF
Click for Bibtex
@book{ramesh2019a, title = {A Comparative Assessment of the Impact of Various Norms on Wasserstein Generative Adversarial Networks}, author = {Ramesh, Chandini}, year = {2019}, school = {Rochester Institute of Technology} }
[T-2019-39] Sari Houchaimi. (2019). “Performing DNA ligation on a low-cost inkjet-printed digital microfluidic device.” Rochester Institute of Technology.
Abstract: DNA Synthesis is a critical component in many biological and medical applications. Unfortunately, the production of DNA is tedious, time consuming, and expensive. To accelerate the production times and lower the cost, we take a closer look at the potential application of digital microfluidics for this process. Microfluidics involves manipulating small volumes of fluid (microliters). It takes advantage of the relative dominance of forces such as surface tension and capillary forces at the submillimeter scale. This allows for lower reagent consumption and shorter reaction times. The technology is also portable and can accommodate for various functions to be performed on the device itself. A particularly appealing focus of this field is Digital Microfluidics (DMF). Digital Microfluidics (DMF) is a relatively recent technology praised for its fast analysis times and small volume requirements (microliters). An obstacle to the production of DNA chains using traditional methods of nucleotide synthesis is the requirement of acetonitrile, which can't consistently be manipulated on DMF. Another obstacle to overcome is the accurate production of long chains of nucleic acids (3000 to 5000 base pair products), much longer than the DNA products used in a typical ELISA assay. For the sake of this project we are partnering with Nuclera Nucleics, a company based in the United Kingdom working on a next-generation DNA synthesis and automation platform. The company has created a novel way of synthesizing DNA using aqueous chemistry. Collaborating with them, we propose to build a DMF device that will perform oligonucleotide synthesis. The first step towards this goal is to verify that DNA ligation can be executed on a DMF device. This device will make DNA synthesis more accessible and significantly reduce production times in the laboratory. This will lead to more advancements in the field of genetics, drug delivery and other biomedical applications.
Publisher Site: RIT Repository
PDF (Requires Sign-In): PDF
Click for Bibtex
@book{houchaimi2019performing, title = {Performing DNA ligation on a low-cost inkjet-printed digital microfluidic device}, author = {Houchaimi, Sari}, year = {2019}, school = {Rochester Institute of Technology} }
[T-2019-38] Zachariah J.L. Carmichael. (2019). “Towards Lightweight AI: Leveraging Stochasticity, Quantization, and Tensorization for Forecasting.” Rochester Institute of Technology.
Abstract: The deep neural network is an intriguing prognostic model capable of learning meaningful patterns that generalize to new data. The deep learning paradigm has been widely adopted across many domains, including for natural language processing, genomics, and automatic music transcription. However, deep neural networks rely on a plethora of underlying computational units and data, collectively demanding a wealth of compute and memory resources for practical tasks. This model complexity prohibits the use of larger deep neural networks for resource-critical applications, such as edge computing. In order to reduce model complexity, several research groups are actively studying compression methods, hardware accelerators, and alternative computing paradigms. These orthogonal research explorations often leave a gap in understanding the interplay of the optimization mechanisms and their overall feasibility for a given task. In this thesis, we address this gap by developing a holistic solution to assess the model complexity reduction theoretically and quantitatively at both high-level and low-level abstractions for training and inference. At the algorithmic level, a novel deep, yet lightweight, recurrent architecture is proposed that extends the conventional echo state network. The architecture employs random dynamics, brain-inspired plasticity mechanisms, tensor decomposition, and hierarchy as the key features to enrich learning. Furthermore, the hyperparameter landscape is optimized via a particle swarm optimization algorithm. To deploy these networks efficiently onto low-end edge devices, both ultra-low and mixed-precision numerical formats are studied within our feedforward deep neural network hardware accelerator. More importantly, the tapered-precision posit format with a novel exact-dot-product algorithm is employed in the low-level digital architectures to study its efficacy in resource utilization. The dynamics of the architecture are characterized through neuronal partitioning and Lyapunov stability, and we show that superlative networks emerge beyond the "edge of chaos" with an agglomeration of weak learners. We also demonstrate that tensorization improves model performance by preserving correlations present in multi-way structures. Low-precision posits are found to consistently outperform other formats on various image classification tasks and, in conjunction with compression, we achieve magnitudes of speedup and memory savings for both training and inference for the forecasting of chaotic time series and polyphonic music tasks. This culmination of methods greatly improves the feasibility of deploying rich predictive models on edge devices.
Publisher Site: RIT Repository
PDF (Requires Sign-In): PDF
Click for Bibtex
@book{carmichael2019towards, title = {Towards Lightweight AI: Leveraging Stochasticity, Quantization, and Tensorization for Forecasting}, author = {Carmichael, Zachariah J.L.}, year = {2019}, school = {Rochester Institute of Technology} }
[T-2019-37] Frank C. Cwitkowitz. (2019). “End-to-End Music Transcription Using Fine-Tuned Variable-Q Filterbanks.” Rochester Institute of Technology.
Abstract: The standard time-frequency representations calculated to serve as features for musical audio may have reached the extent of their effectiveness. General-purpose features such as Mel-Frequency Spectral Coefficients or the Constant-Q Transform, while being pyschoacoustically and musically motivated, may not be optimal for all tasks. As large, comprehensive, and well-annotated musical datasets become increasingly available, the viability of learning from the raw waveform of recordings widens. Deep neural networks have been shown to perform feature extraction and classification jointly. With sufficient data, optimal filters which operate in the time-domain may be learned in place of conventional time-frequency calculations. Since the spectrum of problems studied by the Music Information Retrieval community are vastly different, rather than relying on the fixed frequency support of each bandpass filter within standard transforms, learned time-domain filters may prioritize certain harmonic frequencies and model note behavior differently based on a specific music task. In this work, the time-frequency calculation step of a baseline transcription architecture is replaced with a learned equivalent, initialized with the frequency response of a Variable-Q Transform. The learned replacement is fine-tuned jointly with a baseline architecture for the task of piano transcription, and the resulting filterbanks are visualized and evaluated against the standard transform.
Publisher Site: RIT Repository
PDF (Requires Sign-In): PDF
Click for Bibtex
@book{cwitkowitz2019end-to-end, title = {End-to-End Music Transcription Using Fine-Tuned Variable-Q Filterbanks}, author = {Cwitkowitz, Frank C.}, year = {2019}, school = {Rochester Institute of Technology} }
[T-2019-36] Kayla Stephan. (2019). “State Policies of Medical Marijuana versus Food & Drug Administration Policies of Pharmaceutical Drugs.” Rochester Institute of Technology.
Abstract: In the past 22 years, 32 states have legalized and regulated marijuana for medical use. However, marijuana is scheduled as a Schedule I drug according to the federal government. This means that states have no specific regulations to follow for regulating marijuana for medical use. Because of this, states may be risking the safety of medical marijuana patients. Research was conducted to analyze the policies set out by the Food & Drug Administration (FDA) regarding the regulation of a prescription drug. Since the FDA is responsible for the safety and efficacy of prescription drugs, this analysis included what types of risks were mitigated by FDA policies. State policies on medical marijuana were then compared to FDA policies in order to determine if aforementioned risks are being acknowledged and mitigated by states. This research found that states are implementing some policies similar to aspects of FDA regulations, but states are not eliminating nearly as many safety risks that the FDA focuses on eliminating. States are, however, creating additional policies that encompass social issues regarding the legalization of medical marijuana, which the FDA doesn't do, which could be allowing medical safety to be analyzed in a broader social context.
Publisher Site: RIT Repository
PDF (Requires Sign-In): PDF
Click for Bibtex
@book{stephan2019state, title = {State Policies of Medical Marijuana versus Food & Drug Administration Policies of Pharmaceutical Drugs}, author = {Stephan, Kayla}, year = {2019}, school = {Rochester Institute of Technology} }
[T-2019-35] Humza Syed. (2019). “Performance Analysis of Fixed-Random Weights in Artificial Neural Networks.” Rochester Institute of Technology.
Abstract: Deep neural networks train millions of parameters to achieve state-of-the-art performance on a wide foray of applications. However, finding a global minimum with gradient descent approaches leads to lengthy training times coupled with high computational resource requirements. To alleviate these concerns, the idea of fixed-random weights in deep neural networks is explored. More critically the goal is to maintain performance akin to fully trained models. Metrics such as floating point operations per second and memory size are compared and contrasted for fixed-random and fully trained models. Additional analysis on downsized models that mimic the number of trained parameters of the fixed-random models, shows that fixed-random weights enable slightly higher performance. In a fixed-random convolutional model, ResNet achieves ∼57% image classification accuracy on CIFAR-10. In contrast, a DenseNet architecture, with only fixed-random filters in the convolutional layers, achieves ∼88% accuracy for the same task. DenseNet's fully trained model achieves ∼96% accuracy, which highlights the importance of architectural choice for a high performing model. To further understand the role of architectures, random projection networks trained using a least squares approximation learning rule are studied. In these networks, deep random projection layers and skipped connections are exploited as they are shown to boost the overall network performance. In several of the image classification experiments conducted, additional layers and skipped connectivity consistently outperform a baseline random projection network by 1% to 3%. To reduce the complexity of the models in general, a tensor decomposition technique, known as the Tensor-Train decomposition, is leveraged. The compression of the fully-connected hidden layer leads to a minimum ∼40x decrease in memory size at a slight cost in resource utilization. This research study helps to gain a better understanding of how random filters and weights can be utilized to obtain lighter models.
Publisher Site: RIT Repository
PDF (Requires Sign-In): PDF
Click for Bibtex
@book{syed2019performance, title = {Performance Analysis of Fixed-Random Weights in Artificial Neural Networks}, author = {Syed, Humza}, year = {2019}, school = {Rochester Institute of Technology} }
[T-2019-34] Sultan Mira. (2019). “Task Scheduling Balancing User Experience and Resource Utilization on Cloud.” Rochester Institute of Technology.
Abstract: Cloud computing has been gaining undeniable popularity over the last few years. Among many techniques enabling cloud computing, task scheduling plays a critical role in both efficient resource utilization for cloud service providers and providing an excellent user experience to the clients. In this study, we proposed a priority cloud task scheduling approach that considers users input to calculate priority, while at the same time, efficiently utilizes available resources. This approach is designed for the consideration of both user satisfaction and utilization of cloud services. In the proposed approach, clients will be required to input their time and cost preferences to determine the priority of each task. We conducted our experiments in Python and AWS to best simulate a real-world cloud environment and compared the proposed approach to a first-come-first-serve approach. We measured the performance of our approach in terms of average task wait time AWT, average resource idle time aRIT, and the order the tasks were scheduled. The experimental results show that our approach outperforms the first-come-first-serve approach in AWT,aRIT, and the order the tasks were scheduled.
Publisher Site: RIT Repository
PDF (Requires Sign-In): PDF
Click for Bibtex
@book{mira2019task, title = {Task Scheduling Balancing User Experience and Resource Utilization on Cloud}, author = {Mira, Sultan}, year = {2019}, school = {Rochester Institute of Technology} }
[T-2019-33] Long Pham. (2019). “Joint Source-Channel Coding for Image Transmission over Underlay Multichannel Cognitive Radio Networks.” Rochester Institute of Technology.
Abstract: The increasing prominence of wireless applications exacerbates the problem of radio spectrum scarcity and promotes the usage of Cognitive Radio (CR) in wireless networks. With underlay dynamic spectrum access, CRs can operate alongside Primary Users, the incumbent of a spectrum band, as long as they limit the interference to the Primary Users below a certain threshold. Multimedia streaming transmissions face stringent Quality of Services constraints on top of the CR interference constraints, as some packets in the data stream have higher levels of importance and are the most vulnerable to packet loss over the channel. This raises a need for Unequal Error Protection (ULP) for multimedia streams transmissions, in which the channel encoder assigns different amount of error correction to different parts of the data stream, thereby protecting more the most valuable parts of the stream from packet loss problems. This research presents an end-to-end system setup for image transmission, utilizing ULP as part of a Joint Source-Channel Coding scheme over a multichannel CR network operating through underlay dynamic spectrum access. The setup features a Set Partitioning in Hierarchical Trees (SPIHT) source encoder, and Reed-Solomon forward error correction channel coding, and uses their properties to devise an ULP framework that maximizes the quality of the received image.
Publisher Site: RIT Repository
PDF (Requires Sign-In): PDF
Click for Bibtex
@book{pham2019joint, title = {Joint Source-Channel Coding for Image Transmission over Underlay Multichannel Cognitive Radio Networks}, author = {Pham, Long}, year = {2019}, school = {Rochester Institute of Technology} }
[T-2019-32] Prathibha Rama. (2019). “Exploring the Effectiveness of Privacy Preserving Classification in Convolutional Neural Networks.” Rochester Institute of Technology.
Abstract: A front-runner in modern technological advancement, machine learning relies heavily on the use of personal data. It follows that, when assessing the scope of confidentiality for machine learning models, understanding the potential role of encryption is critical. Convolutional Neural Networks (CNN) are a subset of artificial feed-forward neural networks tailored specifically for image recognition and classification. As the popularity of CNN increases, so too does the need for privacy preserving classification. Homomorphic Encryption (HE) refers to a cryptographic system that allows for computation on encrypted data to obtain an encrypted result such that, when decrypted, the result is the same value that would have been obtained if the operations were performed on the original unencrypted data. The objective of this research was to explore the application of HE alongside CNN with the creation of privacy-preserving CNN layers that have the ability to operate on encrypted images. This was accomplished through (1) researching the underlying structure of preexisting privacy-preserving CNN classifiers, (2) creating privacy-preserving convolution, pooling, and fully-connected layers by mapping the computations found within each layer to a space of homomorphic computations, (3) developing a polynomial-approximated activation function and creating a privacy-preserving activation layer based on this approximation, (4) testing and profiling the designed application to asses efficiency, performance, accuracy, and overall practicality.
Publisher Site: RIT Repository
PDF (Requires Sign-In): PDF
Click for Bibtex
@book{rama2019exploring, title = {Exploring the Effectiveness of Privacy Preserving Classification in Convolutional Neural Networks}, author = {Rama, Prathibha}, year = {2019}, school = {Rochester Institute of Technology} }
[T-2019-31] Gerald Kotas. (2019). “Exploration of GPU Cache Architectures Targeting Machine Learning Applications.” Rochester Institute of Technology.
Abstract: The computation power from graphics processing units (GPUs) has become prevalent in many fields of computer engineering. Massively parallel workloads and large data set capabilities make GPUs an essential asset in tackling today's computationally intensive problems. One field that benefited greatly with the introduction of GPUs is machine learning. Many applications of machine learning use algorithms that show a significant speedup on a GPU compared to other processors due to the massively parallel nature of the problem set. The existing cache architecture, however, may not be ideal for these applications. The goal of this thesis is to determine if a cache architecture for the GPU can be redesigned to better fit the needs of this increasingly popular field of computer engineering. This work uses a cycle accurate GPU simulator, Multi2Sim, to analyze NVIDIA GPU architectures. The architectures are based on the Kepler series, but the flexibility of the simulator allows for emulation of newer features. Changes have been made to source code to expand on the metrics recorded to further the understanding of the cache architecture. Two suites of benchmarks were used: one for general purpose algorithms and another for machine learning. Running the benchmarks with various cache configurations led to insight into the effects the cache architecture had on each of them. Analysis of the results shows that the cache architecture, while beneficial to the general purpose algorithms, does not need to be as complex for machine learning algorithms. A large contributor to the complexity is the cache coherence protocol used by GPUs. Due to the high spacial locality associated with machine learning problems, the overhead needed by implementing the coherence protocol has little benefit, and simplifying the architecture can lead to smaller, cheaper, and more efficient designs.
Publisher Site: RIT Repository
PDF (Requires Sign-In): PDF
Click for Bibtex
@book{kotas2019exploration, title = {Exploration of GPU Cache Architectures Targeting Machine Learning Applications}, author = {Kotas, Gerald}, year = {2019}, school = {Rochester Institute of Technology} }
[T-2019-30] Yidan Yang. (2019). “Low-Rank Multivariate General Linear Model and One-Way Random Effect Models for Brain Response Analysis.” Rochester Institute of Technology.
Abstract: Human brain is the central organ of human nervous system, the activity in brain becomes a significant topic in neuroscience and medical science field. New techniques for detecting brain regions activity has been developed very fast in recent year, a basic method is functional MRIs which can measure brain activities based on oxygen level in bloodstream. This work will introduce a new approach to analyze brain region relationships through low-rank multivariate general linear model and one-way random effect model. By using fMRI and low-rank multivariate general liner model, this model contains a new penalized optimization function, which can lead to smooth HRF (Hemodynamic response functions) temporally and spatially. Also, this new model is flexible to characterize variation across different regions and stimulus types, moreover, it enables information across voxels and use fewer parameters. After analysis our fMRI data through low-rank multivariate general linear model, we apply one-way random effect model to analyze the brain regions connection via multiple subjects.
Publisher Site: RIT Repository
PDF (Requires Sign-In): PDF
Click for Bibtex
@book{yang2019low-rank, title = {Low-Rank Multivariate General Linear Model and One-Way Random Effect Models for Brain Response Analysis}, author = {Yang, Yidan}, year = {2019}, school = {Rochester Institute of Technology} }
[T-2019-29] Samantha “Emma” Sarles. (2019). “Development of Topography Monitors for Inhaled Nicotine Delivery Systems.” Rochester Institute of Technology.
Abstract: Significance – Tobacco related disease is the leading cause of preventable death in the United States. The global inhaled tobacco product market is rapidly evolving as new products such as ‘electronic cigarettes' and ‘heat not burn' grow in popularity around the world. It is not yet fully understood whether these relatively recent tobacco products will have a negative, neutral, or positive impact on public health in the USA and around the world. This thesis is focused on the design, development, and deployment of a family of monitors which can be deployed with users in their natural environment to better understand how these products may shift user behavior, and ultimately, health effects. This thesis is focused on topography monitoring – the observation and recording of tobacco user's product use patterns including number and time of puffs and individual puff flow rate, duration, and volume. Topography monitors developed with evidence driven ergonomic and aesthetic considerations allow the improvement the accuracy of collecting users' true ad lib behavior in their natural environment, which in turn provides accurate and reliable information to inform regulatory policy regarding tobacco products. Methods – The first step in this investigation is to define the inhaled tobacco products to be monitored. Once these products have been analyzed, findings dictate the design of the topography monitor. Monitors are then developed to satisfy ergonomic and aesthetic needs for improved user compliance and true behavior in the natural environment. A key design objective for the family of topography monitors is to reduce deviation from the user's normal product use behavior. Finally, performance metrics are identified and used to quantify user feedback, monitoring accuracy, and overall design effectiveness of each monitor in the family. Results – To date, wPUM™ monitors for hookahs, combustible cigarettes, the JUUL® e-cigarette, the NJOY vape pen, and the Standard Research Electronic Cigarette (SREC) have been developed and prototyped with this method.
Publisher Site: RIT Repository
PDF (Requires Sign-In): PDF
Click for Bibtex
@book{sarles2019development, title = {Development of Topography Monitors for Inhaled Nicotine Delivery Systems}, author = {Sarles, Samantha "Emma"}, year = {2019}, school = {Rochester Institute of Technology} }
[T-2019-28] Prasanna Reddy Pulakurthi. (2019). “Shadow Detection in Aerial Images using Machine Learning.” Rochester Institute of Technology.
Abstract: Shadows are present in a wide range of aerial images from forested scenes to urban environments. The presence of shadows degrades the performance of computer vision algorithms in a diverse set of applications such as image registration, object segmentation, object detection and recognition. Therefore, detection and mitigation of shadows is of paramount importance and can significantly improve the performance of computer vision algorithms in the aforementioned applications. There are several existing approaches to shadow detection in aerial images including chromaticity methods, texture-based methods, geometric, physics-based methods, and approaches using neural networks in machine learning. In this thesis, we developed seven new approaches to shadow detection in aerial imagery. This includes two new chromaticity based methods (i.e., Shadow Detection using Blue Illumination (SDBI) and Edge-based Shadow Detection using Blue Illumination (Edge-SDBI) and five machine learning methods consisting of two neural networks (SDNN and DIV-NN), and three convolutional neural networks (VSKCNN, SDCNN-ver1 and SDCNN ver-2). These algorithms were applied to five different aerial imagery data sets. Results were assessed using both qualitative (visual shadow masks) and quantitative techniques. Conclusions touch upon the various trades between these approaches, including speed, training, accuracy, completeness, correctness and quality.
Publisher Site: RIT Repository
PDF (Requires Sign-In): PDF
Click for Bibtex
@book{pulakurthi2019shadow, title = {Shadow Detection in Aerial Images using Machine Learning}, author = {Pulakurthi, Prasanna Reddy}, year = {2019}, school = {Rochester Institute of Technology} }
[T-2019-27] Pallavi Avinash Mayekar. (2019). “Design and Verification of a DFI-AXI DDR4 Memory PHY Bridge Suitable for FPGA Based RTL Emulation and Prototyping.” Rochester Institute of Technology.
Abstract: System on chip (SoC) designers today are emphasizing on a process which can ensure robust silicon at the first tape-out. Given the complexity of modern SoC chips, there is compelling need to have suitable run time software, such at the Linux kernel and necessary drivers available once prototype silicon is available. Emulation and FPGA prototyping systems are exemplary platforms to run the tests for designs, are naturally efficient and perform well, and enable early software development. While useful, one needs to keep in mind that emulation and FPGA prototyping systems do not run at full silicon speed. In fact, the SoC target ported to the FPGA might achieve a clock speed less than 10 MHz. While still very useful for testing and software development, this low operating speed creates challenges for connecting to external devices such as DDR SDRAM. In this paper, the DDR-PHY INTERFACE (DFI) to Advanced eXtensible Interface (AXI) Bridge is designed to support a DDR4 memory sub-system design. This bridge module is developed based on the DDR PHY Interface version 5.0 specification, and once implemented in an FPGA, it transfers command information and data between the SoC DDR Memory controller being prototypes, across the AXI bus to an FPGA specific memory controller connected to a DDR SDRAM or other physical memory external to the FPGA. This bridge module enables multi-communication with the design under test (DUT) with a synthesizable SCE-MI based infrastructure between the bridge and logic simulator. SCE-MI provides a direct mechanism to inject the specific traffic, and monitor performance of the DFI-AXI DDR4 Memory PHY Bridge. Both Emulation and FPGA prototyping platforms can use this design and its testbench.
Publisher Site: RIT Repository
PDF (Requires Sign-In): PDF
Click for Bibtex
@book{mayekar2019design, title = {Design and Verification of a DFI-AXI DDR4 Memory PHY Bridge Suitable for FPGA Based RTL Emulation and Prototyping}, author = {Mayekar, Pallavi Avinash}, year = {2019}, school = {Rochester Institute of Technology} }
[T-2019-26] Venkatesh Deenadayalan. (2019). “Fabrication of Resistive Thermo-Optic Heaters on Silicon Photonic Integrated Circuits.” Rochester Institute of Technology.
Abstract: A reliable process for the fabrication of active heater components on passive silicon photonic integrated circuits is presented in this thesis. The heater components enable modulation of the photonic circuits' optical response through the concept of Joule heating. This thesis proposes and successfully establishes an optimized process that can be entirely carried out in an academic clean room facility. The entire cycle of design, simulation and modelling, fabrication and testing is covered. Target lithographic resolution of 300 nm was achieved through process optimization (using a spin on carbon hard mask) by using i-line 365 nm lithography. A very good resolution supported by a near-uniform silicon etch enabled successful fabrication and testing of 30 chips (out of the total 36 chips (~80%)) on a 6" wafer. The second target was the successful demonstration of thermal tuning capabilities using a resistive metal heater made from 50 nm Titanium (primary) and 150 nm Aluminum (wire) (bi-layer). A full FSR (Free Spectral Range) shift of 7 nm was measured on a 400 nm double-bus ring resonator (without modulation) and a complete 7 nm shift was achieved on the same circuit with a power supply of 6V at resistance of ~700 Ω. For a 6 dBm (3.98 mW) laser supply, a -25 dBm (0.003 mW) output was measured on a basic loop-back circuit with a propagation loss of -28 dBm. The testing results in this thesis are supported by images from optical microscopes, Scanning Electron Microscopy (SEM) and simulations from Lumerical MODE, DEVICE, FDTD and INTERCONNECT solutions.
Publisher Site: RIT Repository
PDF (Requires Sign-In): PDF
Click for Bibtex
@book{deenadayalan2019fabrication, title = {Fabrication of Resistive Thermo-Optic Heaters on Silicon Photonic Integrated Circuits}, author = {Deenadayalan, Venkatesh}, year = {2019}, school = {Rochester Institute of Technology} }
[T-2019-25] Tuly Hazbar. (2019). “Task Planning and Execution for Human Robot Team Performing a Shared Task in a Shared Workspace.” Rochester Institute of Technology.
Abstract: A cyber-physical system is developed to enable a human-robot team to perform a shared task in a shared workspace. The system setup is suitable for the implementation of a tabletop manipulation task, a common human-robot collaboration scenario. The system integrates elements that exist in the physical (real) and the virtual world. In this work, we report the insights we gathered throughout our exploration in understanding and implementing task planning and execution for human-robot team.
Publisher Site: RIT Repository
PDF (Requires Sign-In): PDF
Click for Bibtex
@book{hazbar2019task, title = {Task Planning and Execution for Human Robot Team Performing a Shared Task in a Shared Workspace}, author = {Hazbar, Tuly}, year = {2019}, school = {Rochester Institute of Technology} }
[T-2019-24] Anjali K. Jogeshwar. (2019). “Tool for the Analysis of Human Interaction with Two-Dimensional Printed Imagery.” Rochester Institute of Technology.
Abstract: The study of human vision can include our interaction with objects. These studies include behavior modeling, understanding visual attention and motor guidance, and enhancing user experiences. But all these studies have one thing in common. To analyze the data in detail, researchers typically have to analyze video data frame by frame. Real world interaction data often comprises of data from both eye and hand. Analyzing such data frame by frame can get very tedious and time-consuming. A calibrated scene video from an eye-tracker captured at 120 Hz for 3 minutes has over 21,000 frames to be analyzed. Automating the process is crucial to allow interaction research to proceed. Research in object recognition over the last decade now allows eye-movement data to be analyzed automatically to determine what a subject is looking at and for how long. I will describe my research in which I developed a pipeline to help researchers analyze interaction data including eye and hand. Inspired by a semi-automated pipeline for analyzing eye tracking data, I have created a pipeline for analyzing hand grasp along with gaze. Putting both pipelines together can help researchers analyze interaction data. The hand-grasp pipeline detects skin to locate the hands, then determines what object (if any) the hand is over, and where the thumbs/fingers occluded that object. I also compare identification with recognition throughout the pipeline. The current pipeline operates on independent frames; future work will extend the pipeline to take advantage of the dynamics of natural interactions.
Publisher Site: RIT Repository
PDF (Requires Sign-In): PDF
Click for Bibtex
@book{jogeshwar2019tool, title = {Tool for the Analysis of Human Interaction with Two-Dimensional Printed Imagery}, author = {Jogeshwar, Anjali K.}, year = {2019}, school = {Rochester Institute of Technology} }
[T-2019-23] Daniel L. Edwards. (2019). “Evaluation of Pixel-scale Tunable Fabry-Perot Filters for Optical Imaging.” Rochester Institute of Technology.
Abstract: The Fabry-Perot interferometer (FPI) is a well-developed and widely used tool to control and measure wavelengths of light. In optical imaging applications, there is often a need for systems with compact, integrated, and widely tunable spectral filtering capabilities. We evaluate the performance of a novel tunable MEMS (Micro-Electro-Mechanical System) Fabry-Perot (FP) filter device intended to be monolithically integrated over each pixel of a focal plane array. This array of individually tunable FPIs have been designed to operate across the visible light spec-trum from 400-750 nm. This design can give rise to a new line of compact spectrometers with fewer moving parts and the ability to perform customizable filtering schemes at the hardware level. The original design was modeled, simulated, and fabricated but not tested and evalu-ated. We perform optical testing on the fabricated devices to measure the spectral resolution and wavelength tunability of these FP etalons. We collect the transmission spectrum through the FP etalons to evaluate their quality, finesse, and free spectral range. We then attempt to thermally actuate the expansion mechanisms in the FP cavity to validate tunability across the visible spectrum. The simulated design materials set was modified to create a more practical device for fabrication in a standard CMOS/MEMS foundry. Unfortunately, metal thin film stress and step coverage issues resulted in device heater failures, preventing actuation. This FP filter array design proves to be a viable manufacturing design for an imaging focal plane with individually tunable pixels. However, it will require more optimization and extensive electrical, optical, thermal, and mechanical testing when integrated with a detector array.
Publisher Site: RIT Repository
PDF (Requires Sign-In): PDF
Click for Bibtex
@book{edwards2019evaluation, title = {Evaluation of Pixel-scale Tunable Fabry-Perot Filters for Optical Imaging}, author = {Edwards, Daniel L.}, year = {2019}, school = {Rochester Institute of Technology} }
[T-2019-22] Rinaldo R. Izzo. (2019). “Combining Hyperspectral Imaging and Small Unmanned Aerial Systems for Grapevine Moisture Stress Assessment.” Rochester Institute of Technology.
Abstract: It has been shown that mild water deficit in grapevine contributes to wine quality, in terms of especially grape and subsequent wine flavor. Water deficit irrigation and selective harvesting are implemented to optimize quality, but these approaches require rigorous measurement of vine water status. While traditional in-field physiological measurements have made operational implementation onerous, modern small unmanned aerial systems (sUAS) have presented the unique opportunity for rigorous management across vast areas. This study sought to fuse hyperspectral remote sensing, sUAS, and sound multivariate analysis techniques for the purposes of assessing grapevine water status. High-spatial and -spectral resolution hyperspectral data were collected in the visible/near-infrared (VNIR; 400-1000nm) and short-wave infrared (SWIR; 950-2500 nm) spectral regions across three flight days at a commercial vineyard in the Finger Lakes region of upstate New York. A pressure chamber was used to collect traditional field measurements of stem water potential (ψstem) during image acquisition. We completed some preliminary exploration of spectral smoothing, signal-to-noise ratio, and calibration techniques in forging our experimental design. We then correlated our hyperspectral data with a limited stress range (wet growing season) of traditional measurements for ψstem using multiple linear regression (R2 between 0.34 and 0.55) and partial least squares regression (R2 between 0.36 and 0.39). We demonstrated statistically significant trends in our experiment, further qualifying the potential of hyperspectral data, collected via sUAS, for the purposes of grapevine water management. There was indication that the chlorophyll and carotenoid absorption regions in the VNIR, as well as several SWIR water band regions warrant further exploration. This work was limited since we did not have access to experimentally-controlled plots, and future work should ensure a full range of water stress. Ultimately, models will need validation in different vineyards with a full range of plant stress.
Publisher Site: RIT Repository
PDF (Requires Sign-In): PDF
Click for Bibtex
@book{izzo2019combining, title = {Combining Hyperspectral Imaging and Small Unmanned Aerial Systems for Grapevine Moisture Stress Assessment}, author = {Izzo, Rinaldo R.}, year = {2019}, school = {Rochester Institute of Technology} }
[T-2019-21] Peter Willis. (2019). “A Performance Analysis of the Meshed Tree Protocol and the Rapid Spanning Tree Protocol.” Rochester Institute of Technology.
Abstract: Loop avoidance is essential in switched networks to avoid broadcast storms. Logical Spanning Trees are constructed on the physical meshed topologies to overcome this issue and preserve the stability of the network. However, during topology changes as the result of a failure, frame forwarding latency or frame loss is introduced when re-converging and identifying new spanning tree paths. The Meshed Tree algorithm (MTA) offers a new approach. Meshed Trees support multiple tree branches from a single root to cut down on re-convergence latency on link failures. A Meshed Tree Protocol (MTP) based on MTA is currently under development as an IEEE standard. MTP is evaluated for convergence delay and frame loss in comparison with Rapid Spanning Tree Protocol (RSTP) on the GENI testbed.
Publisher Site: RIT Repository
PDF (Requires Sign-In): PDF
Click for Bibtex
@book{willis2019a, title = {A Performance Analysis of the Meshed Tree Protocol and the Rapid Spanning Tree Protocol}, author = {Willis, Peter}, year = {2019}, school = {Rochester Institute of Technology} }
[T-2019-20] Nicholas Bitten. (2019). “TIRS-2 and Future Thermal Instrument Band Study and Stray Light Study.” Rochester Institute of Technology.
Abstract: Landsat thermal instruments have been a significant source of data for thermal remote sensing applications, and future Landsat missions will continue this tradition. This work was designed to help inform the requirements for several parameters of future Landsat thermal instruments, and assess the impact that these parameters can have on the retrieved Land Surface Temperature (LST). Two main studies were conducted in this research. The first will investigate the impact that uncertainty in the spectral response of the bands will have on the LST product using the Split Window Algorithm. The main parameters that will be tested are the center and width of he bands. The second study will investigate the impact of stray light on LST, including different magnitudes of stray light and different combinations of in-field and out-of-field targets. The results of the band study showed that shifting of the bands seems to be have a larger impact on the LST than widening of the bands. Small shifts of only +/- 50 nm can cause errors of over 1 K in the LST. This study also showed that atmospheres with more water vapor content will have more effected than those with lower water vapor. The stray light study showed that using the stray light coefficients from TIRS-2 will not have a significant impact, when compared to the residual errors associated with the Split Window Algorithm.
Publisher Site: RIT Repository
PDF (Requires Sign-In): PDF
Click for Bibtex
@book{bitten2019tirs-2, title = {TIRS-2 and Future Thermal Instrument Band Study and Stray Light Study}, author = {Bitten, Nicholas}, year = {2019}, school = {Rochester Institute of Technology} }
[T-2019-19] Kruthika Prasanna Simha. (2019). “Improving Automatic Speech Recognition on Endangered Languages.” Rochester Institute of Technology.
Abstract: As the world moves towards a more globalized scenario, it has brought along with it the extinction of several languages. It has been estimated that over the next century, over half of the world's languages will be extinct, and an alarming 43% of the world's languages are at different levels of endangerment or extinction already. The survival of many of these languages depends on the pressure imposed on the dwindling speakers of these languages. Often there is a strong correlation between endangered languages and the number and quality of recordings and documentations of each. But why do we care about preserving these less prevalent languages? The behavior of cultures is often expressed in the form of speech via one's native language. The memories, ideas, major events, practices, cultures and lessons learnt, both individual as well as the community's, are all communicated to the outside world via language. So, language preservation is crucial to understanding the behavior of these communities. Deep learning models have been shown to dramatically improve speech recognition accuracy but require large amounts of labelled data. Unfortunately, resource constrained languages typically fall short of the necessary data for successful training. To help alleviate the problem, data augmentation techniques fabricate many new samples from each sample. The aim of this master's thesis is to examine the effect of different augmentation techniques on speech recognition of resource constrained languages. The augmentation methods being experimented with are noise augmentation, pitch augmentation, speed augmentation as well as voice transformation augmentation using Generative Adversarial Networks (GANs). This thesis also examines the effectiveness of GANs in voice transformation and its limitations. The information gained from this study will further augment the collection of data, specifically, in understanding the conditions required for the data to be collected in, so that GANs can effectively perform voice transformation. Training of the original data on the Deep Speech model resulted in 95.03% WER. Training the Seneca data on a Deep Speech model that was pretrained on an English dataset, reduced the WER to 70.43%. On adding 15 augmented samples per sample, the WER reduced to 68.33%. Finally, adding 25 augmented samples per sample, the WER reduced to 48.23%. Experiments to find the best augmentation method among noise addition, pitch variation, speed variation augmentation and GAN augmentation revealed that GAN augmentation performed the best, with a WER reduction to 60.03%.
Publisher Site: RIT Repository
PDF (Requires Sign-In): PDF
Click for Bibtex
@book{simha2019improving, title = {Improving Automatic Speech Recognition on Endangered Languages}, author = {Simha, Kruthika Prasanna}, year = {2019}, school = {Rochester Institute of Technology} }
[T-2019-18] Akshat Negi. (2019). “Characterization of Boiling Sound at Conditions Approaching Critical Heat Flux.” Rochester Institute of Technology.
Abstract: In industry, boiling heat transfer is extensively used to efficiently dissipate high heat fluxes from heated substrates. Such industrial applications of boiling include cooling of reactor cores in nuclear power plants, steam generation in industrial boilers, and cooling of high heat flux generating electronic equipment. The maximum heat flux dissipated during boiling is limited by critical heat flux (CHF). At CHF, due to very high bubble generation and coalescence rates, a stable insulating vapor film is formed on the heated surface that leads to very high surface temperatures in a short time. The sudden temperature overshoot causes thermal breakdown, and therefore CHF is disastrous in all industrial applications. Owing to limited visualization of the boiling surfaces and dependence on temperature monitoring only, boiling systems are run at relatively low heat fluxes, ~50% of CHF limit due to safety considerations. The study presented here is focused on developing a method for identifying and analyzing acoustic signatures in the nucleate boiling regimes and using acoustic mapping as a monitoring tool to detect impending CHF. Initially, the sound waves generated through bubble coalescence and bubble collapse during boiling are captured for the plain copper chip. It is observed that the boiling sound is dominant in the frequency range of 400-500 Hz, while additional amplitude peaks in the frequency range of 100-200 Hz are also observed at higher heat fluxes (>100 W/cm²). Further, it is observed that just before CHF, there is a sudden drop in amplitude in the frequency range of 400-500 Hz. A similar study was performed on two additional microporous surfaces and similar acoustic trends as that of the plain copper chip was observed for both the chips. Coupling these observations with high speed visualization, the study indicates that a continuous acoustic mapping during boiling can be used as a tool to predict the impending CHF in boiling systems.
Publisher Site: RIT Repository
PDF (Requires Sign-In): PDF
Click for Bibtex
@book{negi2019characterization, title = {Characterization of Boiling Sound at Conditions Approaching Critical Heat Flux}, author = {Negi, Akshat}, year = {2019}, school = {Rochester Institute of Technology} }
[T-2019-17] Bao Thai. (2019). “Deepfake detection and low-resource language speech recognition using deep learning.” Rochester Institute of Technology.
Abstract: While deep learning algorithms have made significant progress in automatic speech recognition and natural language processing, they require a significant amount of labelled training data to perform effectively. As such, these applications have not been extended to languages that have only limited amount of data available, such as extinct or endangered languages. Another problem caused by the rise of deep learning is that individuals with malicious intents have been able to leverage these algorithms to create fake contents that can pose serious harm to security and public safety. In this work, we explore the solutions to both of these problems. First, we investigate different data augmentation methods and acoustic architecture designs to improve automatic speech recognition performance on low-resource languages. Data augmentation for audio often involves changing the characteristic of the audio without modifying the ground truth. For example, different background noise can be added to an utterance while maintaining the content of the speech. We also explored how different acoustic model paradigms and complexity affect performance on low-resource languages. These methods are evaluated on Seneca, an endangered language spoken by a Native American tribe, and Iban, a low-resource language spoken in Malaysia and Brunei. Secondly, we explore methods to determine speaker identification and audio spoofing detection. A spoofing attack involves using either a text-to-speech voice conversion application to generate audio that mimic the identity of a target speaker. These methods are evaluated on the ASVSpoof 2019 Logical Access dataset containing audio generated using various methods of voice conversion and text-to-speech synthesis.
Publisher Site: RIT Repository
PDF (Requires Sign-In): PDF
Click for Bibtex
@book{thai2019deepfake, title = {Deepfake detection and low-resource language speech recognition using deep learning}, author = {Thai, Bao}, year = {2019}, school = {Rochester Institute of Technology} }
[T-2019-16] Sarthak Arora. (2019). “Perception Methods For Speed And Separation Monitoring Using Time-of-Flight Sensor Arrays.” Rochester Institute of Technology.
Abstract: This work presents the development of a perception pipeline to passively track the partial human ground pose in the context of human robot collaboration. The main motivation behind this work is to provide a speed and separation monitoring based safety controller with an estimate of human position on the factory floor. Three time-of-flight sensing rings affixed to the major links of an industrial manipulator are used to implement the aforementioned. Along with a convolutional neural network based unknown obstacle detection strategy, the ground position of the human operator is estimated and tracked using sparse 3-D point inputs. Experiments to analyze the viability of our approach are presented in depth in the further sections which involve real-world and synthetic datasets. Ultimately, it is shown that the sensing system can provide reliable information intermittently and can be used for higher level perception schemes.
Publisher Site: RIT Repository
PDF (Requires Sign-In): PDF
Click for Bibtex
@book{arora2019perception, title = {Perception Methods For Speed And Separation Monitoring Using Time-of-Flight Sensor Arrays}, author = {Arora, Sarthak}, year = {2019}, school = {Rochester Institute of Technology} }
[T-2019-15] Ethan W. Hughes. (2019). “Spatially-explicit Snap Bean Flowering and Disease Prediction Using Imaging Spectroscopy from Unmanned Aerial Systems.” Rochester Institute of Technology.
Abstract: Sclerotinia sclerotiorum, or white mold, is a fungus that infects the flowers of snap bean plants and causes a subsequent reduction in snap bean pods, which adversely impacts yield. Timing the application of white mold fungicide thus is essential to preventing the disease, and is most effective when applied during the flowering stage. However, most of the flowers are located beneath the canopy, i.e., hidden by foliage, which makes spectral detection of flowering via the leaf/canopy spectra paramount. The overarching objectives of this research therefore are to i) identify spectral signatures for the onset of flowering to optimally time the application of fungicide, ii) investigate spectral characteristics prior to white mold onset in snap beans, and iii) eventually link the location of white mold with biophysical (spectral and structural) metrics to create a spatially-explicit probabilistic risk model for the appearance of white mold in snap bean fields. Spectral angle mapper (SAM) and ratio and thresholding (RT) were used to detect pure vegetation pixels, toward creating the flowering detection models. The pure pixels then were used with a single feature logistic regression (SFLR) to identify wavelengths, spectral ratio indices, and normalized difference indices that best separated the flowering classes. Features with the largest c-index were used to train a support vector machine (SVM) and were then applied to imagery from a different growing season to evaluate model robustness. This research found that single wavelength features in the red (600-700 nm, with a peak at 680 nm) discriminated and predicted flowering up to two weeks before visible flowering occurred, with c-index values above 90%. Structural metrics, such as leaf area index (LAI), have been proven to correlate with white mold incidence, so linear and multivariate regressions were used to ingest spatial- and spectral-related features, derived from the imaging spectroscopy data, and predict ground truth LAI data. These features included raw spectral reflectance values, pixel density, normalized difference index (NDVI), green normalized difference index (GNDVI), and the enhanced vegetation index (EVI). Indicators in the green and red-red edge portion of the spectrum exhibited coefficients of determination (CoD) greater than 0.7. The spatial and spectral indices had CoDs and root mean squared errors (RMSE) ranging from 0.422-0.565 and 0.817-0.942, respectively. The top 28 features were used in a multivariate regression to predict LAI and the results showed a maximum adjusted CoD of 0.849, with an RMSE of 0.390. Future work should include raw reflectance values, LAI correlated spectral features, as well as auxiliary in-field measurements (degree days, average rainfall, average temperature) in the creation of a white mold risk model.
Publisher Site: RIT Repository
PDF (Requires Sign-In): PDF
Click for Bibtex
@book{hughes2019spatially-explicit, title = {Spatially-explicit Snap Bean Flowering and Disease Prediction Using Imaging Spectroscopy from Unmanned Aerial Systems}, author = {Hughes, Ethan W.}, year = {2019}, school = {Rochester Institute of Technology} }
[T-2019-14] Sayantika Bhattacharya. (2019). “Understanding gaps between established Software Engineering Process knowledge and its actual implementation.” Rochester Institute of Technology.
Abstract: A part of Software Engineering (SE) Process Improvement is to identify and bridge the gaps between what we learn about established SE Processes and what we actually execute. Students majoring in SE degrees learn about various established SE processes in class and also try to execute them in their academic projects. In our research, we analyze student SE projects and interview these project teams to identify the gaps between what students learn in class about SE processes, what they think they execute, along with understanding the cause behind these gaps.
Publisher Site: RIT Repository
PDF (Requires Sign-In): PDF
Click for Bibtex
@book{bhattacharya2019understanding, title = {Understanding gaps between established Software Engineering Process knowledge and its actual implementation}, author = {Bhattacharya, Sayantika}, year = {2019}, school = {Rochester Institute of Technology} }
[T-2019-13] Thomas Cenova. (2019). “Exploring HLS Coding Techniques to Achieve Desired Turbo Decoder Architectures.” Rochester Institute of Technology.
Abstract: Software defined radio (SDR) platforms implement many digital signal processing algorithms. These can be accelerated on an FPGA to meet performance requirements. Due to the flexibility of SDR's and continually evolving communications protocols, high level synthesis (HLS) is a promising alternative to standard handcrafted design flows. A crucial component in any SDR is the error correction codes (ECC). Turbo codes are a common ECC that are implemented on an FPGA due to their computational complexity. The goal of this thesis is to explore the HLS coding techniques required to produce a design that targets the desired hardware architecture and can reach handcrafted levels of performance. This work implemented three existing turbo decoder architectures with HLS to produce quality hardware which reaches handcrafted performance. Each targeted design was analyzed to determine its functionality and algorithm so a C implementation could be developed. Then the C code was modified and HLS directives were added to refine the design through the HLS tools. The process of code modification and processing through the HLS tools continued until the desired architecture and performance were reached. Each design was implemented and the bottlenecks were identified and dealt with through appropriate usage of directives and C style. The use of pipelining to bypass bottlenecks added a small overhead from the ramp-up and ramp-down of the pipeline, reducing the performance by at most 1.24%. The impact of the clock constraint set within the HLS tools was also explored. It was found that the clock period and resource usage estimate generated by the HLS tools is not accurate and all evaluations should occur after hardware synthesis.
Publisher Site: RIT Repository
PDF (Requires Sign-In): PDF
Click for Bibtex
@book{cenova2019exploring, title = {Exploring HLS Coding Techniques to Achieve Desired Turbo Decoder Architectures}, author = {Cenova, Thomas}, year = {2019}, school = {Rochester Institute of Technology} }
[T-2019-12] Hrishikesh Barad. (2019). “System-level Eco-driving (SLED): Algorithms for Connected and Autonomous Vehicles.” Rochester Institute of Technology.
Abstract: One of the main reasons for increasing carbon emissions by the transportation sector is the frequent congestion caused in a traffic network. Congestion in transportation occurs when demand for commuting resources exceeds their capacity and with the increasing use of road vehicles, congestion and thereby emissions will continue to rise if proper actions are not taken. Adoption of intelligent transportation systems like autonomous vehicle technology can help in increasing the efficiency of transportation in terms of time, fuel and carbon footprint. This research proposes a System Level Eco-Driving (SLED) algorithm and compares the results, produced by performing microscopic simulations, with conventional driving and the coordination heuristic (COORD) algorithm. The SLED algorithm is designed based on shortcomings and observations of the COORD algorithm to improve the traffic network efficiency. In the SLED strategy, a trailing autonomous vehicle would only request coordination if it is within a set distance from the preceding autonomous vehicle and coordination requests will be evaluated based on their estimated system level emissions impact. Additionally, the human-driven vehicles will not be allowed to change lanes. Average CO2 emissions per vehicle for SLED showed improvements ranging from 0% to 5% compared to COORD. Additionally, the threshold limit to surpass the conventional driving behavior CO2 emissions at 900 vehicles per hour density reduced to 30% using SLED as compared to 40% using the COORD algorithm. Average wait time per vehicle for the SLED algorithm at 1200 vehicles per hour density increased by one to six seconds as compared to the COORD strategy although reduced up to thirty seconds of wait time compared to the conventional driving behavior. This finding can be helpful for policy makers to switch the algorithms based on the requirement i.e. opt for the SLED algorithm if reducing emissions has a higher priority compared to wait and travel time while opt for the COORD algorithm if reducing wait and travel time has a higher priority compared to emissions.
Publisher Site: RIT Repository
PDF (Requires Sign-In): PDF
Click for Bibtex
@book{barad2019system-level, title = {System-level Eco-driving (SLED): Algorithms for Connected and Autonomous Vehicles}, author = {Barad, Hrishikesh}, year = {2019}, school = {Rochester Institute of Technology} }
[T-2019-11] Stephanie Soldavini. (2019). “Using Reduced Graphs for Efficient HLS Scheduling.” Rochester Institute of Technology.
Abstract: High-Level Synthesis (HLS) is the process of inferring a digital circuit from a high-level algorithmic description provided as a software implementation, usually in C/C++. HLS tools will parse the input code and then perform three main steps: allocation, scheduling, and binding. This results in a hardware architecture which can then be represented as a Register-Transfer Level (RTL) model using a Hardware Description Language (HDL), such as VHDL or Verilog. Allocation determines the amount of resources needed, scheduling finds the order in which operations should occur, and binding maps operations onto the allocated hardware resources. Two main challenges of scheduling are in its computational complexity and memory requirements. Finding an optimal schedule is an NP-hard problem, so many tools use elaborate heuristics to find a solution which satisfies prescribed implementation constraints. These heuristics require the Control/Data Flow Graph (CDFG), a representation of all operations and their dependencies, which must be stored in its entirety and therefore use large amounts of memory. This thesis presents a new scheduling approach for use in the HLS tool chain. The new technique schedules operations using an algorithm which operates on a reduced representation of the graph, which does not need to retain individual dependency information in order to generate a schedule. By using the simplified graph, the complexity of scheduling is significantly reduced, resulting in improved memory usage and lower computational effort. This new scheduler is implemented and compared to the existing scheduler in the open source version of the LegUp HLS tool. The results demonstrate that an average of 16 times speedup on the time required to determine the schedule can be achieved, with just a fraction of the memory usage (1/5 on average). All of this is achieved with 0 to 6% of added cost on the final hardware execution time.
Publisher Site: RIT Repository
PDF (Requires Sign-In): PDF
Click for Bibtex
@book{soldavini2019using, title = {Using Reduced Graphs for Efficient HLS Scheduling}, author = {Soldavini, Stephanie}, year = {2019}, school = {Rochester Institute of Technology} }
[T-2019-10] Nilesh Pandey. (2019). “Poly-GAN: A Multi-Conditioned GAN for Multiple Tasks.” Rochester Institute of Technology.
Abstract: We present Poly-GAN, a novel conditional GAN architecture that is motivated by different Image generation and manipulation applications like Fashion Synthesis, an application where garments are automatically placed on images of human models at an arbitrary pose, image inpainting, an application where we try to recover a damaged image using the edges or a rough sketch of the image. While different applications use different GAN setup for image generation, we propose only one architecture for multiple applications with little to no change in the pipeline. Poly-GAN allows conditioning on multiple inputs and is suitable for many different tasks. Our novel architecture enforces the conditions at all layers of the encoder and utilizes skip connections from the coarse layers of the encoder to the respective layers of the decoder. Coarse layers are easier to manipulate in shape change using condition, which results in higher level change in the result. Our system achieves state-of-the-art quantitative results on Fashion Synthesis based on the Structural Similarity Index metric and Inception Score metric using the DeepFashion dataset. For the image inpainting task we are achieving competitive results compared to current state of the art methods.
Publisher Site: RIT Repository
PDF (Requires Sign-In): PDF
Click for Bibtex
@book{pandey2019poly-gan:, title = {Poly-GAN: A Multi-Conditioned GAN for Multiple Tasks}, author = {Pandey, Nilesh}, year = {2019}, school = {Rochester Institute of Technology} }
[T-2019-09] Bryan Matthew Blakeslee. (2019). “LambdaNet: A Novel Architecture for Unstructured Change Detection.” Rochester Institute of Technology.
Abstract: The goal of this thesis is the development of LambdaNet, a new type of network architecture for the performance of unstructured change detection. LambdaNet combines concepts from Siamese and semantic segmentation architectures, and is capable of identifying and localizing the significant differences between image pairs while simultaneously disregarding background noise. Changes are marked at the pixel level, by interpreting change detection as a binary (change/no change) classification problem. Development of this architecture began with an evaluation of several candidate models, inspired by other successful network architectures and layers, including VGG, ResNet, and the Res2Net layer. Once the best performing LambdaNet architecture was determined, it was extended to incorporate a multi-class version of change detection. Referred to as directional change, this technique allows segmentation-based output of change information in four different classes: No change, additive change, subtractive change, and exchange. Lastly, change detection is not the only unstructured operation of interest. One of the most successful unstructured techniques is that of artistic style transfer. This method allows information from a style image to be merged into a supplied content image. In order to implement this technique, a new variant of LambdaNet was developed, called LambdaStyler. This network is capable of learning multiple artistic styles, which can then be selected for application to the desired content image.
Publisher Site: RIT Repository
PDF (Requires Sign-In): PDF
Click for Bibtex
@book{blakeslee2019lambdanet:, title = {LambdaNet: A Novel Architecture for Unstructured Change Detection}, author = {Blakeslee, Bryan Matthew}, year = {2019}, school = {Rochester Institute of Technology} }
[T-2019-08] Jacob Hamilton. (2019). “Additive Manufacturing Materials: Fabrication of Aluminum Matrix Composites.” Rochester Institute of Technology.
Abstract: This study aims to validate the ability of cryomilling for the production of high-quality aluminum matrix composite (AMC) powder for powder bed fusion (PBF) additive manufacturing (AM). The spectrum of aluminum-based materials available for AM remains limited due to complex melting and solidification dynamics inherent to the process. To overcome these problems, fillers are often added to aluminum matrices to create a class of materials called AMCs that combine the ductility of aluminum with the stiffness of ceramic reinforcements. However, producing particulate composite feedstock powder for PBF that promotes full densification and microstructural homogeneity is nontrivial. Traditional liquid-phase processing through atomization is not suited to produce composite powders as particle segregation discourages composite homogeneity. AM powder production through solid-state mechanical alloying has been studied with limited success, primarily due to poor powder spreadability and inclusion of lubricants in the alloying process. Cryogenic mechanical alloying, termed cryomilling, enhances homogeneity between matrix and reinforcement particles by recurrent fracture and cold welding sans lubricants but remains unexplored for the fabrication of PBF feedstock powder. Herein, a method for producing homogeneous, flowable AMC powder designed for PBF is described in detail. Various compositions, powder masses, and milling times were explored to tune particle morphology, composition, and composite homogeneity. A representative spreading test of cryomilled materials qualitatively indicated that distinct cryomilling parameters may produce powder with comparable spreading characteristics to gas atomized AlSi10Mg, a common PBF feedstock material. Cryomilled AMCs displayed superior Vickers microhardness to unmilled AlSi10Mg powder after compression and sintering. This research provides an indication of cryomilling capabilities to become an effective production method of custom alloy powder for PBF-AM.
Publisher Site: RIT Repository
PDF (Requires Sign-In): PDF
Click for Bibtex
@book{hamilton2019additive, title = {Additive Manufacturing Materials: Fabrication of Aluminum Matrix Composites}, author = {Hamilton, Jacob}, year = {2019}, school = {Rochester Institute of Technology} }
[T-2019-07] Ge Song. (2019). “Low-Rank Multivariate General Linear Model with Relationship between Brain Regions and Time Points.” Rochester Institute of Technology.
Abstract: The human brain is hard to study and analysis, not because of the complexity of the brain structure, such as neurons and neurons connections, but also because of the complexity of the brain activities. Since in different scales, for example, different time series, different physical senses, the measurements of the human brain activities can be varied. Tring to measure the brain regions relationships in person, the Functional Magnetic Resonance Imaging (fMRI) is one of the methods. The focus of this paper is on analyzing human brain regions relationships in different time domains and different scans of fMRI by using low-rank multivariate general linear model (LRMGLM). The function of the model is to penalize optimization and characterize variation across different regions and stimulus in hemodynamic response functions (HRFs). After analyzing the fMRI data with LRMGLM model, we also analyzed data by methods of Cross Validation and Principal Components Analysis (PCA).
Publisher Site: RIT Repository
PDF (Requires Sign-In): PDF
Click for Bibtex
@book{song2019low-rank, title = {Low-Rank Multivariate General Linear Model with Relationship between Brain Regions and Time Points}, author = {Song, Ge}, year = {2019}, school = {Rochester Institute of Technology} }
[T-2019-06] Jared A. Loewenthal. (2019). “A 3U Cubesat Platform for Plant Growth Experiments.” Rochester Institute of Technology.
Abstract: This thesis work presents the design, manufacturing, and ground testing of a 3U Cubesat platform intended for plant growth experiments. The structure is comprised of four identical, but independent plant growth chambers. Each of these accommodates about two cubic inches of soil, and the necessary air volume and moisture regulation to grow a fast-growing plant from seed to seed in 3-4 weeks. The plant growth is artificially stimulated by an array of light emitting diodes (LEDs) at grow light wavelengths that match the properties of chlorophyll, and is monitored by a suite of sensors: temperature, pressure, relative humidity, CO2, custom designed soil pH, soil moisture, and imaging. The latter takes periodic still pictures in the visible and infrared spectrum using LED based illumination at different wavelengths. These images are used to analyze the overall health of the plant and record the developmental stages of the plant growth. The platform is complemented with a raspberry Pi on board computer and a solar panel-based power generation system. The current scientific goal of this 3U Cubesat platform is to study the interactions of soil microbes (bacteria and fungus) and plants. The former can be a source of nutrients for plants and decrease induced stress on these in space conditions. The availability of four test chambers allow scientists to quantify changes and investigate emergent properties of the soil bacterial and fungal populations. The Cubesat design affords the opportunity to investigate the impact of physical factors such as pressure, temperature, microgravity, and space radiation on the soil bacteria and fungi, in addition to the overall plant health. While small scale biology experiments have been performed on Cubesats before, to our knowledge none of those involved plant growth stimulation and monitoring. This platform can be adapted and expanded to meet the requirements of similar scientific research.
Publisher Site: RIT Repository
PDF (Requires Sign-In): PDF
Click for Bibtex
@book{loewenthal2019a, title = {A 3U Cubesat Platform for Plant Growth Experiments}, author = {Loewenthal, Jared A.}, year = {2019}, school = {Rochester Institute of Technology} }
[T-2019-05] Manoj Meda. (2019). “Effect of process conditions on feature size of inkjet printed silver MOD ink.” Rochester Institute of Technology.
Abstract: Advancements in functional printing processes have demonstrated the viability of fabricating electronic circuits through deposition of electrically conductive materials. This has opened various novel avenues such as flexible and stretchable electronics that are currently beyond the capabilities of conventional subtractive manufacturing processes. Among the functional printing processes, piezoelectric drop-on-demand inkjet printing has now become of increasing interest due to its versatility. In case of the electrically conductive materials, Metal Organic Decomposition (MOD) inks stand out due to their particle free nature, which improves the jetting behavior. The strict demand from the electronics industry necessities uniform, smooth features of high resolution for printed electronic devices. Numerous studies have discussed the impact of print process conditions on the quality of printed features. However, the process conditions of pre-process and post-process techniques implemented in printed electronics can also have a considerable effect on the feature quality. This research is aimed at investigating the impact of not only some of the key print process parameters but also the impact of key pre-process and post-process parameters on the track width of features printed using a silver MOD ink on polyethylene terephthalate (PET) substrate. A statistical approach was employed for the experiments in identifying the significant control variables effecting the resolution of printed features. Using the results from the experimental study, the process parameters suitable for obtaining desired feature dimensions was derived by identifying the significant main and interaction effects. The results of this study can be used in improving the homogeneity of inkjet printed MOD features in fabricating devices such as flexible electronic displays, thin-film transistors, smart packaging labels etc.
Publisher Site: RIT Repository
PDF (Requires Sign-In): PDF
Click for Bibtex
@book{meda2019effect, title = {Effect of process conditions on feature size of inkjet printed silver MOD ink}, author = {Meda, Manoj}, year = {2019}, school = {Rochester Institute of Technology} }
[T-2019-04] Mandy C. Nevins. (2019). “Point Spread Function Determination in the Scanning Electron Microscope and its Application in Restoring Images Acquired at Low Voltage.” Rochester Institute of Technology.
Abstract: Electron microscopes have the capability to examine specimens at much finer detail than a traditional light microscope. Higher electron beam voltages correspond to higher resolution, but some specimens are sensitive to beam damage and charging at high voltages. In the scanning electron microscope (SEM), low voltage imaging is beneficial for viewing biological, electronic, and other beam-sensitive specimens. However, image quality suffers at low voltage from reduced resolution, lower signal-to-noise, and increased visibility of beam-induced contamination. Most solutions for improving low voltage SEM imaging require specialty hardware, which can be costly or system-specific. Point spread function (PSF) deconvolution for image restoration could provide a software solution that is cost-effective and microscope-independent with the ability to produce image quality improvements comparable to specialty hardware systems. Measuring the PSF (i.e., electron probe) of the SEM has been a notoriously difficult task until now. The goals of this work are to characterize the capabilities and limitations of a novel SEM PSF determination method that uses nanoparticle dispersions to obtain a two-dimensional measurement of the PSF, and to evaluate the utility of the measured PSF for restoration of low voltage SEM images. The presented results are meant to inform prospective and existing users of this technique about its fundamental theory, best operating practices, the expected behavior of output PSFs and image restorations, and factors to be aware of during interpretation of results.
Publisher Site: RIT Repository
PDF (Requires Sign-In): PDF
Click for Bibtex
@book{nevins2019point, title = {Point Spread Function Determination in the Scanning Electron Microscope and its Application in Restoring Images Acquired at Low Voltage}, author = {Nevins, Mandy C.}, year = {2019}, school = {Rochester Institute of Technology} }
[T-2019-03] Anton Travinsky. (2019). “Evaluating the performance of digital micromirror devices for use as programmable slit masks in multi-object spectrometers.” Rochester Institute of Technology.
Abstract: Multi-object spectrometers are extremely useful astronomical instruments that allow simultaneous spectral observations of large numbers of objects. Studies performed with ground-based multi-object spectrometers (MOSs) in the last four decades helped to place unique constraints on cosmology, large scale structure, galaxy evolution, Galactic structure, and contributed to countless other scientific advances. However, terrestrial MOSs use large discrete components for object selection, which, aside from not transferable to space-based applications, are limited in both minimal slit width and minimal time required accommodate a change of the locations of objects of interest in the field of view. There is a pressing need in remotely addressable and fast-re-configurable slit masks, which would allow for a new class of instruments - spacebased MOS. There are Microelectromechanical System (MEMS) - based technologies under development for use in space-based instrumentation, but currently they are still unreliable, even on the ground. A digital micromirror device (DMD) is a highly capable, extremely reliable, and remotely re-configurable spatial light modulator (SLM) that was originally developed by Texas Instruments Incorporated for projection systems. It is a viable and very promising candidate to serve as slit mask for both terrestrial and space-based MOSs. This work focused on assessing the suitability of DMDs for use as slit masks in space-based astronomical MOSs and developing the necessary calibration procedures and algorithms. Radiation testing to the levels of orbit around the second Lagrangian point (L2) was performed using the accelerated heavy-ion irradiation approach. The DMDs were found to be extremely reliable in such radiation environment, the devices did not experience hard failures and there was no permanent damage. Expected single-event upset (SEU) rate was determined to be about 5.6 micro-mirrors per 24 hours on-orbit for 1-megapixel device. Results of vibration and mechanical shock testing performed according to the National Aeronautics and Space Administration (NASA) General Environmental Verification Standard (GEVS) at NASA Goddard Space Flight Center (GSFC) suggest that commercially available DMDs are mechanically suitable for space-deployment with a very significant safety margin. Series of tests to assess the performance and the behaviour of DMDs in cryogenic temperatures (down to 78 K) were also carried out. There were no failures or malfunctions detected in commercially-available devices. An earlier prototype of a terrestrial DMD-based MOS (Rochester Institute of Technology Multi-Object Spectrometer (RITMOS)) was updated with a newer DMD model, and the performance of the instrument was evaluated. All the experiments performed strongly suggest that DMDs are highly reliable and capable devices that are extremely suitable for use as remotely programmable slit masks in MOS.
Publisher Site: RIT Repository
PDF (Requires Sign-In): PDF
Click for Bibtex
@book{travinsky2019evaluating, title = {Evaluating the performance of digital micromirror devices for use as programmable slit masks in multi-object spectrometers}, author = {Travinsky, Anton}, year = {2019}, school = {Rochester Institute of Technology} }
[T-2019-02] Caitlin Rose. (2019). “Identifying Galaxy Mergers with Quantitative Morphological Parameters in Simulated James Webb Space Telescope Images.” Rochester Institute of Technology.
Abstract: Mergers play an important role in the formation and evolution of galaxies by triggering starbursts, AGN activity, and morphological transitions from disks to ellipticals. They can also cause morphological disturbances in a galaxy's appearance, such as double nuclei, tidal tails, and other asymmetries, which can appear before or after a merger has occurred. Therefore, one way to identify low redshift galaxy mergers is to search for these morphological signatures via quantitative morphological parameters, which quantify a galaxy's light distribution (such as Sérsic profiles, or the CAS system, G and M20, and the MID statistics). However, for high redshift galaxies, these parameters can be affected by biases due to poor resolution and noisy images. The upcoming James Webb Space Telescope (JWST) will be able to probe higher redshifts than ever before for morphological studies with high spatial resolution. The Cosmic Evolution Early Release Science (CEERS) Survey will use JWST's near-infrared camera to reveal detailed galaxy morphologies over a wide range of redshifts. In preparation for CEERS images, this works seeks to understand how well those common morphological statistics will be able to identify JWST mergers. Multiwavelength Sérsic profile fitting program Galapagos-2 and the nonparametric morphology program statmorph were run on simulated JWST images from Illustris, which were modified to match the specifications of CEERS imaging. Using Illustris merger history catalogs, plots of different combinations of the rest-frame morphologies of the simulated galaxies, binned by redshift, were made as functions of merger timescales. These plots do not separate mergers from non-mergers as cleanly as previous studies have found, regardless of redshift or merger timescale. This indicates that a more sophisticated analysis method, such as principal component analysis, will be required in order to effectively isolate JWST mergers from other galaxies.
Publisher Site: RIT Repository
PDF (Requires Sign-In): PDF
Click for Bibtex
@book{rose2019identifying, title = {Identifying Galaxy Mergers with Quantitative Morphological Parameters in Simulated James Webb Space Telescope Images}, author = {Rose, Caitlin}, year = {2019}, school = {Rochester Institute of Technology} }
[T-2019-01] Jacob Wirth. (2019). “Point Spread Function and Modulation Transfer Function Engineering.” Rochester Institute of Technology.
Abstract: A novel computational imaging approach to sensor protection based on point spread function (PSF) engineering is designed to suppress harmful laser irradiance without significant loss of image fidelity of a background scene. PSF engineering is accomplished by modifying a traditional imaging system with a lossless linear phase mask at the pupil which diffracts laser light over a large area of the imaging sensor. The approach provides the additional advantage of an instantaneous response time across a broad region of the electromagnetic spectrum. As the mask does not discriminate between the laser and desired scene, a post-processing image reconstruction step is required, which may be accomplished in real time, that both removes the laser spot and improves the image fidelity. This thesis includes significant experimental and numerical advancements in the determination and demonstration of optimized phase masks. Analytic studies of PSF engineering systems and their fundamental limits were conducted. An experimental test-bed was designed using a spatial light modulator to create digitally-controlled phase masks to image a target in the presence of a laser source. Experimental results using already known phase masks: axicon, vortex and cubic are reported. New methods for designing phase masks are also reported including (1) a numeric differential evolution algorithm, (2) a "PSF reverse engineering" method, and (3) a hardware based simulated annealing experiment. Broadband performance of optimized phase masks were also evaluated in simulation. Optimized phase masks were shown to provide three orders of magnitude laser suppression while simultaneously providing high fidelity imaging a background scene.
Publisher Site: RIT Repository
PDF (Requires Sign-In): PDF
Click for Bibtex
@book{wirth2019point, title = {Point Spread Function and Modulation Transfer Function Engineering}, author = {Wirth, Jacob}, year = {2019}, school = {Rochester Institute of Technology} }
[T-2018-37] Thomas G. Guerin. (2018). “Adjustment of Parametric Dynamic Scheduling Heuristics for Heterogeneous Systems to Account for Heterogeneity.” Rochester Institute of Technology.
Abstract: Modern computing applications are becoming increasingly data-hungry and computationally expensive. This trend continues even as hardware performance constraints loom with the impending death of Moore's law. Hence, systems have become increasingly heterogeneous in the pursuits of improving performance and reducing power consumption. Such a heterogeneous system relies on a variety of different specialized processors with differing architectures, rather than processing units of a single type. Given their architectural differences, any given computation will not perform equally on all processors. As such, efficient scheduling of computations to processors is an essential design consideration. In this thesis work, a simulation of an existing dynamic scheduling heuristic - Alternative Processor within Threshold (APT) - was used to model the execution of a variety of heterogeneous workloads and heterogeneous systems. An extended version of this scheduler (APTX) was analyzed in a similar way, as was a simplified version of the existing K-Percent Best (KPB) scheduler. Each of these schedulers has a numeric "parameter" constraining its behavior. In existing analyses, these scheduling heuristics were tested only with a small set of arbitrary values for these parameters. The goal of this research was to use a stochastic method to optimize said parameters for the minimum finishing time of any given set of computations on any given heterogeneous system. An analytical expression to estimate the ideal parameter of each scheduler was developed. Each was based on the statistical analysis of the results of a set of randomly-generated simulations. After these expressions were developed, these optimized APT, APTX, and KPB schedulers were evaluated against three other dynamic schedulers - Minimum Execution Time (MET), Serial Scheduling (SS), and Shortest Process Next (SPN) - by running the randomly-generated simulations on all six. For the most common type of heterogeneous system, APT and APTX were found to have the earliest finish time on average, while MET and KPB generally performed poorly. Ultimately, this research not only demonstrated the advantages of APT and APTX over other dynamic schedulers in a fair comparison, but it also demonstrated a method by which any parametric scheduler can be tuned.
Publisher Site: RIT Repository
PDF (Requires Sign-In): PDF
Click for Bibtex
@book{guerin2018adjustment, title = {Adjustment of Parametric Dynamic Scheduling Heuristics for Heterogeneous Systems to Account for Heterogeneity}, author = {Guerin, Thomas G.}, year = {2018}, school = {Rochester Institute of Technology} }
[T-2018-36] Anthony Shehan Ayam Peruma. (2018). “What the Smell? An Empirical Investigation on the Distribution and Severity of Test Smells in Open Source Android Applications.” Rochester Institute of Technology.
Abstract: The widespread adoption of mobile devices, coupled with the ease of developing mobile-based applications (apps) has created a lucrative and competitive environment for app developers. Solely focusing on app functionality and time-to-market is not enough for developers to ensure the success of their app. Quality attributes exhibited by the app must also be a key focus point; not just at the onset of app development, but throughout its lifetime. The impact analysis of bad programming practices, or code smells, in production code has been the focus of numerous studies in software maintenance. Similar to production code, unit tests are also susceptible to bad programming practices which can have a negative impact not only on the quality of the software system but also on maintenance activities. With the present corpus of studies on test smells primarily on traditional applications, there is a need to fill the void in understanding the deviation of testing guidelines in the mobile environment. Furthermore, there is a need to understand the degree to which test smells are prevalent in mobile apps and the impact of such smells on app maintenance. Hence, the purpose of this research is to: (1) extend the existing set of bad test-code practices by introducing new test smells, (2) provide the software engineering community with an open-source test smell detection tool, and (3) perform a large-scale empirical study on test smell occurrence, distribution, and impact on the maintenance of open-source Android apps. Through multiple experiments, our findings indicate that most Android apps lack an automated verification of their testing mechanisms. As for the apps with existing test suites, they exhibit test smells early on in their lifetime with varying degrees of co-occurrences with different smell types. Our exploration of the relationship between test smells and technical debt proves that test smells are a strong measurement of technical debt. Furthermore, we observed positive correlations between specific smell types and highly changed/buggy test files. Hence, this research demonstrates that test smells can be used as indicators for necessary preventive software maintenance for test suites.
Publisher Site: RIT Repository
PDF (Requires Sign-In): PDF
Click for Bibtex
@book{peruma2018what, title = {What the Smell? An Empirical Investigation on the Distribution and Severity of Test Smells in Open Source Android Applications}, author = {Peruma, Anthony Shehan Ayam}, year = {2018}, school = {Rochester Institute of Technology} }
[T-2018-35] Akram Marseet. (2018). “Application of Convolutional Neural Network Framework on Generalized Spatial Modulation for Next Generation Wireless Networks.” Rochester Institute of Technology.
Abstract: A novel custom auto-encoder Complex Valued Convolutional Neural Network (AE-CVCNN) model is proposed and implemented using MATLAB for multiple-input-multiple output (MIMO) wireless networks. The proposed model is applied on two dierent generalized spatial modulation (GSM) schemes: the single symbol generalized spatial modulation SS - GSM and the multiple symbol generalized spatial modulation (MS-GSM). GSM schemes are used with Massive-MIMO to increase both the spectrum eciency and the energy eciency. On the other hand, GSM schemes are subjected to high computational complexity at the receiver to detect the transmitted information. High computational complexity slows down the throughput and increases the power consumption at the user terminals. Consequently, reducing both the total spectrum eciency and energy eciency. The proposed CNN framework achieves constant complexity reduction of 22.73% for SSGSM schemes compared to the complexity of its traditional maximum likelihood detector (ML). Also, it gives a complexity reduction of 14.7% for the MS-GSM schemes compared to the complexity of its detector. The performance penalty of the two schemes is at most 0.5 dB. Besides to the proposed custom AE CV-CNN model, a dierent ML detector0s formula for "SS -GSM" schemes is proposed that achieves the same performance as the traditional ML detector with a complexity reduction of at least 40% compared to that of the traditional ML detector. In addition, the proposed AE-CV-CNN model is applied to the proposed ML detector,and it gives a complexity reduction of at least 63.6% with a performance penalty of less than 0.5 dB. An interesting result about applying the proposed custom CNN model on the proposed ML detector is that the complexity is reduced as the spatial constellation size is increased which means that the total spectrum eciency is increased by increasing the spatial constellation size without increasing the computational complexity.
Publisher Site: RIT Repository
PDF (Requires Sign-In): PDF
Click for Bibtex
@book{marseet2018application, title = {Application of Convolutional Neural Network Framework on Generalized Spatial Modulation for Next Generation Wireless Networks}, author = {Marseet, Akram}, year = {2018}, school = {Rochester Institute of Technology} }
[T-2018-34] Lydia M. Hays. (2018). “Design Considerations for Training Memristor Crossbars Used in Spiking Neural Networks.” Rochester Institute of Technology.
Abstract: CMOS/Memristor integrated architectures have shown to be powerful for realizing energy-efficient learning machines. These architectures are recently demonstrated in reservoir computing networks, which have reduced training complexity and resource utilization. In reservoir computing, the training time is curtailed due to random weight initialization in the hidden layer, which will remain constant during training. The CMOS/memristor variability can be exploited to generate these random weights and reduce the area overhead. Recent studies have shown that the CMOS/memristor crossbars are ideal for on-device learning machines, including reservoir computing networks. An exemplary CMOS/memristor crossbar based on-device accelerator, Ziksa, was demonstrated on several of these learning networks. While the crossbars are generally area and energy efficient, the peripheral circuitry to control the read/write logic to the crossbars is extremely power hungry. This work focuses on improving the Ziksa accelerator peripheral circuitry for a spiking reservoir network. The optimized training circuitry for Ziksa includes transmission gates, a control unit, and a current amplifier and is demonstrated within a layer of spiking neurons for training and neuron behavior. All the analog circuits are validated using the Cadence 45 nm GPDK on a 2x4 and 1x4 crossbar. For a 32x32 crossbar, the area and power of the peripheral circuitry is ∼2,800 µm^2 and ∼3.685 mW respectively, demonstrating the overall efficacy of the proposed circuits.
Publisher Site: RIT Repository
PDF (Requires Sign-In): PDF
Click for Bibtex
@book{hays2018design, title = {Design Considerations for Training Memristor Crossbars Used in Spiking Neural Networks}, author = {Hays, Lydia M.}, year = {2018}, school = {Rochester Institute of Technology} }
[T-2018-33] Najat A. Alharbi. (2018). “Alkali Activated Slag Characterization by Scanning Electron Microscopy and X-ray Microanalysis.” Rochester Institute of Technology.
Abstract: Blast furnace slag is a non-metallic byproduct generated by the production of iron and steel in a blast furnace at temperatures in the range of 1400°-1600° C. The alkali activation of blast furnace slag has the potential to reduce the environmental impact of cementitious materials and to be applied in geographic zones where weather is a factor that negatively affects performance of materials based on Ordinary Portland Cement. Alkali-activated blast furnace slag cements have been studied since the 1930s due to its high compressive strength; they can exceed 100 MPa in 28 days. The low Ca/Si ratio in slag improves its resistance to aggressive chemical materials such as acids, chlorides and sulphates. Blast furnace slag is a highly heterogeneous material. It is well known that its chemical composition affects the physical properties of the alkali activated material, however there is little work on how these inhomogeneities affect the microstructure and pore formation. In this study we characterize slag cement activated with KOH using several methods: x-ray diffraction (XRD), transmission electron microscopy (TEM), scanning electron microscopy (SEM), x-ray microanalysis (EDS), and quantitative element mapping. Attention is focused on delineating the phases induced by the alkali activation, as these phases are important in determining the mechanical properties of the material. For the alkaline activated slag, we found four phases. One phase was the particles carried over from the unactivated slag, but with significant changes in the chemical composition. In addition, three other phases were found -- one is rich in hydrotalcite and two phases were calcium aluminum silicate hydrate (C-A-S-H) is predominant.
Publisher Site: RIT Repository
PDF (Requires Sign-In): PDF
Click for Bibtex
@book{alharbi2018alkali, title = {Alkali Activated Slag Characterization by Scanning Electron Microscopy and X-ray Microanalysis}, author = {Alharbi, Najat A.}, year = {2018}, school = {Rochester Institute of Technology} }
[T-2018-32] Alexander Leon Krall. (2018). “Comparing Cyber Defense Alternatives Using Rare-Event Simulation Techniques to Compute Network Risk.” Rochester Institute of Technology.
Abstract: Vulnerabilities inherent in a cyber network can be exploited by individuals with malicious intent. Thus, machines on the network are at risk. Formally, security specialists seek to mitigate the risk of intrusion events through network reconfiguration and defense. Comparison between configuration alternatives may be difficult if an event is sufficiently rare; risk estimates may of be questionable quality making definitive inferences unattainable. Furthermore, that which constitutes a “rare” event can imply different rates of occurrence, depending on network complexity. To measure rare events efficiently without the risk of doing damage to a cyber network, special rare-event simulation techniques can be employed, such as splitting or importance sampling. In particular, importance sampling has shown promise when modeling an attacker moving through a network with intent to steal data. The importance sampling technique amplifies certain aspects of the network in order to cause a rare event to happen more frequently. Output statistics collected under these amplified conditions must then be scaled back to the context of the original network to produce meaningful results. This thesis successfully tailors the importance sampling methodology to scenarios where an attacker must search a network. Said tailoring takes the attacker’s successes and failures as well as the attacker’s targeting choices into account. The methodology is shown to be more computationally efficient and can produce higher quality estimates of risk when compared to standard simulation.
Publisher Site: RIT Repository
PDF (Requires Sign-In): PDF
Click for Bibtex
@book{krall2018comparing, title = {Comparing Cyber Defense Alternatives Using Rare-Event Simulation Techniques to Compute Network Risk}, author = {Krall, Alexander Leon}, year = {2018}, school = {Rochester Institute of Technology} }
[T-2018-31] Tegan Ayers. (2018). “Self-Regulation within the Wearable Device Industry and The Alignment to Device Users’ Perceptions of Health Data Privacy.” Rochester Institute of Technology.
Abstract: Health data privacy has become increasingly pertinent as the Internet-of-Things (IoT), specifically, health-monitoring, wearable devices, has become more advanced. Today’s regulatory framework allows wearable device companies to self-regulate how data is collected and used, thus leaving consumer, health data at risk of possible mishandling or abuse. Consequently, this research sought to examine whether data privacy practices adopted by major wearable manufacturers align with consumer expectations about these devices and the data they collect. Both consumers’ understanding of health data privacy and the corresponding tech companies’ stance on protecting consumer privacy were evaluated by performing crowd-sourced surveys and a thematic analyses of current privacy policies. Results of the survey suggest that most consumers are unaware of the possible risks associated with collecting health data; and, this lack of informativeness has led to what appear to be a lack of concern for their health data. However, many consumers still express an interest in protecting their privacy, regardless if they fully comprehend the risks, and most participants (79.4%) believed there should be additional regulations placed on the wearable industry. As such, it is recommended that a widely-known, non-government body, such as IEEE, develop a three-tier data privacy certification that wearable companies may apply for, but not be forced to adhere to. In principle, the market demand for increased data privacy controls would drive companies to classify each of their products as bronze, silver or gold-certified, which corresponds to increasingly stringent data privacy and security regulation.
Publisher Site: RIT Repository
PDF (Requires Sign-In): PDF
Click for Bibtex
@book{ayers2018self-regulation, title = {Self-Regulation within the Wearable Device Industry and The Alignment to Device Users’ Perceptions of Health Data Privacy}, author = {Ayers, Tegan}, year = {2018}, school = {Rochester Institute of Technology} }
[T-2018-30] Austin Hayes. (2018). “Additive Manufactured Microstructures and Designs for High Heat Flux Dissipation During Pool Boiling.” Rochester Institute of Technology.
Abstract: Heat dissipation is vital in industries requiring predictable operating temperatures while also producing large heat fluxes. These industries include electronics and power generation. For electronics, as more devices fit on a smaller area, the heat flux increases dramatically. Pool boiling offers a solution to electronic cooling due to extremely high heat transfer with a low temperature change. Previous research has focused on coatings and precision manufacturing to create microchannels and features for boiling augmentation. However, this is limited to designs for subtractive processes. The use of additive manufacturing (AM) offers a novel way of thinking of design for boiling enhancement. 3-D boiling structures are fabricated out of aluminum using the Vader System's magnetojet printer. Three generations of geometric structures are created: a volcano-with-holes, a miniaturized volcano-with-holes, and a modular volcano-with-holes. These designs are not easily manufactured using standard techniques. As such, three-dimensional bubble dynamics are currently being explored using high speed imaging and particle image velocimetry. By printing a volcano shape with base holes, the liquid and vapor phases are physically separated in a process termed macroscale liquid-vapor pathways. The singular volcano-with-holes chips achieved a maximum heat flux of 217.3 W/cm2 with a maximum heat transfer coefficient (HTC) of 97.2 kW/m2K (81% improvement over plain). By producing four volcanoes on a single chip, the liquid flow length inside the volcano, which acts as the entrance length, is reduced by 50% and the HTC greatly increased. The highest performing miniaturized volcano-with-holes chip reached a maximum heat flux of 223.1W/cm2 with a maximum HTC of 139.1 kW/m2K (150% improvement over plain). Additionally, the highest performing miniaturized chip was printed on top of a microchannel array. This resulted in combined enhancement from both microchannel and bubble dynamics resulting in a maximum heat flux of 228.4 W/cm2 with a HTC of 339.6 kW/m2K (533% improvement over plain). Finally, a modular structure was created to determine the individual influence of conduction and bubble dynamic augmentation on boiling enhancement. The modular designs show an 83% improvement in CHF (202.4 W/cm2) over plain copper chips and a 83% improvement in HTC(139.0 kW/m2K). This indicates boiling enhancement arises from three-dimensional control over bubble dynamics, resulting in macroscale separate liquid-vapor pathways.
Publisher Site: RIT Repository
PDF (Requires Sign-In): PDF
Click for Bibtex
@book{hayes2018additive, title = {Additive Manufactured Microstructures and Designs for High Heat Flux Dissipation During Pool Boiling}, author = {Hayes, Austin}, year = {2018}, school = {Rochester Institute of Technology} }
[T-2018-29] Luke G. Boudreau. (2018). “Contractive Autoencoding for Hierarchical Temporal Memory and Sparse Distributed Representation Binding.” Rochester Institute of Technology.
Abstract: Hierarchical Temporal Memory is a brain inspired memory prediction framework modeled after the uniform structure and connectivity of pyramidal neurons found in the human neocortex. Similar to the neocortex, Hierarchical Temporal Memory processes spatiotemporal information for anomaly detection and prediction. A critical component in the Hierarchical Temporal Memory algorithm is the Spatial Pooler, which is responsible for processing feedforward data into sparse distributed representations. This study addresses three fundamental research questions for Hierarchical Temporal Memory algorithms. What are the metrics for understanding the semantic content of sparse distributed representations? The semantic content and relationships between representations was visualized with uniqueness matrices and dimensionality reduction techniques. How can spatial semantic information in images be encoded into binary representations for the Hierarchical Temporal Memory's Spatial Pooler? A Contractive Autoencoder was exploited to create binary representations containing spatial information from image data. The uniqueness matrix shows that the Contractive Autoencoder encodes spatial information with strong spatial semantic relationships. The final question is how can vector operations of sparse distributed representations be enhanced to produce separable representations? A binding operation that results in a novel vector was implemented as a circular bit shift between two binary vectors. Binding of labeled sparse distributed representations was shown to create separable representations, but more robust representations are limited by vector density.
Publisher Site: RIT Repository
PDF (Requires Sign-In): PDF
Click for Bibtex
@book{boudreau2018contractive, title = {Contractive Autoencoding for Hierarchical Temporal Memory and Sparse Distributed Representation Binding}, author = {Boudreau, Luke G.}, year = {2018}, school = {Rochester Institute of Technology} }
[T-2018-28] Taylor Corrello. (2018). “Architectural Vulnerabilities in Plug-and-Play Systems.” Rochester Institute of Technology.
Abstract: Plug-and-play architectures enhance systems’ extensibility by providing a framework that enables additional functionalities to be added or removed from the system at their runtime. Such frameworks are often implemented through a set of well-defined interfaces that form the extension points for the pluggable functionalities. However, the plug-ins can increase the applications attack surface or introduce untrusted behavior into the system. Designing a secure plug-and-play architecture is critical and non-trivial as the features provided by plug-ins are not known in advance. In this paper, we conduct an in-depth study of seven systems with plug-and-play architectures. In total, we have analyzed 3,183 vulnerabilities from Chromium, Thunderbird, Firefox, Pidgin, WordPress, Apache OfBiz, and OpenMRS whose core architecture is based on a plug-and-play approach. We have also identified the common security vulnerabilities related to the plug-and-play architectures, and mechanisms to mitigate them by following a grounded theory approach. We found a total of 303 vulnerabilities that are rooted in extensibility design decisions. We also observed that these plugin-related vulnerabilities were caused by 15 different types of problems. We present these 15 types of security issues observed in the case studies and the design mechanisms that could prevent such vulnerabilities. Finally, as a result of this study, we have used formal modeling in order to guide developers of plug and play systems in verifying that their architectures are free of many of these types of security issues.
Publisher Site: RIT Repository
PDF (Requires Sign-In): PDF
Click for Bibtex
@book{corrello2018architectural, title = {Architectural Vulnerabilities in Plug-and-Play Systems}, author = {Corrello, Taylor}, year = {2018}, school = {Rochester Institute of Technology} }
[T-2018-27] David Stolze. (2018). “Discriminative Feature Extraction of Time-Series Data to Improve Temporal Pattern Detection using Classification Algorithms.” Rochester Institute of Technology.
Abstract: Time-series data streams often contain predictive value in the form of unique patterns. While these patterns may be used as leading indicators for event prediction, a lack of prior knowledge of pattern shape and irregularities can render traditional forecasting methods ineffective. The research in this thesis tested a means of predetermining the most effective combination of transformations to be applied to time-series data when training a classifier to predict whether an event will occur at a given time. The transformations tested on provided data streams included subsetting of the data, aggregation over various numbers of data points, testing of different predictive lead times, and converting the data set into a binary set of values. The benefit of the transformations is to reduce the data used for training down to only the most useful pattern containing points and clarify the predictive pattern contained in the set. In addition, the transformations tested significantly reduce the number of features used for classifier training through subsetting and aggregation. The performance benefit of the transformations was tested through creating a series of daily positive/negative event predictions over the span of a test set derived from each provided data stream. A landmarking system was then developed that utilizes the prior results obtained by the system to predetermine a “best fit” transformation to use on a new, untested data stream. Results indicate that the proposed set of transformations consistently result in improved classifier performance over the use of untransformed data values. Landmarking system testing shows that the use of prior knowledge results in selection of a near best fit transformation when using as few as 3 reference transformations.
Publisher Site: RIT Repository
PDF (Requires Sign-In): PDF
Click for Bibtex
@book{stolze2018discriminative, title = {Discriminative Feature Extraction of Time-Series Data to Improve Temporal Pattern Detection using Classification Algorithms}, author = {Stolze, David}, year = {2018}, school = {Rochester Institute of Technology} }
[T-2018-26] Nuzhet Nihaar Nasir Ahamed. (2018). “A New Graphene Quantum Dot Sensor for Estimating an Antibiotic Concentration.” Rochester Institute of Technology.
Abstract: The antibiotics have impacted the human ailments by curtailing the growth of microbes and by providing relief from microbial diseases. While there are a large number of analytical methods available for the determination of antibiotics concentration, they are time consuming and impractical for usage in the fields. This thesis is aimed at overcoming the deficiencies of those methods in developing a new sensor. It reports a study of graphene quantum dots (GQD) bound ferric ion for sensing an antibiotic, ciprofloxacin (CP). The interaction of ferric ion with CP was used as a probe for the analytical estimation of CP using differential pulse voltammetry (DPV). A solution containing ferric ion exhibits a well-defined cathodic peak at Epc=0.310 V vs saturated calomel electrode (SCE) with a peak width of 0.100V. When nanomolar to micromolar concentration of CP is present in the solution, along with ferric ion, three new peaks at EpcI=0.200V, EpcII=0.050 V and EpcIII=-0.085V are observed due to the binding of CP to ferric ion. The decrease in peak current of Epc at 0.310 V is proportional to the concentration of CP in the solution. The peak current at 0.200 V shows an increase corresponding to the CP concentration in solution. These results paved the way for examining the prospectus for developing a portable resistive sensor using interdigitated gold electrodes on alumina substrate. The principle of this sensor is based on that ferric ion bound to GQD will have a finite resistance and when it is bound to CP the resistance will increase as the charge transport faces a barrier due to bulky CP molecules. With a view to establish that ferric ion is binding to GQD, fluorescence of GQD has been recorded with ferric ion in solution. The approach adopted in developing resistive sensor is shown below. The numbers in the above picture denotes 1. Interdigitated gold electrodes 2. GQD bound interdigitated gold electrodes 3. Ferric ion bound to GQD 4. Attachment of CP to ferric ion. The sensor response is found to be dependent on the activity of the availability of ferric ion on GQD resulting in the usage of it as a disposable sensor. The interference of urea in the measurement of CP was examined for the practical usage of it in urine analysis
Publisher Site: RIT Repository
PDF (Requires Sign-In): PDF
Click for Bibtex
@book{nasir ahamed2018a, title = {A New Graphene Quantum Dot Sensor for Estimating an Antibiotic Concentration}, author = {Nasir Ahamed, Nuzhet Nihaar}, year = {2018}, school = {Rochester Institute of Technology} }
[T-2018-25] Nelson Henry Powell. (2018). “BATSEN: Modifying the BATMAN Routing Protocol for Wireless Sensor Networks.” Rochester Institute of Technology.
Abstract: The proliferation of autonomous Wireless Sensor Networks (WSN) has spawned research seeking power efficient communications to improve the lifetime of sensor motes. WSNs are characterized by their power limitations, wireless transceivers, and the converge-cast communications techniques. WSN motes use low-power, lossy radio systems deployed in dense, random topologies, working sympathetically to sense and notify a sink node of the detectable information. In an effort to extend the life of battery powered motes, and hence the life of the network, various routing protocols have been suggested in an effort to optimize converge-cast delivery of sensor data. It is well known that reducing the overhead required to perform converge-cast routing and communications reduces the effects of the primary power drain in the mote, the transceiver. Furthermore, WSNs are not well protected; network security costs energy both in computation and in RF transmission. This paper investigates the use of a Mobile Ad-hoc Networking (MANET) routing protocol known as B.A.T.M.A.N. in WSN. This thesis proposes that the features of B.A.T.M.A.N. in the MANET realm may prove beneficial to the WSN routing domain; and that slight modifications to the routing technique may prove beneficial beyond current protocol technologies. The B.A.T.M.A.N. variant will be compared against the contemporary LEACH WSN routing protocol to discern any potential energy savings.
Publisher Site: RIT Repository
PDF (Requires Sign-In): PDF
Click for Bibtex
@book{powell2018batsen:, title = {BATSEN: Modifying the BATMAN Routing Protocol for Wireless Sensor Networks}, author = {Powell, Nelson Henry, III}, year = {2018}, school = {Rochester Institute of Technology} }
[T-2018-24] Nilay Vijay Mokashi. (2018). “Empirical Satellite Characterization for Realistic Imagery Generation.” Rochester Institute of Technology.
Abstract: There is an increasing interest in the use of machine learning deep networks to automatically analyze satellite imagery. However, there are limited annotated satellite imagery datasets available for training these networks. Synthetic image generation offers a solution to this need, but only if the simulated images have comparable characteristics to the real data. This work deals with analysis of commercial satellite imagery to characterize their imaging systems for the purpose of increasing the realism of the synthetic imagery generated by RIT’s Digital Imaging and Remote Sensing Image Generation (DIRSIG) model. The analysis was applied to satellite imagery from Planet Labs and Digital Globe. Local spatial correlation was leveraged for noise estimation and the EMVA1288 standard was used for noise modeling. Real world calibration targets across the world were used together with the slanted edge method based on the ISO 12233 standard for estimation of the sensor optical systems’ point spread function (PSF). The estimated camera models were then used to generate synthetic imagery using DIRSIG. The PSF was applied within DIRSIG using its in-built functionality while noise was added in post processing. Analysis similar to real imagery was performed on the simulated scenes to verify the application of the model on synthetic scenes. Future work is recommended to further characterize the various imagery products produced by the satellite companies to better represent artifacts present in these processed images.
Publisher Site: RIT Repository
PDF (Requires Sign-In): PDF
Click for Bibtex
@book{mokashi2018empirical, title = {Empirical Satellite Characterization for Realistic Imagery Generation}, author = {Mokashi, Nilay Vijay}, year = {2018}, school = {Rochester Institute of Technology} }
[T-2018-23] Benjamin P. Stewart. (2018). “Cryogenic Operation of sCMOS Image Sensors.” Rochester Institute of Technology.
Abstract: Scientific CMOS image sensors have lower read noise and dark current than charge coupled devices. They are also uniquely qualified for operation at cryogenic temperatures due to their MOSFET pixel architecture. This paper follows the design of a cryogenic imaging system to be used as a star tracking rocket attitude regulation system. The detector was proven to retain almost all its sensitivity at cryogenic temperatures with acceptably low read noise. Once the star tracker successfully maintains rocket attitude during the flight of the CIBER-2 experiment, the technology readiness level of scientific CMOS detectors will advance enough that they could see potential applications in deep space imaging experiments.
Publisher Site: RIT Repository
PDF (Requires Sign-In): PDF
Click for Bibtex
@book{stewart2018cryogenic, title = {Cryogenic Operation of sCMOS Image Sensors}, author = {Stewart, Benjamin P.}, year = {2018}, school = {Rochester Institute of Technology} }
[T-2018-22] Saaim Valiani. (2018). “Graph-based Performance Estimation on Customized MIPS Processors.” Rochester Institute of Technology.
Abstract: The desire for greater processor performance with shrinking technologies and increasing heterogeneity, leads to a need for improvement in performance estimation. Being able to estimate the performance of an application without needing to implement the application on the available hardware and soft-core choices can decrease development time and help expedite the process of choosing which platform would be the best choice to use for development. This thesis work focuses on using a graph-based description of an application to estimate performance. By using a graph-based approach, the need for a hardware specific implementation is eliminated and the design space is simplified. Breaking down an application into a graph allows a new approach review to be taken as nodes of the graph can be assigned to levels in the pipelined architecture. This research uses pipelined customized Instruction Set Architecture (ISA) processors as the platform choice. The customized ISA soft-core processors allow the user more control over the resources used in the processor and provides a viable hardware/software choice to demonstrate the capabilities of the graph-based approach. The testcase applications used were the Dot Product, the Advanced Encryption Standard (AES) application, and the AES with TBox application. The results of this work show that performance can be accurately estimated on a customized processor using a graph-based approach for the application with accuracy ranging from approximately 75% to 89%.
Publisher Site: RIT Repository
PDF (Requires Sign-In): PDF
Click for Bibtex
@book{valiani2018graph-based, title = {Graph-based Performance Estimation on Customized MIPS Processors}, author = {Valiani, Saaim}, year = {2018}, school = {Rochester Institute of Technology} }
[T-2018-21] Bradley E. Conn. (2018). “Exploring High Level Synthesis to Improve the Design of Turbo Code Error Correction in a Software Defined Radio Context.” Rochester Institute of Technology.
Abstract: With the ever improving progress of technology, Software Defined Radio (SDR) has become a more widely available technique for implementing radio communication. SDRs are sought after for their advantages over traditional radio communication mostly in flexibility, and hardware simplification. The greatest challenges SDRs face are often with their real time performance requirements. Forward error correction is an example of an SDR block that can exemplify these challenges as the error correction can be very computationally intensive. Due to these constraints, SDR implementations are commonly found in or alongside Field Programmable Gate Arrays (FPGAs) to enable performance that general purpose processors alone cannot achieve. The main challenge with FPGAs however, is in Register Transfer Level (RTL) development. High Level Synthesis (HLS) tools are a method of creating hardware descriptions from high level code, in an effort to ease this development process. In this work a turbo code decoder, a form of computationally intensive error correction codes, was accelerated with the help of FPGAs, using HLS tools. This accelerator was implemented on a Xilinx Zynq platform, which integrates a hard core ARM processor alongside programmable logic on a single chip. Important aspects of the design process using HLS were identified and explained. The design process emphasizes the idea that for the best results the high level code should be created with a hardware mindset, and written in an attempt to describe a hardware design. The power of the HLS tools was demonstrated in its flexibility by providing a method of tailoring the hardware parameters through simply changing values in a macro file, and by exploration the design space through different data types and three different designs, each one improving from what was learned in the previous implementation. Ultimately, the best hardware implementation was over 56 times faster than the optimized software implementation. Comparing the HLS to a manually optimized design shows that the HLS implementation was able to achieve over a 19% throughput, with many areas for further improvement identified, demonstrating the competitiveness of the HLS tools.
Publisher Site: RIT Repository
PDF (Requires Sign-In): PDF
Click for Bibtex
@book{conn2018exploring, title = {Exploring High Level Synthesis to Improve the Design of Turbo Code Error Correction in a Software Defined Radio Context}, author = {Conn, Bradley E.}, year = {2018}, school = {Rochester Institute of Technology} }
[T-2018-20] Yash Nimkar. (2018). “Cache Memory Access Patterns in the GPU Architecture.” Rochester Institute of Technology.
Abstract: Data exchange between a Central Processing Unit (CPU) and a Graphic Processing Unit (GPU) can be very expensive in terms of performance. The characterization of data and cache memory access patterns differ between a CPU and a GPU. The motivation of this research is to analyze the cache memory access patterns of GPU architectures and to potentially improve data exchange between a CPU and GPU. The methodology of this work uses Multi2Sim GPU simulator for AMD Radeon and NVIDIA Kepler GPU architectures. This simulator, used to emulate the GPU architecture in software, enables certain code modifications for the L1 and L2 cache memory blocks. Multi2Sim was configured to run multiple benchmarks to analyze and record how the benchmarks access GPU cache memory. The recorded results were used to study three main metrics: (1) Most Recently Used (MRU) and Least Recently Used (LRU) accesses for L1 and L2 caches, (2) Inter-warp and Intra-warp cache memory accesses in the GPU architecture for different sets of workloads, and (3) To record and compare the GPU cache access patterns for certain machine learning benchmarks with its general purpose counterparts.
Publisher Site: RIT Repository
PDF (Requires Sign-In): PDF
Click for Bibtex
@book{nimkar2018cache, title = {Cache Memory Access Patterns in the GPU Architecture}, author = {Nimkar, Yash}, year = {2018}, school = {Rochester Institute of Technology} }
[T-2018-19] Cody W. Tinker. (2018). “Exploring the Application of Homomorphic Encryption for a Cross Domain Solution.” Rochester Institute of Technology.
Abstract: A cross domain solution is a means of information assurance that provides the ability to access or transfer digital data between varying security domains. Most acceptable cross domain solutions focus mainly on risk management policies that rely on using protected or trusted parties to handle the information in order to solve this problem; thus, a cross domain solution that is able to function in the presence of untrusted parties is an open problem. Homomorphic encryption is a type of encryption that allows its party members to operate and evaluate encrypted data without the need to decrypt it. Practical homomorphic encryption is an emerging technology that may propose a solution to the unsolved problem of cross domain routing without leaking information as well as many other unique scenarios. However, despite much advancement in research, current homomorphic schemes still challenge to achieve high performance. Thus, the plausibility of its implementation relies on the requirements of the tailored application. We apply the concepts of homomorphic encryption to explore a new solution in the context of a cross domain problem. We built a practical software case study application using the YASHE fully homomorphic scheme around the specific challenge of evaluating the gateway bypass condition on encrypted data. Next, we assess the plausibility of such an application through memory and performance profiling in order to find an optimal parameter selection that ensures proper homomorphic evaluation. The correctness of the application was assured for a 64-bit security parameter selection of YASHE resulting in high latency performance. However, literature has shown that the high latency performance can be heavily mitigated through use of hardware accelerators. Other configurations that include reducing number of SIMON rounds or avoiding the homomorphic SIMON evaluation completely were explored that show more promising performance results but either at the cost of security or network bandwidth.
Publisher Site: RIT Repository
PDF (Requires Sign-In): PDF
Click for Bibtex
@book{tinker2018exploring, title = {Exploring the Application of Homomorphic Encryption for a Cross Domain Solution}, author = {Tinker, Cody W.}, year = {2018}, school = {Rochester Institute of Technology} }
[T-2018-18] Dillon R. Graham. (2018). “Holographic Generative Memory: Neurally Inspired One-Shot Learning with Memory Augmented Neural Networks.” Rochester Institute of Technology.
Abstract: Humans quickly parse and categorize stimuli by combining perceptual information and previously learned knowledge. We are capable of learning new information quickly with only a few observations, and sometimes even a single observation. This one-shot learning (OSL) capability is still very difficult to realize in machine learning models. Novelty is commonly thought to be the primary driver for OSL. However, neuroscience literature shows that biological OSL mechanisms are guided by uncertainty, rather than novelty, motivating us to explore this idea for machine learning. In this work, we investigate OSL for neural networks using more robust compositional knowledge representations and a biologically inspired uncertainty mechanism to modulate the rate of learning. We introduce several new neural network models that combine Holographic Reduced Representation (HRR) and Variational Autoencoders. Extending these new models culminates in the Holographic Generative Memory (HGMEM) model. HGMEM is a novel unsupervised memory augmented neural network. It offers solutions to many of the practical drawbacks associated with HRRs while also providing storage, recall, and generation of latent compositional knowledge representations. Uncertainty is measured as a native part of HGMEM operation by applying trained probabilistic dropout to fully-connected layers. During training, the learning rate is modulated using these uncertainty measurements in a manner inspired by our motivating neuroscience mechanism for OSL. Model performance is demonstrated on several image datasets with experiments that reflect our theoretical approach.
Publisher Site: RIT Repository
PDF (Requires Sign-In): PDF
Click for Bibtex
@book{graham2018holographic, title = {Holographic Generative Memory: Neurally Inspired One-Shot Learning with Memory Augmented Neural Networks}, author = {Graham, Dillon R.}, year = {2018}, school = {Rochester Institute of Technology} }
[T-2018-17] Michael P. McClelland. (2018). “An Assessment of Small Unmanned Aerial Systems in Support of Sustainable Forestry Management Initiatives.” Rochester Institute of Technology.
Abstract: Sustainable forest management practices are receiving renewed attention in the growing effort to make efficient long-term use of natural resources. Sustainable management approaches require accurate and timely measurement of the world’s forests to monitor volume, biomass, and changes in sequestered carbon. It is in this context that remote sensing technologies, which possess the capability to rapidly capture structural data of entire forests, have become a key research area. Laser scanning systems, also known as lidar (light detection and ranging), have reached a maturity level where they may be considered a standard data source for structural measurements of forests; however, airborne lidar mounted on manned aircraft can be cost-prohibitive. The increasing performance capabilities and reduction of cost associated with small unmanned aerial systems (sUAS), coupled with the decreasing size and mass of lidar sensors, provide the potential for a cost-effective alternative. Our objectives for this study were to assess the extensibility of established airborne lidar algorithms to sUAS data and to evaluate the use of more cost-effective structure-from-motion (SfM) point cloud generation techniques from imagery obtained by the sUAS. A data collection was completed by both manned and sUAS lidar and imaging systems in Lebanon, VA and Asheville, NC. Both systems produced adequately dense point clouds with the manned system exceeding 30 pts/m^2 and the sUAS exceeding 400 pts/m^2. A cost analysis, two carbon models and a harvest detection algorithm were explored to test performance. It was found that the sUAS performed similarly on one of the two biomass models, while being competitive at a cost of $8.12/acre, compared to the manned aircraft’s cost of $8.09/acre, excluding mobilization costs of the manned system. On the biomass modeling front, the sUAS effort did not include enough data for training the second model or classifier, due to a lack of samples from data corruption. However, a proxy data set was generated from the manned aircraft, with similar results to the full resolution data, which then was compared to the sUAS data from four overlapping plots. This comparison showed good agreement between the systems when ingested into the trained airborne platform’s data model (RMSE = 1.77 Mg/ha). Producer’s accuracy, User’s accuracy, and the Kappa statistic for detection of harvested plots were 94.1%, 92.2% and 89.8%, respectively. A leave-one-out and holdout cross validation scheme was used to train and test the classifier, using 1000 iterations, with the mean values over all trials presented in this study. In the context of an investigative study, this classifier showed that the detection of harvested and non-harvested forest is possible with simple metrics derived from the vertical structure of the forest. Due to the closed nature of the forest canopy, the SfM data did not contain many ground returns, and thus, was not able to match the airborne lidar’s performance. It did, however, provide fine detail of the forest canopy from the sUAS platform. Overall, we concluded that sUAS is a viable alternative to airborne manned sensing platforms for fine-scale, local forest assessments, but there is a level of system maturity that needs to be attained for larger area applications.
Publisher Site: RIT Repository
PDF (Requires Sign-In): PDF
Click for Bibtex
@book{mcclelland2018an, title = {An Assessment of Small Unmanned Aerial Systems in Support of Sustainable Forestry Management Initiatives}, author = {McClelland, Michael P., II}, year = {2018}, school = {Rochester Institute of Technology} }
[T-2018-16] Tucker B. Graydon. (2018). “Novel Detection and Analysis using Deep Variational Autoencoders.” Rochester Institute of Technology.
Abstract: This paper presents a Novel Identification System which uses generative modeling techniques and Gaussian Mixture Models (GMMs) to identify the main process variables involved in a novel event from multivariate data. Features are generated and subsequently dimensionally reduced by using a Variational Autoencoder (VAE) supplemented by a denoising criterion and a β disentangling method. The GMM parameters are learned using the Expectation Maximization(EM) algorithm on features collected from only normal operating conditions. A one-class classification is achieved by thresholding the likelihoods by a statistically derived value. The Novel Identification method is verified as a detection method on existing Radio Frequency (RF) Generators and standard classification datasets. The RF dataset contains 2 different models of generators with almost 100 unique units tested. Novel Detection on these generators achieved an average testing true positive rate of 97.31% with an overall target class accuracy of 98.16%. A second application has the network evaluate process variables of the RF generators when a novel event is detected. This is achieved by using the VAE decoding layers to map the GMM parameters back to a space equivalent to the original input, resulting in a way to directly estimate the process variables fitness.
Publisher Site: RIT Repository
PDF (Requires Sign-In): PDF
Click for Bibtex
@book{graydon2018novel, title = {Novel Detection and Analysis using Deep Variational Autoencoders}, author = {Graydon, Tucker B.}, year = {2018}, school = {Rochester Institute of Technology} }
[T-2018-15] Tanmay Vinay Shinde. (2018). “Design, Fault Modeling and Testing Of a Fully Integrated Low Noise Amplifier (LNA) in 45 nm CMOS Technology for Inter and Intra-Chip Wireless Interconnects.” Rochester Institute of Technology.
Abstract: Research in recent years has demonstrated that intra and inter-chip wireless interconnects are capable of establishing energy-efficient data communications within as well as between multiple chips. This thesis introduces a circuit level design of a source degenerated two stage common source low noise amplifier suitable for such wireless interconnects in 45-nm CMOS process. The design consists of a simple two-stage common source structure based Low Noise Amplifier (LNA) to boost the degraded received signal. Operating at 60GHz, the proposed low noise amplifier consumes only 4.88 mW active power from a 1V supply while providing 17.2 dB of maximum gain at 60 GHz operating frequency at very low noise figure of 2.8 dB, which translates to a figure of merit of 16.1 GHz and IIP3 as -14.38 dBm.
Publisher Site: RIT Repository
PDF (Requires Sign-In): PDF
Click for Bibtex
@book{shinde2018design,, title = {Design, Fault Modeling and Testing Of a Fully Integrated Low Noise Amplifier (LNA) in 45 nm CMOS Technology for Inter and Intra-Chip Wireless Interconnects}, author = {Shinde, Tanmay Vinay}, year = {2018}, school = {Rochester Institute of Technology} }
[T-2018-14] Snehal Sudhir Chitnavis. (2018). “Cross Layer Routing in Cognitive Radio Network Using Deep Reinforcement Learning.” Rochester Institute of Technology.
Abstract: Development of 5G technology and Internet of Things (IoT) devices has resulted in higher bandwidth requirements leading to increased scarcity of wireless spectrum. Cognitive Radio Networks (CRNs) provide an efficient solution to this problem. In CRNs, multiple secondary users share the spectrum band that is allocated to a primary network. This spectrum sharing of the primary spectrum band is achieved in this work by using an underlay scheme. In this scheme, the Signal to Interference plus Noise Ratio (SINR) caused to the primary due to communication between secondary users is kept below a threshold level. In this work, the CRNs perform cross-layer optimization by learning the parameters from the physical and the network layer so as to improve the end-to-end quality of experience for video traffic. The developed system meets the design goal by using a Deep Q-Network (DQN) to choose the next hop for transmitting based on the delay seen at each router, while maintaining SINR below the threshold set by primary channel. A fully connected feed-forward Multilayer Perceptron (MLP) is used by secondary users to approximate the action value function. The action value comprises of SINR to the primary user (at the physical layer) and next hop to the routers for each packet (at the network layer). The reward to this neural network is Mean Opinion Score (MOS) for video traffic which depends on the packet loss rate and the bitrate used for transmission. As compared to the implementation of DQN learning at the physical layer only, this system provides 30\% increase in the video quality for routers with small queue lengths and also achieves a balanced load on a network with routers with unequal service rates.
Publisher Site: RIT Repository
PDF (Requires Sign-In): PDF
Click for Bibtex
@book{chitnavis2018cross, title = {Cross Layer Routing in Cognitive Radio Network Using Deep Reinforcement Learning}, author = {Chitnavis, Snehal Sudhir}, year = {2018}, school = {Rochester Institute of Technology} }
[T-2018-13] Kevin Hannigan. (2018). “An Empirical Evaluation of the Indicators for Performance Regression Test Selection.” Rochester Institute of Technology.
Abstract: As a software application is developed and maintained, changes to the source code may cause unintentional slowdowns in functionality. These slowdowns are known as performance regressions. Projects which are concerned about performance oftentimes create performance regression tests, which can be run to detect performance regressions. Ideally we would run these tests on every commit, however, these tests usually need a large amount of time or resources in order to simulate realistic scenarios. The paper entitled "Perphecy: Performance Regression Test Selection Made Simple but Effective" presents a technique to solve this problem by attempting to predict the likelihood that a commit will cause a performance regression. They use static and dynamic analysis to gather several metrics for their prediction, and then they evaluate those metrics on several projects. This thesis seeks to replicate and expand on their work. This thesis aims in revisiting the above-mentioned research paper by replicating its experiments and extending it by including a larger set of code changes to better understand how several metrics can be combined to approximate a better prediction of any code change that may potentially introduce deterioration at the performance of the software execution. This thesis has successfully replicated the existing study along with generating more insights related to the approach, and provides an open-source tool that can help developers with detecting any performance regression within code changes as software evolves.
Publisher Site: RIT Repository
PDF (Requires Sign-In): PDF
Click for Bibtex
@book{hannigan2018an, title = {An Empirical Evaluation of the Indicators for Performance Regression Test Selection}, author = {Hannigan, Kevin}, year = {2018}, school = {Rochester Institute of Technology} }
[T-2018-12] Felisa Sze. (2018). “Simulation and Framework for the Humanoid Robot TigerBot.” Rochester Institute of Technology.
Abstract: Walking humanoid robotics is a developing field. Different humanoid robots allow for different kinds of testing. TigerBot is a new full-scale humanoid robot with seven degrees-of-freedom legs and with its specifications, it can serve as a platform for humanoid robotics research. Currently TigerBot has encoders set up on each joint, allowing for position control, and its sensors and joints connect to Teensy microcontrollers and the ODroid XU4 single-board computer central control unit. The components’ communication system used the Robot Operating System (ROS). This allows the user to control TigerBot with ROS. It’s important to have a simulation setup so a user can test TigerBot’s capabilities on a model before using the real robot. A working walking gait in the simulation serves as a test of the simulator, proves TigerBot’s capability to walk, and opens further development on other walking gaits. A model of TigerBot was set up using the simulator Gazebo, which allowed testing different walking gaits with TigerBot. The gaits were generated by following the linear inverse pendulum model and the basic zero-moment point (ZMP) concept. The gaits consisted of center of mass trajectories converted to joint angles through inverse kinematics. In simulation while the robot follows the predetermined joint angles, a proportional-integral controller keeps the model upright by modifying the flex joint angle of the ankles. The real robot can also run the gaits while suspended in the air. The model has shown the walking gait based off the ZMP concept to be stable, if slow, and the actual robot has been shown to air walk following the gait. The simulation and the framework on the robot can be used to continue work with this walking gait or they can be expanded on for different methods and applications such as navigation, computer vision, and walking on uneven terrain with disturbances.
Publisher Site: RIT Repository
PDF (Requires Sign-In): PDF
Click for Bibtex
@book{sze2018simulation, title = {Simulation and Framework for the Humanoid Robot TigerBot}, author = {Sze, Felisa}, year = {2018}, school = {Rochester Institute of Technology} }
[T-2018-11] Ian Richard Prechtl. (2018). “LVAD Occlusion Condition Monitoring Using State Augmented Acoustic Spectral Images.” Rochester Institute of Technology.
Abstract: Each year, thousands of people die from heart disease and related illnesses due to the lack of available donor organs. Left ventricular assist devices (LVADs) aim to mitigate that occurrence, serving as a bridge-to-surgery option. While short term survival rates of LVAD patients near that of orthotopic surgery they are not viable long term options due to varied reasons. This work examines one cause, outlet graft thrombosis, and develops an algorithm for increasingly robust classification of device condition as it pertains to thrombosis or more generally occlusion. In order to do so an in vitro heart simulator is developed so that varying degrees of signal non-stationarity can be simulated and tested over a wide range of physiological blood pressure and heart rate conditions. Using a seeded-fault methodology, acoustics are acquired at the LVAD outlet graft location and subsequent spectral images of the sounds are developed. Statistical parameters from the images are used as features for classification using a support vector machine (SVM) which yields promising results. Given a comprehensive training space classification can be performed to fair accuracies (roughly 80%) using only the spectral image parameters. However, when the training space is limited augmenting the image features with patient state parameters elicits more robust identification. The algorithm developed in this work offers non-invasive diagnostic potential for LVAD conditions otherwise requiring invasive means.
Publisher Site: RIT Repository
PDF (Requires Sign-In): PDF
Click for Bibtex
@book{prechtl2018lvad, title = {LVAD Occlusion Condition Monitoring Using State Augmented Acoustic Spectral Images}, author = {Prechtl, Ian Richard}, year = {2018}, school = {Rochester Institute of Technology} }
[T-2018-10] Margot Accetttura. (2018). “Methods for the Detection of Subterranean Methane Leakage.” Rochester Institute of Technology.
Abstract: The development of small unmanned aircraft systems (sUAS) has led to a plethora of industry applications. One such application for a sUAS is detecting subterranean methane leakage. The rapid detection of methane will streamline work in industries such as construction and utilities. However, prior to flying a sUAS, the optimal way to detect methane must be determined so that unknown levels of subterranean methane leakage can be detected accurately and efficiently. In this thesis, two methods were used in conjunction to optimize a sUAS method for methane detection. The primary objective was to use hyperspectral data to locate the optimal wavelengths for methane detection for use on a sUAS. This was accomplished in two parts. The first part of the study was a simulated pipeline experiment where a copper pipe and mass flow controller were used to mimic a natural pipeline leak close to the surface. The methane-stressed and healthy vegetation were measured daily using a handheld spectrometer alongside two other forms of stressed vegetation. The analysis of the data showed potentially important variation at a two band combination of wavelengths. The second part of the study used the measured hyperspectral data as targets for a combination of atmospheric models developed using the MODerate resolution atmospheric TRANsmission (MODTRAN) algorithm at a variety of currently valid sUAS altitudes of operation. This study evaluated whether altitude will affect the ability to detect methane, along with determining which wavelength combination is best for use on a sUAS. The final assessment of an optimal application was made in regards to accuracy of methane detection within the MODTRAN data, as well as the cost analysis for industries who want to implement sUAS methane detection.
Publisher Site: RIT Repository
PDF (Requires Sign-In): PDF
Click for Bibtex
@book{accetttura2018methods, title = {Methods for the Detection of Subterranean Methane Leakage}, author = {Accetttura, Margot}, year = {2018}, school = {Rochester Institute of Technology} }
[T-2018-09] Jordana O’Brien. (2018). “A Mathematical Model of Fluid Flow in Evaporating Droplets Using Wedge Geometry.” Rochester Institute of Technology.
Abstract: As a droplet of liquid evaporates, particles within the droplet are often pulled to the edge and deposited in a ring-shaped pattern. This is known as the coffee-ring effect. The coffee-ring effect is largely due to evaporation taking place at a pinned contact line. Since this formation is an adverse outcome in many practical applications, methods to counteract the coffee-ring effect have become of interest, including the application of an electric field. In this research project, we discuss the first stage in constructing a coupled mathematical model of colloidal transport within an evaporating droplet under the influence of an electric field. The first stage is to simply capture the fluid flow within a pinned evaporating droplet without any consideration of particles or an electric field. We consider a thin axisymmetric droplet of a Newtonian solvent in contact with ambient air that is undergoing diffusion-limited evaporation. Away from the contact line, we model the droplet dynamics by applying the lubrication approximation to simplify the Navier-Stokes equations. To characterize the flow near the contact line, we assume the droplet shape is a wedge and derive analytical solutions of evaporative-driven Stokes flow near a pinned contact line. We connect the lubrication model and the wedge model by specifying height and flux conditions at the boundary between the two regions. We solve for the position of the droplet interface by implementing a method of lines approach in MATLAB. We find that for our specified conditions, the two regions evaporate on different time scales. However, if either the evaporation rate or the rate of contact angle decrease is appropriately adjusted, the two regions evaporate simultaneously.
Publisher Site: RIT Repository
PDF (Requires Sign-In): PDF
Click for Bibtex
@book{o'brien2018a, title = {A Mathematical Model of Fluid Flow in Evaporating Droplets Using Wedge Geometry}, author = {O'Brien, Jordana}, year = {2018}, school = {Rochester Institute of Technology} }
[T-2018-08] Rohan N. Dhamdhere. (2018). “Meta Learning for Graph Neural Networks.” Rochester Institute of Technology.
Abstract: Deep learning has enabled incredible advances in pattern recognition such as the fields of computer vision and natural language processing. One of the most successful areas of deep learning is Convolutional Neural Networks (CNNs). CNNs have helped improve performance on many difficult video and image understanding tasks but are restricted to dense gridded structures. Further, designing their architectures can be challenging, even for image classification problems. The recently introduced graph CNNs can work on both dense gridded structures as well as generic graphs. Graph CNNs have been performing at par with traditional CNNs on tasks such as point cloud classification and segmentation, protein classification and image classification, while reducing the complexity of the network. Graph CNNs provide an extra challenge in designing architectures due to more complex weight and filter visualization of generic graphs. Designing neural network architectures, yielding optimal performance, is a laborious and rigorous process. Hyperparameter tuning is essential for achieving state of the art results using specific architectures. Using a rich suite of predefined mutations, evolutionary algorithms have had success in delivering a high-quality population from a low-quality starter population. This thesis research formulates the graph CNN architecture design as an evolutionary search problem to generate a high-quality population of graph CNN model architectures for classification tasks on benchmark datasets.
Publisher Site: RIT Repository
PDF (Requires Sign-In): PDF
Click for Bibtex
@book{dhamdhere2018meta, title = {Meta Learning for Graph Neural Networks}, author = {Dhamdhere, Rohan N.}, year = {2018}, school = {Rochester Institute of Technology} }
[T-2018-07] Christopher O’Connell. (2018). “An Etching Study for Self-Aligned Double Patterning.” Rochester Institute of Technology.
Abstract: A proposed process flow for a complete FinFET etch module is presented as well as experiments to ensure that the target films are etched uniformly with proper rate, selectivity and anisotropy. The proposed process flow was developed at RIT, designed to closely reproduce what the semiconductor industry uses for a Self-Aligned Double Patterning (SADP) process module while advancing RIT's current cleanroom facility capabilities. The etching experiment is proposed such that a sufficient degree of etch endpoint control can be achieved without a spectrophotometer for endpoint detection using the Magnetically Enhanced Reactive Ion Etching (MERIE) system at RIT. Without the proper etch data a number of critical steps would be incredibly difficult to control. Prior to this work across wafer etch non-uniformity was reported to be approximately 10% with a regular rate of 1400-1500A/min. This was improved through various means to a nonuniformity of
Publisher Site: RIT Repository
PDF (Requires Sign-In): PDF
Click for Bibtex
@book{o'connell2018an, title = {An Etching Study for Self-Aligned Double Patterning}, author = {O'Connell, Christopher}, year = {2018}, school = {Rochester Institute of Technology} }
[T-2018-06] Saloni Mahendra Jain. (2018). “Detection of Autism using Magnetic Resonance Imaging data and Graph Convolutional Neural Networks.” Rochester Institute of Technology.
Abstract: Autism or Autism Spectrum Disorder (ASD) is a development disability which generally begins during childhood and may last throughout the lifetime of an individual. It is generally associated with difficulty in communication and social interaction along with repetitive behavior. One out of every 59 children in the United States is diagnosed with ASD [11] and almost 1% of the world population has ASD [12]. ASD can be difficult to diagnose as there is no definite medical test to diagnose this disorder. The aim of this thesis is to extract features from resting state functional Magnetic Resonance Imaging (rsfMRI) data as well as some personal information provided about each subject to train variations of a Graph Convolutional Neural Network to detect if a subject is Autistic or Neurotypical. The time series information as well as the connectivity information of specific parts of the brain are the features used for analysis. The thesis converts fMRI data into a graphical representation where the vertex represents a part of the brain and the edge represents the connectivity between two parts of the brain. New adjacency matrix filters were added to the Graph CNN model and the model was altered to add a time dimension.
Publisher Site: RIT Repository
PDF (Requires Sign-In): PDF
Click for Bibtex
@book{jain2018detection, title = {Detection of Autism using Magnetic Resonance Imaging data and Graph Convolutional Neural Networks}, author = {Jain, Saloni Mahendra}, year = {2018}, school = {Rochester Institute of Technology} }
[T-2018-05] Tomothy D. Rupright. (2018). “Multi‐Modal Analysis of Deciduous Tree Stands Toward Ash Tree Inventory: Biomass Estimation and Genus‐Level Discrimination in a Mixed Deciduous Forest.” Rochester Institute of Technology.
Abstract: The invasive species Agrilus planipennis (Emerald Ash Borer or EAB) is currently impacting ash trees in a large part of the continental United States. One way of measuring the effect of this infestation on the US markets is to determine the spread of the species and the biomass destruction/loss due to this invasive pest. In order to assess such impacts, it is necessary to determine how and where ash trees are located toward accurately measuring the relevant ash species biomass and tree count. This study utilizes data captured over the campus of Rochester Institute of Technology in Rochester, NY. Hyperspectral data, captured by SpecTIR, and discrete LiDAR data collected by an ALS 50 are used in an attempt to accomplish two tasks: 1) estimate ash biomass and 2) create a discriminant model to determine what types of trees (genera) are located within our surveyed plots. We surveyed four deciduous plots on the RIT campus and integrated the surveyed areas, the LiDAR data, and the hyperspectral data into a single comprehensive dataset. Our assessment incorporated different underlying models, including hyperspectral data only, LiDAR data only, and a combination of hyperspectral and LiDAR data. The results indicate that we can predict biomass with an R2 value between 0.55-0.69, at an α=0.01 statistical threshold and an R2 value between 0.85-0.92 (α=0.05 threshold) with the best models. The results indicate that smaller plot radius hyperspectral plus LiDAR and larger radius hyperspectral approaches scored best for R2 values, but the best RMSE was returned by the model utilizing the larger-radius hyperspectral data plus LiDAR returns. These indicated a slight difference in precision and accuracy between the models. The genus-level classification analysis utilized a stepwise discriminant model to extract relevant variables, followed by a linear discriminant classification which classified each tree based on the stepwise results. These models found that one-meter hyperspectral data plus LiDAR could accurately assess the genus level of the trees 86% of the time, with a KHAT score of 0.86. User and producer accuracies on that model vary from 73-100%, depending on the genus. This study contributes to the effort for combining hyperspectral and LiDAR data to assess deciduous tree stands. Such an approach to biomass modeling is often used in coniferous forests with high accuracy; however, the variability in uneven-aged and complex deciduous forest typically leads to poorer structural assessment outcomes, even though such species are spectrally more differentiable. Results here indicate that utilizing more robust LiDAR scans (point density) and techniques (data fusion), these methods could yield valuable genus-level biomass or carbon maps per forest genus. Such maps in turn could prove useful to decision-makers from policy experts to conservationists.
Publisher Site: RIT Repository
PDF (Requires Sign-In): PDF
Click for Bibtex
@book{rupright2018multi‐modal, title = {Multi‐Modal Analysis of Deciduous Tree Stands Toward Ash Tree Inventory: Biomass Estimation and Genus‐Level Discrimination in a Mixed Deciduous Forest}, author = {Rupright, Tomothy D.}, year = {2018}, school = {Rochester Institute of Technology} }
[T-2018-04] Bhavan Kumar Vasu. (2018). “Visualizing Resiliency Of Deep Convolutional Network Interpretations For Aerial Imagery.” Rochester Institute of Technology.
Abstract: This thesis aims at visualizing deep convolutional neural network interpretations for aerial imagery and understanding how these interpretations change when network weights are damaged. We focus our investigation on networks for aerial imagery, as these may be prone to damages due to harsh operating conditions and are usually inaccessible for regular maintenance once deployed. We simulate damages by zeroing network weights at different levels of the network and analyze their effects on activation maps. Then we re-train the network to observe if it can recover the lost interpretations. Visualizing changes in the neural network's interpretation, when the undamaged weights are retrained, allows us to visually assess the resiliency of a network. Our experiments on the AID and the UC Merced Land Use aerial datasets demonstrate the emergence of object and texture detectors in convolutional networks commonly used for classification. We further analyze these interpretations when the network is trained on one dataset and tested on another to demonstrate the robustness of feature learning across aerial datasets. We also explore the shift in interpretations when transfer learning is performed from an aerial dataset (AID) to a generic object dataset (MS-COCO). These results illustrate how transfer learning benefits the network's internal representations. Additionally, we explore the effects of various kinds of pooling operations for class activation map extraction and their resiliency to coefficient damages. Finally, we investigate the effects of network retraining by visualizing the change in the network's degraded interpretations before and after retraining. Our visualization results offer insights on the resiliency of some of the most commonly used networks, such as VGG16, ResNet50, and DenseNet121. This type of analysis can help guide prudent choices when it comes to selecting the network architecture during development and deployment under challenging conditions.
Publisher Site: RIT Repository
PDF (Requires Sign-In): PDF
Click for Bibtex
@book{vasu2018visualizing, title = {Visualizing Resiliency Of Deep Convolutional Network Interpretations For Aerial Imagery}, author = {Vasu, Bhavan Kumar}, year = {2018}, school = {Rochester Institute of Technology} }
[T-2018-03] Ian Tomeo. (2018). “Covariance Estimation from Limited Data: State-of-the-Art, Algorithm Implementation, and Application to Wireless Communications.” Rochester Institute of Technology.
Abstract: There are many different methods of filtering that have been known to provide better results than basic Sample-Matrix-Inversion (SMI). Providing a well-conditioned covariance matrix to these filters will provide a higher Signal to Interference plus Noise Ratio (SINR) than SMI filtering when there are large numbers of receiver element and interference signals. The available number of coherent snapshots that will be used for filter estimation is “not large enough” with respect to the array dimensionality. Common techniques to deal with this situation is to use Krylov or Singular Value Decomposition (SVD) reduced rank filtering. Auxiliary Vector (AV) filtering with the CV-MOV and J Divergence methods of choosing a termination index is improved upon by the hybrid estimation technique JMOV. When the Signal to Noise Ratio (SNR) is below 0 [dB] the signal of interest is buried in noise and estimation of the total number of signals present in a system is poor. If we fix the eigenvalues to be constant for eigenvectors that are not thought to correspond to signals, we use the eigenvector information that we have already went through the effort of performing SVD for. Sometimes the Eigenvalue Fixing (EIF) filtering produces better results than reduced rank filtering alone. The performance of the aforementioned filters when utilizing modern covariance matrix estimates is analyzed by varying parameters of the system model.
Publisher Site: RIT Repository
PDF (Requires Sign-In): PDF
Click for Bibtex
@book{tomeo2018covariance, title = {Covariance Estimation from Limited Data: State-of-the-Art, Algorithm Implementation, and Application to Wireless Communications}, author = {Tomeo, Ian}, year = {2018}, school = {Rochester Institute of Technology} }
[T-2018-02] Andrew J. Ramsey. (2018). “Automatic Modulation Classification Using Cyclic Features via Compressed Sensing.” Rochester Institute of Technology.
Abstract: Cognitive Radios (CRs) are designed to operate with minimal interference to the Primary User (PU), the incumbent to a radio spectrum band. To ensure that the interference generated does not exceed a specific level, an estimate of the Signal to Interference plus Noise Ratio (SINR) for the PU’s channel is required. This can be accomplished through determining the modulation scheme in use, as it is directly correlated with the SINR. To this end, an Automatic Modulation Classification (AMC) scheme is developed via cyclic feature detection that is successful even with signal bandwidths that exceed the sampling rate of the CR. In order to accomplish this, Compressed Sensing (CS) is applied, allowing for reconstruction, even with very few samples. The use of CS in spectrum sensing and interpretation is becoming necessary for a growing number of scenarios where the radio spectrum band of interest cannot be fully measured, such as low cost sensor networks, or high bandwidth radio localization services. In order to be able to classify a wide range of modulation types, cumulants were chosen as the feature to use. They are robust to noise and provide adequate discrimination between different types of modulation, even those that are fairly similar, such as 16-QAM and 64-QAM. By fusing cumulants and CS, a novel method of classification was developed which inherited the noise resilience of cumulants, and the low sample requirements of CS. Comparisons are drawn between the proposed method and existing ones, both in terms of accuracy and resource usages. The proposed method is shown to perform similarly when many samples are gathered, and shows improvement over existing methods at lower sample counts. It also uses less resources, and is able to produce an estimate faster than the current systems.
Publisher Site: RIT Repository
PDF (Requires Sign-In): PDF
Click for Bibtex
@book{ramsey2018automatic, title = {Automatic Modulation Classification Using Cyclic Features via Compressed Sensing}, author = {Ramsey, Andrew J.}, year = {2018}, school = {Rochester Institute of Technology} }
[T-2018-01] Sanjana Kapisthalam. (2018). “An Application of Deep-Learning to Understand Human Perception of Art.” Rochester Institute of Technology.
Abstract: Eye movement patterns are known to differ when looking at stimuli given a different task, but less is known about how these patterns change as a function of expertise. When a particular visual pattern is viewed, a particular sequence of eye movements are executed and this sequence is defined as scanpath. In this work we made an attempt to answer the question, “Do art novices and experts look at paintings differently?” If they do, we should be able to discriminate between the two groups using machine learning applied to their scanpaths. This can be done using algorithms for Multi-Fixation Pattern Analyses (MFPA). MFPA is a family of machine learning algorithms for making inferences about people from their gaze patterns. MFPA and related approaches have been widely used to study viewing behavior while performing visual tasks, but earlier approaches only used gaze position (x, y) information with duration and temporal order and not the actual visual features in the image. In this work, we extend MFPA algorithms to use visual features in trying to answer a question that has been overlooked by most early studies, i.e. if there is a difference found between experts and novices, how different are their viewing patterns and do these differences exist for both low- and high-level image features. To address this, we combined MFPA with a deep Convolutional Neural Network (CNN). Instead of converting a trial’s 2-D fixation positions into Fisher Vectors, we extracted image features surrounding the fixations using a deep CNN and turn them into Fisher Vectors for a trial. The Fisher Vector is an image representation obtained by pooling local image features. It is frequently used as a global image descriptor in visual classification. We call this approach MFPA-CNN. While CNNs have been previously used to recognize and classify objects from paintings, this work goes the extra step to study human perception of paintings. Ours is the first attempt to use MFPA and CNNs to study the viewing patterns of the subjects in the field of art. If our approach is successful in differentiating novices from experts with and without instructions when both low- and high-level CNN image features were used, we could then demonstrate that novices and experts view art differently. The outcome of this study could be then used to further investigate what image features the subjects are concentrating on. We expect this work to influence further research in image perception and experimental aesthetics.
Publisher Site: RIT Repository
PDF (Requires Sign-In): PDF
Click for Bibtex
@book{kapisthalam2018an, title = {An Application of Deep-Learning to Understand Human Perception of Art}, author = {Kapisthalam, Sanjana}, year = {2018}, school = {Rochester Institute of Technology} }
[T-2017-01] Narde, Rounak Singh. (2017). “Wireless Interconnects for Intra-chip & Inter-chip Transmission.” Rochester Institute of Technology.
Abstract: With the emergence of Internet of Things and information revolution, the demand of high performance computing systems is increasing. The copper interconnects inside the computing chips have evolved into a sophisticated network of interconnects known as Network on Chip (NoC) comprising of routers, switches, repeaters, just like computer networks. When network on chip is implemented on a large scale like in Multicore Multichip (MCMC) systems for High Performance Computing (HPC) systems, length of interconnects increases and so are the problems like power dissipation, interconnect delays, clock synchronization and electrical noise. In this thesis, wireless interconnects are chosen as the substitute for wired copper interconnects. Wireless interconnects offer easy integration with CMOS fabrication and chip packaging. Using wireless interconnects working at unlicensed mm-wave band (57-64GHz), high data rate of Gbps can be achieved. This thesis presents study of transmission between zigzag antennas as wireless interconnects for Multichip multicores (MCMC) systems and 3D IC. For MCMC systems, a four-chips 16-cores model is analyzed with only four wireless interconnects in three configurations with different antenna orientations and locations. Return loss and transmission coefficients are simulated in ANSYS HFSS. Moreover, wireless interconnects are designed, fabricated and tested on a 6'' silicon wafer with resistivity of 55Ω-cm using a basic standard CMOS process. Wireless interconnect are designed to work at 30GHz using ANSYS HFSS. The fabricated antennas are resonating around 20GHz with a return loss of less than -10dB. The transmission coefficients between antenna pair within a 20mm x 20mm silicon die is found to be varying between -45dB to -55dB. Furthermore, wireless interconnect approach is extended for 3D IC. Wireless interconnects are implemented as zigzag antenna. This thesis extends the work of analyzing the wireless interconnects in 3D IC with different configurations of antenna orientations and coolants. The return loss and transmission coefficients are simulated using ANSYS HFSS.
Publisher Site: RIT Repository
PDF (Requires Sign-In): PDF
Click for Bibtex
@book{narde2017wireless, title = {Wireless Interconnects for Intra-chip & Inter-chip Transmission}, author = {Narde, Rounak Singh}, year = {2017}, publisher = {Rochester Institute of Technology} }
Preprints
[P-2024-06] Sankaran, Prashant and McConky, Katie (2024). “Rethinking Selection in Generational Genetic Algorithms to Solve Combinatorial Optimization Problems: An Upper Bound-based Parent Selection Strategy for Recombination.” ArXiv Preprint.
Abstract: Existing stochastic selection strategies for parent selection in generational GA help build genetic diversity and sustain exploration; however, it ignores the possibility of exploiting knowledge gained by the process to make informed decisions for parent selection, which can often lead to an inefficient search for large, challenging optimization problems. This work proposes a deterministic parent selection strategy for recombination in a generational GA setting called Upper Bound-based Parent Selection (UBS) to solve NP-hard combinatorial optimization problems. Specifically, as part of the UBS strategy, we formulate the parent selection problem using the MAB framework and a modified UCB1 algorithm to manage exploration and exploitation. Further, we provided a unique similarity-based approach for transferring knowledge of the search progress between generations to accelerate the search. To demonstrate the effectiveness of the proposed UBS strategy in comparison to traditional stochastic selection strategies, we conduct experimental studies on two NP-hard combinatorial optimization problems: team orienteering and quadratic assignment. Specifically, we first perform a characterization study to determine the potential of UBS and the best configuration for all the selection strategies involved. Next, we run experiments using these best configurations as part of the comparison study. The results from the characterization studies reveal that UBS, in most cases, favors larger variations among the population between generations. Next, the comparison studies reveal that UBS can effectively search for high-quality solutions faster than traditional stochastic selection strategies on challenging NP-hard combinatorial optimization problems under given experimental conditions.
Publisher Site: arXiv
PDF (Requires Sign-In): PDF
Click for Bibtex
@article{sankaran2024rethinking, title = {Rethinking Selection in Generational Genetic Algorithms to Solve Combinatorial Optimization Problems: An Upper Bound-based Parent Selection Strategy for Recombination}, author = {Sankaran, Prashant and McConky, Katie}, journal = {arXiv preprint arXiv:2410.03863}, year = {2024} }
[P-2024-05] Rose, Caitlin, Kartaltepe, Jeyhan S., et al. (2024). “CEERS Key Paper. IX. Identifying Galaxy Mergers in CEERS NIRCam Images Using Random Forests and Convolutional Neural Networks.” ArXiv Preprint.
Abstract: A crucial yet challenging task in galaxy evolution studies is the identification of distant merging galaxies, a task which suffers from a variety of issues ranging from telescope sensitivities and limitations to the inherently chaotic morphologies of young galaxies. In this paper, we use random forests and convolutional neural networks to identify high-redshift JWST CEERS galaxy mergers. We train these algorithms on simulated 3<z<5 CEERS galaxies created from the IllustrisTNG subhalo morphologies and the Santa Cruz SAM lightcone. We apply our models to observed CEERS galaxies at 3<z<5. We find that our models correctly classify ∼60−70% of simulated merging and non-merging galaxies; better performance on the merger class comes at the expense of misclassifying more non-mergers. We could achieve more accurate classifications, as well as test for the dependency on physical parameters such as gas fraction, mass ratio, and relative orbits, by curating larger training sets. When applied to real CEERS galaxies using visual classifications as ground truth, the random forests correctly classified 40−60% of mergers and non-mergers at 3<z<4, but tended to classify most objects as non-mergers at 4<z<5 (misclassifying ∼70% of visually-classified mergers). On the other hand, the CNNs tended to classify most objects as mergers across all redshifts (misclassifying 80−90% of visually-classified non-mergers). We investigate what features the models find most useful, as well as characteristics of false positives and false negatives, and also calculate merger rates derived from the identifications made by the models.
Publisher Site: arXiv
PDF (Requires Sign-In): PDF
Click for Bibtex
@article{rose2024ceers, title = {CEERS Key Paper. IX. Identifying Galaxy Mergers in CEERS NIRCam Images Using Random Forests and Convolutional Neural Networks}, author = {Rose, Caitlin and Kartaltepe, Jeyhan S and Snyder, Gregory F and Huertas-Company, Marc and Yung, LY and Haro, Pablo Arrabal and Bagley, Micaela B and Bisigello, Laura and Calabr{\`o}, Antonello and Cleri, Nikko J and others}, journal = {arXiv preprint arXiv:2407.21279}, year = {2024} }
[P-2024-04] Khanal, Bidur and Dai, Tianhong and Bhattarai, Binod and Linte, Cristian. (2024). “Active Label Refinement for Robust Training of Imbalanced Medical Image Classification Tasks in the Presence of High Label Noise.” ArXiv Preprint.
Abstract: The robustness of supervised deep learning-based medical image classification is significantly undermined by label noise. Although several methods have been proposed to enhance classification performance in the presence of noisy labels, they face some challenges: 1) a struggle with class-imbalanced datasets, leading to the frequent overlooking of minority classes as noisy samples; 2) a singular focus on maximizing performance using noisy datasets, without incorporating experts-in-the-loop for actively cleaning the noisy labels. To mitigate these challenges, we propose a two-phase approach that combines Learning with Noisy Labels (LNL) and active learning. This approach not only improves the robustness of medical image classification in the presence of noisy labels, but also iteratively improves the quality of the dataset by relabeling the important incorrect labels, under a limited annotation budget. Furthermore, we introduce a novel Variance of Gradients approach in LNL phase, which complements the loss-based sample selection by also sampling under-represented samples. Using two imbalanced noisy medical classification datasets, we demonstrate that that our proposed technique is superior to its predecessors at handling class imbalance by not misidentifying clean samples from minority classes as mostly noisy samples.
Publisher Site: arXiv
PDF (Requires Sign-In): PDF
Click for Bibtex
@article{khanal2024active, title={Active Label Refinement for Robust Training of Imbalanced Medical Image Classification Tasks in the Presence of High Label Noise}, author={Khanal, Bidur and Dai, Tianhong and Bhattarai, Binod and Linte, Cristian}, journal={arXiv preprint arXiv:2407.05973}, year={2024} }
[P-2024-03] Fieblinger, Romy and Alam, Md Tanvirul and Rastogi, Nidhi. (2024). “Actionable Cyber Threat Intelligence using Knowledge Graphs and Large Language Models.” ArXiv Preprint.
Abstract: Cyber threats are constantly evolving. Extracting actionable insights from unstructured Cyber Threat Intelligence (CTI) data is essential to guide cybersecurity decisions. Increasingly, organizations like Microsoft, Trend Micro, and CrowdStrike are using generative AI to facilitate CTI extraction. This paper addresses the challenge of automating the extraction of actionable CTI using advancements in Large Language Models (LLMs) and Knowledge Graphs (KGs). We explore the application of state-of-the-art open-source LLMs, including the Llama 2 series, Mistral 7B Instruct, and Zephyr for extracting meaningful triples from CTI texts. Our methodology evaluates techniques such as prompt engineering, the guidance framework, and fine-tuning to optimize information extraction and structuring. The extracted data is then utilized to construct a KG, offering a structured and queryable representation of threat intelligence. Experimental results demonstrate the effectiveness of our approach in extracting relevant information, with guidance and fine-tuning showing superior performance over prompt engineering. However, while our methods prove effective in small-scale tests, applying LLMs to large-scale data for KG construction and Link Prediction presents ongoing challenges.
Publisher Site: arXiv
PDF (Requires Sign-In): PDF
Click for Bibtex
@article{fieblinger2024actionable, title={Actionable Cyber Threat Intelligence using Knowledge Graphs and Large Language Models}, author={Fieblinger, Romy and Alam, Md Tanvirul and Rastogi, Nidhi}, journal={arXiv preprint arXiv:2407.02528}, year={2024} }
[P-2024-02] Giammarese, Adam and Rana, Kamal and Bollt, Erik M. and Malik, Nishant. (2024). “Tree-based Learning for High-Fidelity Prediction of Chaos.” ArXiv Preprint.
Abstract: Model-free forecasting of the temporal evolution of chaotic systems is crucial but challenging. Existing solutions require hyperparameter tuning, significantly hindering their wider adoption. In this work, we introduce a tree-based approach not requiring hyperparameter tuning: TreeDOX. It uses time delay overembedding as explicit short-term memory and Extra-Trees Regressors to perform feature reduction and forecasting. We demonstrate the state-of-the-art performance of TreeDOX using the Henon map, Lorenz and Kuramoto-Sivashinsky systems, and the real-world Southern Oscillation Index.
Publisher Site: arXiv
PDF (Requires Sign-In): PDF
Click for Bibtex
@article{giammarese2024tree, title = {Tree-based Learning for High-Fidelity Prediction of Chaos}, author = {Giammarese, Adam and Rana, Kamal and Bollt, Erik M and Malik, Nishant}, journal = {arXiv preprint arXiv:2403.13836}, year = {2024} }
[P-2024-01] Khanal, Bidur and Shrestha, Prashant and Amgain, Sanskar and Khanal, Bishesh and Bhattarai, Binod and Linte, Cristian A. (2024). “Investigating the Robustness of Vision Transformers against Label Noise in Medical Image Classification.” ArXiv Preprint.
Abstract: Label noise in medical image classification datasets significantly hampers the training of supervised deep learning methods, undermining their generalizability. The test performance of a model tends to decrease as the label noise rate increases. Over recent years, several methods have been proposed to mitigate the impact of label noise in medical image classification and enhance the robustness of the model. Predominantly, these works have employed CNN-based architectures as the backbone of their classifiers for feature extraction. However, in recent years, Vision Transformer (ViT)-based backbones have replaced CNNs, demonstrating improved performance and a greater ability to learn more generalizable features, especially when the dataset is large. Nevertheless, no prior work has rigorously investigated how transformer-based backbones handle the impact of label noise in medical image classification. In this paper, we investigate the architectural robustness of ViT against label noise and compare it to that of CNNs. We use two medical image classification datasets -- COVID-DU-Ex, and NCT-CRC-HE-100K -- both corrupted by injecting label noise at various rates. Additionally, we show that pretraining is crucial for ensuring ViT's improved robustness against label noise in supervised training.
Publisher Site: arXiv
PDF (Requires Sign-In): PDF
Click for Bibtex
@article{khanal2024investigating, title = {Investigating the Robustness of Vision Transformers against Label Noise in Medical Image Classification}, author = {Khanal, Bidur and Shrestha, Prashant and Amgain, Sanskar and Khanal, Bishesh and Bhattarai, Binod and Linte, Cristian A}, journal = {arXiv preprint arXiv:2402.16734}, year = {2024} }
[P-2023-05] Cromer, Michael and Vasquez, Paula A. (2023). “Macro-Micro Coupled Simulations of Dilute Viscoelastic Fluids.” Preprints.
Abstract: Modeling the flow of polymer solutions requires knowledge at various length and time scales. The macroscopic behavior is described by the overall velocity, pressure and stress. The polymeric contribution to the stress requires knowledge of the evolution of polymer chains. In this work, we use a microscopic model, the finitely extensible nonlinear elastic (FENE) model, to capture the polymer behavior. The benefit of microscopic models is they remain faithful to the polymer dynamics without information loss via averaging. The downside is the computational cost needed to solve the thousands to millions of differential equations describing the microstructure. We thus describe a multiscale flow solver that utilizes GPUs for massively parallel, efficient simulations. We compare and contrast the microscopic model with its macroscopic counterpart under various flow conditions. In particular, significant differences are observed under nonlinear flow conditions, where the polymers become highly stretched and oriented.
Publisher Site: Preprints
PDF (Requires Sign-In): PDF
Click for Bibtex
@article{cromer2023macro, title = {Macro-Micro Coupled Simulations of Dilute Viscoelastic Fluids}, author = {Cromer, Michael and Vasquez, Paula A}, year = {2023}, publisher = {Preprints} }
[P-2023-04] Khanal, Bidur and Bhattarai, Binod and Knanal, Bishesh and Linte, Christian A. (2023). “Improving Medical Image Classification in Noisy Labels Using Only Self-Supervised Pretraining.” ArXiv Preprint.
Abstract: Noisy labels hurt deep learning-based supervised image classification performance as the models may overfit the noise and learn corrupted feature extractors. For natural image classification training with noisy labeled data, model initialization with contrastive self-supervised pretrained weights has shown to reduce feature corruption and improve classification performance. However, no works have explored: i) how other self-supervised approaches, such as pretext task-based pretraining, impact the learning with noisy label, and ii) any self-supervised pretraining methods alone for medical images in noisy label settings. Medical images often feature smaller datasets and subtle inter class variations, requiring human expertise to ensure correct classification. Thus, it is not clear if the methods improving learning with noisy labels in natural image datasets such as CIFAR would also help with medical images. In this work, we explore contrastive and pretext task-based self-supervised pretraining to initialize the weights of a deep learning classification model for two medical datasets with self-induced noisy labels -- NCT-CRC-HE-100K tissue histological images and COVID-QU-Ex chest X-ray images. Our results show that models initialized with pretrained weights obtained from self-supervised learning can effectively learn better features and improve robustness against noisy labels.
Publisher Site: arXiv
PDF (Requires Sign-In): PDF
Click for Bibtex
@article{khanal2023improving, title = {Improving Medical Image Classification in Noisy Labels Using Only Self-supervised Pretraining}, author = {Khanal, Bidur and Bhattarai, Binod and Khanal, Bishesh and Linte, Cristian A}, journal = {arXiv preprint arXiv:2308.04551}, year = {2023} }
[P-2023-03] Jahan, Chowdhury Sadman and Savakis, Andreas. (2023). “Continual Domain Adaptation on Aerial Images under Gradually Degrading Weather.” ArXiv Preprint.
Abstract: Domain adaptation (DA) strives to mitigate the domain gap between the source domain where a model is trained, and the target domain where the model is deployed. When a deep learning model is deployed on an aerial platform, it may face gradually degrading weather conditions during operation, leading to widening domain gaps between the training data and the encountered evaluation data. We synthesize two such gradually worsening weather conditions on real images from two existing aerial imagery datasets, generating a total of four benchmark datasets. Under the continual, or test-time adaptation setting, we evaluate three DA models on our datasets: a baseline standard DA model and two continual DA models. In such setting, the models can access only one small portion, or one batch of the target data at a time, and adaptation takes place continually, and over only one epoch of the data. The combination of the constraints of continual adaptation, and gradually deteriorating weather conditions provide the practical DA scenario for aerial deployment. Among the evaluated models, we consider both convolutional and transformer architectures for comparison. We discover stability issues during adaptation for existing buffer-fed continual DA methods, and offer gradient normalization as a simple solution to curb training instability.
Publisher Site: arXiv
PDF (Requires Sign-In): PDF
Click for Bibtex
@article{jahan2023continual, title = {Continual Domain Adaptation on Aerial Images under Gradually Degrading Weather}, author = {Jahan, Chowdhury Sadman and Savakis, Andreas}, journal = {arXiv preprint arXiv:2308.00924}, year = {2023} }
[P-2023-02] Jahan, Chowdhury Sadman and Savakis, Andreas. (2023). “Curriculum Guided Domain Adaptation in the Dark.” ArXiv Preprint.
Abstract: Addressing the rising concerns of privacy and security, domain adaptation in the dark aims to adapt a blackbox source trained model to an unlabeled target domain without access to any source data or source model parameters. The need for domain adaptation of black-box predictors becomes even more pronounced to protect intellectual property as deep learning based solutions are becoming increasingly commercialized. Current methods distill noisy predictions on the target data obtained from the source model to the target model, and/or separate clean/noisy target samples before adapting using traditional noisy label learning algorithms. However, these methods do not utilize the easy-to-hard learning nature of the clean/noisy data splits. Also, none of the existing methods are end-to-end, and require a separate fine-tuning stage and an initial warmup stage. In this work, we present Curriculum Adaptation for BlackBox (CABB) which provides a curriculum guided adaptation approach to gradually train the target model, first on target data with high confidence (clean) labels, and later on target data with noisy labels. CABB utilizes Jensen-Shannon divergence as a better criterion for clean-noisy sample separation, compared to the traditional criterion of cross entropy loss. Our method utilizes co-training of a dual-branch network to suppress error accumulation resulting from confirmation bias. The proposed approach is end-to-end trainable and does not require any extra finetuning stage, unlike existing methods. Empirical results on standard domain adaptation datasets show that CABB outperforms existing state-of-the-art black-box DA models and is comparable to white-box domain adaptation models.
Publisher Site: arXiv
PDF (Requires Sign-In): PDF
Click for Bibtex
@article{jahan2023curriculum, title = {Curriculum Guided Domain Adaptation in the Dark}, author = {Jahan, Chowdhury Sadman and Savakis, Andreas}, journal = {arXiv preprint arXiv:2308.00956}, year = {2023} }
[P-2023-01] ElSaid, AbdElRahman and Ricanek, Karl and Lyu, Zeming and Ororbia, Alexander and Desell, Travis. (2023). “Backpropagation-Free 4D Continuous Ant-Based Neural Topology Search.” ArXiv Preprint.
Abstract: Continuous Ant-based Topology Search (CANTS) is a previously introduced novel nature-inspired neural architecture search (NAS) algorithm that is based on ant colony optimization (ACO). CANTS utilizes a continuous search space to indirectly-encode a neural architecture search space. Synthetic ant agents explore CANTS' continuous search space based on the density and distribution of pheromones, strongly inspired by how ants move in the real world. This continuous search space allows CANTS to automate the design of artificial neural networks (ANNs) of any size, removing a key limitation inherent to many current NAS algorithms that must operate within structures of a size that is predetermined by the user. This work expands CANTS by adding a fourth dimension to its search space representing potential neural synaptic weights. Adding this extra dimension allows CANTS agents to optimize both the architecture as well as the weights of an ANN without applying backpropagation (BP), which leads to a significant reduction in the time consumed in the optimization process. The experiments of this study - using real-world data - demonstrate that the BP-Free CANTS algorithm exhibits highly competitive performance compared to both CANTS and ANTS while requiring significantly less operation time.
Publisher Site: arXiv
PDF (Requires Sign-In): PDF
Click for Bibtex
@article{elsaid2023backpropagation, title = {Backpropagation-Free 4D Continuous Ant-Based Neural Topology Search}, author = {ElSaid, AbdElRahman and Ricanek, Karl and Lyu, Zeming and Ororbia, Alexander and Desell, Travis}, journal = {arXiv preprint arXiv:2305.06715}, year = {2023} }
[P-2022-06] Shah, Ekta A. and Kartaltepe, Jeyhan S. and Magagnoli, Christina T. and Cox, Isabella G. and Wetherell, Caleb T. and Vanderhoof, Brittany N. and Cooke, Kevin C. and Calabro, Antonello and Chartab, Nima and Conselice, Christopher J. and Others. (2022). “Investigating the Effect of Galaxy Interactions on Star Formation at 0.5 < z < 3.0.” ArXiv Preprint.
Abstract: Observations and simulations of interacting galaxies and mergers in the local universe have shown that interactions can significantly enhance the star formation rates (SFR) and fueling of Active Galactic Nuclei (AGN). However, at higher redshift, some simulations suggest that the level of star formation enhancement induced by interactions is lower due to the higher gas fractions and already increased SFRs in these galaxies. To test this, we measure the SFR enhancement in a total of 2351 (1327) massive (M*>1010M) major (1<M1/M2<4) spectroscopic galaxy pairs at 0.5<z<3.0 with ΔV<5000 km s-1 (1000 km s-1) and projected separation <150 kpc selected from the extensive spectroscopic coverage in the COSMOS and CANDELS fields. We find that the highest level of SFR enhancement is a factor of 1.23+0.08-0.09 in the closest projected separation bin (<25 kpc) relative to a stellar mass-, redshift-, and environment-matched control sample of isolated galaxies. We find that the level of SFR enhancement is a factor of ~1.5 higher at 0.5<z<1 than at 1<z<3 in the closest projected separation bin. Among a sample of visually identified mergers, we find an enhancement of a factor of 1.86+0.29-0.18 for coalesced systems. For this visually identified sample, we see a clear trend of increased SFR enhancement with decreasing projected separation (2.40+0.62-0.37 vs. 1.58+0.29-0.20 for 0.5<z<1.6 and 1.6<z<3.0, respectively). The SFR enhancement seen in our interactions and mergers are all lower than the level seen in local samples at the same separation, suggesting that the level of interaction-induced star formation evolves significantly over this time period.
Publisher Site: arXiv
PDF (Requires Sign-In): PDF
Click for Bibtex
@article{shah2022investigating, title = {Investigating the Effect of Galaxy Interactions on Star Formation at 0.5< z< 3.0}, author = {Shah, Ekta A and Kartaltepe, Jeyhan S and Magagnoli, Christina T and Cox, Isabella G and Wetherell, Caleb T and Vanderhoof, Brittany N and Cooke, Kevin C and Calabro, Antonello and Chartab, Nima and Conselice, Christopher J and others}, journal = {arXiv preprint arXiv:2209.15587}, year = {2022} }
[P-2022-05] Rose, Caitlin and Kartaltepe, Jeyhan S and Snyder, Gregory F. and Rodriguez-Gomez, Vicente and Yung, L.Y. and Haro, Pablo Arrabal and Bagley, Micaela B. and Calabrò, Antonello and Cleri, Nikko J. and Cooper, M.C. and Others. (2022). “Identifying Galaxy Mergers in Simulated CEERS NIRCam Images using Random Forests.” ArXiv Preprint.
Abstract: Identifying merging galaxies is an important--but difficult--step in galaxy evolution studies. We present random forest classifications of galaxy mergers from simulated JWST images based on various standard morphological parameters. We describe (a) constructing the simulated images from IllustrisTNG and the Santa Cruz SAM, and modifying them to mimic future CEERS observations as well as nearly noiseless observations, (b) measuring morphological parameters from these images, and (c) constructing and training the random forests using the merger history information for the simulated galaxies available from IllustrisTNG. The random forests correctly classify ~ 60% of non-merging and merging galaxies across 0.5 < z < 4.0. Rest-frame asymmetry parameters appear more important for lower redshift merger classifications, while rest-frame bulge and clump parameters appear more important for higher redshift classifications. Adjusting the classification probability threshold does not improve the performance of the forests. Finally, the shape and slope of the resulting merger fraction and merger rate derived from the random forest classifications match with theoretical Illustris predictions, but are underestimated by a factor of ~ 0.5.
Publisher Site: arXiv
PDF (Requires Sign-In): PDF
Click for Bibtex
@article{rose2022identifying, title = {Identifying Galaxy Mergers in Simulated CEERS NIRCam Images using Random Forests}, author = {Rose, Caitlin and Kartaltepe, Jeyhan S and Snyder, Gregory F and Rodriguez-Gomez, Vicente and Yung, LY and Haro, Pablo Arrabal and Bagley, Micaela B and Calabr{\`o}, Antonello and Cleri, Nikko J and Cooper, MC and others}, journal = {arXiv preprint arXiv:2208.11164}, year = {2022} }
[P-2022-04] Lyu, Zimeng and Desell, Travis. (2022). “ONE-NAS: An Online NeuroEvolution based Neural Architecture Search for Time Series Forecasting.” ArXiv Preprint.
Abstract: Time series forecasting (TSF) is one of the most important tasks in data science, as accurate time series (TS) predictions can drive and advance a wide variety of domains including finance, transportation, health care, and power systems. However, real-world utilization of machine learning (ML) models for TSF suffers due to pretrained models being able to learn and adapt to unpredictable patterns as previously unseen data arrives over longer time scales. To address this, models must be periodically retained or redesigned, which takes significant human and computational resources. This work presents the Online NeuroEvolution based Neural Architecture Search (ONE-NAS) algorithm, which to the authors' knowledge is the first neural architecture search algorithm capable of automatically designing and training new recurrent neural networks (RNNs) in an online setting. Without any pretraining, ONE-NAS utilizes populations of RNNs which are continuously updated with new network structures and weights in response to new multivariate input data. ONE-NAS is tested on real-world large-scale multivariate wind turbine data as well a univariate Dow Jones Industrial Average (DJIA) dataset, and is shown to outperform traditional statistical time series forecasting, including naive, moving average, and exponential smoothing methods, as well as state of the art online ARIMA strategies.
Publisher Site: arXiv
PDF (Requires Sign-In): PDF
Click for Bibtex
@article{lyu2022one, title = {ONE-NAS: An Online NeuroEvolution based Neural Architecture Search for Time Series Forecasting}, author = {Lyu, Zimeng and Desell, Travis}, journal = {arXiv prepint arXiv:2202.13471}, year = {2022} }
[P-2022-03] Kumra, Sulabh and Joshi, Shirin and Sahin, Ferat. (2022). “Learning Multi-step Robotic Manipulation Policies from Visual Observation of Scene and Q-value Predictions of Previous Action.” ArXiv Preprint.
Abstract: In this work, we focus on multi-step manipulation tasks that involve long-horizon planning and considers progress reversal. Such tasks interlace high-level reasoning that consists of the expected states that can be attained to achieve an overall task and low-level reasoning that decides what actions will yield these states. We propose a sample efficient Previous Action Conditioned Robotic Manipulation Network (PAC-RoManNet) to learn the action-value functions and predict manipulation action candidates from visual observation of the scene and action-value predictions of the previous action. We define a Task Progress based Gaussian (TPG) reward function that computes the reward based on actions that lead to successful motion primitives and progress towards the overall task goal. To balance the ratio of exploration/exploitation, we introduce a Loss Adjusted Exploration (LAE) policy that determines actions from the action candidates according to the Boltzmann distribution of loss estimates. We demonstrate the effectiveness of our approach by training PAC-RoManNet to learn several challenging multi-step robotic manipulation tasks in both simulation and real-world. Experimental results show that our method outperforms the existing methods and achieves state-of-the-art performance in terms of success rate and action efficiency. The ablation studies show that TPG and LAE are especially beneficial for tasks like multiple block stacking. Additional experiments on Ravens-10 benchmark tasks suggest good generalizability of the proposed PAC-RoManNet.
Publisher Site: arXiv
PDF (Requires Sign-In): PDF
Click for Bibtex
@article{kumra2022learning, title = {Learning Multi-step Robotic Manipulation Policies from Visual Observation of Scene and Q-value Predictions of Previous Action}, author = {Kumra, Sulabh and Joshi, Shirin and Sahin, Ferat}, journal = {arXiv preprint arXiv:2202.11280}, year = {2022} }
[P-2022-02] Kartaltepe, Jeyhan S. and Rose, Caitlin and Vanderhoof, Brittany N. and McGrath, Elizabeth J. and Costantin, Luca and Cox, Isabella G. and Yung, L.Y. and Kocevski, Dale D. and Wuyts, Stijn and Andrews, Henry C. and Others. (2022). “CEERS Key Paper IV: The Diversity of Galaxy Structure and Morphology at z= 3-9 with JWST.” ArXiv Preprint.
Abstract: We present a comprehensive analysis of the evolution of the morphological and structural properties of a large sample of galaxies at z = 3 - 9 using the early JWST CEERS NIRCam observations taken in June 2022. Our sample consists of 850 galaxies at z > 3 detected in both CANDELS HST imaging of the EGS field as well as JWST CEERS NIRCam images to enable a comparison of HST and JWST morphologies. Our team conducted a set of visual classifications, with each galaxy in the sample classified by three different individuals. Due to the complexity of galaxy morphologies at these redshifts, our classifications are intentionally not mutually exclusive. Instead, we note the presence of disks, spheroidal components, and irregular features in each galaxy, allowing for more than one of these choices to be selected. We also measure quantitative morphologies using the publicly available codes Galfit, Galapagos-2/GalfitM, and statmorph across all seven NIRCam filters. Using these measurements, we present the fraction of galaxies of each morphological type as a function of redshift. Overall, we find that galaxies at z > 3 have a wide diversity of morphologies. Galaxies with disks make up a total of 60% of galaxies at z = 3 and this fraction drops to '30% at z = 6-9, while galaxies with spheroids make up ' 30 - 40% across the whole redshift range and pure spheroids with no evidence for disks or irregular features make up ' 20%. The fraction of galaxies with irregular features is roughly constant at all redshifts (' 40 - 50%), while those that are purely irregular increases from ' 12% to ' 20% at z > 4.5. We note that these are apparent fractions as many selection effects impact the visibility of morphological features at high redshift. The distributions of Sersic index, size, and axis ratios show significant differences between the morphological groups. On average, Spheroid Only galaxies have a higher Sersic index, smaller size, and higher axis ratio than Disk or Irregular galaxies. Across all redshifts, smaller spheroid and disk galaxies tend to be rounder. Overall, these trends suggest that galaxies with established disks and spheroids exist across the full redshift range of this study and further work with large samples at higher redshift is needed to quantify when these features first formed.
Publisher Site: arXiv
PDF (Requires Sign-In): PDF
Click for Bibtex
@article{kartaltepe2022ceers, title = {CEERS Key Paper IV: The Diversity of Galaxy Structure and Morphology at z= 3-9 with JWST}, author = {Kartaltepe, Jeyhan S and Rose, Caitlin and Vanderhoof, Brittany N and McGrath, Elizabeth J and Costantin, Luca and Cox, Isabella G and Yung, LY and Kocevski, Dale D and Wuyts, Stijn and Andrews, Henry C and others}, journal = {arXiv preprint arXiv:2210.14713}, year = {2022} }
[P-2022-01] Cooke, Kevin C. and Kartaltepe, Jeyhan S. and Rose, Caitlin and Tyler, K.D. and Darvish, Behnam and Leslie, Sarah K. and Peng, Ying-jie and Häußler, Boris and Koekemoer, Anton M. (2022). “Morphology & Environment’s Role on the Star Formation Rate-Stellar Mass Relation in COSMOS from 0 < z < 3.5.” ArXiv Preprint.
Abstract: We investigate the relationship between environment, morphology, and the star formation rate--stellar mass relation derived from a sample of star-forming galaxies (commonly referred to as the 'star formation main sequence') in the COSMOS field from 0 < z < 3.5. We constructed and fit the FUV-- FIR SEDs of our stellar mass-selected sample of 111,537 galaxies with stellar and dust emission models using the public packages MAGPHYS and SED3FIT. From the best fit parameter estimates, we construct the star formation rate-stellar mass relation as a function of redshift, local environment, NUVrJ color diagnostics, and morphology. We find that the shape of the main sequence derived from our color-color and sSFR-selected star forming galaxy population, including the turnover at high stellar mass, does not exhibit an environmental dependence at any redshift from 0 < z < 3.5. We investigate the role of morphology in the high mass end of the SFMS to determine whether bulge growth is driving the high mass turnover. We find that star-forming galaxies experience this turnover independent of bulge-to-total ratio, strengthening the case that the turnover is due to the disk component's specific star formation rate evolving with stellar mass rather than bulge growth.
Publisher Site: arXiv
PDF (Requires Sign-In): PDF
Click for Bibtex
@article{cooke2022morphology, title = {Morphology \& Environment's Role on the Star Formation Rate--Stellar Mass Relation in COSMOS from 0< z< 3.5}, author = {Cooke, Kevin C and Kartaltepe, Jeyhan S and Rose, Caitlin and Tyler, KD and Darvish, Behnam and Leslie, Sarah K and Peng, Ying-jie and H{\"a}u{\ss}ler, Boris and Koekemoer, Anton M}, journal = {arXiv preprint arXiv:2211.16527}, year = {2022} }
[P-2021-01] Taufique, Abu Md Niamul and Jahan, Chowdhury Sadman and Savakis, Andreas. (2021). “Conda: Continual Unsupervised Domain Adaptation.” ArXiv Preprint.
Abstract: Domain Adaptation (DA) techniques are important for overcoming the domain shift between the source domain used for training and the target domain where testing takes place. However, current DA methods assume that the entire target domain is available during adaptation, which may not hold in practice. This paper considers a more realistic scenario, where target data become available in smaller batches and adaptation on the entire target domain is not feasible. In our work, we introduce a new, data-constrained DA paradigm where unlabeled target samples are received in batches and adaptation is performed continually. We propose a novel source-free method for continual unsupervised domain adaptation that utilizes a buffer for selective replay of previously seen samples. In our continual DA framework, we selectively mix samples from incoming batches with data stored in a buffer using buffer management strategies and use the combination to incrementally update our model. We evaluate the classification performance of the continual DA approach with state-of-the-art DA methods based on the entire target domain. Our results on three popular DA datasets demonstrate that our method outperforms many existing state-of-the-art DA methods with access to the entire target domain during adaptation.
Publisher Site: arXiv
PDF (Requires Sign-In): PDF
Click for Bibtex
@article{taufique2021conda, title = {Conda: Continual unsupervised domain adaptation}, author = {Taufique, Abu Md Niamul and Jahan, Chowdhury Sadman and Savakis, Andreas}, journal = {arXiv preprint arXiv:2103.11056}, year = {2021} }
[P-2020-02] Lyu, Zimeng and Karns, Joshua and ElSaid, AbdElRahman and Desell, Travis. (2020). “Improving Neuroevolution Using Island Extinction and Repopulation.” ArXiv Preprint.
Abstract: Neuroevolution commonly uses speciation strategies to better explore the search space of neural network architectures. One such speciation strategy is through the use of islands, which are also popular in improving performance and convergence of distributed evolutionary algorithms. However, in this approach some islands can become stagnant and not find new best solutions. In this paper, we propose utilizing extinction events and island repopulation to avoid premature convergence. We explore this with the Evolutionary eXploration of Augmenting Memory Models (EXAMM) neuro-evolution algorithm. In this strategy, all members of the worst performing island are killed of periodically and repopulated with mutated versions of the global best genome. This island based strategy is additionally compared to NEAT's (NeuroEvolution of Augmenting Topologies) speciation strategy. Experiments were performed using two different real world time series datasets (coal-fired power plant and aviation flight data). The results show that with statistical significance, this island extinction and repopulation strategy evolves better global best genomes than both EXAMM's original island based strategy and NEAT's speciation strategy.
Publisher Site: arXiv
PDF (Requires Sign-In): PDF
Click for Bibtex
@article{lyu2020improving, title = {Improving neuroevolution using island extinction and repopulation}, author = {Lyu, Zimeng and Karns, Joshua and ElSaid, AbdElRahman and Desell, Travis}, journal = {arXiv preprint arXiv:2005.07376}, year = {2020} }
[P-2020-01] Lyu, Zimeng and ElSaid, AbdElRahman and Karns, Joshua and Mkaouer, Mohamed and Desell, Travis. (2020). “An Experimental Study of Weight Initialization and Weight Inheritance Effects on Neuroevolution.” ArXiv Preprint.
Abstract: Weight initialization is critical in being able to successfully train artificial neural networks (ANNs), and even more so for recurrent neural networks (RNNs) which can easily suffer from vanishing and exploding gradients. In neuroevolution, where evolutionary algorithms are applied to neural architecture search, weights typically need to be initialized at three different times: when initial genomes (ANN architectures) are created at the beginning of the search, when offspring genomes are generated by crossover, and when new nodes or edges are created during mutation. This work explores the difference between using Xavier, Kaiming, and uniform random weight initialization methods, as well as novel Lamarckian weight inheritance methods for initializing new weights during crossover and mutation operations. These are examined using the Evolutionary eXploration of Augmenting Memory Models (EXAMM) neuroevolution algorithm, which is capable of evolving RNNs with a variety of modern memory cells (e.g., LSTM, GRU, MGU, UGRNN and Delta-RNN cells) as well recurrent connections with varying time skips through a high performance island based distributed evolutionary algorithm. Results show that with statistical significance, utilizing the Lamarckian strategies outperforms Kaiming, Xavier and uniform random weight initialization, and can speed neuroevolution by requiring less backpropagation epochs to be evaluated for each generated RNN.
Publisher Site: arXiv
PDF (Requires Sign-In): PDF
Click for Bibtex
@article{lyu2020experimental, title = {An Experimental Study of Weight Initialization and Weight Inheritance Effects on Neuroevolution}, author = {Lyu, Zimeng and ElSaid, AbdElRahman and Karns, Joshua and Mkaouer, Mohamed and Desell, Travis}, journal = {arXiv preprint arXiv:2009.09644}, year = {2020} }
[P-2019-01] ElSaid, AbdElRahman A. and Ororbia, Alexander G. and Desell, Travis J. (2019). “The Ant Swarm Neuro-evolution Procedure for Optimizing Recurrent Networks.” ArXiv Preprint.
Abstract: Hand-crafting effective and efficient structures for recurrent neural networks (RNNs) is a difficult, expensive, and time-consuming process. To address this challenge, we propose a novel neuro-evolution algorithm based on ant colony optimization (ACO), called ant swarm neuro-evolution (ASNE), for directly optimizing RNN topologies. The procedure selects from multiple modern recurrent cell types such as delta-RNN, GRU, LSTM, MGU and UGRNN cells, as well as recurrent connections which may span multiple layers and/or steps of time. In order to introduce an inductive bias that encourages the formation of sparser synaptic connectivity patterns, we investigate several variations of the core algorithm. We do so primarily by formulating different functions that drive the underlying pheromone simulation process (which mimic L1 and L2 regularization in standard machine learning) as well as by introducing ant agents with specialized roles (inspired by how real ant colonies operate), i.e., explorer ants that construct the initial feed forward structure and social ants which select nodes from the feed forward connections to subsequently craft recurrent memory structures. We also incorporate a Lamarckian strategy for weight initialization which reduces the number of backpropagation epochs required to locally train candidate RNNs, speeding up the neuro-evolution process. Our results demonstrate that the sparser RNNs evolved by ASNE significantly outperform traditional one and two layer architectures consisting of modern memory cells, as well as the well-known NEAT algorithm. Furthermore, we improve upon prior state-of-the-art results on the time series dataset utilized in our experiments.
Publisher Site: arXiv
PDF (Requires Sign-In): PDF
Click for Bibtex
@article{elsaid2019ant, title = {The ant swarm neuro-evolution procedure for optimizing recurrent networks}, author = {ElSaid, AbdElRahman A and Ororbia, Alexander G and Desell, Travis J}, journal = {arXiv preprint arXiv:1909.11849}, year = {2019} }
Abstracts
[A-2023-01] Brilliantova, Angelina and Bezáková, Ivona. (2023). “Model Selection of Graph Signage Models Using Maximum Likelihood.”
Abstract: Complex systems across various domains can be naturally modeled as signed networks with positive and negative edges. In this work, we design a new class of signage models and show how to select the model parameters that best fit real-world datasets using maximum likelihood.
Publisher Site: AAAI
PDF (Requires Sign-In): PDF
Click for Bibtex
@inproceedings{brilliantova2023model, title={Model Selection Of Graph Signage Models Using Maximum Likelihood (Student Abstract)}, author={Brilliantova, Angelina and Bez{\'a}kov{\'a}, Ivona}, booktitle={Proceedings of the AAAI Conference on Artificial Intelligence}, volume={37}, number={13}, pages={16168--16169}, year={2023} }
[A-2022-01] Miller, Hannah. (2022). “Enumerating Nontrivial Knot Mosaics with SAT.”
Abstract: Mathematical knots are interesting topological objects. Using simple arcs, lines, and crossings drawn on eleven possible tiles, knot mosaics are a representation of knots on a mosaic board. Our contribution is using SAT solvers as a tool for enumerating nontrivial knot mosaics. By encoding constraints for local knot mosaic properties, we computationally reduce the search space by factors of up to 6600. Our future research directions include encoding constraints for global properties and using parallel SAT techniques to attack larger boards.
Publisher Site: AAAI
PDF (Requires Sign-In): PDF
Click for Bibtex
@inproceedings{miller2022enumerating, title = {Enumerating Nontrivial Knot Mosaics with SAT (Student Abstract)}, author = {Miller, Hannah}, booktitle = {Proceedings of the AAAI Conference on Artificial Intelligence}, year = {2022} }